Clinical significance of carotid intima-media thickness and plasma homocysteine in acute ST-segment elevation myocardial infarction
Highlight box
Key findings
• The inclusion of homocysteine (Hcy), carotid intima-media thickness (CIMT), and carotid plaque in addition to traditional risk factors can more fully and accurately predict the risk of ST-segment elevation myocardial infarction (STEMI).
What is known and what is new?
• Hcy, CIMT and carotid plaque have been considered as independent risk factors for cardiovascular diseases;
• However, few studies have been conducted on the combination of CIMT and Hcy in acute coronary syndrome. It is particularly rare in Asian populations. We presented an interesting nomogram based on the results of the study which is feasible and can bring clinical net benefit.
What is the implication, and what should change now?
• The inclusion of Hcy, CIMT, and carotid plaque on the basis of traditional risk factors can significantly improve the diagnostic and predictive value of STEMI, and has certain significance for guiding clinical work. Clinicians and researchers should pay more attention to the collection and application of these indicators in the future.
Introduction
Cardiovascular disease (CVD) has gradually become one of the major diseases threatening human health. Its morbidity, disability rate and mortality rate are increasing year by year, and the age of onset is gradually getting younger, which brings a heavy burden to the family and country. These situations remind us that the prevention and treatment of coronary heart disease (CHD) is urgent. Current scoring systems for CVD risk, such as the Framingham risk Score, Atherosclerotic Cardiovascular Disease (ASCVD) (Atherosclerotic Cardiovascular Disease) risk score, Reynold Risk Score, etc., are based on a combination of traditional risk factors, including high blood pressure, high blood lipids, diabetes, and smoking. Research and clinical practice demonstrate that the accuracy of various scoring systems is still controversial (1-3). Firstly, new CVD risk factors have been discovered in recent years; secondly, the current risk scoring system does not include parameters that directly assess the vascular status of patients; and at present, interest in risk prediction is shifting from 10-year risk to lifetime risk. In light of this, we have been exploring more sensitive risk scoring systems for predicting CVD. Homocysteine (Hcy) has been newly discovered in recent years and is considered as an independent risk factor for CVDs (4-6). A large number of research data suggest that carotid intima-media thickness (CIMT) can be used as an independent predictor of CVDs. CIMT is considered to be a preclinical substitute marker for atherosclerotic burden and endothelial dysfunction, as well as a local manifestation of systemic atherosclerosis, and is often used as a window to evaluate the risk and progress of cardiovascular and cerebrovascular diseases (7-9). The combined detection of carotid plaque can further improve the prediction effect (10).
However, both Hcy and CIMT studies mainly involve follow-up studies in the study cohorts without CVD or explore the prognostic value of CIMT or Hcy in the prediction of cardiovascular events, recurrence, and death in the population with CVD. However, few studies have been conducted on the combination of CIMT and Hcy in acute coronary syndrome (ACS). It is particularly rare in Asian populations. As the most serious type of ACS, patients with acute ST-segment elevation myocardial infarction (STEMI) tend to have fewer identifiable traditional risk factors than those with other types of ACS, so it may be more difficult to identify STEMI risk groups using traditional risk assessment tools (11). In view of the above considerations, our study explored the predictive value of Hcy and CIMT for STEMI through a comparative study between STEMI and non-coronary populations, in an attempt to explore a more sensitive prediction model to improve the predictive ability of STEMI and better identify risk groups. We present this article in accordance with the STROBE reporting checklist (available at https://cdt.amegroups.com/article/view/10.21037/cdt-23-312/rc).
Methods
The study was conducted in accordance with the Declaration of Helsinki (as revised in 2013). The study was reviewed and approved by Hefei Hospital Affiliated to Anhui Medical University Ethics Committee (No. 2020-ke-058). Written informed consent for participation was not required for this retrospective study.
Study cohorts
This study was a retrospective case-control study. A total of 198 patients with STEMI were collected through the hospital’s case inquiry system, who received emergency coronary angiography in Hefei Hospital Affiliated to Anhui Medical University from January 2020 to January 2022 (see Figure 1). A total of 129 patients who received coronary angiography to exclude CHD were selected as the control group in the above hospitals during the same period. Exclusion criteria: (I) patients with previous myocardial infarction; patients who had received coronary bypass grafting or percutaneous coronary intervention; (II) having received heart transplantation; (III) no coronary angiography results and incomplete clinical data; (IV) complicated with malignant tumor; (V) combined with severe infection and liver and kidney insufficiency; (VI) previous use of lipid-regulating drugs.
Data collection and definition
General information (such as gender, age, height, weight, alcohol consumption, smoking, history of hypertension, and history of diabetes) and laboratory test results {such as blood routine [including white blood cells, hemoglobin, platelets (PLT), etc.], liver function, kidney function (including creatinine, urea nitrogen, etc.), electrolytes, myocardial enzymes, brain natriuretic peptide, uric acid (UA), Hcy, blood lipids [including triglycerides (TG), total cholesterol (TC), low-density lipoprotein cholesterol (LDL-C), very-low-density lipoprotein cholesterol (VLDL-C), high-density lipoprotein cholestasis (HDL-C), apolipoprotein B (ApoB), apolipoprotein A1 (ApoA1), fasting plasma glucose (FPG), 2 h postmeal blood glucose, glycosylated hemoglobin (HbA1c), etc.]} were collected through the hospital’s electronic medical record system. Among them, blood lipid, fasting blood glucose, HbA1c, Hcy and uric acid were all biochemical indexes determined by fasting blood sampling within 48 h after admission. Coronary angiography is performed by qualified interventional cardiologists. Coronary angiography results were evaluated by two experienced interventional coronary cardiologists. If there is any disagreement between the two, the results will be reinterpreted by a third professional interventional coronary cardiologist.
The diagnostic criteria for STEMI are based on the European Society of Cardiology/ACC/American Heart Association (AHA)/World Federation of Cardiology Common Definition of Myocardial Infarction, 4th Edition, 2018 (12). The definition of absence of coronary artery disease is stenosis of each branch of coronary artery cavity <50%, which include coronary atherosclerosis, coronary muscle Bridges, coronary slow flow, and complete coronary normality. Hypertension is defined as elevated systolic blood pressure (≥140 mmHg) or diastolic blood pressure (≥90 mmHg) or current use of antihypertensive medications. Diabetes was defined as diabetic symptoms (i.e., polyuria, polydipsia, unexplained weight loss) plus random blood glucose ≥11.1 mmol/L; or FPG ≥7.0 mmol/L; or plasma glucose ≥11.1 mmol/L after 2 hours of oral glucose tolerance test (OGTT); or those with a history of diabetes who are currently taking oral hypoglycemic drugs or receiving insulin therapy. Smoking history is defined as at least one cigarette a day for more than one year according to the World Health Organization (WHO) smoking survey criteria; or long-term smoking, smoking cessation less than half a year. Alcohol consumption history was defined as daily alcohol intake ≥25 g for adult males and ≥15 g for adult females or >100 g per week according to Chinese Guidelines for Healthy Lifestyle Prevention of Cardiovascular and Metabolic Diseases.
The ultrasonic examination of carotid artery was performed by the color Doppler ultrasound instrument ACUSON of Germany with a frequency of 10L4 probe (10–12 MHz), which was operated by the ultrasound department of our hospital. The subject was placed in the supine position, and the head was tilted to the opposite side of the examiner. The One-point measurement method was adopted: the maximum longitudinal section of the common carotid artery was selected, and the site was selected about 10 mm below the bifurcation of the bilateral common carotid artery. The thickness of the posterior wall was taken as the CIMT for bilateral CIMT measurement. The maximum CIMT value was selected to be included in the study. According to the American Society of Echocardiography (ASE) guideline consensus, carotid plaque is recommended to be defined as localized echo structure protruding lumen, or IMT ≥1.5 mm (with or without acoustic shadow) in any part of the carotid artery (13). The subjects were examined by two sonographers who had been working in ultrasound for more than 5 years and had received unified training. One person operated the machine, one person recorded, the two observed and judged the ultrasound images together, and the two checked and recorded the examination results in detail. If there was any dispute between the two, a third professional ultrasound doctor would re-examine the carotid ultrasound.
Statistical analysis
SPSS 25.0 software was used for statistical analysis. Continuous variables were tested for normality. Measurement data conforming to normal distribution were expressed as mean ± standard deviation (). Independent sample t-test was used for comparison between the two groups. The non-normal distribution data were represented by the median and percentile M (P25, P75), and the Mann-Whitney U test was used for comparison between groups. The classified data were expressed as number of cases and percentage, and the chi-squared test was used for comparison between groups. Univariate and multivariate logistic regression analyses were used to evaluate whether the study factors were independently associated with STEMI. The receiver operating characteristic curve (ROC) of single indicator and multi-indicator combined diagnosis were plotted to evaluate the clinical usefulness of the study factors or diagnostic models. P<0.05 was considered statistically significant.
Based on the multifactor regression model, a nomogram model is constructed to calculate the predicted value of individual outcome events. R Project for Statistical Computing 4.2.3 was used to establish and verify the nomogram model, and independent predictors derived from multifactor regression analysis were used for modeling. Two indexes of differentiation and calibration were used in model evaluation. Differentiation refers to the ability of the prediction model to distinguish groups with different risk levels of outcome events correctly. Calibration refers to the degree of consistency between the forecast risk and the actual risk. Both of them are important indicators to evaluate the quality of the forecast model and reflect the ability of the model to predict the absolute risk correctly. The differentiation was represented by the concordance index (C-index), equivalent to the area under the curve (AUC), which is an indicator of the accuracy of the model. The calibration was internally verified in the bootstrap resampling data, and the deviation generated by the model in the verification samples was evaluated by analyzing the model. The closer the predicted risk was to the actual risk, the better the model fit. The “rmda” package in R software was used to draw a clinical decision curve analysis (DCA) to evaluate the clinical efficacy of the nomogram model.
Results
Comparison of clinical data between STEMI group and control group
The comparison between the two groups showed that: STEMI group had a higher male proportion, smoking proportion, diabetes prevalence, creatinine level, LDL-C level, ApoB level, Hcy level, CIMT value, and carotid artery plaque detection rate, with statistical significance (P<0.05), and lower HDL-C level and ApoA1 level, with statistical significance (P<0.05). There were no significant differences in age, BMI, drinking history, hypertension, platelet count, UA, TG, VLDL-C and TC (P>0.05) (Table 1).
Table 1
Factor | STEMI group | Control group | t/χ2/z value | P value |
---|---|---|---|---|
Age (years) | 61.14±14.14 | 61.35±10.22 | −0.157 | 0.875 |
Gender male | 159 (80.30) | 79 (61.24) | 14.329 | <0.001* |
BMI (kg/m2) | 24.56±3.13 | 25.19±2.96 | −1.842 | 0.066 |
Smoking | 124 (62.63) | 36 (27.91) | 37.680 | <0.001* |
Drinking | 51 (25.76) | 28 (21.71) | 0.700 | 0.403 |
Hypertension | 108 (54.55) | 72 (55.81) | 0.051 | 0.822 |
Diabetes | 64 (32.32) | 19 (14.73) | 12.767 | <0.001* |
PLT (×109/L) | 206.36±66.49 | 197.69±55.88 | 1.225 | 0.222 |
Creatinine (μmol/L) | 76.30±27.02 | 68.04±17.46 | 3.357 | 0.001* |
UA (μmol/L) | 368.79±105.12 | 356.26±92.21 | 0.223 | 0.824 |
TG (mmol/L) | 1.54 (1.04, 2.37) | 1.35 (0.97, 1.91) | −1.737 | 0.082 |
TC (mmol/L) | 4.56±1.15 | 4.33±1.06 | 1.864 | 0.063 |
LDL-C (mmol/L) | 2.99±0.90 | 2.64±0.82 | 3.611 | <0.001* |
HDL-C (mmol/L) | 1.04±0.23 | 1.18±0.33 | −4.081 | <0.001* |
VLDL-C (mmol/L) | 0.31 (0.21, 0.48) | 0.28 (0.20, 0.39) | −1.493 | 0.135 |
ApoB (g/L) | 0.89±0.22 | 0.80±0.22 | 3.652 | <0.001* |
ApoA1 (g/L) | 1.05±0.19 | 1.17±0.23 | −5.215 | <0.001* |
HbA1c | 5.8 (5.3, 7.5) | 5.5 (5.1, 6.0) | −4.778 | <0.001* |
Hcy (μmol/L) | 14.39 (10.28, 21.05) | 10.03 (8.60, 12.48) | −7.001 | <0.001* |
CIMT (mm) | 0.96 (0.80, 1.03) | 0.80 (0.69, 0.90) | −8.079 | <0.001* |
Plaque | 144 (72.73) | 52 (40.31) | 34.184 | <0.001* |
Data are presented as mean ± standard deviation, M (P25, P75) or n (%). *, P<0.05. STEMI, ST-segment elevation myocardial infarction; BMI, body mass index; PLT, blood platelet; UA, uric acid; TG, triglycerides; TC, total cholesterol; LDL-C, low-density lipoprotein cholesterol; HDL-C, high-density lipoprotein cholesterol; VLDL-C, very-low-density lipoprotein cholesterol; ApoB, apolipoprotein B; ApoA1, apolipoprotein A1; HbA1c, glycosylated hemoglobin; Hcy, homocysteine; CIMT, carotid intima-media thickness.
Multi-factor logistics regression analysis results
Variables found to be significant at univariate analysis (smoking history, hypertension, diabetes mellitus, creatinine, HDL-C, LDL-C, Hcy, CIMT, carotid plaque detection) were included in the multivariate logistics regression analysis. The results showed that smoking (OR =3.541, 95% CI: 1.662–7.546, P=0.001), diabetes mellitus (OR =3.324, 95% CI: 1.554–7.112, P=0.002), LDL-C (OR =2.704, 95% CI: 1.785–4.094, P<0.001), Hcy (OR =1.161, 95% CI: 1.084–1.244, P<0.001), CIMT (OR =206.968, 95% CI: 22.375–1,914.468, P<0.001), carotid plaque (OR =2.499, 95% CI: 1.214–5.142, P=0.013) were independent risk factors or predictive factors for STEMI, and HDL-C (OR =0.079, 95% CI: 0.021–0.293, P<0.001) was a protective factor for STEMI (Table 2).
Table 2
XXX | Univariate logistic regression analysis | Multivariate logistic regression analysis | |||||||
---|---|---|---|---|---|---|---|---|---|
β | P value | Odds ratio | 95% CI | β | P value | Odds ratio | 95% CI | ||
Age | −0.001 | 0.882 | 0.999 | 0.981, 1.016 | – | – | – | – | |
Gender | 0.948 | <0.001* | 2.580 | 1.568, 4.246 | −0.338 | 0.435 | 0.713 | 0.305, 1.668 | |
BMI | −0.068 | 0.068 | 0.934 | 0.868, 1.005 | −0.165 | 0.006* | 0.848 | 0.754, 0.955 | |
Smoking | 1.465 | <0.001* | 4.329 | 2.677, 6.999 | 1.264 | 0.001* | 3.541 | 1.662, 7.546 | |
Hypertension | −0.051 | 0.822 | 0.950 | 0.608, 1.484 | −0.328 | 0.345 | 0.720 | 0.365, 1.422 | |
Diabetes | 1.017 | <0.001* | 2.765 | 1.563, 4.893 | 1.201 | 0.002* | 3.324 | 1.554, 7.112 | |
Creatinine | 0.017 | 0.003* | 1.017 | 1.006, 1.029 | −0.004 | 0.586 | 0.996 | 0.980, 1.011 | |
UA | 0.000 | 0.823 | 1.000 | 0.998, 1.002 | – | – | – | – | |
TG | 0.078 | 0.355 | 1.082 | 0.916, 1.277 | – | – | – | – | |
TC | 0.196 | 0.065 | 1.217 | 0.988, 1.498 | – | – | – | – | |
HDL-C | −1.794 | <0.001* | 0.166 | 0.071, 0.392 | −2.542 | <0.001* | 0.079 | 0.021, 0.293 | |
LDL-C | 0.497 | 0.001* | 1.644 | 1.242, 2.175 | 0.995 | <0.001* | 2.704 | 1.785, 4.094 | |
VLDL-C | 0.243 | 0.538 | 1.275 | 0.589, 2.761 | – | – | – | – | |
ApoB | 1.920 | <0.001* | 6.823 | 2.339, 19.897 | – | – | – | – | |
ApoA1 | −2.883 | <0.001* | 0.056 | 0.017, 0.183 | – | – | – | – | |
Hcy | 0.157 | <0.001* | 1.170 | 1.106, 1.238 | 0.149 | <0.001* | 1.161 | 1.084, 1.244 | |
CIMT | 6.076 | <0.001* | 435.146 | 80.672, 2,347.178 | 5.333 | <0.001* | 206.968 | 22.375, 1,914.468 | |
Plaque | 1.373 | <0.001* | 3.949 | 2.466, 6.322 | 0.916 | 0.013* | 2.499 | 1.214, 5.142 |
*, P<0.05. STEMI, ST-segment elevation myocardial infarction; CI, confidence interval; BMI, body mass index; UA, uric acid; TG, triglycerides; TC, total cholesterol; LDL-C, low-density lipoprotein cholesterol; HDL-C, high-density lipoprotein cholesterol; VLDL-C, very-low-density lipoprotein cholesterol; ApoB, apolipoprotein B; ApoA1, apolipoprotein A1; Hcy, homocysteine; CIMT, carotid intima-media thickness.
ROC curve of different indexes and multi-index combined diagnosis
ROC curve of different indexes
Compared with traditional risk factors, increased CIMT and serum Hcy levels were more valuable in the diagnosis and prediction of STEMI occurrence. The AUC of Hcy was 0.729, accompanied by the following values: (I) optimal cut-off value =13.525 µmol/L; (II) sensitivity =0.551; (III) specificity =0.853. The AUC of CIMT is 0.763, accompanied by the following values: (I) optimal cut-off value =0.875 mm; (II) sensitivity =0.692; (III) specificity =0.736 (Figure 2 and Table 3).
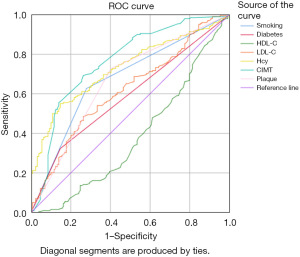
Table 3
Factor | AUC | 95% CI | Cut-off value | Sensitivity | Specificity | P |
---|---|---|---|---|---|---|
Smoking | 0.674 | 0.614–0.733 | 0.5 | 0.626 | 0.721 | <0.001 |
Diabetes | 0.588 | 0.526–0.650 | 0.5 | 0.323 | 0.851 | 0.007 |
HDL-C | 0.379 | 0.316–0.442 | 0.685 mmol/L | 0.970 | 0.039 | <0.001 |
LDL-C | 0.616 | 0.554–0.677 | 3.025 mmol/L | 0.485 | 0.736 | <0.001 |
Hcy | 0.729 | 0.675–0.783 | 13.525 μmol/L | 0.551 | 0.853 | <0.001 |
CIMT | 0.763 | 0.709–0.818 | 0.875 mm | 0.692 | 0.736 | <0.001 |
Plaque | 0.662 | 0.601–0.723 | 0.5 | 0.727 | 0.597 | <0.001 |
STEMI, ST-segment elevation myocardial infarction; AUC, area under the curve; CI, confidence interval; LDL-C, low-density lipoprotein cholesterol; HDL-C, high-density lipoprotein cholesterol; Hcy, homocysteine; CIMT, carotid intima-media thickness.
ROC curve of different combined diagnosis models
The diagnosis model 1 was defined to include traditional risk factors such as smoking, diabetes, HDL-C, and LDL-C. The area under the ROC curve for STEMI was 0.767 (95% CI: 0.715–0.819, P<0.001). Based on model 1, Hcy was introduced to form the diagnostic model 2. The area under ROC curve was 0.835 (95% CI: 0.792–0.879, P<0.001). Based on Model 2, CIMT was further introduced to form the diagnosis model 3. The area under the ROC curve was 0.886 (95% CI: 0.849–0.923, P<0.001). Based on the Model 3, carotid plaque was introduced to form the diagnostic model 4. The area under ROC curve was 0.892 (95% CI: 0.856–0.928, P<0.001) (Figure 3).
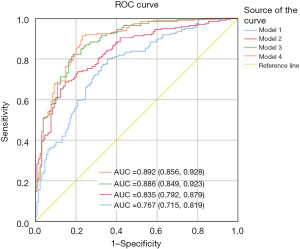
Construction and validation of a nomogram model for predicting STEMI occurrence
Construction of nomogram model
A nomogram model was constructed based on independent predictors of STEMI: smoking, diabetes, LDL-C, HDL-C, Hcy, CIMT, and carotid artery plaque, as shown in Figure 4.
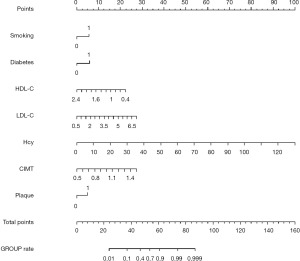
Validation of nomogram model
The C-index of the model was 0.892 (95% CI: 0.856–0.928), suggesting that the model had good differentiation and prediction ability. H-L fitting test results showed that χ2=1.5049, df=2, P=0.4712, indicating that the model was well fitted. Using the Bootstrap method, the sample was resampled 100 times for internal verification, and a Calibration curve was drawn. The results show that the C-index of internal verification is 0.880, and the calibration curve of the line graph model is close to the ideal curve, indicating that the model has high consistency, as shown in Figure 5.
Analysis of clinical value of nomogram model
A clinical DCA was plotted with the probability of risk threshold as the horizontal coordinate and the net benefit rate as the vertical coordinate to evaluate the net benefit of the model for predicting STEMI occurrence. As shown in Figure 6, the “nomogram line” of the model was far from the “All line” and “None line”, indicating that the model could bring net benefit to the clinic.
Discussion
In recent years, the incidence of CHD has been increasing yearly, which seriously endangers human health. Smoking, hyperlipidemia, hypertension, and diabetes are well-known traditional risk factors. However, 15–20% of patients with CHD still have no established traditional risk factors (5). Attention has been paid to the correlation between Hcy and the prevalence of CHD. More and more studies and meta-analyses have suggested that Hcy is an independent risk factor for atherosclerosis and is associated with the incidence and prognosis of CHD (4-6). However, few studies have been conducted specifically in the specific population of acute myocardial infarction (AMI). As the most serious CHD, STEMI has a very high disability and mortality rate, and more studies are urgently needed to guide the prevention and treatment of the disease. A study involving 120 patients with AMI found that serum Hcy was significantly higher in the AMI group than in the healthy group (4). Another study found that thrombosis was predominant in young patients with AMI, while atherosclerosis was predominant in elderly patients with AMI, and young patients with AMI had a higher serum Hcy level and higher prevalence of hyperhomocysteinemia (HHcy) (14). Our study showed that serum Hcy level in STEMI group was significantly higher than that in the control group, with statistical significance, consistent with the above results. In addition, the results suggested that Hcy was an independent risk factor for STEMI. At present, clinicians do not pay much attention to Hcy. This study proposed that adding Hcy on the basis of traditional risk factors can more fully assess individual risk factors for cardiovascular and cerebrovascular diseases, improve the predictive diagnosis rate of STEMI, and conduct an early assessment and diagnosis of high-risk groups as well as realize individualized guided treatment, which has significant clinical and social benefits.
Hcy is an intermediate product of cysteine and methionine metabolism. Dietary methionine is catalyzed by S-adenosylmethionine synthetase, methyltransferase, and S-adenosylhomocysteine hydrolase to form Hcy, which is the only pathway for Hcy synthesis in human body (15,16). Hcy is mainly metabolized in liver and kidney through the following ways (17): the folate-dependent/independent remethylation to form Met and the transsulfuration pathway to form cysteine. Studies have found that high methionine diet, deficiency or dysfunction of enzymes related to Hcy metabolism, deficiency of vitamins (B6, B12) and folate are closely related to the occurrence and development of HHcy, in addition, renal insufficiency is related to reduced Hcy excretion (15-18). The Chinese Guidelines for the Prevention and Treatment of Hypertension revised in 2018 (19) adopted fasting plasma total Hcy ≥15 µmol/L as the diagnostic criteria for HHcy. According to the degree of Hcy elevation, it was divided into mild (Hcy 15–30 µmol/L), moderate (Hcy 31–100 µmol/L) and severe (Hcy >100 µmol/L). The possible mechanism of HHcy leading to CVD: Hcy can directly or indirectly lead to vascular endothelial cell injury and apoptosis, promote the proliferation of vascular smooth muscle cells, mediate oxidative stress and immune inflammation, affect the aggregation and peroxidation of low-density lipoproteins, enhance platelet function, and promote thrombosis (5,18).
From the development of CVD risk factors to the occurrence of target organ damage, vascular disease exists in the whole pathophysiological process. More evidence supports the need to focus on systemic vascular disease rather than simply attributing the disease to single and isolated lesions in the epicardial coronary arteries. A large number of studies have confirmed that the scope and severity of vascular lesions can change the classification of the risk factor scoring system (3,11). CIMT has been used in cardiovascular research for 30 years and is considered as a preclinical surrogate marker reflecting atherosclerotic burden and endothelial dysfunction. A large number of basic experimental, clinical, and epidemiological studies have shown that increased CIMT is associated with coronary artery disease, stroke, and peripheral vascular disease (7-9). There is also a persistent and progressive association with the risk and prognosis of cardiovascular events (20). The correlation between the detection and severity of carotid plaque and CVD is more confirmed. The 2019 ESC/European Atherosclerosis Society (EAS) Guidelines for the Management of Dyslipidemia (21) and the 2021 ESC Guidelines for the Prevention of Cardiovascular Disease (22) both recommend the detection of carotid plaque load as a risk correction tool for low- and intermediate-risk patients. So we suggest that CIMT combined with carotid plaque may serve as a window to assess the risk and progression of CVD. Previous studies have found that most studies involving CIMT have explored the correlation between CIMT and the risk of CVD in asymptomatic individuals, or explored the correlation between CIMT and CVD recurrence and adverse cardiovascular events in people with CVD. However, there is limited data on CIMT’s ability to identify patients at risk for STEMI. In view of this, our experimental design was to compare a specific population of STEMI with a population who received coronary angiography to exclude significant coronary artery disease to explore the predictive value of CIMT and carotid plaque combined with traditional cardiovascular risk factors and a novel risk factor Hcy in the occurrence of STEMI. The results of this study showed that the CIMT value and carotid plaque detection rate in STEMI group were higher than those in control group, with statistical significance. Multifactor logistics regression analysis was conducted, and the results showed that CIMT, carotid plaque were independent risk factors for STEMI. At the same time, the prediction accuracy of CIMT for STEMI was even better than that of traditional risk factors such as smoking, blood lipid and diabetes. Although the current gold standard for the diagnosis of CHD still relies on coronary angiography or coronary CTA, it is invasive, expensive, difficult to popularize, and has certain complications. Carotid ultrasound Doppler examination, as a cheap, popular, simple, non-invasive method to detect arteriosclerosis, can be better accepted by patients because of the superficial anatomy of the carotid artery and the relative fixation of blood vessels. So we propose that the integration of vascular lesions and risk factors will be significantly superior to the traditional approach in risk assessment and guiding clinical diagnosis and treatment decisions.
To further study the diagnostic and predictive value of Hcy, CIMT, and carotid plaque in STEMI, and to explore the best prediction model, we conducted a comparative study of different combination models. The results of this analysis suggest that the inclusion of Hcy, CIMT, and carotid plaque on the basis of traditional risk factors can significantly improve the diagnostic and predictive value of STEMI, and has certain significance for guiding clinical work.
Nomogram is a new individualized statistical model for predicting clinical outcomes. It is used to predict the onset, progression, and outcome of diseases with multiple variables in clinical studies. The basic principle is to build a multi-factor regression model (such as logistic regression, Cox regression, etc.), assign a value to each value level of each influence factor according to the contribution degree of each influence factor in the model to the outcome variable (the size of the regression coefficient), and then add each score to get the total score. Finally, the predicted value of the outcome event is calculated by the function transformation relationship between the total score and the probability of the outcome event. As shown in Figure 4, the nomogram constructed in this study included seven independent predictors: smoking, diabetes, LDL-C, HDL-C, Hcy, CIMT, and carotid plaque. The C-index of the prediction model was 0.892, indicating that the model could accurately predict the occurrence of STEMI and had good prediction ability. The C index of internal verification is 0.880, which still indicates that the model has good discriminability. The calibration curve of the drawn model is close to the ideal model (see Figure 5), indicating that the predicted risk is highly consistent with the actual occurrence risk and the prediction efficiency is good. Clinical DCA showed that the clinical net benefit of using the nomogram model constructed in this study to assess the risk of STEMI was higher (see Figure 6).
This study still has shortcomings: (I) this study is a single-center retrospective study with a relatively small sample size, which inevitably leads to some bias; (II) the control group selected patients who were admitted to hospital with suspected symptoms of CHD and were explicitly excluded by CHD by coronary angiography. In contrast, patients who were clinically recommended to undergo coronary angiography were more or less combined with risk factors, so these patients were not completely blank controls. Therefore, there may be some bias in the trial results (for example, there is no statistically significant difference between the two groups in traditional risk factors such as age, BMI, and hypertension); (III) the nomogram model is only verified internally, and lacks external verification.
Conclusions
The results of this study suggest that HHcy and increased CIMT/carotid plaque could be independent risk factors or predictors of STEMI in patients seen for chest pain. The inclusion of Hcy, CIMT, and carotid plaque in addition to traditional risk factors can more fully and accurately predict the risk of STEMI. The nomogram based on the results of this study can visually present the prediction results, is more readable and intuitive and more convenient to provide individualized risk assessment for STEMI risk groups, and then facilitate early intervention.
Acknowledgments
Funding: The study was supported by
Footnote
Reporting Checklist: The authors have completed the STROBE reporting checklist. Available at https://cdt.amegroups.com/article/view/10.21037/cdt-23-312/rc
Data Sharing Statement: Available at https://cdt.amegroups.com/article/view/10.21037/cdt-23-312/dss
Peer Review File: Available at https://cdt.amegroups.com/article/view/10.21037/cdt-23-312/prf
Conflicts of Interest: All authors have completed the ICMJE uniform disclosure form (available at https://cdt.amegroups.com/article/view/10.21037/cdt-23-312/coif). The authors have no conflicts of interest to declare.
Ethical Statement: The authors are accountable for all aspects of the work in ensuring that questions related to the accuracy or integrity of any part of the work are appropriately investigated and resolved. The study was conducted in accordance with the Declaration of Helsinki (as revised in 2013). The study was reviewed and approved by Hefei Hospital Affiliated to Anhui Medical University Ethics Committee (No. 2020-ke-058). Written informed consent for participation was not required for this retrospective study.
Open Access Statement: This is an Open Access article distributed in accordance with the Creative Commons Attribution-NonCommercial-NoDerivs 4.0 International License (CC BY-NC-ND 4.0), which permits the non-commercial replication and distribution of the article with the strict proviso that no changes or edits are made and the original work is properly cited (including links to both the formal publication through the relevant DOI and the license). See: https://creativecommons.org/licenses/by-nc-nd/4.0/.
References
- Zhao D, Liu J, Xie W, et al. Cardiovascular risk assessment: a global perspective. Nat Rev Cardiol 2015;12:301-11. [Crossref] [PubMed]
- Kabłak-Ziembicka A, Przewłocki T. Clinical Significance of Carotid Intima-Media Complex and Carotid Plaque Assessment by Ultrasound for the Prediction of Adverse Cardiovascular Events in Primary and Secondary Care Patients. J Clin Med 2021;10:4628. [Crossref] [PubMed]
- Biswas M, Saba L, Omerzu T, et al. A Review on Joint Carotid Intima-Media Thickness and Plaque Area Measurement in Ultrasound for Cardiovascular/Stroke Risk Monitoring: Artificial Intelligence Framework. J Digit Imaging 2021;34:581-604. [Crossref] [PubMed]
- Zhang N, Shi F, Liang H, et al. The feasibility of using Hcy, CRP, and Cys-C to analyze AMI patients’ disease conditions and prognoses. Am J Transl Res 2021;13:2724-30. [PubMed]
- Wang B, Mo X, Wu Z, et al. Systematic review and meta-analysis of the correlation between plasma homocysteine levels and coronary heart disease. J Thorac Dis 2022;14:646-53. [Crossref] [PubMed]
- Muzaffar R, Khan MA, Mushtaq MH, et al. Hyperhomocysteinemia as an Independent Risk Factor for Coronary Heart Disease. Comparison with Conventional Risk Factors. Braz J Biol 2021;83:e249104. [Crossref] [PubMed]
- van den Oord SC, Sijbrands EJ, ten Kate GL, et al. Carotid intima-media thickness for cardiovascular risk assessment: systematic review and meta-analysis. Atherosclerosis 2013;228:1-11. [Crossref] [PubMed]
- Fernández-Alvarez V, Linares Sánchez M, López Alvarez F, et al. Evaluation of Intima-Media Thickness and Arterial Stiffness as Early Ultrasound Biomarkers of Carotid Artery Atherosclerosis. Cardiol Ther 2022;11:231-47. [Crossref] [PubMed]
- Georgiopoulos G, Mavraganis G, Delialis D, et al. Carotid ultrasonography improves residual risk stratification in guidelines-defined high cardiovascular risk patients. Eur J Prev Cardiol 2022;29:1773-84. [Crossref] [PubMed]
- Poredos P, Jezovnik MK. Preclinical carotid atherosclerosis as an indicator of polyvascular disease: a narrative review. Ann Transl Med 2021;9:1204. [Crossref] [PubMed]
- Kabra A, Neri L, Weiner H, et al. Carotid intima-media thickness assessment in refinement of the Framingham Risk Score: can it predict ST-elevation myocardial infarction? A pilot study. Echocardiography 2013;30:1209-13. [Crossref] [PubMed]
- Thygesen K, Alpert JS, Jaffe AS, et al. Fourth universal definition of myocardial infarction (2018). Eur Heart J 2019;40:237-69. [Crossref] [PubMed]
- Johri AM, Nambi V, Naqvi TZ, et al. Recommendations for the Assessment of Carotid Arterial Plaque by Ultrasound for the Characterization of Atherosclerosis and Evaluation of Cardiovascular Risk: From the American Society of Echocardiography. J Am Soc Echocardiogr 2020;33:917-33. [Crossref] [PubMed]
- Kaur R, Das R, Ahluwalia J, et al. Genetic polymorphisms, Biochemical Factors, and Conventional Risk Factors in Young and Elderly North Indian Patients With Acute Myocardial Infarction. Clin Appl Thromb Hemost 2016;22:178-83. [Crossref] [PubMed]
- Jakubowski H. Homocysteine Modification in Protein Structure/Function and Human Disease. Physiol Rev 2019;99:555-604. [Crossref] [PubMed]
- Škovierová H, Vidomanová E, Mahmood S, et al. The Molecular and Cellular Effect of Homocysteine Metabolism Imbalance on Human Health. Int J Mol Sci 2016;17:1733. [Crossref] [PubMed]
- Hasan T, Arora R, Bansal AK, et al. Disturbed homocysteine metabolism is associated with cancer. Exp Mol Med 2019;51:1-13. [Crossref] [PubMed]
- Koklesova L, Mazurakova A, Samec M, et al. Homocysteine metabolism as the target for predictive medical approach, disease prevention, prognosis, and treatments tailored to the person. EPMA J 2021;12:477-505. [Crossref] [PubMed]
- Revision Committee of the Chinese Guidelines for the Prevention and Control of Hypertension. Liu LS. Chinese Guidelines for the Prevention and Treatment of Hypertension (revised 2018). Prevention and Treatment of Cardiovascular Disease 2019;19:1-44.
- Willeit P, Tschiderer L, Allara E, et al. Carotid Intima-Media Thickness Progression as Surrogate Marker for Cardiovascular Risk: Meta-Analysis of 119 Clinical Trials Involving 100 667 Patients. Circulation 2020;142:621-42. [Crossref] [PubMed]
- Mach F, Baigent C, Catapano AL, et al. 2019 ESC/EAS Guidelines for the management of dyslipidaemias: lipid modification to reduce cardiovascular risk. Eur Heart J 2020;41:111-88. [Crossref] [PubMed]
- Visseren FLJ, Mach F, Smulders YM, et al. 2021 ESC Guidelines on cardiovascular disease prevention in clinical practice. Eur Heart J 2021;42:3227-337. [Crossref] [PubMed]