Discrimination models with radiomics features derived from cardiovascular magnetic resonance images for distinguishing hypertensive heart disease from hypertrophic cardiomyopathy
Highlight box
Key findings
• Radiomics applied to cardiovascular magnetic resonance (CMR) provide great help in differentiating hypertrophic cardiomyopathy (HCM) from hypertensive heart disease (HHD).
What is known and what is new?
• Discriminating HHD and HCM is difficult, but accurate diagnose is crucial for clinical management.
• Building a model by integrating radiomics with conventional CMR indicators will be beneficial in distinguishing between HCM and HHD.
What is the implication, and what should change now?
• The application of the model constructed in this study to clinical settings could potentially enhance the diagnostic accuracy of HCM. The models constructed in this study need to be validated by multi-center data.
Introduction
Hypertrophic cardiomyopathy (HCM) is characterized by left ventricular hypertrophy (LVH) in the absence of another cause of hypertrophy (1,2). However, LVH can also be secondary to other disorders, such as hypertension history, as defined as hypertensive heart disease (HHD). Although a history of hypertension, symmetrical LVH, indexed left ventricular (LV) mass, without right ventricular (RV) insertion late gadolinium enhancement (LGE) and systolic anterior motion (SAM) of mitral valve were found in a previous study to be effective in discriminating HHD from HCM (3). However, not all patients exhibit typical characteristics. RV insertion LGE and asymmetric LVH are not uncommon in HHD patients (4). Hypertension also co-occurs in approximately 40–60% of adults with HCM (5). Therefore, relying solely on traditional characteristics is not sufficient to completely differentiate between HCM and HHD. But accurately distinguishing between these two diseases is crucial for precise clinical management, and, as a result, there is a need to seek a more effective method of differentiation.
Radiomics can provide a variety of hidden information within image data and has demonstrated significant value in the differential diagnosis of various diseases (6). Therefore, we hypothesize that radiomics may also hold important value in differentiating between HCM and HHD. Furthermore, combining multiparametric cardiac magnetic resonance (CMR) findings and radiomics features may provide additional value.
In this study, we aimed to investigate discriminators and build discrimination models between HHD and HCM using multiparametric CMR findings and radiomics score (radscore) derived from cine and LGE images. We present this article in accordance with the STARD reporting checklist (available at https://cdt.amegroups.com/article/view/10.21037/cdt-23-350/rc).
Methods
Study population
The study was conducted in accordance with the Declaration of Helsinki (as revised in 2013). The study was approved by the Institutional Review Board of the Beijing Anzhen Hospital (No. 2023178X) with a waiver for informed consent due to the retrospective analysis of the study. Consecutive HCM subjects from January 2017 to September 2021 and consecutive HHD subjects from September 2015 to July 2022 who met the inclusion criteria were reviewed.
Inclusion criteria for HCM group: (I) maximal LV end diastolic wall thickness (LVEDWT) ≥15 mm in the absence of another cause of hypertrophy; (II) maximal LVEDWT of 13–14 mm with HCM family history or accompanied by a positive genetic test.
Inclusion criteria for HHD group: (I) definite diagnosis of stage II hypertension for at least 5 years (7); (II) maximal LVEDWT ≥12 mm; (III) without LV cavity dilatation, severe chronic kidney disease and cardiac diseases that could result in a similar magnitude of hypertrophy (8,9).
Exclusion criteria: (I) HCM patient with any hypertension history of any degree; (II) patients with a history of myocardial infarction; (III) patients coexisting with other heart conditions, such as congenital heart disease; (IV) patients with a history of cardiac surgery; (V) patients who were less than 14 years old; (VI) images with significant artifacts or a lack of LGE sequence.
Ultimately, 421 HCM patients [median and interquartile range (IQR), 50.0 (38.0–59.0) years; male, 70.5%] and 200 HHD patients [median and IQR, 44.5 (35.0–57.0) years; male, 88.5%] were retrospectively included in our study. Patients were randomly stratified into a training group (n=252 for HCM, 120 for HHD) and a validation group (n=169 for HCM, 80 for HHD) at a proportion of 6:4. The flow chart of our study is shown in Figure 1.
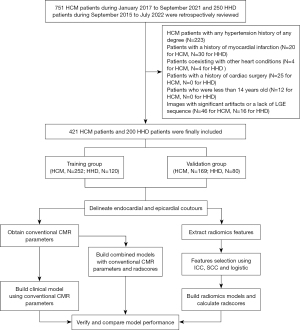
Magnetic resonance image acquisition
Following routine scan protocols, images were acquired with a 32-channel surface phased array cardiac coil using a variety of scanners from 3 vendors (Ingenia 3.0T, Philips Healthcare, Best, Netherlands; MAGNETOM Siemens Verio 3.0T, Siemens Health Care, Erlangen, Germany; Discovery MR750 3.0T, GE Medical Systems, Milwaukee, WI, USA). Cardiac cine images from the base to the apex of the LV were collected using balanced steady-state free precession (bFISP) sequences. Retrospective cardiac gating was used, and each cardiac cycle included 25 phases. A breath-hold two dimensional (2D) phase sensitive inversion-recovery (PSIR) segmented gradient echo sequence was used to collect LGE images at 10 minutes after contrast agent administration, as for cine images. Contrast agent (gadopentetate dimeglumine, Bayer Healthcare, Leverkusen, Germany) was intravenously administered at a dose of 0.2 mmol/kg body weight. The inversion time was set to obtain maximal nulling of remote normal LV myocardium. The parameters for CMR images were listed in Table S1. All slice thicknesses were 8 mm for the short axis and 5 mm for the long axis, with no interval between slice locations.
Image analysis
We used deep learning-based commercial software (cvi42, version 5.11.2, Circle Cardiovascular Imaging Inc., Calgary, Canada) to draw LV epicardial and endocardial borders from the base to the apex automatically, and then the borders were manually adjusted by an experienced operator. The left ventricular ejection fraction (LVEF), left ventricular end diastolic volume (LVEDV), left ventricular end systolic volume (LVESV) and left ventricular mass (LVM) were automatically calculated by the software. LVM index (LVMi), LVEDV index (LVEDVi), and LVESV index (LVESVi) were calculated by dividing the respective indices by body surface area. Maximal LVEDWT was manually measured by an experienced observer.
The epicardial and endocardial borders of LGE images were manually delineated by two experienced operators. We also defined a visually normal-appearing area of myocardium without hyperenhancement as a normal myocardial region of interest. LGE was defined as myocardium 6 standard deviations (SD) above the mean signal intensity. Quantification of LGE was automatically measured by cvi42 software.
SAM was defined as mitral valve leaflets moving toward the septum of the LV during systole. The presence of SAM as well as the location of LGE were visually analyzed by two experienced observers blinded to the clinical data. LVH asymmetry was defined as the maximal LVEDWT >1.5-fold the wall thickness of the opposing segment.
Radiomics feature extraction and selection
After the borders of LV cine and LGE images were drawn, images were resampled to an in-plane voxel size of 1×1×1 mm3 and voxel intensity values were discretized by using a fixed bin width of 25 HU prior to feature extraction. Pyradiomics of Python (version 3.7) was used to extract radiomics features from the LGE images and the end-diastolic phase of cine images. The radiomics features include semantic, first-, second- and higher-order features. Semantic features include location, shape, size features, etc. First-order features describe the distribution of individual voxels and are median, mean, minimum or maximum of the intensities on the image. Second-order features describe interrelationships between voxels, which include gray-level size-zone matrix (GLSZM), gray-level co-occurrence matrix (GLCM), gray-level dependence matrix (GLDM), gray-level run-length matrix (GLRLM) and neighborhood gray-tone difference matrix (NGTDM). Higher-order features impose filter grids on the image, which include log, exponential, logarithm, gradient, square, square root, wavelet and local binary pattern (LBP).
HHD samples comprised less than half of the HCM samples, which may cause adverse impacts on the performance of an optimal subset with the logistic classifier. Thus, to balance the size of the majority group, the synthetic minority oversampling technique (SMOTE) was performed with joint weighting of features in the optimal subset to generate samples from the minority group (10,11). By using these methods, we can enhance the representation of the minority group while retaining the original structure of the samples (10,11).
Because most of the extracted high-dimensional features were redundant, dimensionality reduction was essential. We first used the intraclass correlation coefficient (ICC) to assess the reliability and reproducibility of the features and selected features with an ICC higher than 0.8 in both the interobserver and intraobserver analyses (12,13). Then, a Spearman correlation matrix for all features was calculated, and one random feature was excluded in any feature pair with a Spearman correlation coefficient (SCC) greater than 0.7 (14). Finally, multivariate logistic regression was applied to choose the optimized subset of features (13). The radscore was calculated by summing these features weighted by their coefficients (which were determined using logistic method) (Figure 2). All features were standardized by using the z score standardization.
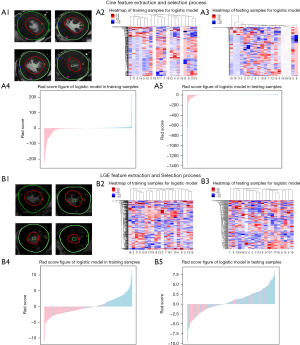
Discrimination model building
Discrimination models were built using multiparametric CMR findings with or without the radscore. Characteristics that were statistically significant with P<0.05 in univariate logistic analysis were included in multivariate logistic analysis. Finally, discrimination models were built based on multivariate logistic regression. To evaluate the differential diagnostic performance of the cine radscore, LGE radscore, combined radscore and combined model in different scanners, we compared the area under the receiver operator characteristic curve (AUC) of these methods for various MR scanners by using Delong test.
Reproducibility
The contours of 30 randomly selected subjects (18 HCM patients and 12 HHD patients) were redrawn after 3 months by observer 1. Observer 2, blinded to the first observer’s result, drew the same set of subjects (13). Radiomics features were extracted by the same method and were used to analyze intraobserver and interobserver reproducibility. In addition, multiparameter CMR characteristics (e.g., SAM, LVH asymmetry) were reanalyzed by observer 1 and observer 2 to assess reproducibility.
Statistical analysis
Because the quantitative data in this study does not conform to a normal distribution according to the Shapiro-Wilk test. They were expressed as the median and IQR, and the Mann-Whitney U tests were used to compare differences between two groups. Categorical variables are presented as frequencies or percentages, and the chi square test or Fisher’s exact test was used to compare differences between two groups. We evaluated the discriminating performance of the radscore and discrimination models by AUC in both the training and validation groups. The Youden index was used to assess optimal cut-off value of different models. The calibration of different models was assessed using calibration curves, which were plotted by bootstrapping with 1,000 resamples and accompanied by the Hosmer-Lemeshow goodness-of-fit test. The likelihood ratio test was used to compare the performance of nested models and the Delong test was used to compare the performance of non-nested models. The ICC and Kappa coefficient were used to evaluate interobserver and intraobserver reproducibility. Two sides P value <0.05 was considered statistically significant. The statistical analysis was performed with IPM statistics (IPMs, version 2.4.0, GE Healthcare, Milwaukee, WI, USA), SPSS software (IBM SPSS Statistics for Windows, Version 25.0; IBM Corp., Armonk, NY, USA), MedCalc Software (MedCalc for Windows, version 19.3.1, Ostend, Belgium), Python (version 3.7, https://www.python.org) and R programming language (version 3.4.2, http://www.r-project.org).
Results
Patient characteristics and multiparametric CMR findings
The patient characteristics of the HCM and HHD patients are presented in Table 1. Patient characteristics showed no difference between the training group and the validation group (Table S2). The multiparameter CMR findings and MRI scanners of HCM and HHD patients are provided in Table 2.
Table 1
Characteristics | Training group | Validation group | |||||
---|---|---|---|---|---|---|---|
HCM (n=252) | HHD (n=120) | P value | HCM (n=169) | HHD (n=80) | P value | ||
Gender | <0.001 | 0.004 | |||||
Male | 177 (70%) | 107 (89%) | 120 (71%) | 70 (88%) | |||
Female | 75 (30%) | 13 (11%) | 49 (29%) | 10 (13%) | |||
Age (years) | 50 [38–59] | 45 [34–57] | 0.22 | 50 [38–60] | 42 [36–57] | 0.07 | |
Body surface area (m2) | 2.0 [1.8–2.1] | 2.1 [1.9–2.1] | <0.001 | 2.0 [1.8–2.0] | 2.1 [1.9–2.1] | <0.001 | |
Systolic blood pressure (mmHg) | 119 [112–121] | 140 [130–145] | <0.001 | 119 [112–121] | 142 [126–147] | <0.001 | |
Diastolic blood pressure (mmHg) | 75 [71–78] | 90 [80–96] | <0.001 | 75 [71–79] | 90 [79–95] | <0.001 |
Quantitative data were expressed as median and interquartile range [IQR], categorical variables were present as frequencies (percentages). HCM, hypertrophic cardiomyopathy; HHD, hypertensive heart disease; IQR, interquartile range.
Table 2
Parameters or scanners | Training group | Validation group | |||||
---|---|---|---|---|---|---|---|
HCM (n=252) | HHD (n=120) | P value | HCM (n=169) | HHD (n=80) | P value | ||
LV morphology and function | |||||||
Indexed LV end-diastolic volume (mL/m2) | 63 [54–74] | 93 [60–118] | <0.001 | 61 [53–73] | 86 [66–122] | <0.001 | |
Indexed LV end-systolic volume (mL/m2) | 21 [16–27] | 53 [24–89] | <0.001 | 21 [17–28] | 59 [27–90] | <0.001 | |
LV ejection fraction (%) | 66 [59–72] | 38 [20–59] | <0.001 | 64 [57–70] | 32 [20–60] | <0.001 | |
Maximal LVEDWT (mm) | 20 [17–23] | 16 [14–17] | <0.001 | 20 [17–24] | 16 [14–17] | <0.001 | |
Indexed LV mass at diastole (g/m2) | 61 [51–80] | 74 [50–85] | 0.08 | 64 [49–82] | 71 [55–89] | 0.25 | |
Quantification of LGE (%) | 4 [1–11] | 3 [0–6] | <0.001 | 5 [1–13] | 1 [0–4] | <0.001 | |
LVH asymmetry | 216 (86%) | 56 (47%) | <0.001 | 135 (80%) | 32 (40%) | <0.001 | |
SAM | 64 (25%) | 2 (2%) | <0.001 | 28 (17%) | 1 (1%) | <0.001 | |
LGE | 210 (83%) | 86 (72%) | 0.009 | 140 (83%) | 44 (55%) | <0.001 | |
Mid-wall LGE | 199 (79%) | 77 (64%) | 0.002 | 127 (75%) | 39 (49%) | <0.001 | |
RV insertion point LGE | 171 (68%) | 59 (49%) | 0.001 | 114 (67%) | 27 (34%) | <0.001 | |
Scanner 3.0T | <0.001 | 0.007 | |||||
Siemens | 106 (42%) | 65 (54%) | 70 (41%) | 44 (55%) | |||
Philips | 91 (36%) | 17 (14%) | 55 (33%) | 11 (14%) | |||
GE | 55 (22%) | 38 (32%) | 44 (26%) | 25 (31%) |
Quantitative data were expressed as median and interquartile range [IQR], categorical variables were present as frequencies (percentages). Siemens refers to MAGNETOM Siemens Verio 3.0T, Siemens Health Care, Erlangen, Germany; Philips refers to Ingenia 3.0T, Philips Healthcare, Best, Netherlands; GE refers to Discovery MR750 3.0T, GE Medical Systems, Milwaukee, WI, USA. MRI, magnetic resonance imaging; HCM, hypertrophic cardiomyopathy; HHD, hypertension heart disease; LV, left ventricle; LVEDWT, left ventricular end diastolic wall thickness; LGE, late gadolinium enhancement; LVH, left ventricular hypertrophy; SAM, systolic anterior motion; RV, right ventricle; IQR, interquartile range.
Feature extraction and selection
A total of 1,409 radiomic features for each patient were finally extracted from both the LGE images and the cine images at the end diastolic phase. Radiomics features include 14 semantic features, 18 first-order features, 75 second-order features and 1,302 high-order features. Among the cine images, 1,091 features with ICCs higher than 0.8 by intraobserver and interobserver analysis were selected. Subsequently, we used Spearman correlation analysis to further select 81 features. Finally, multivariate logistic regression was used to determine the 20 optimal features. Among the LGE images, 1,155 features with ICCs higher than 0.8 by intraobserver and interobserver analysis were selected. Then, 108 features remained using Spearman correlation analysis. Finally, 20 optimal features remained using multivariate logistic regression. The cine radscore and LGE radscore were calculated by summing these optimal features weighted by their efficiency (Appendix 1: Eq. [S1,S2]). We also calculated the combined radscore using multivariate logistic regression with the cine radscore and LGE radscore (Appendix 1: Eq. [S3]), which achieved an AUC of 0.964 (0.948–0.980) in the training group and 0.924 (0.890–0.958) in the validation group (Figure 3).
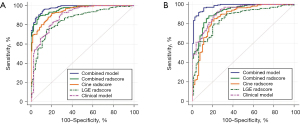
Discrimination model establishment and evaluation
The maximal LVEDWT, LVEDVi, LVESVi, LVEF, LVH asymmetry, SAM, quantification of LGE, mid-wall LGE, RV insertion point LGE, cine radscore and LGE radscore were identified as significant characteristics at P<0.05 in univariate logistic analysis. In multivariate logistic regression without the radscore, the maximal LVEDWT, LVEF, LVH asymmetry and quantification of LGE were identified as significant characteristics and used to build clinical discrimination model (Table 3 & Eq. [1]), which achieved an AUC of 0.892 (0.855–0.929) and 0.919 (0.884–0.954) in the training and validation group, respectively (Figure 3). In multivariate logistic regression including the radscore, the maximal LVEDWT, LVEF, presence of LGE, cine radscore and LGE radscore were identified as significant characteristics and used to build a combined discrimination model (Table 3 & Eq. [2]), which achieved an AUC of 0.979 (0.968–0.990) and 0.981 (0.967–0.995) in the training and validation group, respectively (Figure 3). The differential diagnostic performance of the combined model was significantly higher than that of the clinical model in both the training group and validation group (P<0.001). The calibration curves of the different models are provided in Figure 4. The independent value of each discriminator in the discrimination models for distinguishing HHD from HCM was also analysed in Table S3.
Table 3
Variable | Univariate analysis | Multivariate analysis without radscore | Multivariate analysis with radscore | |||||
---|---|---|---|---|---|---|---|---|
OR* (95% CI) | P value | OR* (95% CI) | P value | OR* (95% CI) | P value | |||
Maximal LVEDWT (mm) | 1.508 (1.360–1.671) | <0.001 | 1.380 (1.210–1.574) | <0.001 | 1.276 (1.082–1.505) | 0.004 | ||
LVEDVi (mL/m2) | 0.966 (0.957–0.975) | <0.001 | ||||||
LVESVi (mL/m2) | 0.950 (0.938–0.962) | <0.001 | ||||||
LVEF (%) | 1.081 (1.064–1.099) | <0.001 | 1.082 (1.012–1.157) | 0.02 | 1.111 (1.063–1.161) | <0.001 | ||
LVMi (g/m2) | 0.994 (0.986–1.003) | 0.20 | ||||||
LVH asymmetry | 6.857 (4.146–11.341) | <0.001 | 2.927 (1.451–5.908) | 0.003 | ||||
SAM | 20.193 (4.851–84.053) | <0.001 | ||||||
Quantification of LGE (%) | 1.040 (1.011–1.070) | 0.007 | 1.067 (1.012–1.125) | 0.02 | ||||
LGE | 1.977 (1.179–3.315) | 0.01 | 0.050 (0.006–0.409) | 0.005 | ||||
Mid-wall LGE | 2.097 (1.297–3.391) | 0.003 | ||||||
RV insertion point LGE | 2.183 (1.399–3.406) | 0.001 | ||||||
Cine radscore | 2.450 (1.981–3.031) | <0.001 | 3.118 (1.967–4.943) | <0.001 | ||||
LGE radscore | 1.895 (1.633–2.201) | <0.001 | 2.631 (1.745–3.964) | <0.001 |
*, OR >1 means the higher the variable is, the higher the probability of HCM will be. OR, odds ratio; CI, confidence interval; LVEDWT, left ventricular end diastolic wall thickness; LVEDVi, left ventricular end diastolic volume index; LVESVi, left ventricular end systolic volume index; LVEF, left ventricular ejection fraction; LVMi, left ventricular mass index; LVH, left ventricular hypertrophy; SAM, systolic anterior motion; LGE, late gadolinium enhancement; RV, right ventricle.
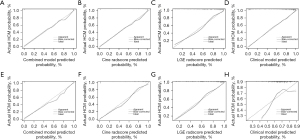
The optimal cut-off value of cine radscore, LGE radscore, combined radscore, clinical model and combined model were −0.40, 0.21, −0.44, 87.81 and 72.02, respectively. The score higher than the cut-off value indicate a higher likelihood of HCM. The sensitivity, specificity, positive predictive value (PPV), negative predictive value (NPV) and F1 score of different models when using the best cutoff values were shown in Table 4.
Table 4
Model | Sensitivity | Specificity | PPV | NPV | F1 score |
---|---|---|---|---|---|
Training group | |||||
Combined model | 0.952 | 0.883 | 0.945 | 0.898 | 0.950 |
Clinical model | 0.929 | 0.692 | 0.863 | 0.822 | 0.895 |
Cine radscore | 0.917 | 0.800 | 0.906 | 0.821 | 0.911 |
LGE radscore | 0.750 | 0.850 | 0.913 | 0.618 | 0.824 |
Combined radscore | 0.885 | 0.925 | 0.961 | 0.793 | 0.921 |
Validation group | |||||
Combined model | 0.970 | 0.863 | 0.937 | 0.932 | 0.953 |
Clinical model | 0.923 | 0.750 | 0.886 | 0.822 | 0.904 |
Cine radscore | 0.893 | 0.713 | 0.868 | 0.760 | 0.880 |
LGE radscore | 0.740 | 0.800 | 0.887 | 0.593 | 0.806 |
Combined radscore | 0.870 | 0.840 | 0.919 | 0.753 | 0.894 |
The best cut-off value of cine radscore, LGE radscore, combined radscore, clinical model and combined model were −0.40, 0.21, −0.44, 87.81 and 72.02, respectively. The score higher than the cut-off value indicate a higher likelihood of HCM. PPV, positive predictive value; NPV, negative predictive value; LGE, late gadolinium enhancement; HCM, hypertrophic cardiomyopathy.
We compared the AUC of the cine radscore, LGE radscore, combined radscore and combined model using different scanner types for both the training group and validation group, and no significant difference was found (Figure 5 & Table S4).
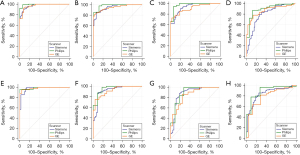
Reproducibility
All CMR parameters exhibited excellent reproducibility in both intraobserver and interobserver analyses (with ICC or kappa coefficients >0.8) (Table S5). Among the cine images, 1,091 features exhibited excellent reproducibility (ICC >0.8 in both intraobserver and interobserver analyses); 269 feature exhibited fair reproducibility (0.8≥ the lower ICC value in the intraobserver and interobserver analyses >0.5); 49 features exhibited poor reproducibility (intraobserver analyses and interobserver analyses showed at least one ICC ≤0.5). Among the LGE images, 1,155 features exhibited excellent reproducibility; 121 feature exhibited fair reproducibility; 133 features exhibited poor reproducibility.
Discussion
As the most common genetic heart disease, approximately 20,000,000 people may be affected by HCM (15). HCM is characterized by LVH, but this may also be found in other disorders, such as HHD. Accurate diagnosis of HCM is necessary for timely management of these patients (1).
In our study, we firstly summarized CMR discriminators between HHD and HCM patients. Then, we extracted radiomics features from cardiac cine and LGE images, which had great differential diagnostic value for HCM and HHD. Further discrimination models combined with multiparameter CMR findings and radiomics features were built in our study for the first time, which performed much better than the model including multiparameter CMR findings alone.
In HHD patients, increased LVEDWT is a result of LV remodeling, which results in a normalization of stroke volume (SV) (16,17). In HCM patients, increased LVEDWT is caused by sarcomere variants (1). Different patterns and degrees of wall thickening have been identified in HCM and HHD due to the different pathophysiologies (17,18). Patients with HHD often present with symmetrical and mild LVH, while patients with HCM often present with asymmetry and severe LVH (3). In our study, maximal LVEDWT and LVH asymmetry were useful to discriminate HHD from HCM in multivariable logistic regression. We further analyzed the maximal LVEDWT of these patients. We found that 186 (93%) HHD patients presented with maximal LVEDWT of 12–20 mm but that only 1 (0.5%) HHD patient presented with maximal LVEDWT ≥25 mm; 212 (50%) HCM patients presented with maximal LVEDWT of 15–20 mm, and 72 (17%) HCM patient presented with maximal LVEDWT ≥25 mm. Thus, a larger LVEDWT suggests a higher probability of HCM, and patients with maximal LVEDWT ≥25 mm are highly likely to be diagnosed with HCM. Moreover, the incidence of LVH asymmetry was higher in HCM patients (83%) than in HHD patients (44%), and it thus may be used as an index to differentiate HCM from HHD and build discrimination models.
HCM is characterized by a hypertrophied but nondilated LV, while dilated cardiac remodeling is the pathophysiology of HHD (1,19). Thus, a lower LVEDVi or LVESVi suggests a higher probability of HCM. Although increased wall thickness is compensatory in pressure overload and allows for preservation of LVEF in the early stage of LVH, progression to heart failure due to LV decompensation may occur (16,17). In our study, LVEF was significantly lower in HHD patients than in HCM patients; LVEF <45% was found in 32 (8%) HCM patients and 119 (60%) HHD patients. This may illustrate that heart failure is more common in HHD than HCM patients.
LGE can accurately reflect myocardial fibrosis, which is more frequently found in HCM patients than in HHD patients, and mid-wall LGE can be used as a discriminator between HCM and HHD (3,20,21). In this study, LGE was detected in 350 (83%) HCM and 130 (65%) HHD patients; quantification of LGE in HHD patients was also significantly lower than that in HCM patients. Despite the presence of LGE, quantification of LGE, mid-wall LGE and RV insertion point LGE was able to significantly discriminate HCM and HHD in univariate analysis, yet only quantification of LGE was significant in multivariate analysis. Thus, quantification of LGE rather than mid-wall LGE or RV insertion point LGE is recommended as a discriminator of HCM and HHD.
Radiomics is a rapidly evolving field in medical imaging and nuclear medicine, focusing on extracting quantitative and reproducible information from diagnostic images and including complex texture features that are difficult to quantify or recognize by the human eye (22,23). Although research on radiomics is mainly focused on cancer, recent studies have suggested that radiomics may also be used to predict LGE using cine images and improve the diagnostic accuracy of myocarditis (24,25).
This study used cine and LGE images to extract radiomics features, and built a discrimination model combined with radiomics features and multiparametric CMR findings. The final selected radiomics features quantified the differences in the morphology, texture, and voxel distribution of images between the two diseases with or without various filter. Radscores were calculated by the quantitative combination of these differences, which enables the differentiation of the two diseases. The discrimination model combined with the radscore performed much better than that using multiparameter CMR findings only, showed that the diagnostic accuracy of HCM increases significantly with the addition of radiomics techniques.
In this study, both the clinical model and the combined model showed excellent performance in discriminating HHD from HCM (with AUC >0.9), we further analyzed these models to understand how such excellent performances were obtained. The AUC of the discriminators in the clinical model and the combined model were calculated independently. Both maximal LVEDWT and LVEF showed good performance in distinguishing HHD from HCM (with AUC >0.8 in both the training group and the validation group), which contribute to the excellent performance of the clinical model. And in the combined model, the AUC of four discriminators (LVEF, maximal LVEDWT, cine radscore and LGE radscore) were higher than 0.8 in both the training group and the validation group, the combination of these discriminators resulted in an excellent distinguishing performance.
This study has several limitations. First, this was a single-center study, and the models built were not validated using external data. Although different scanners were used in this study to extract radiomics features and build discrimination models, the performance of these models showed no significant difference when different equipment was used. Additionally, T1 mapping was not included in our study, which may be useful to differentiate the two diseases, and this will be addressed in future research. Then, genetic test results were not included in this study because they were unavailable for most patients, which will be explored in future studies. Next, due to the high requirements of sample size for radiomics research, this study did not consider the discrimination of other diseases which may lead to LVH. This will be considered in future multicenter studies. Finally, due to our center’s status as a national hub for cardiovascular diseases in China, patients usually come to our center for treatment only when their condition is severe, the distribution of cases may be uneven.
Conclusions
In conclusion, the maximal LVEDWT, LVEF, LVH asymmetry and quantification of LGE, cine radscore and LGE radscore are effective CMR discriminators for HCM and HHD. Discrimination models combined with radiomics features were built in our study, and they performed much better than the model using multiparameter CMR findings only. The radscore calculated by radiomics features derived from cardiac cine and LGE images may be used for discriminating between HCM and HHD.
Acknowledgments
We would like to thank Prof. Paul Schoenhagen and the American Journal Experts (AJE) company for their help in polishing the paper.
Funding: This work was supported by National Natural Science Foundation of China (No. 82071875), Beijing Natural Science Foundation (No. 7212025), Beijing Natural Science Foundation (No. 7222302), and National Key Research and Development Program of China (Project No. 2021YFF0501400).
Footnote
Reporting Checklist: The authors have completed the STARD reporting checklist. Available at https://cdt.amegroups.com/article/view/10.21037/cdt-23-350/rc
Data Sharing Statement: Available at https://cdt.amegroups.com/article/view/10.21037/cdt-23-350/dss
Peer Review File: Available at https://cdt.amegroups.com/article/view/10.21037/cdt-23-350/prf
Conflicts of Interest: All authors have completed the ICMJE uniform disclosure form (available at https://cdt.amegroups.com/article/view/10.21037/cdt-23-350/coif). P.S. serves as the Editor-in-Chief of Cardiovascular Diagnosis and Therapy. The other authors have no conflicts of interest to declare.
Ethical Statement: The authors are accountable for all aspects of the work in ensuring that questions related to the accuracy or integrity of any part of the work are appropriately investigated and resolved. The study was conducted in accordance with the Declaration of Helsinki (as revised in 2013). The study was approved by the Institutional Review Board of the Beijing Anzhen Hospital (No. 2023178X) with a waiver for informed consent due to the retrospective analysis of the study.
Open Access Statement: This is an Open Access article distributed in accordance with the Creative Commons Attribution-NonCommercial-NoDerivs 4.0 International License (CC BY-NC-ND 4.0), which permits the non-commercial replication and distribution of the article with the strict proviso that no changes or edits are made and the original work is properly cited (including links to both the formal publication through the relevant DOI and the license). See: https://creativecommons.org/licenses/by-nc-nd/4.0/.
References
- Ommen SR, Mital S, Burke MA, et al. 2020 AHA/ACC Guideline for the Diagnosis and Treatment of Patients With Hypertrophic Cardiomyopathy: A Report of the American College of Cardiology/American Heart Association Joint Committee on Clinical Practice Guidelines. J Am Coll Cardiol 2020;76:e159-240. [Crossref] [PubMed]
- Licordari R, Trimarchi G, Teresi L, et al. Cardiac Magnetic Resonance in HCM Phenocopies: From Diagnosis to Risk Stratification and Therapeutic Management. J Clin Med 2023;12:3481. [Crossref] [PubMed]
- Rodrigues JC, Rohan S, Ghosh Dastidar A, et al. Hypertensive heart disease versus hypertrophic cardiomyopathy: multi-parametric cardiovascular magnetic resonance discriminators when end-diastolic wall thickness ≥ 15 mm. Eur Radiol 2017;27:1125-35. [Crossref] [PubMed]
- Rodrigues JC, Amadu AM, Dastidar AG, et al. Prevalence and predictors of asymmetric hypertensive heart disease: insights from cardiac and aortic function with cardiovascular magnetic resonance. Eur Heart J Cardiovasc Imaging 2016;17:1405-13. [Crossref] [PubMed]
- Arabadjian M, Nicolas B, Montgomery S, et al. Clinical course and outcomes in adults with co-occurring hypertrophic cardiomyopathy and hypertension: a scoping review protocol. BMJ Open 2023;13:e075087. [Crossref] [PubMed]
- Li Y, Liu Y, Liang Y, et al. Radiomics can differentiate high-grade glioma from brain metastasis: a systematic review and meta-analysis. Eur Radiol 2022;32:8039-51. [Crossref] [PubMed]
- Whelton PK, Carey RM, Aronow WS, et al. 2017 ACC/AHA/AAPA/ABC/ACPM/AGS/APhA/ASH/ASPC/NMA/PCNA Guideline for the Prevention, Detection, Evaluation, and Management of High Blood Pressure in Adults: Executive Summary: A Report of the American College of Cardiology/American Heart Association Task Force on Clinical Practice Guidelines. Circulation 2018;138:e426-83. [PubMed]
- Tadic M, Cuspidi C, Plein S, et al. Comprehensive assessment of hypertensive heart disease: cardiac magnetic resonance in focus. Heart Fail Rev 2021;26:1383-90. [Crossref] [PubMed]
- Mavrogeni S, Katsi V, Vartela V, et al. The emerging role of Cardiovascular Magnetic Resonance in the evaluation of hypertensive heart disease. BMC Cardiovasc Disord 2017;17:132. [Crossref] [PubMed]
- Feng Z, Rong P, Cao P, et al. Machine learning-based quantitative texture analysis of CT images of small renal masses: Differentiation of angiomyolipoma without visible fat from renal cell carcinoma. Eur Radiol 2018;28:1625-33. [Crossref] [PubMed]
- Xu X, Liu Y, Zhang X, et al. Preoperative prediction of muscular invasiveness of bladder cancer with radiomic features on conventional MRI and its high-order derivative maps. Abdom Radiol (NY) 2017;42:1896-905. [Crossref] [PubMed]
- Ji GW, Zhu FP, Xu Q, et al. Radiomic Features at Contrast-enhanced CT Predict Recurrence in Early Stage Hepatocellular Carcinoma: A Multi-Institutional Study. Radiology 2020;294:568-79. [Crossref] [PubMed]
- Ji GW, Zhang YD, Zhang H, et al. Biliary Tract Cancer at CT: A Radiomics-based Model to Predict Lymph Node Metastasis and Survival Outcomes. Radiology 2019;290:90-8. [Crossref] [PubMed]
- Grosu S, Wesp P, Graser A, et al. Machine Learning-based Differentiation of Benign and Premalignant Colorectal Polyps Detected with CT Colonography in an Asymptomatic Screening Population: A Proof-of-Concept Study. Radiology 2021;299:326-35. [Crossref] [PubMed]
- Maron BJ. Clinical Course and Management of Hypertrophic Cardiomyopathy. N Engl J Med 2018;379:655-68. [Crossref] [PubMed]
- Ismail TF, Frey S, Kaufmann BA, et al. Hypertensive Heart Disease-The Imaging Perspective. J Clin Med 2023;12:3122. [Crossref] [PubMed]
- Triposkiadis F, Sarafidis P, Briasoulis A, et al. Hypertensive Heart Failure. J Clin Med 2023;12:5090. [Crossref] [PubMed]
- Bazgir F, Nau J, Nakhaei-Rad S, et al. The Microenvironment of the Pathogenesis of Cardiac Hypertrophy. Cells 2023;12:1780. [Crossref] [PubMed]
- Tadic M, Cuspidi C, Marwick TH. Phenotyping the hypertensive heart. Eur Heart J 2022;43:3794-810. [Crossref] [PubMed]
- Tore D, Faletti R, Gaetani C, et al. Cardiac magnetic resonance of hypertrophic heart phenotype: A review. Heliyon 2023;9:e17336. [Crossref] [PubMed]
- Zhao S. Letter to the editor: is it time for imaging to level with pathology? Int J Cardiovasc Imaging 2020;36:2249-50.
- Gillies RJ, Kinahan PE, Hricak H. Radiomics: Images Are More than Pictures, They Are Data. Radiology 2016;278:563-77. [Crossref] [PubMed]
- Yip SS, Aerts HJ. Applications and limitations of radiomics. Phys Med Biol 2016;61:R150-66. [Crossref] [PubMed]
- Mancio J, Pashakhanloo F, El-Rewaidy H, et al. Machine learning phenotyping of scarred myocardium from cine in hypertrophic cardiomyopathy. Eur Heart J Cardiovasc Imaging 2022;23:532-42. [Crossref] [PubMed]
- Baessler B, Luecke C, Lurz J, et al. Cardiac MRI Texture Analysis of T1 and T2 Maps in Patients with Infarctlike Acute Myocarditis. Radiology 2018;289:357-65. [Crossref] [PubMed]