Mechanism of angiotensin-converting enzyme inhibitors in the treatment of dilated cardiomyopathy based on a protein interaction network and molecular docking
Highlight box
Key findings
• Fifteen hub genes and seven pathways on account of angiotensin-converting enzyme inhibitors (ACEIs) were found and may be useful in providing curative effects in Dilated cardiomyopathy (DCM).
What is known and what is new?
• ACEI has become a hot topic in the treatment of cardiovascular disease.
• This study primarily revealed that the preventive and curative effects of ACEI treatment on DCM could be realized through multiple targets and pathways, which helping understand the effect of ACEI-related genes on DCM.
What are the implications, and what should change now?
• Fifteen hub genes and seven pathways on account of ACEI may be used for the clinical treatment of DCM to help clinicians develop personalized therapy.
Introduction
Dilated cardiomyopathy (DCM) is one of the most frequent causes of heart failure and demand for heart transplantation worldwide, with a prevalence of 40 per 100,000 cases and an annual incidence of 7 per 100,000 cases (1,2). DCM exhibits differences in terms of race but not gender. Moreover, it accounts for approximately 60% of childhood cardiomyopathies, with the highest incidence among infants aged younger than 10 years old (3). The current treatment of DCM is mostly pharmacologic; β-blockers, renin-angiotensin-aldosterone system (RAAS) inhibitors, and mineralocorticoid receptor antagonists (MRAs) are the most effective agents for DCM treatment and are regarded as the cornerstones of DCM therapy (4). Angiotensin converting enzyme inhibitors (ACEIs) have antihypertensive effects, which can delay and reverse ventricular remodeling, prevent further development of myocardial hypertrophy, improve vascular endothelial function and cardiac function, and reduce the occurrence of arrhythmia (5-7). ACEIs can effectively block the RAAS and delay the progression of heart failure and myocardial fibrosis in DCM. ACEIs, which exert significant effects in the treatment of DCM (8), are the first-line drugs for DCM management in clinical practice. In recent years, clinical studies have identified an increasing number of adverse drug reactions and increased drug tolerance, leading to a poorer prognosis in patients with DCM (9,10). Therefore, an in-depth understanding of DCM pathogenesis is necessary to provide a foundation for the clinical development of new drug targets.
The development of DCM involves cardiac dysfunction, and under its influence, the occurrence of insufficient blood perfusion, water and sodium retention, activation of the neuroendocrine system, and ventricular remodeling (11-13). Advances in molecular biology research have also promoted the mechanistic studies of DCM at the genomic level, allowing for further exploration of the molecular changes in DCM from multiple aspects, including proteins, DNA, metabolism, epigenetic changes, and RNA, and has also simultaneously contributed to the discovery of new targets for drug design (14-16). Evidence indicates that transcriptomic changes in DCM cells may significantly increase the expression of inflammatory genes and affect the prognosis of DCM (17). In addition, genes related to metabolic activity are highly enriched in the cells of DCM patients (18). The above research suggests that an in-depth understanding of the altered gene levels in DCM is important for the optimization of DCM diagnosis and treatment.
In the present study, bioinformatics analysis tools were applied to analyze the genomic profile of DCM patients, aiming to provide new evidence about the genomic expression profile of DCM, the process of DCM formation, and find new targets for DCM drug therapy. We present this article in accordance with the STREGA reporting checklist (available at https://cdt.amegroups.com/article/view/10.21037/cdt-23-112/rc).
Methods
Sample source and data processing
This study was a retrospective study. Gene chip data named GSE42955, submitted by Molina-Navarro et al. (19), were selected by searching the Gene Expression Omnibus (GEO) database (https://www.ncbi.nlm.nih.gov/gds) for samples with “human (Homo sapiens)” as the study species. This dataset consisted of 29 samples from the HuGene-1_0-st Array gene expression microarray platform GPL6244, including five controls and 12 congestive DCMs. To obtain the significantly differentially expressed genes (DEGs), the DEGs between the DCM and control subgroups were analyzed using the eBayes function of “Limma” package (http://bioconductor.org/packages/release/bioc/html/limma.html) in R software with parameter proportion = 0.01, stdev.coef.lim = c(0.1,4), trend = FALSE, robust = FALSE, winsor.tail. P=c(0.05, 0.1), and filtered at a threshold of P<0.05 and |log fold change (FC)| >1.2. The P value is obtained from the moderated t-statistic with limma package. The study was conducted in accordance with the Declaration of Helsinki (as revised in 2013).
Identification of the target genes in drug therapy
Previous studies have reported that ACEI drugs have a significant influence on the treatment of DCM (8,20,21). Hence, this study focused on exploring the target genes of six ACEI drugs: Fosinopril, Enalapril, Ramipril, Benazepril, Perindopril, and Lisinopril. The structural information and biological activities of chemical substances could be acquired from PubChem (https://pubchem.ncbi.nlm.nih.gov/), from which, we acquired the two-dimensional (2D) molecular structures of Fosinopril, Enalapril, Ramipril, Benazepril, Perindopril, and Lisinopril. The prediction of drug-related targets in SwissTargetPrediction (http://www.swisstargetprediction.ch) was based on ligand-based pharmacophore models and structural similarity. We then obtained the target genes of the drug from the Comparative Toxicogenomics Database (CTD, http://ctdbase.org/). Finally, the genes from both SwissTargetPrediction and CTD databases were integrated together to obtain combined results.
PPI construction and topology analysis
DEGs were imported into the STRING database (https://string db.org/, version 11.5), which is one of the most data-rich and widely applied databases in the study of protein interactions and helps to mine hub regulatory genes. The STRING database is designed to collect, evaluate, and integrate publicly available protein-protein interaction (PPI) data sources and to complement them with computer predictions (22), and helped us ascertain the drug interactions with DCM cross-targets, with PPI scores <0.4 signifying low confidence, 0.4–0.7 reflecting moderate confidence, and >0.7 denoting high confidence. In this study, genes with PPI scores <0.4 were removed. Cytoscape software (international consortium of open source developers, http://cytoscape.org/, version 3.7.2), which graphically displays the network, could at the same time perform analysis and editing (23). Herein, we applied Cytoscape to display the PPI content.
The topological parameters of all nodes in the PPI network described above were calculated using Cytoscape. For each node in the interaction network, three parameters could reflect its topological characteristics. Specifically, “Degree” is defined as the number of edges of node i; “Intermediate centrality” refers to the shortest paths running through the node between node pairs; and “Closeness centrality” denotes the reciprocal sum of distances from node i to other nodes. In general, the node with the highest ranking based on the degree, intermediate centrality, and closeness centrality parameters can be identified as the core objective.
Functional enrichment analysis and module-based network analysis
The function of DEGs was further explored through Gene Ontology (GO) and Kyoto Encyclopedia of Genes and Genomes (KEGG) enrichment analyses using the “WebGestaltR” R package (24), with a threshold false discovery rate (FDR) <0.05 detecting by Benjamini-Hochberg (BH). The paraments for WebGestaltR is geneFile = ‘interestingGenes.txt’, refFile = system.file(“extdata”, “referenceGenes.txt”, package = “WebGestaltR”) outputDirectory <- getwd(), enrichMethod = “ORA”, organism = “hsapiens”, enrichDatabase = c(‘pathway_KEGG’, ‘geneontology_Biological_Process’, ‘geneontology_Cellular_Component’, ‘geneontology_Molecular_Function’), minNum = 10, maxNum = 500, sigMethod = ‘fdr’, fdrMethod = ‘BH’, fdrThr = 0.5, topThr = 10, reportNum = 20, perNum = 1000, interestGeneFile = geneFile, interestGeneType = “genesymbol”, referenceGeneFile = refFile, referenceGeneType = “genesymbol”, isOutput = F, outputDirectory = outputDirectory, projectName = NULL. Based on the target-regulated biological processes, the drug mechanisms in DCM could be analyzed.
Module-based network analysis was performed using Metascape (UCSD, USA, www.metascape.org/) Similarly to GO and KEGG, it can also realize gene function annotation (25).
Correlation analysis of hub genes with immunity and pathways
Three scores (StromalScore, ImmuneScore, and ESTIMATEScore) of the GSE42955 dataset were evaluated using the “ESTIMATE” R package with parameter is platform = ‘affymetrix’. (26). The scores of 28 immune cell types were evaluated by utilizing the “ssGSEA” package with parameter is method = ‘ssgsea’, min.sz = 10, max.sz = 500, verbose = TRUE. The Pearson correlation coefficients of immune scores with hub genes were then calculated and visualized (27). To explore the correlation between hub genes and pathways, we first obtained HALLMARK’s h.all.v7.4.symbols.gmt pathways and from the gene set enrichment analysis (GSEA) website, and then performed single-sample GSEA (ssGSEA) to determine the scores of these pathways, followed by calculation of the Pearson correlation coefficients of the pathway scores with hub genes and visualization using corr.test function of R psych package (Default parameter: use = “pairwise”, method = “pearson”, adjust = “holm”, alpha = 0.05, ci = TRUE, minlength = 5). P value was determined by “holm” method.
Molecular docking
Molecular docking is a method of drug design that is achieved based on the characteristics of receptors and the interaction between the drug molecule and the receptor. As a theoretical simulation method in the study of the interaction between molecules (e.g., ligand and receptor), molecular docking is applied to predict their binding modes and affinity, and has therefore become an important technique in the field of computer-aided drug research (28,29). Autodock Vina software (Molecular Graphics Lab, The Scripps Research Institute, USA) was used for molecular docking experiments. First, AutoDockTools 1.5.6 was utilized to prepare all of the input files. The Protein Data Bank (pdb) files of the proteins were downloaded from Protein Data Bank with PDB ID 6SUK. All potassium ions, protein B chains, and water molecules were removed, and instead, polar hydrogens were added into the system. The charge of the zinc ion of the Protein Data Bank, Partial Charge (Q), & Atom Type (T) (PDBQT) file of the receptor protein was changed to +2.0. During molecular docking, the coordinates of the grid in each X, Y, and Z direction were −19.5 Å, 74.5 Å, and 34.8 Å, respectively, and the lengths in each XYZ direction were 20 Å, respectively.
Statistical analysis
All statistical analyses were performed using R (version 4.2.1, Bell Laboratories, USA, https://www.r-project.org/). Venn diagram was presented by upset function in R UpSet package with parameter is nintersects = 30, mb.ratio = c(0.5, 0.5), order.by = c(“freq”, “degree”), decreasing = c(TRUE, FALSE). Strong correlation indicated by r>0.9. The log-rank test was performed in the Kaplan-Meier survival analysis. Pearson correlation coefficients was used to analysis the correlation between the pathway scores with hub genes. The parameters were considered default if there was no special indication. P<0.05 was considered statistically significant.
Results
Acquisition of the DEGs and construction of the PPI network
DEGs of the DCM and control samples in the GSE42955 dataset were obtained by conducting differential expression analysis. Then, the obtained genes were intersected with the target genes of six drugs (Enalapril, Benazepril, Lisinopril, Ramipril, Perindopril, and Fosinopril), and we acquired a total of 62 intersected genes. These 62 target genes of ACEIs and the DEGs of GSE42955 are shown in Figure 1A in the form of upset plots, which indicated these genes may be associated with the occurrence and development of DCM. We also plotted the PPI network of the drug and target genes (Figure 1B).
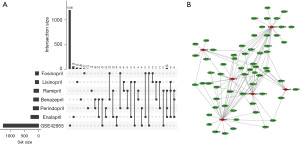
Based on the targets’ scores in the PPI, the minimum required interaction score was set to 0.4. In this way, we obtained a total of 71 targets. Based on the PPI network, we imported the data into Cytoscape software and retained 62 targets in the PPI network (Figure 2). Next, we calculated the topological characteristics of all nodes in the PPI network using Cytoscape software. According to the topological parameters of intermediate centrality, degree, and closeness centrality, we identified the 15 highest-ranked central genes (Table 1): namely, TNF, AKT1, VEGFA, CCL2, IL6, CCND1, erb-b2 receptor tyrosine kinase 2 (ERBB2), signal transducer and activator of transcription 3 (STAT3), toll like receptor 4 (TLR4), vascular cell adhesion molecule 1 (VCAM1), collagen type I alpha 1 chain (COL1A1), intercellular adhesion molecule 1 (ICAM1), integrin subunit beta 3 (ITGB3), transforming growth factor beta receptor 2 (TGFBR2), and nuclear factor kappa B subunit 1 (NFKB1).
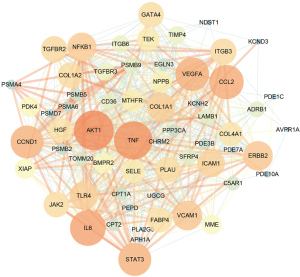
Table 1
Number | Name | Degree | Betweenness Centrality | Closeness Centrality |
---|---|---|---|---|
1 | AKT1 | 51 | 0.09347377 | 0.85915493 |
2 | TNF | 49 | 0.08969674 | 0.83561644 |
3 | IL6 | 45 | 0.04122689 | 0.79220779 |
4 | CCL2 | 43 | 0.03350728 | 0.7721519 |
5 | VEGFA | 42 | 0.03167331 | 0.75308642 |
6 | CCND1 | 41 | 0.04876528 | 0.73493976 |
7 | STAT3 | 41 | 0.02745317 | 0.75308642 |
8 | ERBB2 | 36 | 0.01914102 | 0.70930233 |
9 | VCAM1 | 36 | 0.00886999 | 0.70114943 |
10 | NFKB1 | 35 | 0.03477308 | 0.69318182 |
11 | TLR4 | 34 | 0.01099059 | 0.69318182 |
12 | COL1A1 | 34 | 0.00823632 | 0.68539326 |
13 | ICAM1 | 33 | 0.00645025 | 0.67777778 |
14 | ITGB3 | 31 | 0.01693205 | 0.65591398 |
15 | TGFBR2 | 31 | 0.01137074 | 0.66304348 |
PPI, protein-protein interaction.
Enrichment analysis and module-based network analysis
Further exploration of the function of DEGs was realized by performing GO and KEGG enrichment analysis on these 71 targets. GO enrichment analysis comprised biological process (BP), cellular component (CC), and molecular function (MF), which are shown in Figure 3A-3C, respectively. The KEGG enrichment analysis is displayed in Figure 3D. Through the GO and KEGG analyses, it could be found that the 71 target genes were mainly related to T helper type 17 (Th17) cell differentiation as well as the nuclear factor kappa-B (NF-kappa B), interleukin 17 (IL-17), mitogen-activated protein kinase (MAPK), tumor necrosis factor (TNF), phosphatidylinositol 3 kinase (PI3K)/protein kinaseB (AKT) (PI3K-Akt) and Toll-like receptor signaling pathways.
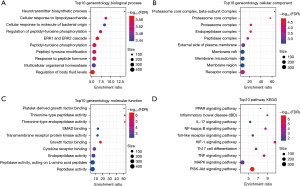
The target genes’ functions were validated by Metascape using a module-based network analysis. To find the core genes in the potential target network and annotate the biological functions of each group, the molecular complex detection (MCODE) algorithm was applied. We extracted network modules using 30 of the 62 target genes (Figure 4), and the obtained modules were then analyzed by pathway and enrichment analysis. GO enrichment analysis demonstrated that these genes were mainly enriched in the cell membrane receptors and regions, the regulation of cellular humoral stress, cytokines, growth factors, peptide molecular activities, etc. From the KEGG results, these modules were found to be mainly associated with the JAK-STAT, NF-kappa B, PI3K-Akt, IL-17, MAPK, and other signaling pathways.
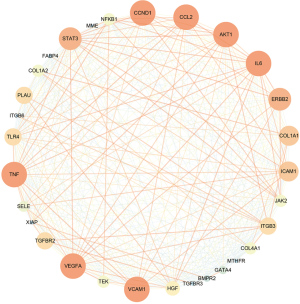
Correlation of hub genes with immunity and pathways
To explore whether the hub genes were associated with immunity, three scores (StromalScore, ImmuneScore, and ESTIMATEScore) from the GSE42955 dataset were evaluated using the “ESTIMATE” R package and those of 28 immune cells were analyzed using the “ssGSEA” package. We then calculated and visualized the Pearson correlation coefficients of the immune scores using 15 hub genes. Most of the immune scores were found to be negatively related to VEGFA, CCND1, and IL6 expression levels, while the remaining 12 gene expressions showed positive correlations with most of the immune scores (Figure 5, purple means positive correlation, green means negative correlation). The pathway correlation analysis results demonstrated that STAT3 was most strongly correlated with the P53 signaling pathway (r=0.91); moreover, it was also significantly positively correlated with the inflammatory response and the IL6-JAK-STAT3 signaling pathway (r=0.89, 0.88). VEGFA was significantly negatively correlated with the P53 signaling pathway, the inflammatory response, and the IL6-JAK-STAT3 signaling pathway (r=−0.68, −0.66, −0.58, respectively) (Figure 6).
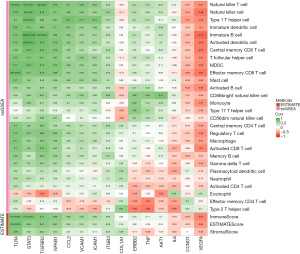
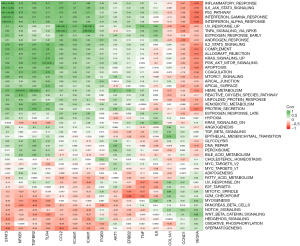
Targeted genes were indirectly or directly related to DCM phenotype
Next, we evaluated the direct and indirect associations between anti-DCM drug target genes and disease phenotypes using the VarElect algorithm. Correlation analyses between the potential therapeutic targets and DCM phenotypes demonstrated that among 62 potential targets, 28 were indirectly associated with DCM phenotypes, whereas 34 were directly associated with DCM phenotypes (Figure 7). Table 2 lists the top 20 most relevant target genes; specifically, NPPB, JAK2, CPT2, CHRM2, ADRB1, TNF, KCNH2, GATA4, CCL2, STAT3, VCAM1, IL6, ICAM1, COL1A1, TLR4, and NFKB1, were directly correlated with the phenotype, while LAMB1, VEGFA, Tie-2, and ITGB3 were indirectly correlated with the phenotype.
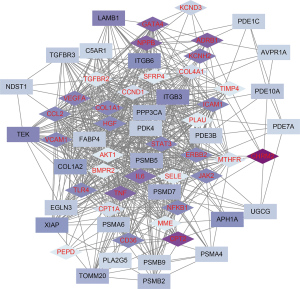
Table 2
Number | Symbol | Description | Type | Direct/indirect | Score | Average disease causing likelihood |
---|---|---|---|---|---|---|
1 | CHRM2 | Cholinergic Receptor Muscarinic 2 | Protein | Direct | 27.52 | 63.1 |
2 | CPT2 | Carnitine Palmitoyltransferase 2 | Protein | Direct | 18.01 | 17.5 |
3 | NPPB | Natriuretic Peptide B | Protein | Direct | 13.8 | 46.2 |
4 | ADRB1 | Adrenoceptor Beta 1 | Protein | Direct | 13.62 | 63.8 |
5 | TNF | Tumor Necrosis Factor | Protein | Direct | 13.25 | 70.4 |
6 | GATA4 | GATA Binding Protein 4 | Protein | Direct | 12.86 | 33.8 |
7 | IL6 | Interleukin 6 | Protein | Direct | 11.13 | 56.1 |
8 | KCNH2 | Potassium Voltage-Gated Channel Subfamily H Member 2 | Protein | Direct | 10.2 | 51.1 |
9 | LAMB1 | Laminin Subunit Beta 1 | Protein | Indirect | 9.03 | 53.4 |
10 | VEGFA | Vascular Endothelial Growth Factor A | Protein | Direct | 8.83 | 64.8 |
11 | Tie-2 | Tyrosine-protein kinase receptor (Tie-2) Receptor Tyrosine Kinase | Protein | Indirect | 8.42 | 56.5 |
12 | STAT3 | Signal Transducer And Activator Of Transcription 3 | Protein | Direct | 8.27 | 78.9 |
13 | VCAM1 | Vascular Cell Adhesion Molecule 1 | Protein | Direct | 8.12 | 53.5 |
14 | ICAM1 | Intercellular Adhesion Molecule 1 | Protein | Direct | 8.07 | 20.1 |
15 | CCL2 | C-C Motif Chemokine Ligand 2 | Protein | Direct | 8.04 | 69.2 |
16 | ITGB3 | Integrin Subunit Beta 3 | Protein | Indirect | 8.01 | 58.7 |
17 | COL1A1 | Collagen Type I Alpha 1 Chain | Protein | Direct | 8 | 75.8 |
18 | NFKB1 | Nuclear Factor Kappa B Subunit 1 | Protein | Direct | 7.95 | 72.4 |
19 | JAK2 | Janus Kinase 2 | Protein | Direct | 7.91 | 71.6 |
20 | TLR4 | Toll Like Receptor 4 | Protein | Direct | 7.89 | 25.4 |
DCM, dilated cardiomyopathy.
Molecular docking
We identified 15 hub genes using the PPI and Cytoscape, and the most relevant genes to the DCM phenotype by VarElect, among which TNF was screened as the top hub gene that was directly relevant and scored high in the VarElect results. TNF is the target gene of four drugs (Enalapril, Fosinopril, Benazepril, and Perindopril), which were therefore selected for molecular docking with gene TNF (Table 3). Collectively, compound Benazepril had the highest molecular docking score and was regarded as a relatively more promising TNF inhibitor. Compound DB03742 bound well to the active pocket of TNF proteins, with a relatively high molecular docking score of −8.3 kcal/mol. Moreover, the compound could support hydrogen-bonding interactions with SER60 in the B chain of TNF proteins and hydrophobic interactions with LEU57, TYR59, and TYR119 in the A chain and with LEU57 and TYR59 in the B chain (Figure 8A-8C). The relatively high molecular docking score and the ability of the compound Benazepril to produce favorable interactions with TNF proteins suggested that this compound has great potential to serve as an inhibitor of TNF.
Table 3
Compound | AutoDock Vina score | H-Bond interactions | Hydrophobic interactions |
---|---|---|---|
Benazepril | −8.3 | SER60(B) | LEU57(A), TYR59(A), TYR119(A), LEU57(B), TYR59(B) |
Enalapril | −7.1 | GLY121(A) | TYR59(A), TYR59(B) |
Fosinopril | −7.2 | TYR59(A), TYR151(A), TYR119(A), TYR59(B) | |
Perindopril | −6.4 | LEU120(A), SER60(B) | LEU57(A), TYR59(A), TYR59(B), TYR119(B) |
TNF, tumor necrosis factor; SER, serine; LEU, leucine; TYR, tryptophan; GLY, glycine.
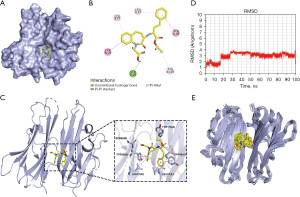
The root-mean-squared deviation (RMSD) values of Benazepril binding to the active site of TNF protein during the 100 ns molecular dynamics (MD) simulation (Figure 8D) showed that the RMSD values of Benazepril were generally stable at around 3 Å. The RMSD values of Benazepril demonstrated two significant increases at 17 ns and 27 ns in the first 30 ns and then remained relatively stable for the next 70 ns. This can also be seen in Figure 8E, in which compound Benazepril showed stable binding to the TNF protein active site and maintained a relatively stable conformation. Although this study utilized semi-flexible docking (that is, the structure of the protein was rigid with only a flexible conformation of the ligand molecule during the docking process), the structure of the protein in the existing crystal 2AZ5 was a structure that can better adapt to its original crystal. Therefore, at the early stage of MD, the structures of the protein and ligand required mutual adaptation to reach a low-energy conformation, which also explained the period of significant fluctuation in the RMSD value of compound Benazepril at the early stage of MD simulation. In general, compound Benazepril exhibited relative stability in binding to the active site of TNF proteins, further suggesting that Benazepril is a very promising potential inhibitor of the TNF protein.
Discussion
ACEIs block RAAS by inhibiting angiotensin-converting enzyme (ACE) activity, but induce ACE-independent enzyme-mediated angiotensin II and aldosterone production (30,31). Activation of RAAS plays a key role in the development of left ventricular remodeling (21). To date, ACEI has been studied in patients with ischemic heart failure (32). In this study, 15 core genes were identified based on ACEI target genes for DCM samples, which were enriched in a variety of inflammatory pathways, further demonstrating that the compound Benazepril had a good interaction with TNF protein.
Previous studies have demonstrated the significance of hub genes in the occurrence, development, treatment, and prognosis of DCM (33-35). The present study screened AKT1, TNF, IL6, CCL2, and VEGFA as the most important genes for DCM. A previous research has shown abnormal mitral flow pattern and reduced systolic function in Akt1-deficient fetuses, while in vitro micro-computed tomography (µCT) and histological studies have revealed structural alterations in these fetuses, such as DCM and ventricular septal defects (36). There is substantial evidence showing that DCM is associated with the induction of cytokines and chemokines and that B-cell function, including frequency and proliferation, is significantly elevated in patients with DCM. TNF-α protein secretion and messenger RNA (mRNA) levels were elevated in lipopolysaccharide (LPS)-stimulated B cells isolated from DCM patients. The frequency of TNF-α-secreting B cells was negatively correlated with left ventricular ejection fraction (LVEF) in all DCM patients, suggesting that TNF-α-secreting B cells may be involved in myocardial fibrosis, which also revealed a novel pathogenic mechanism of B cells in DCM (37). A significantly lower follicular regulatory T cells (Tfr) cell rate and Tfr/follicular helper T cell (Tfh) ratio and a significantly higher Tfh rate were detected by flow cytometry in patients with DCM compared with normal controls. Correlation analysis showed that the Tfr rate in DCM patients was positively correlated with the LVEF but negatively correlated with N-terminal brain natriuretic peptide (NT-proBNP) levels. In DCM patients, lower forkhead box P3 (Foxp3), higher mRNA expression levels of B cell lymphoma 6 (Bcl-6), programmed cell death 1 (PD-1), and inducible T cell costimulator (ICOS) were found; moreover, these patients also demonstrated significantly higher levels of IL-21, TNF-α, and plasma IL-6 (38). Enalapril, Benazepril, Lisinopril, Ramipril, Perindopril, and Fosinopril are drugs that can treat high blood pressure and heart failure (39). Our study found that Enalapril, Fosinopril, Benazepril, and Perindopril can play a role in targeting TNF, among which Benazepril has the strongest binding degree with TNF, which may be TNF inhibitor drugs. CCL2 has been shown to be significantly and consistently upregulated in an experimental model of DCM (40). In heart failure, CCL2 is localized to cardiomyocytes, monocytes, vascular smooth muscle cells, and endothelial cells (41,42), and its induction may involve the activation of TLR signaling, neurohumoral cascades, or pro-inflammatory cytokine-mediated pathways, which are associated with poor myocardial remodeling, dysfunction, and fibrosis (43). VEGFA has long been considered a potential vascular and proliferative regenerative therapeutic agent for patients with ischemic heart disease; for instance, VEGFA mRNA exhibits a more transient pharmacokinetic profile but could better unlock the pharmacotherapeutic potential of VEGFA (44).
By analyzing the potential biological functions of the hub DEGs, it could be seen from the results of GO and KEGG enrichment analyses that differential gene functions were mainly expressed in the NF-kappa B, IL-17, PI3K-Akt, MAPK, JAK-STAT, and other signaling pathways. This suggested that the DEGs may be implicated in DCM progression and drug effects may be released via the above pathways. The results of the current work were also consistent with previously published studies (45,46), together offering a solid basis for the development of new drug targets for DCM.
Previous studies have validated the role of immunity in DCM development and treatment (47-49). Our study detected significant correlations between VEGFA, STAT3, and the P53 signaling pathway, the IL6-JAK-STAT3 signaling pathway, and the inflammatory response, illustrating the great potential of these signaling pathways to contribute to the development of DCM drugs. Adaptive immune activation requires highly specific cooperation between different antigen-specific receptors and antigen-presenting cells on T cells. In vivo studies have demonstrated that culturing of sensitized T cells could be achieved in approximately 50% of endomyocardial myocardial biopsies derived from DCM patients or those with myocarditis. The significance of the cellular immune response in human disease has been demonstrated by transferring peripheral blood leukocytes from chronic myocarditis patients into severely combined immunodeficient mice, in which left ventricular functional impairment and human cellular infiltration of the myocardium could be developed within 60 days. These findings confirmed the importance and presence of cellular immune responses in DCM pathogenesis (50), and are supportive of evidence-based medicine for the design of immune-related target drugs in clinical practice (51).
Limitations
However, this study had certain limitations that should be noted and considered. Firstly, although the use of a wide variety of bioinformatics tools and their rapid development helped predict the potential hub molecules and pathways and narrow the scope of research, the credibility of final research results is still dependent on validation by studies performed in basic and clinical settings. Moreover, although the database used in this study for the functional and signaling pathway enrichment analyses was comprehensive, but the small samples maybe affect the reproducibility of our findings, and a slow update did not include the newest research results, which may limit the universality and reproducibility of our findings. Although we do molecular docking experiments at the molecular level to maximize the validity of the data. In addition, the results were obtained based on the raw bioinformatics algorithm, which requires further laboratory and clinical evidence support. Last but not least, we did not consider possible confounding factors for ACEI usage, including long term/short term medications, co-morbidities- substance use, and hereditary linkage.
Conclusions
This study revealed that ACEI treatment of DCM may exert its preventive and curative effects via multiple pathways and targets, the mechanism of which was related to TNF, AKT1, CCL2, IL6, CCND1, VEGFA, and some other genes. The mechanism of action also involved the IL-17, TNF, PI3K-Akt, NF-kappa B, Toll-like receptor, and MAPK signaling pathways, as well as Th17 cell differentiation. The study is the first report of both a genetic association and a replication effort.
Acknowledgments
Funding: The research was funded by the Shenzhen Traditional Chinese Medicine Hospital “3030 Program” Chinese Medicine Clinical Research Project (No. G3030202128).
Footnote
Reporting Checklist: The authors have completed the STREGA reporting checklist. Available at https://cdt.amegroups.com/article/view/10.21037/cdt-23-112/rc
Peer Review File: Available at https://cdt.amegroups.com/article/view/10.21037/cdt-23-112/prf
Conflicts of Interest: All authors have completed the ICMJE uniform disclosure form (available at https://cdt.amegroups.com/article/view/10.21037/cdt-23-112/coif). The authors have no conflicts of interest to declare.
Ethical Statement: The authors are accountable for all aspects of the work in ensuring that questions related to the accuracy or integrity of any part of the work are appropriately investigated and resolved. The study was conducted in accordance with the Declaration of Helsinki (as revised in 2013).
Open Access Statement: This is an Open Access article distributed in accordance with the Creative Commons Attribution-NonCommercial-NoDerivs 4.0 International License (CC BY-NC-ND 4.0), which permits the non-commercial replication and distribution of the article with the strict proviso that no changes or edits are made and the original work is properly cited (including links to both the formal publication through the relevant DOI and the license). See: https://creativecommons.org/licenses/by-nc-nd/4.0/.
References
- Maron BJ, Towbin JA, Thiene G, et al. Contemporary definitions and classification of the cardiomyopathies: an American Heart Association Scientific Statement from the Council on Clinical Cardiology, Heart Failure and Transplantation Committee; Quality of Care and Outcomes Research and Functional Genomics and Translational Biology Interdisciplinary Working Groups; and Council on Epidemiology and Prevention. Circulation 2006;113:1807-16. [Crossref] [PubMed]
- Taylor MR, Carniel E, Mestroni L. Cardiomyopathy, familial dilated. Orphanet J Rare Dis 2006;1:27. [Crossref] [PubMed]
- Nugent AW, Daubeney PE, Chondros P, et al. The epidemiology of childhood cardiomyopathy in Australia. N Engl J Med 2003;348:1639-46. [Crossref] [PubMed]
- Ilieșiu AM, Hodorogea AS. Treatment of Heart Failure with Preserved Ejection Fraction. Adv Exp Med Biol 2018;1067:67-87. [Crossref] [PubMed]
- Lo KB, Toroghi HM, Salacup G, et al. Angiotensin converting enzyme inhibitors and angiotensin receptor blockers in acute heart failure: invasive hemodynamic parameters and clinical outcomes. Rev Cardiovasc Med 2021;22:199-206. [Crossref] [PubMed]
- Messerli FH, Bangalore S, Bavishi C, et al. Angiotensin-Converting Enzyme Inhibitors in Hypertension: To Use or Not to Use? J Am Coll Cardiol 2018;71:1474-82. [Crossref] [PubMed]
- Mungmunpuntipantip R, Wiwanitkit V. Angiotensin-converting enzyme inhibitors and angiotensin receptor blockers and clinical outcomes of COVID-19. Ann Palliat Med 2022;11:1603-4. [Crossref] [PubMed]
- Reichart D, Magnussen C, Zeller T, et al. Dilated cardiomyopathy: from epidemiologic to genetic phenotypes: A translational review of current literature. J Intern Med 2019;286:362-72. [Crossref] [PubMed]
- Balmforth C, Simpson J, Shen L, et al. Outcomes and Effect of Treatment According to Etiology in HFrEF: An Analysis of PARADIGM-HF. JACC Heart Fail 2019;7:457-65. [Crossref] [PubMed]
- Zhong JH, Chen XP, Zeng CF, et al. Effect of benazepril on heart rate turbulence in patients with dilated cardiomyopathy. Clin Exp Pharmacol Physiol 2007;34:612-6. [Crossref] [PubMed]
- Tanai E, Frantz S. Pathophysiology of Heart Failure. Compr Physiol 2015;6:187-214. [Crossref] [PubMed]
- McMurray JJ, Pfeffer MA. Heart failure. Lancet 2005;365:1877-89. [Crossref] [PubMed]
- Yin J, Lu X, Qian Z, et al. New insights into the pathogenesis and treatment of sarcopenia in chronic heart failure. Theranostics 2019;9:4019-29. [Crossref] [PubMed]
- Kolur V, Vastrad B, Vastrad C, et al. Identification of candidate biomarkers and therapeutic agents for heart failure by bioinformatics analysis. BMC Cardiovasc Disord 2021;21:329. [Crossref] [PubMed]
- Liu X, Xu S, Li Y, et al. Identification of CALU and PALLD as Potential Biomarkers Associated With Immune Infiltration in Heart Failure. Front Cardiovasc Med 2021;8:774755. [Crossref] [PubMed]
- Yan Y, Song D, Zhang X, et al. GEO Data Sets Analysis Identifies COX-2 and Its Related Micro RNAs as Biomarkers for Non-Ischemic Heart Failure. Front Pharmacol 2020;11:1155. [Crossref] [PubMed]
- McNally EM, Mestroni L. Dilated Cardiomyopathy: Genetic Determinants and Mechanisms. Circ Res 2017;121:731-48. [Crossref] [PubMed]
- Abplanalp WT, John D, Cremer S, et al. Single-cell RNA-sequencing reveals profound changes in circulating immune cells in patients with heart failure. Cardiovasc Res 2021;117:484-94. [Crossref] [PubMed]
- Molina-Navarro MM, Roselló-Lletí E, Ortega A, et al. Differential gene expression of cardiac ion channels in human dilated cardiomyopathy. PLoS One 2013;8:e79792. [Crossref] [PubMed]
- Enzan N, Matsushima S, Ide T, et al. The Use of Angiotensin-Converting Enzyme Inhibitors or Angiotensin II Receptor Blockers Is Associated with the Recovered Ejection Fraction in Patients with Dilated Cardiomyopathy. Int Heart J 2021;62:801-10. [Crossref] [PubMed]
- Enzan N, Matsushima S, Ide T, et al. The use of angiotensin II receptor blocker is associated with greater recovery of cardiac function than angiotensin-converting enzyme inhibitor in dilated cardiomyopathy. ESC Heart Fail 2022;9:1175-85. [Crossref] [PubMed]
- Szklarczyk D, Gable AL, Nastou KC, et al. The STRING database in 2021: customizable protein-protein networks, and functional characterization of user-uploaded gene/measurement sets. Nucleic Acids Res 2021;49:D605-12. [Crossref] [PubMed]
- Doncheva NT, Morris JH, Gorodkin J, et al. Cytoscape StringApp: Network Analysis and Visualization of Proteomics Data. J Proteome Res 2019;18:623-32. [Crossref] [PubMed]
- Liao Y, Wang J, Jaehnig EJ, et al. WebGestalt 2019: gene set analysis toolkit with revamped UIs and APIs. Nucleic Acids Res 2019;47:W199-205. [Crossref] [PubMed]
- Zhou Y, Zhou B, Pache L, et al. Metascape provides a biologist-oriented resource for the analysis of systems-level datasets. Nat Commun 2019;10:1523. [Crossref] [PubMed]
- Yoshihara K, Shahmoradgoli M, Martínez E, et al. Inferring tumour purity and stromal and immune cell admixture from expression data. Nat Commun 2013;4:2612. [Crossref] [PubMed]
- Yi M, Nissley DV, McCormick F, et al. ssGSEA score-based Ras dependency indexes derived from gene expression data reveal potential Ras addiction mechanisms with possible clinical implications. Sci Rep 2020;10:10258. [Crossref] [PubMed]
- Lohning AE, Levonis SM, Williams-Noonan B, et al. A Practical Guide to Molecular Docking and Homology Modelling for Medicinal Chemists. Curr Top Med Chem 2017;17:2023-40. [Crossref] [PubMed]
- Saikia S, Bordoloi M. Molecular Docking: Challenges, Advances and its Use in Drug Discovery Perspective. Curr Drug Targets 2019;20:501-21. [Crossref] [PubMed]
- Cicoira M, Zanolla L, Franceschini L, et al. Relation of aldosterone "escape" despite angiotensin-converting enzyme inhibitor administration to impaired exercise capacity in chronic congestive heart failure secondary to ischemic or idiopathic dilated cardiomyopathy. Am J Cardiol 2002;89:403-7. [Crossref] [PubMed]
- Dzau VJ, Bernstein K, Celermajer D, et al. The relevance of tissue angiotensin-converting enzyme: manifestations in mechanistic and endpoint data. Am J Cardiol 2001;88:1L-20L. [Crossref] [PubMed]
- Lee S, Oh J, Kim H, et al. Sacubitril/valsartan in patients with heart failure with reduced ejection fraction with end-stage of renal disease. ESC Heart Fail 2020;7:1125-9. [Crossref] [PubMed]
- Hershberger RE, Hedges DJ, Morales A. Dilated cardiomyopathy: the complexity of a diverse genetic architecture. Nat Rev Cardiol 2013;10:531-47. [Crossref] [PubMed]
- Marian AJ, Braunwald E. Hypertrophic Cardiomyopathy: Genetics, Pathogenesis, Clinical Manifestations, Diagnosis, and Therapy. Circ Res 2017;121:749-70. [Crossref] [PubMed]
- Nair NU, Das A, Amit U, et al. Putative functional genes in idiopathic dilated cardiomyopathy. Sci Rep 2018;8:66. [Crossref] [PubMed]
- Vandoorne K, Vandsburger MH, Weisinger K, et al. Multimodal imaging reveals a role for Akt1 in fetal cardiac development. Physiol Rep 2013;1:e00143. [Crossref] [PubMed]
- Yu M, Wen S, Wang M, et al. TNF-α-secreting B cells contribute to myocardial fibrosis in dilated cardiomyopathy. J Clin Immunol 2013;33:1002-8. [Crossref] [PubMed]
- Liu X, Zhang W, Han Z. Decreased circulating follicular regulatory T cells in patients with dilated cardiomyopathy. Braz J Med Biol Res 2021;54:e11232. [Crossref] [PubMed]
- Fischer K, Diec S. Once- Versus Twice-Daily Angiotensin-Converting Enzyme Inhibitors for Blood Pressure Control in Adult Patients With Hypertension. Cureus 2021;13:e17331. [Crossref] [PubMed]
- Behr TM, Wang X, Aiyar N, et al. Monocyte chemoattractant protein-1 is upregulated in rats with volume-overload congestive heart failure. Circulation 2000;102:1315-22. [Crossref] [PubMed]
- Kumar AG, Ballantyne CM, Michael LH, et al. Induction of monocyte chemoattractant protein-1 in the small veins of the ischemic and reperfused canine myocardium. Circulation 1997;95:693-700. [Crossref] [PubMed]
- Frangogiannis NG, Dewald O, Xia Y, et al. Critical role of monocyte chemoattractant protein-1/CC chemokine ligand 2 in the pathogenesis of ischemic cardiomyopathy. Circulation 2007;115:584-92. [Crossref] [PubMed]
- Hayashidani S, Tsutsui H, Shiomi T, et al. Anti-monocyte chemoattractant protein-1 gene therapy attenuates left ventricular remodeling and failure after experimental myocardial infarction. Circulation 2003;108:2134-40. [Crossref] [PubMed]
- Collén A, Bergenhem N, Carlsson L, et al. VEGFA mRNA for regenerative treatment of heart failure. Nat Rev Drug Discov 2022;21:79-80. [Crossref] [PubMed]
- Xue GL, Li DS, Wang ZY, et al. Interleukin-17 upregulation participates in the pathogenesis of heart failure in mice via NF-κB-dependent suppression of SERCA2a and Cav1.2 expression. Acta Pharmacol Sin 2021;42:1780-9. [Crossref] [PubMed]
- Zhao X, Ren Y, Ren H, et al. The mechanism of myocardial fibrosis is ameliorated by myocardial infarction-associated transcript through the PI3K/Akt signaling pathway to relieve heart failure. J Int Med Res 2021;49:3000605211031433. [Crossref] [PubMed]
- Kawai C, Matsumori A. Dilated cardiomyopathy update: infectious-immune theory revisited. Heart Fail Rev 2013;18:703-14. [Crossref] [PubMed]
- Zhao L, Fu Z. Roles of Host Immunity in Viral Myocarditis and Dilated Cardiomyopathy. J Immunol Res 2018;2018:5301548. [Crossref] [PubMed]
- Schultheiss HP, Fairweather D, Caforio ALP, et al. Dilated cardiomyopathy. Nat Rev Dis Primers 2019;5:32. [Crossref] [PubMed]
- Schwimmbeck PL, Badorff C, Rohn G, et al. The role of sensitized T-cells in myocarditis and dilated cardiomyopathy. Int J Cardiol 1996;54:117-25. [Crossref] [PubMed]
- Ma X, Xu L, Li Y, et al. Immunotherapy reversed myopathy but not cardiomyopathy in a necrotizing autoimmune myopathy patient with positive anti-SRP and MDA-5 autoantibodies. BMC Cardiovasc Disord 2021;21:88. [Crossref] [PubMed]
(English Language Editor: A. Kassem)