A LASSO-derived developing and validating risk model for chronic total occlusion in Asian population before coronary angiography
Highlight box
Key findings
• The nomogram based on sex (male), LYM%, EF, Mb, non-high-density lipoprotein (non-HDL), and NT-proBNP could be used to predict CTO in CAD patients, enhancing the ability to predict their prognosis in clinical practice.
What is known and what is new?
• Despite several previous studies that have explored the predictors of high morbidity in coronary artery disease (CAD) and developed nomograms for CAD patients prior to coronary angiography (CAG), there is a lack of models available to predict chronic total occlusion (CTO).
• This study aims to construct a risk model and a nomogram to predict the likelihood of CTO before CAG.
What is the implication, and what should change now?
• Further research is needed to validate the efficacy of the nomogram in other populations.
Introduction
Chronic total occlusion (CTO) refers to a complete blockage in the coronary artery that has been present for over three months and is a common occurrence in patients with coronary artery disease (CAD) during a coronary angiography (CAG) procedure (1). Due to the complexity of the blocked lesion, CTO is considered to be the “last fortress” of percutaneous coronary intervention (PCI), which may result in lower procedural success rates and higher rates of restenosis. Studies have shown that around 20% of patients with CAD have at least one CTO lesion at the same time (2). Recent advancements in new crossing equipment, techniques, and improved operator skills have led to a gradual increase in procedural success (3). Effective CTO revascularization has been demonstrated to improve symptoms and enhance patients’ quality of life (4).
Stratifying patients’ risk for CTO has become increasingly important in the field of CAD management. Despite several studies having explored the predictors of high morbidity for CAD and developed relevant nomograms for patients prior to CAG, there is still a lack of models available for predicting CTO (5). The presence of multiple risk factors, such as male gender, smoking, chronic kidney disease, diabetes, and dyslipidemia, contribute to the development of CTO (6). Developing a predictive model based on preoperative demographic and laboratory data might help clinicians identify high-risk patients and improve treatment strategies.
In this study, we collected the clinical characteristics of patients diagnosed with CTO through CAG, developed a risk model based on the clinical data, and analyzed the factors influencing the diagnosis of CTO. We then evaluated the accuracy of the nomogram in predicting CTO patients, with the aim of providing additional useful information for the clinical prediction of the disease. We present the following article in accordance with the TRIPOD reporting checklist (available at https://cdt.amegroups.com/article/view/10.21037/cdt-22-466/rc).
Methods
Study cohort
In this retrospective study, we enrolled a total of 1,105 patients with unstable angina pectoris from Beijing Anzhen Hospital, Capital Medical University, between January 2019 and December 2019. This group served as the derivation cohort, with 235 patients diagnosed with CTO and 870 patients without CTO. Another group of 368 patients was enrolled between January 2020 and December 2020, consisting of 82 CTO patients and 286 non-CTO patients, and served as the validation cohort. All patients underwent CAG and were classified into either the CTO group (n=317) or the non-CTO group (n=1,156). CTO was diagnosed based on the presence of a grade 0 TIMI occlusion lasting for more than three months, as determined by the onset of angina pectoris, previous angiograms, or previous infarctions. Non-CTO was defined as a stenosis in a major coronary artery branch with a diameter reduction of over 50%. The study protocol was approved by the Human Research Ethics Committee of the Beijing Anzhen Hospital, Capital Medical University, Beijing, China (No. 2022177X), and the study adhered to the principles of the Declaration of Helsinki (revised in 2013). As a retrospective study, informed consent was waived by the Human Research Ethics Committee of the Beijing Anzhen Hospital, Capital Medical University, Beijing, China.
Data collection
The collected data included demographic information such as age, gender, and medical history, as well as laboratory test results such as lipid profile, glucose level, kidney function, and others. The echocardiogram results were also recorded to provide additional information on the patient’s heart function. All laboratory indices were analyzed using an automated biochemical analyzer to ensure accurate and consistent results.
Regression analysis
To identify factors associated with CTO prediction, we conducted a least absolute shrinkage and selection operator (LASSO) regression analysis using 10-fold cross-validation to select Lambda values. Subsequently, a multivariate logistic regression analysis was performed, including factors selected from the LASSO regression analysis with a P value of 0.05 as the inclusion criterion.
Model development
The final model was determined based on the receiver operating characteristic (ROC) curves and the Harrell concordance index (C-index). The resulting nomogram was created from this final model.
Performance of the nomogram
The external validation of the model was performed by using all the data from the validation cohort. The discriminatory performance was measured by the C-index. The calibration was assessed through a 1,000 re-sample bootstrapping calibration plot, which determined the level of agreement between the actual and predicted CTO cases according to the nomogram.
Clinical usage
The nomogram developed in this study was evaluated for its clinical utility using decision curve analysis (DCA), with statistical significance set at a P value less than 0.05. The methodology of DCA has been previously described in detail (7).
Statistical analysis
Continuous variables were reported as mean ± standard deviation (SD), while categorical variables were presented as numbers (percentage). The Mann-Whitney U test was used to assess differences in continuous variables between groups, while the Chi-square test or Fisher’s exact test was performed for categorical variables, depending on their sample size. A two-tailed P value of less than 0.05 was considered statistically significant. SPSS version 23.0 (SPSS Inc., Chicago, IL, USA) was used for all statistical analyses. Nomogram analysis and calibration curve were conducted using R software version 4.2.0 (http://www.R-project.org, R Foundation for Statistical Computing, Vienna, Austria).
Results
Population clinical characteristics of the derivation and validation cohort
Table 1 presents the clinical characteristics of the derivation (n=1,105) and validation cohort (n=368). There were no significant differences in demographic factors such as sex, age, current tobacco use, alcohol consumption, body mass index (BMI), systolic blood pressure (SP), and diastolic blood pressure (DP) between the two groups (all P>0.05). The incidence of hypertension, diabetes, dyslipidemia, and stroke was also not significantly different between both groups (all P>0.05). Echocardiography and laboratory indices did not show significant differences between the two groups (all P>0.05).
Table 1
Variable | Overall (n=1,473) | Derivation cohort (n=1,105) | Validation cohort (n=368) | P value |
---|---|---|---|---|
Clinical demographic | ||||
Gender (male), n (%) | 1,049 (71.2) | 795 (71.9) | 254 (69.0) | 0.28 |
Age (years) | 60.3±9.4 | 60.4±9.3 | 60.0±9.6 | 0.87 |
Current tobacco use, n (%) | 697 (47.3) | 512 (46.3) | 185 (50.3) | 0.19 |
Alcohol consumption, n (%) | 479 (32.5) | 370 (33.5) | 109 (29.6) | 0.17 |
BMI (kg/m2) | 25.9±3.2 | 25.8±3.2 | 26.0±3.3 | 0.47 |
SP (mmHg) | 131.6±16.8 | 131.8±16.8 | 130.9±16.7 | 0.30 |
DP (mmHg) | 78.0±10.9 | 78.0±10.8 | 77.9±11.0 | 0.97 |
Hypertension, n (%) | 940 (63.8) | 709 (64.2) | 231 (62.8) | 0.63 |
Diabetes mellitus, n (%) | 475 (32.2) | 358 (32.4) | 117 (31.8) | 0.83 |
Dyslipidemia, n (%) | 1,163 (79.0) | 877 (79.4) | 286 (77.7) | 0.50 |
Prior stroke, n (%) | 133 (9.0) | 93 (8.4) | 40 (10.9) | 0.16 |
Echocardiography | ||||
EF (%) | 62.6±7.1 | 62.8±7.0 | 62.3±7.5 | 0.42 |
LVEDD (mm) | 30.9±5.3 | 30.9±5.3 | 31.0±5.4 | 0.84 |
Laboratory indexes | ||||
WBC (×1012/L) | 7.1±2.0 | 7.1±2.0 | 7.1±1.9 | 0.62 |
RBC (×1012/L) | 4.5±0.5 | 4.5±0.5 | 4.6±0.5 | 0.66 |
Hb (g/L) | 139.6±16.0 | 139.7±15.7 | 139.4±16.6 | 0.92 |
HCT (%) | 40.4±4.3 | 40.4±4.3 | 40.4±4.5 | 0.99 |
PLT (×109/L) | 220.1±57.3 | 219.9±56.5 | 220.7±59.7 | 0.96 |
NE (×109/L) | 4.7±1.7 | 4.7±1.8 | 4.6±1.7 | 0.83 |
LYM (×109/L) | 1.9±0.6 | 1.9±0.6 | 1.9±0.6 | 0.71 |
LYM% | 0.3±0.1 | 0.3±0.1 | 0.3±0.1 | 0.92 |
MONO (×109/L) | 0.4±0.1 | 0.4±0.1 | 0.4±0.1 | 0.25 |
Glu (mmol/L) | 6.6±2.5 | 6.6±2.6 | 6.6±2.2 | 0.33 |
HbA1C (%) | 6.4±1.2 | 6.4±1.2 | 6.5±1.3 | 0.30 |
TG (mmol/L) | 1.7±1.0 | 1.7±1.0 | 1.7±1.0 | 0.08 |
TC (mmol/L) | 4.2±1.0 | 4.2±1.0 | 4.1±1.0 | 0.14 |
HDL (mmol/L) | 1.1±0.3 | 1.1±0.3 | 1.1±0.2 | 0.09 |
LDL (mmol/L) | 2.4±0.9 | 2.4±0.8 | 2.4±0.9 | 0.19 |
FFA (mmol/L) | 0.5±0.3 | 0.5±0.3 | 0.5±0.2 | 0.95 |
Non-HDL (mmol/L) | 3.0±1.0 | 3.0±1.0 | 3.0±1.0 | 0.34 |
sdLDL (mmol/L) | 0.7±0.4 | 0.7±0.4 | 0.7±0.4 | 0.93 |
Hcy (mmol/L) | 16.0±8.8 | 16.2±8.9 | 15.4±8.3 | 0.29 |
UA (mmol/L) | 340.9±86.0 | 340.7±86.5 | 341.6±84.7 | 0.95 |
Cr (μmol/L) | 74.4±18.4 | 74.3±18.5 | 74.6±18.2 | 0.75 |
eGFR (CKD-EPI) | 91.5±14.9 | 91.6±14.9 | 91.2±15.2 | 0.76 |
BNP (pg/mL) | 85.6±166.3 | 82.7±162.9 | 94.3±176.3 | 0.19 |
NT-proBNP (pg/mL) | 306.3±678.7 | 290.4±657.9 | 354.2±736.5 | 0.04 |
hs-CRP (mg/L) | 2.0±2.6 | 2.0±2.5 | 2.0±2.6 | 0.88 |
Mb (ng/mL) | 79.3±157.6 | 75.7±149.7 | 90.0±179.1 | 0.96 |
TnI (μg/L) | 0.2±0.8 | 0.2±0.8 | 0.3±0.8 | 0.84 |
Data are shown as n (%) or mean ± SD. BMI, body mass index; SP, systolic blood pressure; DP, diastolic blood pressure; EF, ejection fraction; LVEDD, left ventricular end diastolic diameter; WBC, white blood cell; RBC, red blood cell; Hb, hemoglobin; HCT, hematocrit; PLT, platelet; NE, neutrophil; LYM, lymphocyte; LYM%, lymphocyte percentage; MONO, monocyte; Glu, glucose; HbA1C, glycosylated hemoglobin type A1C; TG, triglyceride; TC, total cholesterol; HDL, high-density lipoprotein; LDL, low-density lipoprotein; FFA, free fatty acids; sdLDL, small dense low density lipoprotein; Hcy, homocysteine; UA, uric acid; Cr, creatinine; eGFR, estimated glomerular filtration rate; CKD-EPI, chronic kidney disease epidemiology collaboration; BNP, brain natriuretic peptide; NT-proBNP, N-terminal pro-B-type natriuretic peptide; hs-CRP, high-sensitivity C-reactive protein; Mb, myoglobin; TnI, troponin I; SD, standard deviation.
Comparison of the baseline characteristics of the derivation cohort between the CTO and non-CTO Groups
The baseline characteristics of the patients in the derivation cohort were described in Table 2. Compared to patients without CTO, patients with CTO were more likely to be male (85.5% vs. 68.3%; P<0.001) and smokers (55.7% vs. 43.8%; P=0.001). Surprisingly, they have lower SP (129.013±17.1 vs. 132.514±16.672 mmHg; P=0.001) and DP (76.796±11.163 vs. 78.336±10.697 mmHg; P=0.018), but the prevalence of hypertension is comparable (63.8% vs. 64.3%; P=0.904). Additionally, patients with CTO had a higher frequency of decreased ejection fraction (EF) (60%±9 % vs. 63%±6%; P<0.001) and an increased left ventricular end diastolic diameter (LVEDD) (85.5% vs. 68.3%; P<0.001). For the laboratory indices, the CTO group had significantly higher levels of white blood cell (WBC) (×1012/L) (7.673±2.365 vs. 6.97±1.922; P<0.001), neutrophil (NE) (×109/L) (5.258±2.156 vs. 4.504±1.613; P<0.001), glycosylated hemoglobin type A1C (HbA1C) (6.515%±1.204% vs. 6.384%±1.186%; P=0.040), triglyceride (TG) (164.294±102.854 vs. 147.373±86.962 mmol/L; P=0.007), high-density lipoprotein (HDL) (1.063±0.252 vs. 1.141±0.278 mmol/L; P<0.001), homocysteine (Hcy) (17.386±8.884 vs. 15.822±8.882 mmol/L; P=0.001), creatinine (Cr) (79.277±21.542 vs. 72.959±17.327 µmol/L; P<0.001), brain natriuretic peptide (BNP) (128.63±199.296 vs. 70.238±149.258 pg/mL; P<0.001), N-terminal pro-B-type natriuretic peptide (NT-proBNP) (518.068±889.899 vs. 228.9±564.628 pg/mL; P<0.001), and myoglobin (Mb) (109.876±174.986 vs. 66.443±140.778 ng/mL; P<0.001), but lower platelet (PLT) (×109/L) (212.809±57.495 vs. 221.878±56.051; P=0.016), lymphocyte percentage (LYM%) (0.248±0.078 vs. 0.283±0.080; P<0.001), high-density lipoprotein (HDL) (1.063±0.252 vs. 1.141±0.278 mmol/L; P<0.001), and troponin I (TnI) (0.187±0.763 vs. 0.216±0.812 µg/L; P=0.011).
Table 2
Variable | Overall (n=1,105) | CTO | P value | |
---|---|---|---|---|
Yes (n=235) | No (n=870) | |||
Clinical demographic | ||||
Gender (male) | 795 (71.9) | 201 (85.5) | 594 (68.3) | <0.01 |
Age (years) | 60.4±9.3 | 60.2±10.2 | 60.4±9.1 | 0.84 |
Current tobacco use, n (%) | 697 (63.1) | 131 (55.7) | 381 (43.8) | <0.01 |
Alcohol consumption, n (%) | 370 (33.5) | 81 (34.5) | 289 (33.2) | 0.72 |
BMI (kg/m2) | 25.8±3.2 | 26.0±3.2 | 25.8±3.2 | 0.29 |
SP (mmHg) | 131.8±16.8 | 129.0±17.1 | 132.5±16.7 | <0.01 |
DP (mmHg) | 78.0±10.8 | 76.8±11.2 | 78.3±10.7 | 0.02 |
Hypertension, n (%) | 709 (64.2) | 150 (63.8) | 559 (64.3) | 0.90 |
Diabetes mellitus, n (%) | 358 (32.4) | 87 (37.0) | 271 (31.1) | 0.09 |
Dyslipidemia, n (%) | 877 (79.4) | 184 (78.3) | 693 (79.7) | 0.65 |
Prior stroke, n (%) | 93 (8.4) | 20 (8.5) | 73 (8.4) | 0.95 |
Echocardiography | ||||
EF (%) | 62.8±7.0 | 60.2±9.2 | 63.5±6.1 | <0.01 |
LVEDD (mm) | 30.9±5.3 | 32.8±6.6 | 30.3±4.7 | <0.01 |
Laboratory indexes | ||||
WBC (×1012/L) | 7.1±2.0 | 7.7±2.4 | 7.0±1.9 | <0.01 |
RBC (×1012/L) | 4.5±0.5 | 4.5±0.6 | 4.6±0.5 | 0.29 |
Hb (g/L) | 139.7±15.7 | 137.8±16.7 | 140.2±15.4 | 0.18 |
PLT (×109/L) | 219.9±56.5 | 212.8±57.5 | 221.9±56.1 | 0.02 |
NE (×109/L) | 4.7±1.8 | 5.3±2.2 | 4.5±1.6 | <0.01 |
LYM (×109/L) | 1.9±0.6 | 1.8±0.7 | 1.9±0.6 | 0.07 |
LYM% | 0.3±0.1 | 0.2±0.1 | 0.3±0.1 | <0.01 |
MONO (×109/L) | 0.4±0.1 | 0.4±0.2 | 0.4±0.1 | 0.07 |
Glu (mmol/L) | 6.6±2.6 | 6.7±2.7 | 6.5±2.6 | 0.11 |
HbA1C (%) | 6.4±1.2 | 6.5±1.2 | 6.4±1.2 | 0.04 |
TG (mmol/L) | 151.0±90.8 | 164.3±102.9 | 147.4±87.0 | 0.01 |
TC (mmol/L) | 4.2±1.0 | 4.2±1.1 | 4.1±1.0 | 0.46 |
HDL (mmol/L) | 1.1±0.3 | 1.1±0.3 | 1.1±0.3 | <0.01 |
LDL (mmol/L) | 2.4±0.8 | 2.5±0.9 | 2.4±0.8 | 0.28 |
FFA (mmol/L) | 0.5±0.3 | 0.5±0.3 | 0.5±0.3 | 0.40 |
Non-HDL (mmol/L) | 3.0±1.0 | 3.1±1.1 | 3.0±0.9 | 0.11 |
sdLDL (mmol/L) | 0.7±0.4 | 0.7±0.4 | 0.7±0.4 | 0.64 |
Hcy (mmol/L) | 16.2±8.9 | 17.4±8.9 | 15.8±8.9 | <0.01 |
UA (mmol/L) | 340.7±86.5 | 350.6±90.8 | 338.0±85.1 | 0.12 |
Cr (μmol/L) | 74.3±18.5 | 79.3±21.5 | 73.0±17.3 | <0.01 |
eGFR (CKD-EPI) | 91.6±14.9 | 89.9±16.6 | 92.1±14.3 | 0.09 |
BNP (pg/mL) | 82.7±162.9 | 128.6±199.3 | 70.2±149.3 | <0.01 |
NT-proBNP (pg/mL) | 290.4±657.9 | 518.1±889.9 | 228.9±564.6 | <0.01 |
hs-CRP (mg/L) | 2.0±2.5 | 2.3±2.7 | 2.0±2.5 | 0.10 |
Mb (ng/mL) | 75.7±149.7 | 109.9±175.0 | 66.4±140.8 | <0.01 |
TnI (μg/L) | 0.2±0.8 | 0.2±0.8 | 0.2±0.8 | 0.01 |
Data are shown as n (%) or mean ± SD. BMI, body mass index; SP, systolic blood pressure; DP, diastolic blood pressure; EF, ejection fraction; LVEDD, left ventricular end diastolic diameter; WBC, white blood cell; RBC, red blood cell; Hb, hemoglobin; HCT, hematocrit; PLT, platelet; NE, neutrophil; LYM, lymphocyte; LYM%, lymphocyte percentage; MONO, monocyte; Glu, glucose; HbA1C, glycosylated hemoglobin type A1C; TG, triglyceride; TC, total cholesterol; HDL, high-density lipoprotein; LDL, low-density lipoprotein; FFA, free fatty acids; sdLDL, small dense low density lipoprotein; Hcy, homocysteine; UA, uric acid; Cr, creatinine; eGFR, estimated glomerular filtration rate; CKD-EPI, chronic kidney disease epidemiology collaboration; BNP, brain natriuretic peptide; NT-proBNP, N-terminal pro-B-type natriuretic peptide; hs-CRP, high-sensitivity C-reactive protein; Mb, myoglobin; TnI, troponin I; CTO, chronic total occlusion; SD, standard deviation.
LASSO regression analysis
The LASSO regression is a technique used to identify important predictors in a set of variables. In this case, 40 related variables were initially considered for the LASSO regression and the selection of predictors was performed through a 10-fold cross-validation process. The results showed that 10 potential factors with non-zero coefficients were selected as the predictors for CTO. As shown in Figure 1A, 10 potential factors with non-zero coefficients were selected: sex (coefficient, 0.0140), LYM% (coefficient, −0.9614), NE (coefficient, 0.0654), HDL (coefficient, −0.0807), LVEDD (coefficient, 0.0102), EF (coefficient, −0.0191), Cr (coefficient, 0.0004), Mb (coefficient, 0.0004), non-HDL (coefficient, 0.0236), and NT-proBNP (coefficient, 0.0002). Figure 1B depicted the changes in the LASSO coefficients.
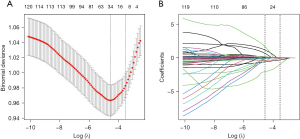
Multivariate logistic regression analysis
The multivariate logistic regression analysis, which aimed to identify independent indicators for predicting CTO before CAG, resulted in six variables being included in the final model out of ten selected by LASSO regression analysis in Table 3. The figure displayed the odds ratios (OR), 95% confidence intervals (CI), and P values for each variable. The variables were: sex (OR: 2.054; 95% CI: 1.419–2.972; P<0.001), LYM% (OR: 17.455; 95% CI: 1.912–159.342; P=0.011), NE (OR: 0.915; 95% CI: 0.831–1.007; P=0.069), HDL (OR: 1.959; 95% CI: 0.935–4.104; P=0.075), LVEDD (OR: 0.987; 95% CI: 0.953–1.022; P=0.466), EF (OR: 1.030; 95% CI: 1.003–1.057; P=0.029), Cr (OR: 0.998; 95% CI: 0.991–1.005; P=0.579), Mb (OR: 0.999; 95% CI: 0.998–1.000; P=0.006), non-HDL (OR: 0.792; 95% CI: 0.681–0.921; P=0.002), and NT-proBNP (OR: 1.000; 95% CI: 0.999–1.000; P=0.002).
Table 3
Variables | Multivariate logistic regression | ||||
---|---|---|---|---|---|
β | Standard error | OR | 95% CI | P | |
Gender (male) | 0.720 | 0.189 | 2.054 | 1.419–2.972 | 0.000 |
LYM% | 2.860 | 1.128 | 17.455 | 1.912–159.342 | 0.011 |
NE (×109/L) | −0.089 | 0.049 | 0.915 | 0.831–1.007 | 0.069 |
HDL (mmol/L) | 0.672 | 0.377 | 1.959 | 0.935–4.104 | 0.075 |
LVEDD (mm) | −0.013 | 0.018 | 0.987 | 0.953–1.022 | 0.466 |
EF (%) | 0.029 | 0.013 | 1.030 | 1.003–1.057 | 0.029 |
Cr (μmol/L) | −0.002 | 0.004 | 0.998 | 0.991–1.005 | 0.579 |
Mb (ng/mL) | −0.001 | 0.000 | 0.999 | 0.998–1.000 | 0.006 |
Non-HDL (mmol/L) | −0.233 | 0.077 | 0.792 | 0.681–0.921 | 0.002 |
NT-proBNP (pg/mL) | 0.000 | 0.000 | 1.000 | 0.999–1.000 | 0.002 |
CTO, chronic total occlusion; OR, odds ratios; CI, confidence interval; LYM%, lymphocyte percentage; NE, neutrophil; HDL, high-density lipoprotein; LVEDD, left ventricular end diastolic diameter; EF, ejection fraction; Cr, creatinine; Mb, myoglobin; non-HDL, non-high-density lipoprotein; NT-proBNP, N-terminal pro-B-type natriuretic peptide.
Construction of a novel nomogram scoring system
A novel nomogram scoring system was constructed using the results of the multivariate logistic regression analysis (Figure 2), which predicts the likelihood of CTO based on six independent predictors: male sex, LYM%, EF, Mb, non-HDL, and NT-proBNP. Each predictor was assigned a score, and the total score was plotted on the prediction axis to indicate the patient’s risk of having CTO. For example, a patient who is male (33 points), has a LYM% of 35% (31 points), an EF of 40% (58 points), Mb of 500 ng/mL (23 points), non-HDL of 3.0 mmol/L (30 points), and NT-proBNP of 1,000 pg/mL (12 points) would have a total score of 187 and an estimated 50% probability of having CTO.
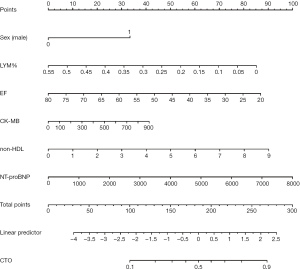
Evaluation and validation of the nomogram
To assess the validity of the model, a separate cohort of 368 patients from January to December 2020 was utilized for validation. The calibration of the model was evaluated through calibration curves for both the derivation (Figure 3A) and validation (Figure 3B) cohorts. The discrimination of the model was also analyzed using receiver operating characteristic (ROC) curve analysis, with the areas under the curve (AUC) being 0.744 and 0.729 for the derivation and validation cohorts, respectively (Figure 4). The findings revealed that the new nomogram had higher clinical utility, as demonstrated by the DCA curves (Figure 5).
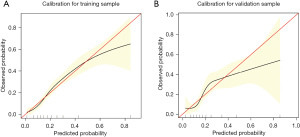
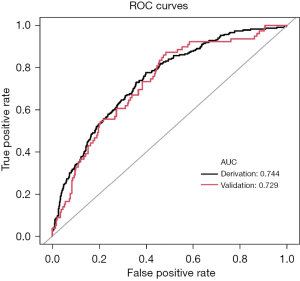
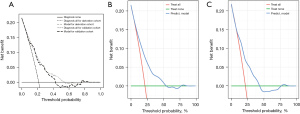
Discussion
In this study, various baseline demographic, echocardiography, and laboratory indices were gathered to explore the link between these predictors and the diagnosis of CTO. We developed a novel predictive model using LASSO regression analysis and multivariate logistic regression analysis, which was subsequently constructed and validated a nomogram. The resulting nomogram included sex (male), LYM%, EF, Mb, non-HDL, and NT-proBNP as predictors, and may provide important clues for the evaluation and diagnosis of CTO (Figure 2). Overall, our study offers a unique perspective on indicators that have a relatively high discriminatory power.
In our previous work, we developed a nomogram using logistic regression analysis (8). However, we found that LASSO regression analysis is a superior method to univariate logistic regression analysis as it accounts for multicollinearity between variables. Therefore, we replaced the univariate logistic regression analysis with LASSO regression analysis to identify better risk factors for CTO patients. This approach selected more relevant risk factors while using fewer variables.
Despite the well-established association between conventional risk factors and CAD, such as male sex and lipid metabolism, their role in the etiology of CTO remains unclear (9,10). Previous studies have primarily focused on individuals with stable CAD or acute coronary syndrome, with limited research on the risk factors for CTO (11). Furthermore, there is currently no reliable model for predicting the likelihood of CTO before CAG. By analyzing clinical and demographic data, we have gained new insights into the traditional risk factors associated with CTO and developed a nomogram that can provide relatively accurate predictions of CTO likelihood prior to CAG (12). This tool may be useful in effectively stratifying cardiovascular risks, as the recanalization of completely occluded vessels can be time-consuming and costly (13).
Despite the fact that CTO and CAD share similar pathological processes, the mechanism by which coronary arteries progress from stenosis to complete obstruction is not yet fully understood (14). Therefore, we recruited two groups of patients, in order to identify any differences in their clinical features and potentially shed some light on the distinct mechanisms underlying each condition (15).
In our study, we found that the occurrence of CTO in patients was linked to male sex and lipid metabolism, which is consistent with prior studies (16,17). However, it is noteworthy that predictive model developed using LASSO regression analysis included non-HDL rather than LDL, which has long been considered a secondary target of lipid-lowering therapy in CAD or in patients at high risk (18). Non-HDL includes several types of lipoprotein cholesterol, such as LDL, very low-density lipoprotein cholesterol (VLDL), intermediate-density lipoprotein cholesterol (IDL), lipoprotein(a), and chylomicrons (19). Increasingly, researchers have confirmed that new lipoprotein cholesterol, such as lipoprotein(a), plays a crucial part in CAD (20). The superiority of non-HDL over LDL in the nomogram may be due to its ability to reflect the features of CTO that distinguish it from CAD (21). Alternatively, LDL may be a common risk predictor for CAD and CTO, but non-HDL may be a more accurate marker reflecting the specific features of CTO that distinguish it from CAD (22).
Recent evidence suggested that cholesterol may also regulate immune cell function by activating immune signaling pathways, which can enhance antitumor effectiveness (23). In patients with CAD, lymphocytopenia has been associated with neurohormonal activation and is considered a marker for the physiologic stress response (24). A low percentage of lymphocytes has also been independently and significantly related to CAD and is considered an indicator of a poorer prognosis (25). Therefore, the inclusion of LYM% in the predictive nomogram is reasonable.
In addition to CAD-related risk factors, our study found that heart-related laboratory indexes, including Mb, EF, and NT-proBNP, were independent positive predictors of CTO. Undiagnosed acute myocardial infarction can cause CTO in some cases. CTO lesions may develop from an undetected acute myocardial infarction (26). Therefore, Mb, EF, and NT-proBNP may predict the likelihood of CTO in patients with CAD (27).
Based on the available information, we previously constructed a nomogram using logistic regression analysis (8). To address the issue of multicollinearity between variables, we used LASSO regression analysis instead of univariate logistic regression analysis, which enabled us to identify relevant risk factors for CTO patients. This approach allowed us to select a smaller number of variables while still maintaining the predictive power of the model.
In summary, the use of non-invasive testing has become more prevalent in the evaluation of patients with suspected illnesses (28). Consequently, the development of nomograms for various diseases that offer greater accuracy in predicting prognoses than conventional staging systems (29). This study has demonstrated the utility of a nomogram for improving our understanding of CTO risk factors and predicting the diagnosis of CTO prior to CAG.
Limitations
Our study has some limitations that should be taken into account. Firstly, the study only included Asian patients, and therefore the results may not be generalizable to other populations. Secondly, as a single-center retrospective study, selection bias may be present. However, the hospital where the study was conducted is the largest cardiac intervention center in China, with the largest number of CTO patients. Finally, although external validation was conducted, the validation cohort was smaller than the derivation cohort. Therefore, it is crucial to conduct multicenter validation studies with larger, diverse populations to obtain more robust conclusions and develop more accurate predictive models.
Conclusions
In conclusion, a clinical nomogram was developed and validated in this study that can predict the likelihood of CTO in patients with CAD with reasonable accuracy. The findings suggest that the nomogram may be a valuable tool for clinicians in developing a preoperative risk factor nomogram, which could aid in the detection of CTO in CAD patients. However, further validation studies involving larger, diverse patient populations from multiple centers are needed to confirm the accuracy and generalizability of the nomogram.
Acknowledgments
We would like to thank Jesse Luo for his help in polishing our paper.
Funding: The study was supported by the National Natural Science Fund of China (No. 82200441, No. 81970291, No. 82170344), Beijing Hospitals Authority Youth Programme (No. QML20230607), Young Elite Scientists Sponsorship Program by BAST (No. BYESS2023238), and the Major State Basic Research Development Program of China (973 Program, No. 2015CB554404).
Footnote
Reporting Checklist: The authors have completed the TRIPOD reporting checklist. Available at https://cdt.amegroups.com/article/view/10.21037/cdt-22-466/rc
Data Sharing Statement: Available at https://cdt.amegroups.com/article/view/10.21037/cdt-22-466/dss
Conflicts of Interest: All authors have completed the ICMJE uniform disclosure form (available at https://cdt.amegroups.com/article/view/10.21037/cdt-22-466/coif). The authors have no conflicts of interest to declare.
Ethical Statement: The authors are accountable for all aspects of the work in ensuring that questions related to the accuracy or integrity of any part of the work are appropriately investigated and resolved. The study protocol was approved by the Human Research Ethics Committee of the Beijing Anzhen Hospital, Capital Medical University, Beijing, China (No. 2022177X), and the study adheres all principles of the Declaration of Helsinki (as revised in 2013). Given the retrospective study design, informed consent is waived by the Human Research Ethics Committee of the Beijing Anzhen Hospital, Capital Medical University, Beijing, China.
Open Access Statement: This is an Open Access article distributed in accordance with the Creative Commons Attribution-NonCommercial-NoDerivs 4.0 International License (CC BY-NC-ND 4.0), which permits the non-commercial replication and distribution of the article with the strict proviso that no changes or edits are made and the original work is properly cited (including links to both the formal publication through the relevant DOI and the license). See: https://creativecommons.org/licenses/by-nc-nd/4.0/.
References
- Moses JW, Prasad M. Are All Total Occlusions Created Equal?: Striving for Complete Revascularization While Differentiating Apples From Oranges. J Am Coll Cardiol 2021;77:541-3. [Crossref] [PubMed]
- Assali M, Buda KG, Megaly M, et al. Update on chronic total occlusion percutaneous coronary intervention. Prog Cardiovasc Dis 2021;69:27-34. [Crossref] [PubMed]
- Picard F, Pighi M, Marquis-Gravel G, et al. The Ongoing Saga of the Evolution of Percutaneous Coronary Intervention: From Balloon Angioplasty to Recent Innovations to Future Prospects. Can J Cardiol 2022;38:S30-41. [Crossref] [PubMed]
- Zaman S, Berry C. Chronic Total Occlusions With Multivessel Disease; Does Bypass Grafting Beat Percutaneous Coronary Intervention? Circ Cardiovasc Interv 2022;15:e011786. [Crossref] [PubMed]
- Wu YJ, Mar GY, Wu MT, et al. A LASSO-Derived Risk Model for Subclinical CAC Progression in Asian Population With an Initial Score of Zero. Front Cardiovasc Med 2021;7:619798. [Crossref] [PubMed]
- Wu EB, Brilakis ES, Mashayekhi K, et al. Global Chronic Total Occlusion Crossing Algorithm: JACC State-of-the-Art Review. J Am Coll Cardiol 2021;78:840-53. [Crossref] [PubMed]
- Vickers AJ, Elkin EB. Decision curve analysis: a novel method for evaluating prediction models. Med Decis Making 2006;26:565-74. [Crossref] [PubMed]
- Shi YC, Zheng Z, Wang P, et al. Development and validation of a novel nomogram to predict chronic total occlusion before coronary angiography. Eur Rev Med Pharmacol Sci 2022;26:8011-21. [PubMed]
- Azzalini L, Karmpaliotis D, Santiago R, et al. Contemporary Issues in Chronic Total Occlusion Percutaneous Coronary Intervention. JACC Cardiovasc Interv 2022;15:1-21. [Crossref] [PubMed]
- Hoefer IE, Steffens S, Ala-Korpela M, et al. Novel methodologies for biomarker discovery in atherosclerosis. Eur Heart J 2015;36:2635-42. [Crossref] [PubMed]
- King SB 3rd, Gogas BD. Opening chronic coronary total occlusions: light in the tunnel or sleeping in Seattle? Eur Heart J 2018;39:2494-6. [Crossref] [PubMed]
- Shah SH, Kraus WE, Newgard CB. Metabolomic profiling for the identification of novel biomarkers and mechanisms related to common cardiovascular diseases: form and function. Circulation 2012;126:1110-20. [Crossref] [PubMed]
- Escárcega RO, Bailey D, Omar AF, et al. Treating Chronic Total Occlusions Successfully. JACC Cardiovasc Interv 2020;13:1834-5. [Crossref] [PubMed]
- Allahwala UK, Brilakis ES, Byrne J, et al. Applicability and Interpretation of Coronary Physiology in the Setting of a Chronic Total Occlusion. Circ Cardiovasc Interv 2019;12:e007813. [Crossref] [PubMed]
- Stone GW. Percutaneous Coronary Intervention of Chronic Total Occlusions: Conquering the Final Frontier. JACC Cardiovasc Interv 2018;11:1336-9. [Crossref] [PubMed]
- Brilakis ES, Burke MN. Chronic Total Occlusion Percutaneous Coronary Intervention: Bridging the Gap. JACC Cardiovasc Interv 2016;9:923-5. [Crossref] [PubMed]
- Shi Y, Zheng Z, Liu Y, et al. Leveraging Machine Learning Techniques to Forecast Chronic Total Occlusion before Coronary Angiography. J Clin Med 2022;11:6993. [Crossref] [PubMed]
- Ellims AH, Wong G, Weir JM, et al. Plasma lipidomic analysis predicts non-calcified coronary artery plaque in asymptomatic patients at intermediate risk of coronary artery disease. Eur Heart J Cardiovasc Imaging 2014;15:908-16. [Crossref] [PubMed]
- Zhu CG, Zhang Y, Xu RX, et al. Circulating non-HDL-C levels were more relevant to atherogenic lipoprotein subfractions compared with LDL-C in patients with stable coronary artery disease. J Clin Lipidol 2015;9:794-800. [Crossref] [PubMed]
- Bergmark BA, Marston NA, Bramson CR, et al. Effect of Vupanorsen on Non-High-Density Lipoprotein Cholesterol Levels in Statin-Treated Patients With Elevated Cholesterol: TRANSLATE-TIMI 70. Circulation 2022;145:1377-86. [Crossref] [PubMed]
- Wang B, Guo Z, Li H, et al. Non-HDL cholesterol paradox and effect of underlying malnutrition in patients with coronary artery disease: A 41,182 cohort study. Clin Nutr 2022;41:723-30. [Crossref] [PubMed]
- Nestel P, Loh WJ, Ward NC, et al. New Horizons: Revival of Lipoprotein (a) as a Risk Factor for Cardiovascular Disease. J Clin Endocrinol Metab 2022;107:e4281-94. [Crossref] [PubMed]
- Yang W, Bai Y, Xiong Y, et al. Potentiating the antitumour response of CD8(+) T cells by modulating cholesterol metabolism. Nature 2016;531:651-5. [Crossref] [PubMed]
- Chen SC, Yang YL, Wu CH, et al. Association between Preoperative Nutritional Status and Clinical Outcomes of Patients with Coronary Artery Disease Undergoing Percutaneous Coronary Intervention. Nutrients 2020;12:1295. [Crossref] [PubMed]
- Bian C, Wu Y, Shi Y, et al. Predictive value of the relative lymphocyte count in coronary heart disease. Heart Vessels 2010;25:469-73. [Crossref] [PubMed]
- Råmunddal T, Hoebers LP, Henriques JP, et al. Prognostic Impact of Chronic Total Occlusions: A Report From SCAAR (Swedish Coronary Angiography and Angioplasty Registry). JACC Cardiovasc Interv 2016;9:1535-44. [PubMed]
- Song L, Wang Y, Guan C, et al. Impact of Periprocedural Myocardial Injury and Infarction Definitions on Long-Term Mortality After Chronic Total Occlusion Percutaneous Coronary Intervention. Circ Cardiovasc Interv 2021;14:e010923. [Crossref] [PubMed]
- Huang Z, Tang J, Zheng S, et al. Prognostic significance of coronary artery calcium scoring and single-photon emission computed tomographic myocardial perfusion imaging on major adverse cardiac events in patients at low risk for suspected coronary artery disease. Acta Cardiol 2019;74:508-14. [Crossref] [PubMed]
- Thomas LE, O'Brien EC, Piccini JP, et al. Application of net reclassification index to non-nested and point-based risk prediction models: a review. Eur Heart J 2019;40:1880-7. [Crossref] [PubMed]