Screening plasma metabolites as potential biomarkers for type B aortic dissection
Highlight box
Key findings
• Three plasma metabolites, including 1,5-anhydro-D-glucitol, D-(+)-sucrose and PC(O-16:0/0:0), were associated with the risk of type B aortic dissection (TBAD).
• Hydrocinnamic acid, glycine deoxycholic acid and glycochenodeoxycholic acid were independently associated with TBAD severity.
What is known and what is new?
• Previous studies have identified several potential biomarkers for aortic dissection.
• The TBAD risk prediction model and the biomarkers for the severity of TBAD have not been reported before.
What is the implication, and what should change now?
• This research aimed to identify biomarkers related to the risk and severity of TBAD, which also implies the potential mechanism of this disease.
Introduction
Aortic dissection (AD) is a life-threatening emergency with an incidence of 4.4–10 per 100,000 person-years (1-3), and 30 days and 5 years mortality rates of 55.8% and 64.5%, respectively (4). Imaging studies including computed tomography, magnetic resonance imaging, and echocardiography are the most important diagnostic methods for AD, while laboratory tests serve as necessary supplementary methods for the diagnosis (5). D-dimer is significantly elevated in patients with AD, and is therefore widely used (6,7). However, its specificity is low as it is also elevated in pulmonary embolism and ischemic heart diseases. Furthermore, the specific pathogenesis of AD remains elusive. Therefore, it is of great importance to search for novel AD related biomarkers to explore the pathogenesis.
Metabolites revealed by metabolomics have been identified as potent biomarkers for AD. Metabolites may change after diseases onset and progression, which plays an important role in distinguishing diseases from health states, including indicating the severity and revealing the specific mechanisms (8). Zhou and colleagues reported that the level of lysophosphatidylcholines significantly decreased in the plasma of acute AD patients compared to that of healthy individuals (9). Several metabolites, including sphinganine, phytosphingosine and ceramide, were identified as the potential biomarkers to differentiate between Stanford type A and B AD. Wang et al. reported that the concentrations of plasma amino acids are different between patients with AD and coronary heart disease (10). Although most of these studies reported changes in certain kinds of metabolites, very few focused on their predictive value for the severity of AD. Thus, in this study we utilized metabolomics to unbiasedly screen for metabolites that could differentiate hypertensive patients with or without Society for Vascular Surgery/Society of Thoracic Surgeons (SVS/STS) classification type B AD (TBAD). We subsequently constructed a metabolites risk score (MRS) to predict the risk of TBAD. Furthermore, we screened out three metabolites which could indicate the severity of TBAD. In this article, we aimed to find out the metabolites related to the risk and severity of TBAD, and shed light on exploring underlying mechanisms of AD. We present this article in accordance with the STROBE reporting checklist (available at https://cdt.amegroups.com/article/view/10.21037/cdt-23-183/rc).
Methods
Study design and subjects
A total of 16 hypertensive patients with SVS/STS classification TBAD (9 with uncomplicated and 7 with complicated TBAD) and 7 hypertensive patients without TBAD were included in this cross-sectional study. All patients were enrolled consecutively from December 2021 to April 2022 from the Department of Cardiology, Jieyang People’s Hospital. The diagnosis of TBAD was confirmed by computed tomography and classified according to SVS/STS AD classification system published in 2020 (6). All patients with TBAD were admitted due to an episode of chest and/or back pain and the plasma was collected and separated within 48 h after symptom onset. The severity of AD was also classified by the SVS/STS AD classification system: uncomplicated TBAD and complicated TBAD were defined as the dissection without or with the evidence of rupture or end-organ malperfusion, respectively (6). All 7 patients with complicated TBAD demonstrated malperfusion syndrome, while 9 patients with uncomplicated TBAD demonstrated neither rupture or end-organ malperfusion. Medical information of patients was extracted from medical records. TBAD patients without the history of neoplasm, autoimmune or inflammatory systemic disease were included, while those with Marfan syndrome, connective tissue diseases, intramural hematoma and penetrating atherosclerotic ulcer were excluded. The diagnosis of hypertension followed the criteria recommended by World Health Organization (WHO) in 2021 (11). The plasma of hypertension patients without TBAD was collected and separated at physical examination.
The protocol was approved by the ethics committee of Jieyang People’s Hospital (No. 2021108) and conformed to the principles of the Declaration of Helsinki (as revised in 2013). The informed consent was obtained from all individual participants included in the study.
Plasma sample collection
Peripheral venous blood samples were collected in vacuum anticoagulant tubes and subsequently centrifuged at 3,000 g for 15 min at 4 ℃. Plasma was immediately separated and stored at −80 ℃ for widely targeted metabolomics.
Clinical biochemistry measurements
Plasma biochemical indexes, including creatinine, urea nitrogen, aspartate transaminase (AST), and alanine transaminase (ALT), were carried out by the 7600 automatic biochemical analyzer (Hitachi, Tokyo, Japan).
Metabolomics analysis
Sample preparation
The plasma samples were thawed on ice and vortexed for 10 s. Then, 50 µL samples were mixed with 300 µL extraction solution (Acetonitrile: Methanol =1:4, V/V) containing internal standards and centrifuged at 12,000 rpm for 10 min (4 ℃); 200 µL supernatant was collected and placed at −20 ℃ for 30 min, and subsequently centrifuged at 12,000 rpm for 3 min (4 ℃); 180 µL supernatant was collected for liquid chromatography-mass spectrometry (LC-MS) analysis.
LC-MS analysis
The plasma samples were analyzed by the LC-ESI-MS/MS system (ExionLC AD, SCIEX, California, USA; QTRAP® system, SCIEX, California, USA). The ultra performance liquid chromatography-tandem mass spectrometer (UPLC-MS/MS) was performed to identify the metabolites in each sample by detecting the retention time, precursor/product ion pairs, and secondary mass spectrum data, and subsequently comparing the acquired information with the metabolite from the Metware database which includes reference compound information. Then, quantitative data were obtained via calculating the peak area of the characteristic ions formed in the mass spectrometer. Raw data were analyzed by Analyst 1.6.3 software (SCIEX, California, USA).
Data processing
The acquired LC-MS data was performed by log transformation (log2) and mean centering, and the orthogonal partial least squares-discriminant analysis (OPLS-DA) was subsequently performed using R package MetaboAnalystR (12). To identify the differential metabolites between hypertensive patients with or without TBAD, the screen criteria were determined by variable importance in projection (VIP, VIP ≥1) and fold change (fold change >2 or fold change <1/2). To identify the differential metabolites among patients with only hypertension, uncomplicated TBAD or complicated TBAD, the screen criteria were determined by VIP (VIP ≥0.9) and fold change (fold change >1.5 or fold change <2/3). VIP values were extracted from OPLS-DA results.
Enrichment analysis
Metabolic pathway enrichment analysis was based on small molecule pathway database (SMPDB) (13). The significance of the pathways was determined by hypergeometric test’s P value.
K-means clustering analysis
K-means clustering analysis of the differential metabolites was performed by using R package base package. The data were transformed by unit variance scaling before K-means.
MRS
First, the unadjusted binary logistic regression analysis was performed by the differential metabolites for preliminary screening. The multivariate binary logistic regression analysis was further used to identify the differential metabolites associated with TBAD by adjusting for age, gender and body mass index (BMI). The data were performed by log2 before logistic regression analysis.
Second, the selected metabolites were implemented by least absolute shrinkage and selection operator (LASSO) with tenfold cross validation to build MRS for diagnosis of TBAD (14). LASSO regression has unique advantages for processing high-dimensional omics data. The largest λ value within 1 standard error of minimum mean cross-validated error was chosen to regulate the parameters of LASSO. LASSO was performed by R package glmnet (15). MRS was built based on the metabolites with independent predict values, and the formula was as follows:
MRS = constant term + (Coefficient metabolite 1 * Log2 abundance of metabolite 1) + (Coefficient metabolite 2 * Log2 abundance of metabolite 2) + … + (Coefficient metabolite n * Log2 abundance of metabolite n).
The receiver operating characteristic (ROC) curve was used to assess the prediction accuracy of the MRS. The ROC curve was plotted by R package ROCR (16).
Statistical analysis
The demographic and medical data are presented using mean ± standard deviation or count (%). Continuous data were analyzed using Student’s t-test. Categorical data were analyzed by chi-square test. Spearman correlation analysis was used for correlation analysis between continuous variables and ordered variables. The identification of metabolites related to AD severity was completed by ordered logistic regression analysis. Data were analyzed by SPSS 22 (IBM, NY, USA). P<0.05 was considered statistically significant.
Results
Baseline characteristics and the metabolite profile of subjects
The flow chart of the study was shown in Figure 1. The baseline characteristics of 16 hypertensive patients with TBAD and 7 hypertensive patients without TBAD are presented in Table 1. None of the participants had diabetes or coronary heart disease. No significant differences were found for age, BMI, systolic blood pressure and diastolic blood pressure between the two groups. There were no significant differences in plasma creatinine, urea nitrogen, ALT and AST between the two groups.
Table 1
Parameters | TBAD (n=16) | Control (n=7) | P |
---|---|---|---|
Age, years | 58.8±11.96 | 60.7±7.68 | 0.70 |
Male gender | 12 (75.0) | 6 (85.7) | >0.99 |
BMI, kg/m2 | 23.6±3.44 | 22.8±1.26 | 0.56 |
Systolic blood pressure, mmHg | 187.1±34.66 | 159.4±17.88 | 0.06 |
Diastolic blood pressure, mmHg | 96.7±16.46 | 87.9±13.02 | 0.22 |
Creatinine, μmol/L | 94.1±45.17 | 73.0±10.20 | 0.24 |
BUN, mmol/L | 6.1±2.29 | 5.0±0.90 | 0.23 |
ALT, U/L | 17.7± 7.78 | 19.6±7.50 | 0.60 |
AST, U/L | 22.1±8.09 | 18.0±4.44 | 0.22 |
Data are illustrated as mean ± standard deviation and n (%). TBAD, type B aortic dissection; BMI, body mass index; BUN, blood urea nitrogen; ALT, alanine aminotransferase; AST, aspartate transaminase.
Widely targeted metabolomics were used to describe plasma metabolite profiles of different groups and identify differential plasma metabolites in patients with TBAD. The OPLA-DA demonstrated significantly different clusters between hypertensive patients with or without TBAD (Figure 2A). Based on the criteria of VIP (VIP ≥1) and fold change (fold change >2 or fold change <1/2), 60 metabolites were upregulated, while 70 metabolites were downregulated in the TBAD group compared to the control group, as visualized by volcano plot (Figure 2B). The relative abundance of the differential metabolites in each sample was illustrated by the heat map in Figure 2C. These differential metabolites could be divided into 15 categories (Figure 2D), including amino acid and its metabolites, organic acid and its derivatives, benzene and substituted derivatives, and carbohydrate and its metabolites. Among them, amino acid and its metabolites, which included 29 metabolites, accounted for the highest proportion. SMPDB pathway enrichment analysis was performed for the differential metabolites involving metabolic pathways. As shown in Figure 2E, the top 10 significantly enriched metabolic pathways, including glutathione metabolism, galactose metabolism, and glycerolipid metabolism, were identified based on the rich factor.
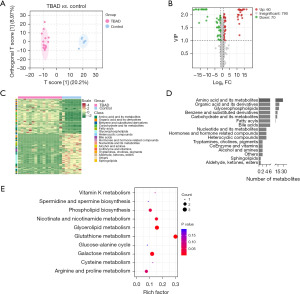
Construction of MRS model
In order to further identify metabolites associated with TBAD, 130 differential metabolites were implemented by binary logistic regression models (Table 2). The unadjusted model was performed by the differential metabolites only and we found that 55 metabolites were significantly associated with TBAD. Then, we used an adjusted model adjusting for age, gender and BMI, and found that 40 metabolites were still significantly associated with TBAD (Table 2). LASSO regression model was subsequently performed to select diagnostic metabolites to predict the risk of TBAD (Figure 3A,3B) and construct the MRS model. When we set λ to the largest λ value within 1 standard error of minimum mean cross-validated error, we identified 3 significant metabolites including 1,5-anhydro-D-glucitol, D-(+)-sucrose and PC(O-16:0/0:0), while the regression coefficients of other metabolites were shrunk to zero. Thus, we established the MRS based on the 3 metabolites: MRS = 0.3024 + (0.0270 * log2 1,5-anhydro-D-glucitol) + [0.0425 * log2 D-(+)-sucrose] + [−0.0388 * log2 PC(O-16:0/0:0)]. The MRS of TBAD group was significantly higher than that of the control group (Figure 3C). The abundance of 1,5-anhydro-D-glucitol and D-(+)-sucrose were significantly increased (Figure 3D,3E) while PC(O-16:0/0:0) was significantly reduced (Figure 3F) in TBAD group. ROC curve was performed to assess the prediction accuracy of the MRS with an area under ROC curve (AUC) of 0.98214 (Figure 3G).
Table 2
Compounds | Unadjusted model | Adjusted model* | |||||
---|---|---|---|---|---|---|---|
OR | 95% CI | P | OR | 95% CI | P | ||
1,5-anhydro-D-glucitol | 10.726 | (1.27, 90.76) | 0.029 | 14.642 | (1.10, 194.17) | 0.042 | |
1-pyrroline-4-hydroxy-2-carboxylate | 0.513 | (0.33, 0.81) | 0.004 | 0.374 | (0.17, 0.83) | 0.016 | |
2'-deoxycytidine-5'-monophosphate | 0.449 | (0.26, 0.79) | 0.005 | – | – | – | |
2-methylsuccinic acid | 7.945 | (1.75, 36.11) | 0.007 | 13.824 | (1.54, 123.98) | 0.019 | |
3-hydroxyglutaric acid | 435.797 | (1.39, 136,380.70) | 0.038 | – | – | – | |
5-oxoproline | 0.447 | (0.25, 0.80) | 0.007 | 0.194 | (0.04, 0.999) | 0.0498 | |
Ala-Lys | 0.344 | (0.17, 0.71) | 0.004 | – | – | – | |
Biliverdin | 12.838 | (1.06, 154.79) | 0.045 | – | – | – | |
Carnitine C8:0 | 0.112 | (0.02, 0.60) | 0.011 | 0.090 | (0.01, 0.63) | 0.015 | |
Carnitine C9:0 | 0.166 | (0.03, 0.84) | 0.030 | 0.106 | (0.01, 0.78) | 0.028 | |
Coenzyme-II (β-NADP) | 70.658 | (1.01, 4,949.04) | 0.0495 | – | – | – | |
Cycloleucine | 0.281 | (0.12, 0.65) | 0.003 | 0.098 | (0.01, 0.98) | 0.048 | |
D-(+)-cellobiose | 4.101 | (1.06, 15.94) | 0.042 | – | – | – | |
D-(+)-sucrose | 4.681 | (1.42, 15.43) | 0.011 | 5.170 | (1.39, 19.29) | 0.015 | |
D-mannitol | 1.649 | (1.18, 2.30) | 0.003 | 2.129 | (1.13, 4.02) | 0.020 | |
D-sorbitol | 1.649 | (1.18, 2.30) | 0.003 | 2.129 | (1.13, 4.02) | 0.020 | |
D-cellobiose | 2.728 | (1.19, 6.25) | 0.018 | – | – | – | |
D-piperidine acid | 0.509 | (0.32, 0.80) | 0.004 | 0.293 | (0.09, 0.91) | 0.034 | |
Dimethylmalonic acid | 7.945 | (1.75, 36.11) | 0.007 | 13.824 | (1.54, 123.98) | 0.019 | |
Dipyrocetyl | 0.062 | (0.01, 0.57) | 0.014 | 0.014 | (0.0003, 0.58) | 0.025 | |
Dulcitol | 1.649 | (1.18, 2.30) | 0.003 | 2.129 | (1.13, 4.02) | 0.020 | |
Ethyl hydrogen malonate | 7.945 | (1.75, 36.11) | 0.007 | 13.824 | (1.54, 123.98) | 0.019 | |
Galactinol hydrate | 4.510 | (1.43, 14.25) | 0.010 | 8.746 | (1.04, 73.55) | 0.046 | |
Galactitol | 1.626 | (1.18, 2.24) | 0.003 | 2.118 | (1.11, 4.05) | 0.023 | |
Glu-Ile | 0.373 | (0.17, 0.83) | 0.016 | 0.316 | (0.12, 0.83) | 0.019 | |
Gluceptate | 37.439 | (1.98, 706.79) | 0.016 | – | – | – | |
Glutaric acid | 7.945 | (1.75, 36.11) | 0.007 | 13.824 | (1.54, 123.98) | 0.019 | |
Glycine deoxycholic acid | 0.413 | (0.19, 0.92) | 0.031 | 0.391 | (0.16, 0.94) | 0.037 | |
Glycochenodeoxycholic acid | 0.405 | (0.18, 0.92) | 0.032 | 0.382 | (0.15, 0.95) | 0.038 | |
Glycohyodeoxycholic acid | 0.211 | (0.06, 0.78) | 0.020 | 0.222 | (0.06, 0.82) | 0.024 | |
Glycoursodeoxycholic acid | 0.188 | (0.05, 0.77) | 0.021 | 0.195 | (0.05, 0.81) | 0.025 | |
Hyodeoxycholic acid | 0.387 | (0.15, 0.99) | 0.048 | 0.356 | (0.13, 0.998) | 0.049 | |
Hypoxanthine | 10.642 | (1.51, 75.01) | 0.018 | 158.428 | (1.33, 18,894.16) | 0.038 | |
Ile-Glu | 0.373 | (0.17, 0.83) | 0.016 | 0.316 | (0.12, 0.83) | 0.019 | |
Ile-Lys | 0.401 | (0.21, 0.75) | 0.004 | 0.191 | (0.04, 0.95) | 0.043 | |
L-rhamnose | 10.726 | (1.27, 90.76) | 0.029 | 14.642 | (1.10, 194.17) | 0.042 | |
LPC(0:0/14:0) | 0.008 | (0.0001, 0.46) | 0.020 | – | – | – | |
LPC(12:0/0:0) | 0.221 | (0.06, 0.80) | 0.022 | 0.079 | (0.01, 0.85) | 0.036 | |
LPC(O-0:0/18:0) | 0.050 | (0.004, 0.58) | 0.017 | 0.042 | (0.003, 0.59) | 0.019 | |
LPC(O-18:0/0:0) | 0.050 | (0.004, 0.58) | 0.017 | 0.042 | (0.003, 0.59) | 0.019 | |
Leu-Glu | 0.373 | (0.17, 0.83) | 0.016 | 0.316 | (0.12, 0.83) | 0.019 | |
Lys-Ala | 0.344 | (0.17, 0.71) | 0.004 | – | – | – | |
Lys-Leu | 0.401 | (0.21, 0.75) | 0.004 | 0.191 | (0.04, 0.95) | 0.043 | |
Nicotinamide | 0.020 | (0.001, 0.66) | 0.028 | – | – | – | |
PC(O-16:0/0:0) | 0.005 | (0.00004, 0.61) | 0.031 | 0.004 | (0.00004, 0.48) | 0.024 | |
PC(O-16:0/O-2:0) | 0.050 | (0.0043, 0.58) | 0.017 | 0.042 | (0.003, 0.59) | 0.019 | |
Phe-Ala | 0.084 | (0.01, 0.71) | 0.023 | 0.059 | (0.01, 0.69) | 0.024 | |
Pro-Asn | 179.708 | (1.76, 18,384.06) | 0.028 | – | – | – | |
Pro-Asp | 237.465 | (1.59, 35,441.49) | 0.032 | – | – | – | |
Pyroglutamic acid | 0.513 | (0.33, 0.79) | 0.003 | – | – | – | |
Pyrroloquinoline quinone | 0.03 | (0.001, 0.92) | 0.045 | 0.009 | (0.0002, 0.55) | 0.025 | |
S-methyl-L-cysteine-S-oxide | 0.323 | (0.12, 0.84) | 0.021 | – | – | – | |
Taurolithocholic acid | 0.509 | (0.27, 0.95) | 0.032 | 0.141 | (0.02, 0.84) | 0.031 | |
Uridine-5'-diphospho-N-acetylgalactosamine disodium salt | 57.448 | (1.50, 2,204.39) | 0.030 | 51.435 | (1.41, 1,880.39) | 0.032 | |
Cyclo(glu-glu) | 0.608 | (0.44, 0.85) | 0.003 | 0.501 | (0.28, 0.90) | 0.021 |
*, adjusted model adjusted for age, gender and BMI. OR, odds ratio; TBAD, type B aortic dissection; CI, confidence interval; BMI, body mass index.
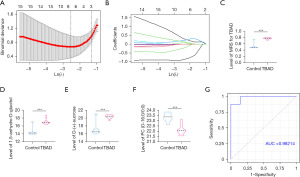
Screening for the differential metabolites for severity of TBAD
To further identify the metabolites that could distinguish the severity of AD, we divided TBAD patients into uncomplicated and complicated groups (9 with uncomplicated and 7 with complicated TBAD) and compared the control group with these two groups in pairs. The OPLA-DA demonstrated significantly different clusters between the control group and the uncomplicated TBAD group (Figure 4A) or the complicated TBAD group (Figure 4B). The uncomplicated TBAD group was also significantly distinguished from the complicated TBAD group (Figure 4C). Then, we subsequently used volcano plots to demonstrate the differential metabolites. Based on the criteria of VIP (VIP ≥0.9) and fold change (fold change >1.5 or fold change <2/3), 92 metabolites were upregulated while 127 metabolites were downregulated in the uncomplicated TBAD group (Figure 4D), and 107 metabolites were upregulated while 139 metabolites were downregulated in the complicated TBAD group (Figure 4E) when compared with the control group. Compared with the uncomplicated TBAD group, 72 metabolites were upregulated while 21 metabolites were downregulated in the complicated TBAD group (Figure 4F). We subsequently used VENN plot to further screen the metabolites, and 16 differential metabolites were identified after comparing the three groups in pairs (Figure 4G). K-means cluster analysis was used to cluster the 16 metabolites (Figure 4H). When we set the subclass to three, each cluster could be distinguished from other clusters. We found that the abundance of two metabolites, glycine deoxycholic acid and glycochenodeoxycholic acid, gradually decreased in parallel with the severity of TBAD (subclass 1). The abundance of one metabolite, hydrocinnamic acid gradually increased in parallel with the severity of TBAD (one of three metabolites in subclass 3). Tandem mass spectrometry figures of the metabolites were shown in the Figure S1.
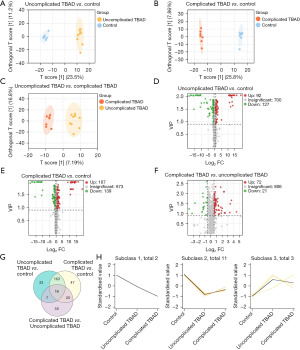
Identification of metabolites for the severity of TBAD
Correlation analysis showed that hydrocinnamic acid, glycine deoxycholic acid and glycochenodeoxycholic acid were significantly correlated with the severity of TBAD (Figure 5A-5C). Then, we used ordered logistic regression model after adjusting for gender, age and BMI. As shown in Figure 5D, the increasing abundance of hydrocinnamic acid as well as the decreasing abundance of glycine deoxycholic acid and glycochenodeoxycholic acid were associated with a higher risk of severe TBAD. Therefore, we concluded that hydrocinnamic acid was independently correlated with the severity of TBAD, while glycine deoxycholic acid and glycochenodeoxycholic acid were inversely correlated with the severity of TBAD independently.
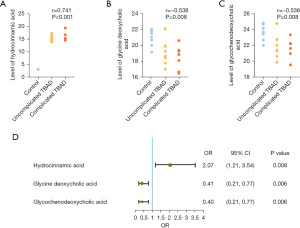
Discussion
AD is a life-threatening disease with a high mortality rate. Imaging tests are the main tools to confirm the diagnosis and predict the prognosis, while reliable diagnostic and predictive biomarkers are scarce (5). Therefore, it is of importance to identify biomarkers related to AD. Using metabolomics, we found that plasma metabolite profile of hypertensive patients with TBAD were significantly different from those in hypertensive patients without TBAD. We screened out three plasma metabolites associated with TBAD disease, and built an MRS model to predict the risk of TBAD. We subsequently identified three metabolites to indicate the severity of AD.
The plasma metabolites were significantly different between hypertensive patients with or without AD (17). How to screen specific metabolites in high dimensional metabolomics data is of great significance. We identified three plasma metabolites, including 1,5-anhydro-D-glucitol, D-(+)-sucrose and PC(O-16:0/0:0), to distinguish AD in an unbiased manner. Previous studies have suggested that some of these metabolites were associated with cardiovascular events. 1,5-anhydro-D-glucitol serves as a sensitive clinical marker of short-term glycemic status (18). Some studies have reported that it was associated with coronary artery diseases (19,20). Sucrose is a human metabolite and an important added sugar in our lives. Yang et al. reported a significant relationship between sugar consumption and increased risk for cardiovascular disease mortality (21). PC(O-16:0/0:0) is derived from lysophosphatidylcholine. Zhou et al. illustrated that many kinds of lysophosphatidylcholine, such as LysoPC(16:0), LysoPC(16:1(9Z)) and LysoPC(18:0) were decreased in acute Stanford type A and B AD (9). However, the relationship between these three metabolites and TBAD has not been reported. In this study, we proposed the combination of these three metabolites as a predictor for the risk of TBAD in hypertensive patients, and built an MRS model. Nevertheless, it is necessary to enroll more samples to verify this model. Moreover, exploring the role of these metabolites in TBAD is of great importance to further understand the pathogenesis of AD in future studies.
Interestingly, we found that one metabolite, hydrocinnamic acid, was increased and two metabolites, glycine deoxycholic acid and glycochenodeoxycholic acid, were decreased in parallel with TBAD severity. Few studies demonstrated the effect of hydrocinnamic acid on AD. In patients with AD, whether the metabolism of hydrocinnamic acid is abnormal warrants further study. Glycine deoxycholic acid and glycochenodeoxycholic acid are two types of bile acids, which might reduce the risk of cardiovascular diseases. It was reported that ursodeoxycholic acid could alleviate the formation of AD in in vivo experiments (22), deoxycholylglycine could reduce vascular tone in in vitro experiments (23), while glycoursodeoxycholic acid could reduce atherosclerosis via gut microbiota mediated pathways (24). Our results also illustrated that the abundance of glycine deoxycholic acid, glycochenodeoxycholic acid, glycoursodeoxycholic acid and glycohyodeoxycholic acid were significantly reduced in patients with TBAD. Thus, the reduction of bile acids might aggravate TBAD. Nuclear farnesoid X receptor and membrane Takeda G protein-coupled receptor 5 are bile acid-related receptors, which could maintain glucose homeostasis in metabolic syndrome (25,26) and exert anti-inflammation effects on atherosclerosis (27). Therefore, we speculated that the increased severity of TBAD caused by decreased bile acids might be related to the reduction of bile acid receptor activation, which needs to be validated in future studies.
Nevertheless, this study is limited by a relatively small number of subjects included, and the changes of these metabolites need to be verified in larger scale studies in the future. Moreover, we cannot obtain the exact concentrations of the metabolites in the blood via widely targeted metabolomics, which should be addressed in the future. Finally, the MRS model is only self-verified in our data, an external verification is needed in future study.
Conclusions
In this study, we screened out three plasma metabolites associated with the risk of TBAD and constructed an MRS model. Subsequently, we identified three metabolites that were independently correlated with the severity of TBAD. This research may shed light on identifying more AD related biomarkers, exploring mechanisms of AD, and searching for potential therapeutic targets.
Acknowledgments
We would like to thank Wayne Zhang for his help in polishing our paper.
Funding: This work was supported by the grants of National Natural Science Foundation of China (No. 81970406), Guangdong Science and Technology Department (No. 2020A1515011471), and Guangzhou Science and Technology Program Key Projects (No. 202206010031).
Footnote
Reporting Checklist: The authors have completed the STROBE reporting checklist. Available at https://cdt.amegroups.com/article/view/10.21037/cdt-23-183/rc
Data Sharing Statement: Available at https://cdt.amegroups.com/article/view/10.21037/cdt-23-183/dss
Peer Review File: Available at https://cdt.amegroups.com/article/view/10.21037/cdt-23-183/prf
Conflicts of Interest: All authors have completed the ICMJE uniform disclosure form (available at https://cdt.amegroups.com/article/view/10.21037/cdt-23-183/coif). The authors have no conflicts of interest to declare.
Ethical Statement: The authors are accountable for all aspects of the work in ensuring that questions related to the accuracy or integrity of any part of the work are appropriately investigated and resolved. The study was conducted in accordance with the Declaration of Helsinki (as revised in 2013) and was approved by the ethics committee of Jieyang People’s Hospital (No. 2021108). The informed consent was obtained from all individual participants included in the study.
Open Access Statement: This is an Open Access article distributed in accordance with the Creative Commons Attribution-NonCommercial-NoDerivs 4.0 International License (CC BY-NC-ND 4.0), which permits the non-commercial replication and distribution of the article with the strict proviso that no changes or edits are made and the original work is properly cited (including links to both the formal publication through the relevant DOI and the license). See: https://creativecommons.org/licenses/by-nc-nd/4.0/.
References
- DeMartino RR, Sen I, Huang Y, et al. Population-Based Assessment of the Incidence of Aortic Dissection, Intramural Hematoma, and Penetrating Ulcer, and Its Associated Mortality From 1995 to 2015. Circ Cardiovasc Qual Outcomes 2018;11:e004689. [Crossref] [PubMed]
- Vilacosta I, San Román JA, di Bartolomeo R, et al. Acute Aortic Syndrome Revisited: JACC State-of-the-Art Review. J Am Coll Cardiol 2021;78:2106-25. [Crossref] [PubMed]
- Baman JR, Malaisrie SC. What Is Aortic Dissection? JAMA 2023;330:198. [Crossref] [PubMed]
- Howard DP, Banerjee A, Fairhead JF, et al. Population-based study of incidence and outcome of acute aortic dissection and premorbid risk factor control: 10-year results from the Oxford Vascular Study. Circulation 2013;127:2031-7. [Crossref] [PubMed]
- Isselbacher EM, Preventza O, Hamilton Black J 3rd, et al. 2022 ACC/AHA Guideline for the Diagnosis and Management of Aortic Disease: A Report of the American Heart Association/American College of Cardiology Joint Committee on Clinical Practice Guidelines. Circulation 2022;146:e334-482. [Crossref] [PubMed]
- Lombardi JV, Hughes GC, Appoo JJ, et al. Society for Vascular Surgery (SVS) and Society of Thoracic Surgeons (STS) reporting standards for type B aortic dissections. J Vasc Surg 2020;71:723-47. [Crossref] [PubMed]
- Suzuki T, Distante A, Zizza A, et al. Diagnosis of acute aortic dissection by D-dimer: the International Registry of Acute Aortic Dissection Substudy on Biomarkers (IRAD-Bio) experience. Circulation 2009;119:2702-7. [Crossref] [PubMed]
- Nicholson JK, Holmes E, Kinross JM, et al. Metabolic phenotyping in clinical and surgical environments. Nature 2012;491:384-92. [Crossref] [PubMed]
- Zhou X, Wang R, Zhang T, et al. Identification of Lysophosphatidylcholines and Sphingolipids as Potential Biomarkers for Acute Aortic Dissection via Serum Metabolomics. Eur J Vasc Endovasc Surg 2019;57:434-41. [Crossref] [PubMed]
- Wang L, Liu S, Yang W, et al. Plasma Amino Acid Profile in Patients with Aortic Dissection. Sci Rep 2017;7:40146. [Crossref] [PubMed]
- WHO Guidelines Approved by the Guidelines Review Committee. Guideline for the pharmacological treatment of hypertension in adults. Geneva: World Health Organization © World Health Organization 2021; 2021.
- Pang Z, Chong J, Li S, et al. MetaboAnalystR 3.0: Toward an Optimized Workflow for Global Metabolomics. Metabolites 2020;10:186. [Crossref] [PubMed]
- Jewison T, Su Y, Disfany FM, et al. SMPDB 2.0: big improvements to the Small Molecule Pathway Database. Nucleic Acids Res 2014;42:D478-84. [Crossref] [PubMed]
- Tibshirani R. Regression Shrinkage and Selection Via the Lasso. Journal of the Royal Statistical Society: Series B (Methodological) 1996;58:267-88. [Crossref]
- Friedman J, Hastie T, Tibshirani R. Regularization Paths for Generalized Linear Models via Coordinate Descent. J Stat Softw 2010;33:1-22. [Crossref] [PubMed]
- Sing T, Sander O, Beerenwinkel N, et al. ROCR: visualizing classifier performance in R. Bioinformatics 2005;21:3940-1. [Crossref] [PubMed]
- Doppler C, Arnhard K, Dumfarth J, et al. Metabolomic profiling of ascending thoracic aortic aneurysms and dissections - Implications for pathophysiology and biomarker discovery. PLoS One 2017;12:e0176727. [Crossref] [PubMed]
- Dungan KM, Buse JB, Largay J, et al. 1,5-anhydroglucitol and postprandial hyperglycemia as measured by continuous glucose monitoring system in moderately controlled patients with diabetes. Diabetes Care 2006;29:1214-9. [Crossref] [PubMed]
- Ikeda N, Hara H, Hiroi Y. Ability of 1,5-Anhydro-d-glucitol Values to Predict Coronary Artery Disease in a Non-Diabetic Population. Int Heart J 2015;56:587-91. [Crossref] [PubMed]
- Ikeda N, Hara H, Hiroi Y. 1,5-Anhydro-D-glucitol predicts coronary artery disease prevalence and complexity. J Cardiol 2014;64:297-301. [Crossref] [PubMed]
- Yang Q, Zhang Z, Gregg EW, et al. Added sugar intake and cardiovascular diseases mortality among US adults. JAMA Intern Med 2014;174:516-24. [Crossref] [PubMed]
- Liu W, Wang B, Wang T, et al. Ursodeoxycholic Acid Attenuates Acute Aortic Dissection Formation in Angiotensin II-Infused Apolipoprotein E-Deficient Mice Associated with Reduced ROS and Increased Nrf2 Levels. Cell Physiol Biochem 2016;38:1391-405. [Crossref] [PubMed]
- Jadeja RN, Thounaojam MC, Bartoli M, et al. Deoxycholylglycine, a conjugated secondary bile acid, reduces vascular tone by attenuating Ca(2+) sensitivity via rho kinase pathway. Toxicol Appl Pharmacol 2018;348:14-21. [Crossref] [PubMed]
- Huang K, Liu C, Peng M, et al. Glycoursodeoxycholic Acid Ameliorates Atherosclerosis and Alters Gut Microbiota in Apolipoprotein E-Deficient Mice. J Am Heart Assoc 2021;10:e019820. [Crossref] [PubMed]
- Sun L, Xie C, Wang G, et al. Gut microbiota and intestinal FXR mediate the clinical benefits of metformin. Nat Med 2018;24:1919-29. [Crossref] [PubMed]
- Zheng X, Chen T, Jiang R, et al. Hyocholic acid species improve glucose homeostasis through a distinct TGR5 and FXR signaling mechanism. Cell Metab 2021;33:791-803.e7. [Crossref] [PubMed]
- Pols TW, Nomura M, Harach T, et al. TGR5 activation inhibits atherosclerosis by reducing macrophage inflammation and lipid loading. Cell Metab 2011;14:747-57. [Crossref] [PubMed]