Fractal analysis in cardiovascular magnetic resonance: prognostic value of biventricular trabecular complexity in hypertrophic cardiomyopathy
Highlight box
Key findings
• Higher biventricular fractal dimensions (FDs) are associated with increased risks of sudden cardiac death events and rehospitalization due to heart failure in patients with hypertrophic cardiomyopathy (HCM).
• The addition of biventricular FDs and late gadolinium enhancement to European Society of Cardiology risk factors provides incremental prognostic value.
What is known and what is new?
• Hypertrabeculation is observed as a feature of some genetically characterized cardiomyopathies.
• We found that both left and right ventricular FDs are independent prognostic factors in HCM.
What is the implication, and what should change now?
• The existing model of HCM risk stratification needs update with more novel markers.
• Further histological and pathological investigations are needed to explain the underlying mechanism of the influence of ventricular trabeculae.
Introduction
Hypertrophic cardiomyopathy (HCM) is a common genetically inherited heart muscle disease with reported occurrence of 1 in 500 (1). Since HCM can lead to sudden cardiac death (SCD), heart failure and other adverse events, the prediction of HCM prognosis is important for early intervention and enhanced risk stratification. Previous researches found predictors for poor prognosis in HCM including clinical history, genetic factors, serologic markers, and imaging indicators (2-5). The European Society of Cardiology (ESC) provides a widely accepted model for risk stratification of SCD and the need for implanting cardioverter defibrillator in HCM (6), including maximum LV wall thickness, LV atrial size, peak left ventricular outflow tract (LVOT) gradient (collected by echocardiography), age, family history of SCD, unexplained syncope, and non-sustained ventricular tachycardia. Since the ESC model (7) was published in 2014, the HCM Risk-SCD variables need an update with American Heart Association and American College of Cardiology (AHA/ACC) guidelines (8) and more competitive markers. Late gadolinium enhancement (LGE) was proven to be a promising risk factor of poor outcome in patients with HCM in extensive studies (9-11). Although considerable advances, risk stratification in HCM is still incomplete since some under-risk patients are not identified by the current risk factors (12). Thus, we try to investigate the influence of trabecular complexity in HCM prognosis besides existing variables.
Trabeculae is a complex network of myocardial strands that protrudes into the cavity of cardiac ventricular chambers (13). Trabecular complexity can be quantified by fractal analysis based on cine images of cardiovascular magnetic resonance (CMR), which yields the fractal dimension (FD) index (14). The FDs reflect surface complexity of endocardial contours, which were independent of the influence of wall thickness, and a higher value indicates a greater degree of surface complexity (15,16). HCM is a diffuse cardiomyopathy that can involve both ventricles. The left ventricular (LV) FDs have been applied in HCM evaluation as a novel marker (17,18). Although the previous study reached the conclusion that high apical LV FD is an independent predictor of adverse events in patients with HCM (18), the impact of RV trabecular complexity in HCM remains unknown and the prognostic value of LV FD needs more external validation.
Therefore, in this study, we tried to investigate the prognostic value of LV and right ventricular (RV) (biventricular) FDs in HCM. In addition, we assessed the incremental value of biventricular FDs to the existing predictive variables (the ESC model and LGE) in HCM. We present this article in accordance with the TRIPOD reporting checklist (available at https://cdt.amegroups.com/article/view/10.21037/cdt-23-162/rc).
Methods
Study subjects
This is a two-center observational study. Two hundred eighty-four patients who underwent CMR from August 2017 to December 2020 were enrolled. We have 10 variables in the multivariable cox regression model, so that 20 times the variables is a sufficient sample size (19). The follow-up collection ended in December 2021, and 15 patients received implantable cardioverter defibrillator (ICD). Center 1 represents Renji Hospital Affiliated to Shanghai Jiao Tong University. Center 2 represents Beijing Anzhen Hospital Affiliated Capital Medical University. HCM is diagnosed according to the following criteria: (I) the left ventricle wall thickness (LVWT) was above or equal to 15 mm at the end-diastolic stage and couldn’t be explained by a loading condition; (II) the LVWT was 13 to 14 mm with a relevant HCM family history and couldn’t be explained by a loading condition (7,8). Patients who met the diagnostic criteria of HCM and with adequate CMR sequences and acceptable image quality were recruited. Those lost in follow-up and with insufficient images such as un-enhanced CMR were excluded (Figure 1).
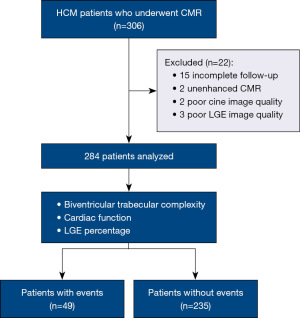
The study was conducted in accordance with the Declaration of Helsinki (as revised in 2013). The study was approved by institutional review board of Beijing Anzhen Hospital (No. 2023128) and Shanghai Renji Hospital (No. 2018193). Informed consent was not required because this is a retrospective study.
CMR protocol
CMR scans were performed at two sites. In center 1, CMR scans were conducted by a 3.0 T MR scanner (Ingenia, Philips, Best, The Netherlands) equipped with a 12-element phased-array coil. In center 2, CMR scans were performed using a 3.0 T MR system (MAGNETOM Verio, Siemens Healthcare, Erlangen, Germany) with a 32-channel cardiac coil. The sequences in our study included: functional cine imaging, and phase-sensitive inversion recovery (PSIR). Cine and PSIR sequences were performed from LV base to apex with no gap. The detailed CMR protocols were presented in Supplementary file (Appendix 1).
Imaging analysis
CMR ventricular functions and volumes and LGE percentage were measured by two experienced radiologists with 10 and 2 years’ experience respectively using a commercially available software (Circle Cardiovascular Imaging, version 5.13.5, Calgary, Canada). Maximal LV wall thickness was measured on the end-diastolic phase of short-axis cine slices. The percentage of LGE was identified and calculated by CVI software with a full-width at half-maximum (FWHM) method.
Fractal analysis
FD, a number between 1–2 to quantify trabecular complexity, was derived from LV and RV short-axis cine images by fractal analysis. In our study, fractal analysis was performed by FracAnalyse (18,20) using Matlab software (MathWorks, Natick, Mass) with custom-written code which was available online for free (21) (Figure 2). For each slice, a polygonal contour of the right ventricle and a round contour of the left ventricle were manually placed in the mid-wall myocardium on the end-diastolic phase cine image. Then, the endocardial contour was delineated automatically and the FD value was calculated by FracAnalyse software. The average FD of each slice in the ventricle was defined as the global FD. Ventricular slices were split into basal and apical stacks by half and the highest FD value was obtained separately to generate the maximal apical and basal FDs. The core principle of FD calculation was extracting endocardial border through image segmentation and then calculating the endocardial border complexity with the box-counting method (22,23). The analysis process included: image binarization and thresholding of the ventricle myocardium and blood pool by a region-based level-set algorithm; extraction of the endocardial borders of trabeculae were detected using the Sobel operator; papillary muscles and sub-valvular apparatus exclusion; calculation of FD with the box-counting method.
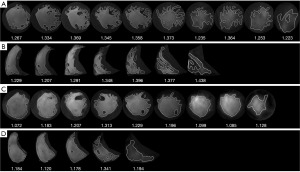
Clinical data collection
The clinical data and follow-up information were collected by two cardiologists over 10 years of experience who were blinded to imaging information. Follow-up data were obtained with hospital records, clinic visits, and telephone interviews. The primary end point was defined as SCD events including SCD, resuscitated cardiac arrest, and received defibrillation interventions from an ICD, which are potentially equivalent to SCD. The secondary end point included both primary end point and rehospitalization due to heart failure.
Statistical analysis
Data were analyzed by SPSS software (version 26.0; IBM Corp., Armonk, NY, USA) and R software (version 4.1.2; The R Project for Statistical Computing, Vienna, Austria). Patients with and without adverse events (including both primary and secondary end points) were compared using the Student t-test or Mann-Whitney U-test for the continuous variables and the χ2 or Fisher exact test for the categorical variables. Pearson correlation was used to evaluate the correlation between biventricular FDs and cardiac function parameters, and to test the independence of RV FDs with LV FDs. The survival curves were established by the Kaplan-Meier method and compared with the log-rank test. The optimal cutoff was defined by the most significant (log-rank test) split (surv_cutpoint in survminer R package) (24). Univariable and multivariable Cox regressions were used to determine whether different parts of biventricular FDs were predictors of events. P<0.05 was considered statistically significant. Since different parts of RV FDs were not completely independent of each other, separate multivariable models were built to examine whether each RV FD marker was an independent predictor of events and which had the best performance after the adjustment of ESC predictors and LGE percentage. Hosmer-Lemeshow goodness of fit test was used for evaluating the consistency of the predicted probabilities with the actual events. To evaluate the incremental prognostic value of both ventricular FDs, models were established by adding one or both ventricular FDs to the ESC risk predictors and LGE percentage. The additional predictive power of each model was established by Harrell’s C-statistic after the addition of the ventricular FDs and LGE to the ESC model (age, maximum LV wall thickness, LV atrial size, peak LVOT gradient, family history of SCD, unexplained syncope, non-sustained ventricular tachycardia). The calculation of continuous net reclassification improvement (NRI) was used to evaluate model reclassification improvement. Internal validation of C-index was done by the bootstrapping method. To evaluate the intra- and inter-observer agreement, the intraclass correlation coefficient (ICC; two-way random, agreement) was computed.
Results
Study population characteristics
Two hundred eighty-four patients (median age, 53 years; 192 men) with HCM were included finally. During a median follow-up of 24 months [interquartile range (IQR), 11–31 months], 8% of patients reached the primary end point, including SCD in 18 (6%), resuscitated cardiac arrest and ICD discharge because of ventricular tachycardia or fibrillation in 5 (2%). Forty-nine (17%) patients reached secondary end point which was composite events including primary end points and rehospitalization due to heart failure (9%).
The demographic, clinical, and CMR characteristics of HCM patients with and without events are exhibited in Table 1. There were significant differences in heart rate, age, body surface area, hypertension, obstructive HCM, and CMR parameters between the two groups. The group with events had higher peak left ventricular outflow track resting gradients, higher LV mass index, larger left atrium size and maximum left ventricle thickness, with significantly lower LV ejection fraction. The LGE% of myocardium was distinctly higher in patients with adverse events compared with the group without adverse events [median 7.8 (IQR, 2.8–20.1) vs. 1.7 (IQR, 0–4.9); P<0.001] (Table 1).
Table 1
Characteristics | All patients (n=284) | With adverse events (n=49) | Without adverse events (n=235) | P value |
---|---|---|---|---|
Demographics | ||||
Age (years) | 53 [40, 62] | 59 [50, 68] | 52 [38, 61] | 0.001 |
Male | 192 [68] | 37 [76] | 155 [66] | 0.444 |
BMI (kg/m²) | 24.9±1.9 | 25.5±1.3 | 24.8±1.9 | 0.02 |
BSA (m²) | 1.82±0.17 | 1.83±0.15 | 1.81±0.17 | 0.901 |
SBP (mmHg) | 117±12 | 118±18 | 117±10 | 0.944 |
DBP (mmHg) | 76±7 | 77±10 | 75±6 | 0.92 |
HR (beats/min) | 71 [67, 77] | 77 [69, 98] | 71 [67, 76] | <0.001 |
Risk factors | ||||
Hypertension | 48 [17] | 13 [27] | 35 [15] | 0.002 |
Diabetes | 17 [6] | 4 [8] | 13 [6] | 0.344 |
CAD | 14 [5] | 4 [8] | 10 [4] | 0.323 |
Obstructive HCM | 83 [29] | 23 [47] | 60 [26] | 0.043 |
Family history of SCD | 45 [16] | 5 [10] | 40 [17] | 0.732 |
History of syncope | 25 [9] | 7 [14] | 18 [8] | 0.947 |
NSVT | 26 [9] | 8 [16] | 18 [8] | 0.556 |
NYHA functional class | ||||
I | 147 [52] | 1 [2] | 146 [62] | – |
II | 71 [25] | 20 [41] | 51 [22] | – |
III | 23 [8] | 5 [10] | 18 [8] | – |
IV | 43 [15] | 23 [47] | 20 [9] | – |
Echocardiography parameters | ||||
LA size (mm) | 43 [38, 48] | 47 [41, 51] | 42 [37, 48] | 0.001 |
Max LVT (mm) | 18.8 [15.8, 21.9] | 19.8 [18.0, 23.9] | 18.5 [15.6, 21] | 0.001 |
Peak LVOT resting gradients (mmHg) | 12 [6, 31] | 23 [14, 31] | 9 [6, 30] | 0.001 |
CMR parameters | ||||
LVEDVi (mL/m²) | 65.6±26.8 | 79.8±37.8 | 62.6±22.9 | 0.002 |
LVESVi (mL/m²) | 27.2±22.2 | 47.6±32.0 | 22.9±16.7 | <0.001 |
LVEF (%) | 61.3±16.3 | 42.0±15.1 | 65.3±13.3 | <0.001 |
SV (mL) | 69.6±26.6 | 59.0±25.3 | 71.9±26.4 | 0.004 |
LV mass index (g/m²) | 94.2±42.2 | 104.8±37.9 | 91.9±42.9 | 0.006 |
LGE (%) | 2.4 [0, 6.1] | 7.8 [2.8, 20.1] | 1.7 [0, 4.9] | <0.001 |
RV FD | ||||
Global FD | 1.261±0.038 | 1.284±0.039 | 1.257±0.037 | <0.001 |
Maximal apical FD | 1.348±0.054 | 1.372±0.050 | 1.343±0.053 | 0.001 |
Maximal basal FD | 1.283±0.063 | 1.304±0.063 | 1.278±0.062 | 0.011 |
LV FD | ||||
Global FD | 1.241±0.040 | 1.262±0.038 | 1.236±0.039 | <0.001 |
Maximal apical FD | 1.297±0.058 | 1.331±0.053 | 1.289±0.053 | <0.001 |
Maximal basal FD | 1.325±0.045 | 1.332±0.049 | 1.324±0.044 | 0.211 |
Data are mean ± SD or median [interquartile range] or n [%]. Obstructive HCM, LV outflow tract gradient greater than or equal to 30 mmHg at rest by echocardiography. Adverse events, composite of primary and secondary end points. CMR, cardiovascular magnetic resonance; HCM, hypertrophic cardiomyopathy; BMI, body mass index; BSA, body surface area; SBP, systolic blood pressure; DBP, diastolic blood pressure; HR, heart rate; CAD, coronary artery disease; SCD, sudden cardiac death; NSVT, non-sustained ventricular tachycardia; NYHA, New York Heart Association; LA, left atrium; Max LVT, maximal LV wall thickness; LVOT, left ventricular outflow tract; LVEDVi, LV end-diastolic volume index; LVESVi, LV end-systolic volume index; LVEF, LV ejection fraction; SV, stroke volume; LV, left ventricle; LGE, late gadolinium enhancement; RV, right ventricle; FD, fractal dimension; SD, standard deviation.
Correlations between biventricular FDs and cardiac function parameters
According to the Pearson correlation (Figure 3), in patients with HCM, biventricular FDs were weakly associated with higher LV mass index, left atrium size, and maximal left ventricle thickness. RV FDs had weak association with lower LV and RV ejection fraction, and higher RV mass index (Figure S1). These findings indicated that HCM may alter the degree of trabeculation independently of ventricular size and functions. Moreover, RV FDs had weak association with LV FDs (r=0.04–0.3, all P<0.05). Thus, RV FDs were independent of LV FDs and could have its own prediction value of survival in HCM.
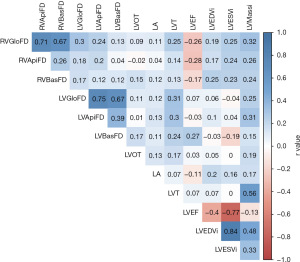
Biventricular FD characteristics in HCM patients
LV maximal apical FD in patients with events elevated significantly compared to patients without events (1.331±0.053 vs. 1.289±0.053; P<0.001). The same trend also occurred in RV global FD (1.284±0.039 vs. 1.257±0.037; P<0.001). LV Global FD, RV maximal basal and apical FD were also higher in patients with events than in patients without events yet (all P<0.05) (Table 1).
We separated the patients into high and low LV FD groups with the cutoff value at 1.347 in LV maximal apical FD, while RV groups used the cutoff value at 1.290 in RV global FD. The optimal cutoff was defined as the point with the most significant (log-rank test) split according to the significance of correlation with survival variable (24). According to the Kaplan-Meier analysis (Figure 4), patients with higher LV maximal apical FD (≥1.347) had higher possibility of reaching primary and secondary end points in HCM patients (all P<0.001). Higher RV global FD (≥1.290) in patients also exhibited higher risks of both end points (all P<0.001).
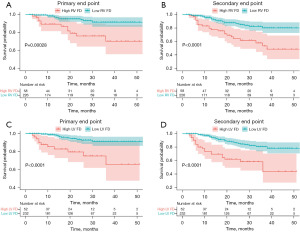
Prognostic value of biventricular FDs
According to the univariable Cox regression result (Table 2), RV FDs were significant prognostic factors of both primary and secondary end points (all P<0.05), while the RV global FD was the most outstanding one (hazard ratio, 1.179; P<0.001). LV maximal apical FD was the only LV FDs which was significantly correlated with both end points (hazard ratio range, 1.081–1.109; all P<0.05).
Table 2
Characteristics | Primary end point | Secondary end point | |||
---|---|---|---|---|---|
Unadjusted hazard ratio | P value | Unadjusted hazard ratio | P value | ||
Male | 3.275 (0.973, 11.020) | 0.055 | 1.538 (0.802, 2.949) | 0.195 | |
ESC risk predictors | |||||
Age (years) | 1.044 (1.011, 1.079) | 0.008 | 1.039 (1.017, 1.061) | 0.001 | |
Peak LVOT resting gradients (mmHg) | 1.025 (0.993, 1.058) | 0.123 | 1.030 (1.008, 1.053) | 0.008 | |
NSVT | 2.254 (0.766, 6.631) | 0.140 | 2.157 (1.011, 4.604) | 0.047 | |
Family history of SCD | 0.727 (0.216, 2.448) | 0.607 | 0.551 (0.219, 1.390) | 0.207 | |
History of syncope | 2.842 (1.055, 7.655) | 0.039 | 1.720 (0.773, 3.829) | 0.184 | |
Left atrium size (mm) | 1.056 (1.027, 1.087) | <0.001 | 1.056 (1.027, 1.087) | <0.001 | |
Max LVT (mm) | 1.061 (0.994, 1.132) | 0.074 | 1.047 (1.001, 1.096) | 0.047 | |
Risk factors for adverse events | |||||
Obstructive HCM | 1.562 (0.676, 3.608) | 0.297 | 2.193 (1.251, 3.845) | 0.006 | |
Max LVT >30 mm | 0.787 (0.106, 5.842) | 0.815 | 1.106 (0.344, 3.557) | 0.866 | |
LGE (%) | 1.068 (1.041, 1.096) | <0.001 | 1.069 (1.050, 1.088) | <0.001 | |
RV FD | |||||
Global FD | 1.179 (1.068, 1.302) | 0.001 | 1.179 (1.099, 1.264) | <0.001 | |
Max apical FD | 1.092 (1.009, 1.181) | 0.029 | 1.095 (1.037, 1.155) | 0.001 | |
Max basal FD | 1.076 (1.012, 1.144) | 0.018 | 1.065 (1.021, 1.111) | 0.004 | |
LV FD | |||||
Global FD | 1.079 (0.976, 1.193) | 0.135 | 1.135 (1.059, 1.216) | <0.001 | |
Max apical FD | 1.081 (1.007, 1.161) | 0.031 | 1.109 (1.056, 1.165) | <0.001 | |
Max basal FD | 0.944 (0.863, 1.033) | 0.211 | 1.025 (0.962, 1.092) | 0.440 |
Fractal dimension is per 1% increase. Numbers in parentheses are 95% confidence intervals. HCM, hypertrophic cardiomyopathy; ESC, European Society of Cardiology; LVOT, left ventricular outflow tract; NSVT, non-sustained ventricular tachycardia; SCD, sudden cardiac death; Max LVT, maximal LV wall thickness; LGE, late gadolinium enhancement; RV, right ventricular; FD, fractal dimension; LV, left ventricular.
In the multivariable Cox analysis (Table 3), after the adjustment of ESC risk factors and LGE percentage, LV maximal apical was still an independent prognostic factor for events (hazard ratio range, 1.114–1.133; all P<0.05). Since RV FDs were relevant with each other, multivariable models were built separately for each RV FD marker. The RV global FD was the most significant independent predictor among RV FDs for the primary end point (hazard ratio, 1.135, 95% CI: 1.006–1.280; P=0.039) and the secondary end point (hazard ratio, 1.150, 95% CI: 1.059–1.248; P=0.001). Hosmer-Lemeshow goodness of fit test showed great calibration of the models (all P>0.05, range from 0.991 to 0.994). The RV FDs had more consistent cox regression results than LV FDs, and RV global FD had the highest hazard ratio, which may indicate that hypertrabeculation involving RV has worse prognosis.
Table 3
Characteristics | Primary end point | Secondary end point | |||
---|---|---|---|---|---|
Unadjusted hazard ratio | P value | Unadjusted hazard ratio | P value | ||
ESC risk predictors | |||||
Age (years) | 1.080 (1.036, 1.127) | <0.001 | 1.063 (1.034, 1.093) | <0.001 | |
Peak LVOT resting gradients (mmHg) | 1.007 (0.970, 1.044) | 0.724 | 1.022 (0.997, 1.047) | 0.088 | |
NSVT | 1.019 (0.237, 4.378) | 0.980 | 1.947 (0.668, 5.676) | 0.222 | |
Family history of SCD | 1.581 (0.276, 9.049) | 0.607 | 1.088 (0.312, 3.799) | 0.894 | |
History of syncope | 3.996 (0.718, 22.246) | 0.114 | 1.270 (0.348, 4.639) | 0.717 | |
Left atrium size (mm) | 1.035 (0.985, 1.088) | 0.169 | 1.035 (1.001, 1.070) | 0.045 | |
Max LVT (mm) | 0.987 (0.899, 1.082) | 0.775 | 0.956 (0.897, 1.019) | 0.167 | |
LGE (%) | 1.066 (1.030, 1.103) | <0.001 | 1.081 (1.055, 1.108) | <0.001 | |
LV max apical FD | 1.114 (1.029, 1.206) | 0.007 | 1.133 (1.074, 1.195) | <0.001 | |
RV global FD | 1.135 (1.006, 1.280) | 0.039 | 1.150 (1.059, 1.248) | 0.001 | |
RV max apical FD | 1.059 (0.968, 1.158) | 0.209 | 1.082 (1.015, 1.152) | 0.015 | |
RV max basal FD | 1.086 (1.002, 1.176) | 0.044 | 1.061 (1.006, 1.119) | 0.029 |
Fractal dimension is per 1% increase. Numbers in parentheses are 95% confidence intervals. HCM, hypertrophic cardiomyopathy; ESC, European Society of Cardiology; LVOT, left ventricular outflow tract; NSVT, non-sustained ventricular tachycardia; SCD, sudden cardiac death; Max LVT, maximal LV wall thickness; LGE, late gadolinium enhancement; LV, left ventricular; FD, fractal dimension; RV, right ventricular.
Incremental prognostic value of biventricular FDs
The model 4 with addition of biventricular FDs to existing risk factors (ESC risk factors and LGE percentage) had the highest C-index (0.864, 0.877 respectively) in both end points, yielding the most effective prediction model (Table 4). The internal validation using 1000-bootstrapping method yielded the C-index distribution supporting good reproducibility of the models (Figure S2). The addition of biventricular FDs had greatest improvement over the existing predictors to model reclassification to predict the occurrence of both end points (NRI =0.447 and 0.475 respectively) (Table 4). The coefficients of variables and the model intercept of model 4 are reported in Table S1.
Table 4
Models | Primary end point | Secondary end point | |||
---|---|---|---|---|---|
C-index (95% CI) | NRI (95% CI) | C-index (95% CI) | NRI (95% CI) | ||
Model 1 (ESC factors + LGE%) | 0.814 (0.739–0.889) | Baseline | 0.809 (0.751–0.868) | Baseline | |
Model 2 (model 1 + LV FD) | 0.840 (0.775–0.906) | 0.379 (0.017–0.585) | 0.848 (0.803–0.894) | 0.370 (0.050–0.581) | |
Model 3 (model 1 + RV FD) | 0.852 (0.799–0.905) | 0.192 (0.042–0.342) | 0.844 (0.798–0.890) | 0.211 (0.049–0.348) | |
Model 4 (model 1 + LV FD + RV FD) | 0.864 (0.813–0.914) | 0.447 (0.062–0.606) | 0.877 (0.841–0.913) | 0.475 (0.046–0.582) |
ESC factors including age, maximum LV wall thickness, left atrium size, peak LV outflow tract gradient, family history of sudden cardiac death, unexplained syncope, non-sustained ventricular tachycardia. Covariates in model 1: seven ESC risk factors + LGE%; covariates in model 2: model 1 + LV max apical FD; covariates in model 3: model 1 + RV global FD; covariates in model 4: model 1 + LV max apical FD + RV global FD. FD, fractal dimension; CI, confidence interval; NRI, net reclassification improvement; ESC, European Society of Cardiology; LGE, late gadolinium enhancement; LV, left ventricular; RV, right ventricular.
Reproducibility
Reproducibility was excellent for FDs and LGE percentage with intra- and interobserver ICC range from 0.90–0.99 (P<0.001) (Table S2).
Discussion
Our study investigated the prognosis value of biventricular trabecular complexity in HCM patients by a multicenter cohort. The main findings are (I) higher biventricular FDs are associated with higher adverse event incidence in patients with HCM; (II) both LV maximal apical FD and RV global FD are independent prognostic factors in patients with HCM; and (III) the addition of biventricular FDs yields the greatest incremental prognostic value over the existing risk factors including ESC HCM Risk-SCD variables and LGE.
In previous studies, the ESC prediction model could provide prognostic information regarding to SCD in HCM (6,25). In addition, LGE in contrast-enhanced CMR could improve the risk stratification model of SCD in HCM, but the proposed model required further external validation (9). Findings of additional markers are important to allow more indication of who may benefit from primary prevention like ICD therapy (9,26,27). Thus, our study provides more validation for updating ESC model with LGE and new markers for risk stratification in HCM. Besides SCD, heart failure is also an important complication of HCM. However, available models mainly focus on predicting SCD, and more evidence is needed for prediction of heart failure in HCM. Our study took heart failure into considerations in the secondary end point for detecting susceptibility of more adverse events in HCM.
FD is believed to have prognostic value in HCM according to many previous researches. Captur et al. (17) and Wang et al. (18) indicated that LV FDs were elevated in HCM patients, compared to healthy people. Our study found that both LV and RV FDs were elevated in HCM patients with adverse events compared to patients without events (all P<0.001), and the appearance of hypertrabeculation in RV may indicate worse prognosis in patients with HCM. The reason why LV basal FD had no significance may be relatively sparse trabeculae in basal myocardium, and the apical endocardium of distal ventricle is more susceptible to developmental arrest of the compaction process (20,28,29).
Hyper-trabeculation is observed to be a feature of some genetically characterized cardiomyopathies (15). A previous study indicated that abnormal trabecular patterning was found in HCM and preclinical HCM (17). They found trabecular complexity increased in HCM patients as well as subclinical carriers with sarcomere gene mutation compared to healthy people. Meyer et al. (30) suggested that the structural complexity of myocardial trabeculae had impact on susceptibility to cardiovascular disease and physiology function of the heart. Trabecular morphology is thought to be related with genetic, environmental, and hemodynamic factors, indicating that it may be modified and adapt to altered loading conditions and ventricle remodeling (16). HCM is a myocardial disease with hyper-trabeculation and diverse patterns of phenotypic expression. Therefore, the reason why higher RV FD is associated with poorer outcome in patients with HCM may be because hyper-trabeculation in the right ventricle indicates more cardiac ventricle remodeling or worse HCM phenotype.
FD is highly practical and reproducible to reflect trabecular complexity, and the degree of trabeculation has influence on ventricular mechanics, as reported by previous studies (9,14,16,18,20). Further researches are required to reveal whether trabecular complexity is a passive compensatory consequence of remodeling or an adaptive response to maintain contractile efficiency (20).
Limitations
First, it was retrospective and could not avoid all confounding factors including management decisions. Second, our study had a modest sample size, which needs more external validation. Moreover, our follow-up period was relatively short, which the events of patients might be underestimated and weakened the prognostic strength of FD. In addition, our study was conducted in a relatively high-risk HCM cohort, which may overestimate the efficacy of some variables. The potential reason may be some patients in their early stage didn’t visit our centers. Finally, genetic data were unavailable in this cohort.
Conclusions
Biventricular FDs provide significant prognostic value for SCD and composite adverse events including rehospitalization due to heart failure in HCM. LV maximal apical FD and RV global FD are independent risk factors in patients with HCM. In addition, they together provide incremental prognostic value to the existing predictors including ESC model variables and LGE percentage.
Acknowledgments
The abstract was presented at the International Society for Magnetic Resonance in Medicine (ISMRM) 2023 annual meeting on June 8th, 2023. We appreciate Luke Wesemann (Department of Radiology, Wanye State University, School of Medicine) for polishing the language of our paper.
Funding: The study was supported by the National Natural Science Foundation of China (Nos. 81873887 and 82171884); National Natural Science Foundation of China Youth Project (No. 82101981); Shanghai Science and Technology Innovation Action Plan, Technology Standard Project (No. 19DZ2203800); Shanghai Science and Technology Innovation Action Plan (No. 20Y11912200); Shanghai Municipal Education Commission-Gaofeng Clinical Medicine Grant; Shanghai Jiao Tong University School of Medicine Double Hundred Outstanding Person Project (No. 20191904); Beijing Natural Science Foundation (No. 7222302); and the National Key Research and Development Program of China (project No. 2021YFF0501400).
Footnote
Reporting Checklist: The authors have completed the TRIPOD reporting checklist. Available at https://cdt.amegroups.com/article/view/10.21037/cdt-23-162/rc
Data Sharing Statement: Available at https://cdt.amegroups.com/article/view/10.21037/cdt-23-162/dss
Peer Review File: Available at https://cdt.amegroups.com/article/view/10.21037/cdt-23-162/prf
Conflicts of Interest: All authors have completed the ICMJE uniform disclosure form (available at https://cdt.amegroups.com/article/view/10.21037/cdt-23-162/coif). The authors have no conflicts of interest to declare.
Ethical Statement: The authors are accountable for all aspects of the work in ensuring that questions related to the accuracy or integrity of any part of the work are appropriately investigated and resolved. The study was conducted in accordance with the Declaration of Helsinki (as revised in 2013). The study was approved by institutional review board of Beijing Anzhen Hospital (No. 2023128) and Shanghai Renji Hospital (No. 2018193). Informed consent was not required because this is a retrospective study.
Open Access Statement: This is an Open Access article distributed in accordance with the Creative Commons Attribution-NonCommercial-NoDerivs 4.0 International License (CC BY-NC-ND 4.0), which permits the non-commercial replication and distribution of the article with the strict proviso that no changes or edits are made and the original work is properly cited (including links to both the formal publication through the relevant DOI and the license). See: https://creativecommons.org/licenses/by-nc-nd/4.0/.
References
- Maron BJ, Gardin JM, Flack JM, et al. Prevalence of hypertrophic cardiomyopathy in a general population of young adults. Echocardiographic analysis of 4111 subjects in the CARDIA Study. Coronary Artery Risk Development in (Young) Adults. Circulation 1995;92:785-9. [Crossref] [PubMed]
- Geske JB, Ong KC, Siontis KC, et al. Women with hypertrophic cardiomyopathy have worse survival. Eur Heart J 2017;38:3434-40. [Crossref] [PubMed]
- Geske JB, McKie PM, Ommen SR, et al. B-type natriuretic peptide and survival in hypertrophic cardiomyopathy. J Am Coll Cardiol 2013;61:2456-60. [Crossref] [PubMed]
- Tower-Rader A, Mohananey D, To A, et al. Prognostic Value of Global Longitudinal Strain in Hypertrophic Cardiomyopathy: A Systematic Review of Existing Literature. JACC Cardiovasc Imaging 2019;12:1930-42. [Crossref] [PubMed]
- Marian AJ, Braunwald E. Hypertrophic Cardiomyopathy: Genetics, Pathogenesis, Clinical Manifestations, Diagnosis, and Therapy. Circ Res 2017;121:749-70. [Crossref] [PubMed]
- O'Mahony C, Jichi F, Pavlou M, et al. A novel clinical risk prediction model for sudden cardiac death in hypertrophic cardiomyopathy (HCM risk-SCD). Eur Heart J 2014;35:2010-20. [Crossref] [PubMed]
- Authors/Task Force members. 2014 ESC Guidelines on diagnosis and management of hypertrophic cardiomyopathy: the Task Force for the Diagnosis and Management of Hypertrophic Cardiomyopathy of the European Society of Cardiology (ESC). Eur Heart J 2014;35:2733-79. [Crossref] [PubMed]
- Ommen SR, Mital S, Burke MA, et al. 2020 AHA/ACC Guideline for the Diagnosis and Treatment of Patients With Hypertrophic Cardiomyopathy: Executive Summary: A Report of the American College of Cardiology/American Heart Association Joint Committee on Clinical Practice Guidelines. Circulation 2020;142:e533-57. [PubMed]
- Chan RH, Maron BJ, Olivotto I, et al. Prognostic value of quantitative contrast-enhanced cardiovascular magnetic resonance for the evaluation of sudden death risk in patients with hypertrophic cardiomyopathy. Circulation 2014;130:484-95. [Crossref] [PubMed]
- Habib M, Adler A, Fardfini K, et al. Progression of Myocardial Fibrosis in Hypertrophic Cardiomyopathy: A Cardiac Magnetic Resonance Study. JACC Cardiovasc Imaging 2021;14:947-58. [Crossref] [PubMed]
- Mahrholdt H, Seitz A. Refining the Prognostic Value of LGE in Hypertrophic Cardiomyopathy: Presence, Extent, and Location-What's Next? JACC Cardiovasc Imaging 2023;16:1178-80. [Crossref] [PubMed]
- Maron MS. Clinical utility of cardiovascular magnetic resonance in hypertrophic cardiomyopathy. J Cardiovasc Magn Reson 2012;14:13. [Crossref] [PubMed]
- Sedmera D, Pexieder T, Hu N, et al. Developmental changes in the myocardial architecture of the chick. Anat Rec 1997;248:421-32. [Crossref] [PubMed]
- Captur G, Muthurangu V, Cook C, et al. Quantification of left ventricular trabeculae using fractal analysis. J Cardiovasc Magn Reson 2013;15:36. [Crossref] [PubMed]
- van Waning JI, Caliskan K, Hoedemaekers YM, et al. Genetics, Clinical Features, and Long-Term Outcome of Noncompaction Cardiomyopathy. J Am Coll Cardiol 2018;71:711-22. [Crossref] [PubMed]
- Captur G, Zemrak F, Muthurangu V, et al. Fractal Analysis of Myocardial Trabeculations in 2547 Study Participants: Multi-Ethnic Study of Atherosclerosis. Radiology 2015;277:707-15. [Crossref] [PubMed]
- Captur G, Lopes LR, Patel V, et al. Abnormal cardiac formation in hypertrophic cardiomyopathy: fractal analysis of trabeculae and preclinical gene expression. Circ Cardiovasc Genet 2014;7:241-8. [Crossref] [PubMed]
- Wang J, Li Y, Yang F, et al. Fractal Analysis: Prognostic Value of Left Ventricular Trabecular Complexity Cardiovascular MRI in Participants with Hypertrophic Cardiomyopathy. Radiology 2021;298:71-9. [Crossref] [PubMed]
- Norman G, Monteiro S, Salama S. Sample size calculations: should the emperor's clothes be off the peg or made to measure? BMJ 2012;345:e5278. [Crossref] [PubMed]
- Dawes TJW, Cai J, Quinlan M, et al. Fractal Analysis of Right Ventricular Trabeculae in Pulmonary Hypertension. Radiology 2018;288:386-95. [Crossref] [PubMed]
-
Cai J. UK-Digital-Heart-Project/fracAnalyse: fracAnalyse v1.2. 2017 . Available online: https://zenodo.org/records/837246 - Zheng T, Ma X, Li S, et al. Value of Cardiac Magnetic Resonance Fractal Analysis Combined With Myocardial Strain in Discriminating Isolated Left Ventricular Noncompaction and Dilated Cardiomyopathy. J Magn Reson Imaging 2019;50:153-63. [Crossref] [PubMed]
- Krupickova S, Hatipoglu S, DiSalvo G, et al. Left ventricular noncompaction in pediatric population: could cardiovascular magnetic resonance derived fractal analysis aid diagnosis? J Cardiovasc Magn Reson 2021;23:90. [Crossref] [PubMed]
- Budczies J, Klauschen F, Sinn BV, et al. Cutoff Finder: a comprehensive and straightforward Web application enabling rapid biomarker cutoff optimization. PLoS One 2012;7:e51862. [Crossref] [PubMed]
- Vriesendorp PA, Schinkel AF, Liebregts M, et al. Validation of the 2014 European Society of Cardiology guidelines risk prediction model for the primary prevention of sudden cardiac death in hypertrophic cardiomyopathy. Circ Arrhythm Electrophysiol 2015;8:829-35. [Crossref] [PubMed]
- Salerno M, Kramer CM. Prognosis in hypertrophic cardiomyopathy with contrast-enhanced cardiac magnetic resonance: the future looks bright. J Am Coll Cardiol 2010;56:888-9. [Crossref] [PubMed]
- Maron BJ. Contemporary insights and strategies for risk stratification and prevention of sudden death in hypertrophic cardiomyopathy. Circulation 2010;121:445-56. [Crossref] [PubMed]
- Eidem BW. Noninvasive evaluation of left ventricular noncompaction: what's new in 2009?. Pediatr Cardiol 2009;30:682-9. [Crossref] [PubMed]
- Mohun TJ, Weninger WJ. Imaging heart development using high-resolution episcopic microscopy. Curr Opin Genet Dev 2011;21:573-8. [Crossref] [PubMed]
- Meyer HV, Dawes TJW, Serrani M, et al. Genetic and functional insights into the fractal structure of the heart. Nature 2020;584:589-94. [Crossref] [PubMed]