Artificial intelligence-enhanced automation of left ventricular diastolic assessment: a pilot study for feasibility, diagnostic validation, and outcome prediction
Highlight box
Key findings
• The current study developed an artificial intelligence (AI)-based framework for evaluating left ventricular diastolic function (LVDF) using echocardiography, which demonstrated a 94% concordance with manually-derived LVDF and significant prognostic value for clinical outcome.
What is known and what is new?
• LVDF assessment requires time demands of acquiring a comprehensive set of diastolic parameters and applying complex flowcharts, which challenges clinicians to adhere to the guideline recommendations consistently.
• The current study presented an AI-enhanced automated framework for diastolic assessment on echocardiography. The AI system automatically identified the required views and measured diastolic parameters precisely, leading to accurate discrimination of diastolic dysfunction concordant with the current guidelines. AI-derived diastolic assessment further demonstrated predictive capabilities for patient outcomes.
What is the implication, and what should change now?
• Our AI-based framework holds the potential to enhance diastolic assessment on echocardiography, capable of reducing human labour and time expenditures in routine clinical practice.
• The AI-enhanced approach accurately diagnoses diastolic dysfunction, thereby improving risk stratification for clinical outcomes.
Introduction
Background
The integration of artificial intelligence (AI) into the field of medicine is progressively alleviating human workloads by automating tasks traditionally requiring human labour. Transthoracic echocardiography, a crucial cardiac imaging modality, is also moving in this direction, with the initial focus on automating quantitative assessment. In the early stages, the emphasis was primarily on measuring left ventricular (LV) dimension and volumes, as well as calculating parameters such as the LV ejection fraction (LVEF) to assess LV systolic function (1-4). However, the scope of AI application is currently progressively expanding to encompass spectral and tissue Doppler imaging (TDI), as well as measurement of left atrial (LA) volume (5,6). This progression implies that AI-driven automated analysis is no longer limited to the assessment of LV systolic function; instead, it holds the potential to broaden its capabilities to include LV diastolic function (LVDF) assessment.
Rationale and knowledge gap
While assessing LVDF is a crucial aspect of a standard echocardiographic examination, an accurate evaluation of LVDF is not straightforward. Current guidelines encompass a comprehensive set of parameters derived from spectral and TDI, as well as two-dimensional (2D) echocardiography (7-10). Despite efforts to simplify the guidelines and make them more practical for daily clinical practice, the inclusion of numerous parameters and the need to follow complex flowcharts continue to present challenges. Consequently, adherence to these guidelines remains inconsistent in actual clinical practice, potentially leading to parameter omission or the subjective evaluation of diastolic function based on examiner experience (11).
Objective
In the current study, we have designed an AI-based pipeline by integrating multiple algorithms to fully automate the entire process of LVDF assessment. In this study, we aimed to determine the feasibility of the AI-based system for automatic LVDF assessment, encompassing accurate view classification, precise calculation of diastolic parameters and novel metrics such as LA reservoir strain (LARS), and ultimately, the precise assessment of LVDF. Additionally, we sought to investigate the prognostic value of AI-based automatically derived LVDF grades in predicting long-term clinical outcomes, in comparison to both manually derived LVDF grades and prior clinical assessments. We present this article in accordance with the STROBE reporting checklist (available at https://cdt.amegroups.com/article/view/10.21037/cdt-24-25/rc).
Methods
Study population
The AI-based framework utilized in this study was developed and validated using the Open AI Dataset Project (AI-Hub) dataset, which was initiated by the South Korean government (Ministry of Science and ICT) (12). The dataset consists of 30,000 echocardiographic examinations, retrospectively collected from five tertiary hospitals between 2012 and 2021. It encompasses a wide range of cardiovascular diseases categorises, including normal cases, ischemic heart disease, cardiomyopathy, pulmonary hypertension and embolism, pericardial disease, valvular heart disease, cardiac mass, congenital heart disease, etc. For external validation of automatic Doppler measurement algorithms, we also utilized a separate dataset of 100 patients who underwent echocardiography at the Mayo Clinic in Arizona, USA.
To determine the feasibility and accuracy of our AI-based approach for LVDF assessment, we compiled a retrospectively screened patients with suspected diastolic dysfunction who underwent transthoracic echocardiography at Seoul National University Bundang Hospital between May 2012 and June 2022. Given that our main objective was to evaluate the feasibility and accuracy of AI-based LVDF assessment in alignment with current guidelines, we carefully curated our study population. We included patients with New York Heart Association functional class II or worse dyspnea and preserved LVEF of 50% or higher, ensuring that LVDF assessment could be conducted following the guideline’s recommended flow. Conversely, we excluded patients who were missing diastolic parameters due to a lack of image acquisition, as these factors can render the determination of LVDF according to the current guidelines (8-10). In addition, to maintain consistency with the recommended flow of LVDF assessment, patients with the following disease groups, which cause difficulties in applying the diagnostic algorithm, were excluded: (I) mitral valve disease (mitral stenosis of any severity, moderate-to-severe mitral regurgitation, prosthetic mitral valve and annuloplasty rings, and moderate-to-severe mitral annular calcification); (II) hypertrophic cardiomyopathy; (III) pericardial constriction; (IV) atrial fibrillation or atrial flutter; (V) left bundle branch block; (VI) right ventricular or biventricular pacing; and (VII) LV assist devices (10). Finally, 167 patients were included for analysis. Additionally, we included 33 individuals with normal echocardiographic findings as the control group. These individuals were randomly selected from those who underwent transthoracic echocardiography as a part of routine health evaluation and exhibited normal findings (see https://cdn.amegroups.cn/static/public/cdt-24-25-1.pdf).
The study was conducted in accordance with the Declaration of Helsinki (as revised in 2013). The study was approved by institutional review boards of Seoul National University Bundang Hospital (No. B-2301-807-114) and Mayo Clinic in Arizona (No. 21-009795), and the requirement for informed consent was waived because of the retrospective and observational nature of the study design. All clinical and echocardiographic data were fully anonymized before data analysis.
Fully automated LV diastolic function evaluation
Our AI-based system automated the conventional human workflow for LVDF evaluation. This included the following processes: (I) identifying the necessary 2D or Doppler views; (II) conducting corresponding measurements and calculating diastolic parameters; and (III) determining the presence and severity of diastolic dysfunction (Figure 1).
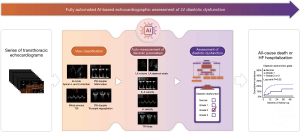
In the initial stage of the AI pipeline, the view classification algorithm automatically identified the essential views required for diastolic functional assessment on echocardiography. The current version of the view classification algorithm was designed to classify the 31 most commonly acquired echocardiographic views, including 15 B-mode, 3 M-mode, and 13 spectral or tissue Doppler classes, with the capability of out-of-distribution detection (see https://cdn.amegroups.cn/static/public/cdt-24-25-1.pdf). In this study, specifically for diastolic function evaluation, the algorithm selected the following necessary views: apical 4- and 2-chamber views, along with pulsed wave (PW) Doppler at the mitral leaflet tips, TDI at the mitral annulus, and continuous wave (CW) Doppler to assess peak tricuspid regurgitation (TR) velocity.
In the second stage, the suitable algorithm for each selected view was chosen, and the required measurements were performed. For apical 4-chamber and 2-chamber views, the segmentation model automatically delineated the LA cavity (see https://cdn.amegroups.cn/static/public/cdt-24-25-1.pdf). Subsequently, the LA volume was calculated using the biplane anterior-to-posterior length method at the LV end-systolic phase (13). For patients with echocardiograms with multiple apical views or apical views spanning multiple cardiac cycles, the LA area was assessed on all cardiac cycles within all images. The image and cardiac cycle displaying the maximal LA area were chosen for the calculation of the LA volume. Additionally, on apical 4-chamber views, LARS was quantified by tracking the motion of the LA wall, utilizing the QRS complex on the electrocardiography as the reference time point (see https://cdn.amegroups.cn/static/public/cdt-24-25-1.pdf).
For spectral and TDI, the segmentation model automatically segmented the Doppler envelope, and the post-processing algorithm extracted measurements from each Doppler segmentation (see https://cdn.amegroups.cn/static/public/cdt-24-25-1.pdf). Specifically, the system calculated the early diastolic mitral inflow velocity (E velocity), late diastolic mitral inflow velocity (A velocity), and deceleration time from PW Doppler mitral valve views; early diastolic mitral annular velocity (e' velocity), late diastolic mitral annular velocity (a' velocity), and systolic annular velocity (s' velocity) from septal and lateral mitral annulus TDI, and maximal velocity (Vmax) and velocity time integral from CW Doppler TR imaging. Although the Doppler analysis algorithm could distinguish between septal and lateral mitral annulus TDI and analyze both (14), in the present study, not all cases included both septal and lateral TDI. To ensure consistency in the analysis, we used only septal mitral annulus TDI and derived the septal e' velocity (e’sep) to evaluate diastolic function in all cases. For spectral or tissue Doppler images, where a single image typically captures three to five cardiac cycles, automatic measurements were conducted on all measurable cardiac cycles and subsequently averaged.
Finally, the presence and severity of diastolic dysfunction were determined according to current guidelines (8-10). Firstly, the presence of diastolic dysfunction was assessed by evaluating the following diastolic parameter criteria: (I) E/e’sep >15, (II) e’sep <7 cm/sec, (III) TR velocity >2.8 m/sec, and (IV) LA volume index (LAVI) >34 mL/m2. If zero or one parameter criterion was met, normal diastolic function was determined. If two criteria were met, the result was considered as indeterminate. If three to four criteria were met, diastolic dysfunction was considered to be present. Subsequently, in patients with clinically suspected or diagnosed diastolic dysfunction, the severity of diastolic dysfunction was assessed. In our study, since the patient group already consisted of individuals clinically suspected of diastolic dysfunction, the severity of diastolic dysfunction was evaluated in all these cases. For grading diastolic dysfunction, cases with the ratio of E velocity to A velocity (E/A) <0.8 and E velocity <50 cm/sec were classified as grade 1 diastolic dysfunction. Conversely, cases with E/A ratio >2 were classified as grade 3 diastolic dysfunction. For remaining cases, diastolic dysfunction was further evaluated using the following parameter criteria: (I) E/e’sep >15, (II) TR velocity >2.8 m/sec, and (III) LAVI >34 mL/m2. Cases in which zero or one parameter criterion was met were classified as grade 1 diastolic dysfunction; cases in which two to three criteria were met were classified as grade 2 diastolic dysfunction.
Conventional evaluation of LVDF
To evaluate the accuracy of LVDF assessment by the AI framework, we compared it against conventional evaluations. An independent observer over 5-year of clinical experience in echocardiography conducted the evaluations in the similar manner as the AI-enhanced assessments. Specifically, the observer selected the necessary views and performed manual measurements to obtain the required diastolic parameters, as well as LARS, using commercially available software (TOMTEC ARENA TTA2, TOMTEC IMAGING SYSTEMS GMBH, Munich, Germany). Subsequently, LVDF was assessed following the guideline recommendations (8-10). Additionally, to provide a comparison with real-world clinical practice, we collected information on clinician’s prior assessment of LVDF from the echocardiographic reports, without any alteration or influence by the investigators.
Ascertainment of clinical information and outcome definition
The clinical information, including demographics, clinical risk factors, laboratory test results, medications, and outcome events, were obtained through a dedicated review of the electronic health records at the study institution, which was founded in fully digitalized form (15). The clinical endpoint was defined as a composite of all-cause death and hospitalization for heart failure. All-cause death was confirmed through linkage with national death registries. Hospitalization for heart failure was defined based on documented symptoms (e.g., dyspnea, edema), findings on physical examination (e.g., pulmonary rales, jugular venous distension), and supporting evidence from diagnostic tests (e.g., chest X-ray showing pulmonary congestion, elevated natriuretic peptides), as well as the final diagnosis made by a cardiologist, as documented in the electronic health records.
Statistical analysis
Firstly, we assessed the accuracy of the view classification algorithm in identifying the necessary views for LVDF evaluation. The view classification algorithm’s results were compared to the label assigned by human experts. Precision (positive predictive value), recall (sensitivity), and the F1 score were calculated for each view. Overall accuracy was determined by dividing the number of correctly classified images by the total number of images. We then evaluated the concordance between AI-derived and manually-derived diastolic parameter values (E velocity, E/A ratio, e’sep velocity, E/e’sep, TR velocity, and LAVI). Additionally, we compared AI-derived LARS values with those manually measured following recommended guidelines (16). The association between the two sets of parameter values was evaluated by creating scatterplots and calculating the Pearson correlation coefficient (r) and mean absolute error (MAE). Agreement between the two sets was further evaluated by Bland-Altman analysis, which included the mean difference and lower and upper limits of agreement. In addition, we evaluated the discrimination ability of AI-derived measurements for identifying abnormal manually derived diastolic parameter values (E/e’sep >15, e’sep <7 cm/sec, TR velocity >2.8 m/sec, and LAVI >34 mL/m2). The area under the receiver operating characteristic curve (AUC) was calculated for this purpose. Finally, we compared the AI-derived guideline-directed LVDF grade to two other methods: (I) manually-derived guideline-directed assessment, and (II) clinician’s prior assessment, which may reflect possible inconsistency in real-world adherence to guidelines (11). We evaluated the concordance by employing confusion matrix plots and calculating overall agreement rates.
For outcome prediction, event curves were plotted to illustrate the cumulative incidence of the composite endpoint according to LVDF grade based on each assessment method (AI-derived, manually-derived, and prior clinician’s assessments). Significance in the event-free-period was assessed using the log-rank test. Independent association between AI-derived and manually-derived diastolic parameter values and the composite endpoint were evaluated using Cox-regression risk modelling, with satisfying proportional hazards assumption. Hazard ratios (HRs) were estimated, with adjustment for clinical covariates, including age, sex, hypertension, diabetes, dyslipidaemia, and LVEF.
All statistical analyses were performed using Python 3.8.8, with open-source Python libraries (SciPy 1.6.2, statsmodels 0.12.2, and pingouin 0.5.2), and R software, V.4.1.1 (R Development Core Team, Vienna, Austria). Two-sided P values <0.05 were considered statistically significant.
Results
The clinical characteristics of the study population are presented in Table 1. Patients with suspected LV diastolic dysfunction were significantly older (median age, 77 years) than those in the normal control group (median age, 44 years), and presented with a greater prevalence of clinical risk factors. However, the baseline LVEF was comparable between the two groups (median, 64% vs. 63%). The diastolic dysfunction group had higher rates of medical treatment than the normal control group. All participants exhibited sinus rhythm on the baseline electrocardiogram.
Table 1
Total population | Diastolic dysfunction (n=167) | Normal control (n=33) | Pdifference |
---|---|---|---|
Age (years) | 77 (70–82) | 44 (35–55) | <0.001* |
Male | 54 (32.3) | 14 (42.4) | 0.36 |
BMI (kg/m2) | 24.8 (22.6–27.0) | 21.3 (19.7–23.4) | <0.001* |
NYHA Fc ≥2 | 167 (100.0) | 0 (0.0) | <0.001* |
Hypertension | 130 (77.8) | 2 (6.1) | <0.001* |
Diabetes mellitus | 56 (33.5) | 1 (3.0) | 0.001* |
Dyslipidaemia | 37 (22.2) | 3 (9.1) | 0.14 |
Smoker | 34 (20.4) | 1 (3.0) | 0.04* |
Stroke | 12 (7.2) | 0 (0.0) | 0.24 |
Coronary disease | 47 (28.1) | 0 (0.0) | 0.001* |
Hb (g/dL) | 12 (10–13) | 13 (12–15) | <0.001* |
GFR (mL/min) | 65 (40–81) | 100 (89–116) | <0.001* |
LVEF (%) | 64 (59–68) | 63 (61–66) | 0.75 |
RAS inhibitors | 79 (47.3) | 1 (3.0) | <0.001* |
Beta-blockers | 65 (38.9) | 1 (3.0) | <0.001* |
MRA | 18 (10.8) | 0 (0.0) | 0.10 |
Statin | 73 (43.7) | 1 (3.0) | <0.001* |
Follow-up (months) | 21 (8–49) | 10 (1–79) | 0.25 |
Values are presented as median (interquartile range) or number (percentage). *, represents significant difference. BMI, body mass index; NYHA Fc, New York Heart Association functional class; Hb, haemoglobin; GFR, glomerular filtration rate; LVEF, left ventricular ejection fraction; RAS, renin-angiotensin system; MRA, mineralocorticoid receptor antagonist.
In the initial phase of LVDF assessment, the AI-based framework autonomously identifies necessary views, including apical 4- and 2-chamber views, PW Doppler mitral valve, mitral annulus TDI, and CW Doppler TR. The algorithm achieved an overall view classification accuracy of 99.1%. Precision and recall metrics for each specific view were robust, with precision values ranging 0.948–0.997 and recall values ranging 0.937–0.978. Additional details, including metrics and the confusion matrix, are available in the supplementary online material (see https://cdn.amegroups.cn/static/public/cdt-24-25-1.pdf). Given the relatively low number of TDI images for lateral mitral annulus, we only used septal annulus TDI for LVDF assessment.
The AI-based framework then automatically calculates diastolic parameters specific to each view, successfully measuring all diastolic parameters in every study subject. The average running time of the AI-based framework to derive the full list of diastolic parameters was less than 10 seconds (https://cdn.amegroups.cn/static/public/cdt-24-25-1.pdf). These AI-derived automatic measurements exhibited robust correlations and agreement with manually-derived measurements: r=0.907 (MAE 0.1 m/s) for E velocity; r=0.901 (MAE 0.1) for E/A ratio; r=0.957 (MAE 0.5 cm/s) for e' velocity; r=0.927 (MAE 2.4) for E/e' ratio; r=0.959 (MAE 0.1 m/s) for TR Vmax; and r=0.928 (MAE 5.8 mL/m2) for LAVI (Figure 2). Additionally, the Bland-Altman plots demonstrated good agreement between AI-derived and manually-derived measurements. Furthermore, AI-derived measurements demonstrated excellent performance in identifying abnormal manually derived diastolic parameter values, with AUC values of 0.961 for septal e' velocity <7 cm/s, 0.966 for E/e' ratio >15, 0.967 for TR Vmax >2.8 m/s, and 0.977 for LAVI >34 mL/m2 (see https://cdn.amegroups.cn/static/public/cdt-24-25-1.pdf).
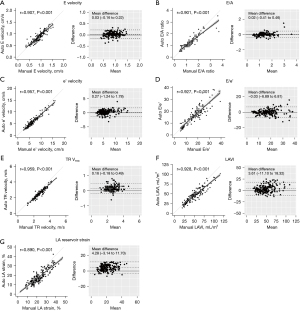
When evaluating the presence of diastolic dysfunction based on guideline-directed assessment using AI-derived measurements, all 33 control subjects were classified as normal. Among the 167 patients, 112 were classified as having diastolic dysfunction, and 55 were labeled as indeterminate. Similarly, when guideline-directed assessment was conducted based on manually-derived measurements, all 33 control subjects were classified as normal and 113 of 167 suspected diastolic dysfunction patients were determined to have diastolic dysfunction, while 54 were labeled as indeterminate. However, since all 167 patients were clinically suspected of having diastolic dysfunction, the grading of diastolic dysfunction was performed for all of them. This resulted in the creation of a confusion matrix, as shown in Figure 2. As a result, AI-derived LVDF grades exhibited a high level of agreement with manually-derived guideline-directed LVDF grades (94.0%) (Figure 3A). In contrast, there was lower concordance with the clinician’s prior assessment (77.5%) (Figure 3B). This inconsistency was not limited to AI-derived LVDF grades but also applied to manually-derived LVDF grades (78.5%) (Figure 3C). These findings suggest inconsistent adherence to guidelines in real-world practice.
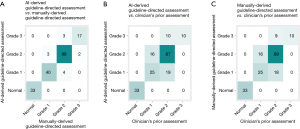
During a median follow-up of 20.1 (range, 6.1–53.7) months, the composite endpoint occurred in 28 (14%) participants (death in 9 cases and heart failure hospitalization in 22 cases). The risk curves for the composite endpoint indicated that as LVDF grade increased, the risk gradually rose (Figure 4); this trend was evident for both AI-derived and manually-derived LVDF grade assessments (log-rank P=0.02 and P=0.01, respectively). However, for the clinician’s prior assessment of LVDF grade, the significance was marginal (log-rank P=0.05). Since none of the participants in the normal LVDF group experienced adverse outcomes, this group was not used as a reference group in the Cox regression analysis. Instead, a reference group comprising individuals with either normal or grade 1 diastolic dysfunction was employed (Table 2). Both AI-derived and manually-derived assessments of grade 2 or 3 dysfunction exhibited independent prognostic value, even after adjusting for clinical risk factors such as age, sex, hypertension, diabetes, dyslipidaemia, and LVEF (adjusted HR =2.75; P=0.04 and adjusted HR =3.03; P=0.03, respectively). However, the predictive ability of grade 2 or 3 dysfunction in the clinician’s prior assessment was relatively weaker and did not remain significant after adjusting for clinical risk factors (adjusted HR =1.63; P=0.36).
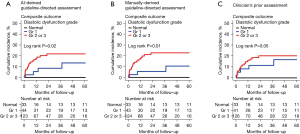
Table 2
Variables | Univariate model | Multivariate model 1† | Multivariate model 2‡ | |||||
---|---|---|---|---|---|---|---|---|
HR (95% CI) | P value | HR (95% CI) | P value | HR (95% CI) | P value | |||
Age, per 10-year increase | 1.31 (0.92–1.85) | 0.13 | – | – | – | – | ||
Male sex | 2.65 (1.26–5.57) | 0.01* | – | – | – | – | ||
LVEF, per 10% decrease | 2.30 (1.15–4.57) | 0.02* | – | – | – | – | ||
Manually-derived measurements | ||||||||
e' velocity, per 1-cm/s decrease | 1.38 (1.09–1.73) | 0.007* | 1.37 (1.06–1.77) | 0.02* | 1.25 (0.96–1.63) | 0.09 | ||
E/e', per 1-unit increase | 1.05 (1.02–1.08) | 0.001* | 1.05 (1.02–1.09) | <0.001* | 1.05 (1.01–1.09) | 0.007* | ||
TR Vmax, per 1-m/s increase | 1.25 (0.63–2.49) | 0.53 | 1.10 (0.53–2.30) | 0.80 | 0.87 (0.44–1.71) | 0.68 | ||
LAVI, per 1-mL/m2 increase | 1.04 (1.02–1.05) | <0.001* | 1.04 (1.02–1.05) | <0.001* | 1.03 (1.02–1.05) | <0.001* | ||
LA reservoir strain, per 1% decrease | 1.05 (1.00–1.10) | 0.05 | 1.04 (0.98–1.10) | 0.20 | 1.03 (0.97–1.09) | 0.36 | ||
AI-derived measurements | ||||||||
e' velocity, per 1-cm/s decrease | 1.35 (1.07–1.71) | 0.01* | 1.37 (1.06–1.78) | 0.02* | 1.26 (0.97–1.64) | 0.08 | ||
E/e', per 1-unit increase | 1.05 (1.02–1.08) | <0.001* | 1.06 (1.03–1.09) | <0.001* | 1.05 (1.02–1.09) | 0.004* | ||
TR Vmax, per 1-m/s increase | 1.06 (0.51–2.20) | 0.88 | 0.88 (0.41–1.89) | 0.74 | 0.71 (0.36–1.42) | 0.33 | ||
LAVI, per 1-mL/m2 increase | 1.04 (1.02–1.05) | <0.001* | 1.04 (1.02–1.05) | <0.001* | 1.03 (1.02–1.05) | <0.001* | ||
LA reservoir strain, per 1% decrease | 1.08 (1.01–1.15) | 0.03* | 1.06 (0.99–1.14) | 0.12 | 1.05 (0.98–1.12) | 0.20 | ||
Manually-derived guideline-directed assessment | ||||||||
Normal or grade 1 dysfunction | Reference | Reference | Reference | |||||
Grade 2 or 3 dysfunction | 4.12 (1.41–12.04) | 0.01* | 3.91 (1.29–11.87) | 0.02* | 3.03 (1.16–9.14) | 0.03* | ||
AI-derived guideline-directed assessment | ||||||||
Normal or grade 1 dysfunction | Reference | Reference | Reference | |||||
Grade 2 or 3 dysfunction | 3.24 (1.21–8.67) | 0.02* | 3.14 (1.11–8.89) | 0.03* | 2.75 (1.02–7.88) | 0.04* | ||
Clinician’s prior assessment | ||||||||
Normal or grade 1 dysfunction | Reference | Reference | Reference | |||||
Grade 2 or 3 dysfunction | 2.74 (1.03–7.26) | 0.04* | 2.17 (0.77–6.12) | 0.14 | 1.63 (0.58–4.60) | 0.36 |
†, adjusted for age, and sex; ‡, adjusted for age, sex, hypertension, diabetes, dyslipidaemia, and LVEF; *, represents significant difference. AI, artificial intelligence; HR, hazard ratio; CI, confidence interval; LVEF, left ventricular ejection fraction; e' velocity, early diastolic mitral annular velocity; E/e', ratio of early diastolic mitral inflow velocity to early diastolic mitral annular velocity; TR Vmax, tricuspid regurgitation maximal velocity; LAVI, left atrial volume index; LA, left atrium.
Further, AI-derived and manually-derived measurements of LARS were highly correlated (r=0.890, MAE 3.1%) (Figure 2G). Additionally, as LVDF worsened, the LARS gradually decreased, with a significant trend (P for trend <0.001) (Figure 5A). Furthermore, when the study population was stratified according to the median LARS value (17%), patients with LARS <17% showed a higher rate of the composite endpoint compared to that for patients with LARS ≥17% (log-rank P=0.04) (Figure 5B). Lower LARS was associated with increased risk of the composite endpoint on Cox regression analysis, although this relationship did not remain significant after adjusting for clinical risk factors (Table 2).
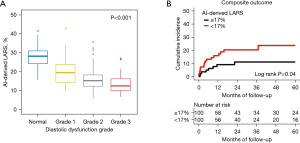
Discussion
Key findings
The present study successfully demonstrated the feasibility and accuracy of an AI-based framework for fully automating LVDF assessment on echocardiography. The AI-based approach precisely identified the required views, measured diastolic parameters accurately, and confirmed abnormalities. Consequently, it enabled an accurate evaluation of LVDF in accordance with current guidelines and exhibited excellent predictive capabilities for patient outcomes. Furthermore, the present study showcased the system’s ability to accurately measure LARS, a potential diastolic parameter.
Strengths and limitations
A key strength of this study is the careful design and validation of our AI-based fully automated LVDF assessment, which adheres closely to current guidelines. We confirmed a high level of agreement between AI-derived and manually-derived diastolic parameters, and showed strong alignment between LVDF grades obtained from our AI-based framework and those derived manually according to guidelines. Furthermore, our comparison with clinician’s prior assessments highlights the disparities between guideline-directed practice and real-world clinical application.
Another significant strength of the present study is the inclusion of clinical outcome data, which allowed us to demonstrate that AI-based diastolic parameters and LVDF grades have comparable excellent prognostic capabilities to manually-derived parameters and grades. Furthermore, our findings indicate that both AI-derived and manually-derived LVDF grading according to current guidelines demonstrated superior prognostic value to that for conventional grading by clinicians. This implies that employing an AI-based approach not only enables a more precise evaluation of LVDF, with savings in time and effort, but also enhances the quality of care. Additionally, given its predictive capabilities for patient outcomes, AI-based approaches have potential in significantly improving the prognosis.
Furthermore, AI-based automation can include additional parameters, such as LARS without requiring additional time or effort. Our AI-based framework was able to calculate the LARS, as well as the LAVI, when the apical 4-chamber view was provided. Further, the automatically-derived LARS values were highly correlated with manually-derived LARS values and exhibited prognostic value. Importantly, by harnessing AI, future advancements could automate the measurement of additional diastolic parameters, such as the LV global longitudinal strain, isovolumic relaxation time, transmitral Doppler’s A duration, and pulmonic vein Doppler’s A-wave flow reversal duration, which are challenging to assess in real-world clinical scenarios due to time constraints. Thus, a significantly larger array of metrics could be accessed, potentially addressing current limitations in LVDF assessment, especially in cases where conventional parameters are limited or inconclusive.
Despite the promising results, it is important to consider some limitations when interpreting our findings. First, the sample size was relatively small. However, this limited sample size allowed for a comprehensive evaluation of LVDF, facilitating a robust validation of the AI-based framework’s feasibility and accuracy. Nonetheless, further validation is warranted with larger sample sizes in real-world settings. Specifically, since our patient group underwent echocardiography due to suspected diastolic dysfunction, we assessed LVDF grade, recognizing the possibility of its presence even when the presence of diastolic dysfunction was indeterminate (i.e., only two positive findings). Moreover, as there were no missing diastolic parameter values, we did not encounter cases where we could not determine the LVDF grade. Although many patients did not have lateral annulus TDI images, necessitating the use of only the e' values measured from the septal TDI, this situation is common in daily clinical practice. The guidelines accommodate such scenarios by providing criteria for evaluation based on the available TDI images, whether septal or lateral, thus ensuring that the absence of lateral annulus TDI measurements did not pose a problem for our study. Therefore, this study validated the functionality of AI-derived LVDF assessments in a group where clinicians suspected diastolic dysfunction and evaluated it through echocardiography. Consequently, additional consideration and validation are required before expanding this approach to unselected populations. Specifically, since this study focused solely on patients with preserved LVEF, further validation is necessary for those with reduced LVEF. To address this, we are planning further research on a larger scale within an unselected heart failure population.
Furthermore, we excluded certain disease groups, such as hypertrophic cardiomyopathy and moderate-to-severe mitral annular calcification, for which the current guidelines provide specific diagnostic algorithms for evaluating LVDF (8-10). Hence, AI-based automatically-derived LVDF assessment should be re-evaluated within disease-specific guideline-based workflows to further assess its applicability and accuracy.
Lastly, in this study, the AI-driven automatic LARS measurement results were compared only with manual measurements using a specific strain analysis program (TOMTEC software). Given that our system is vendor-neutral and capable of analyzing images from any type of echocardiography equipment, future comparisons with various strain analysis programs are also necessary.
Comparison with similar researches
Over the past decade, remarkable advancements in AI technology have spurred numerous efforts to automate various processes in the field of echocardiography (4,17-19). Initially, much of the focus was on automating the assessment of LV volume and systolic function (1,5,17,18). However, the scope has expanded to encompass the evaluation of LVDF (5,6,20). Tromp et al. proposed a fully automated deep learning workflow for assessing both LV systolic and diastolic function and evaluated its performance across different cohorts (5). However, their evaluation of LVDF focused solely on the accuracy of automatically-derived measurements of specific diastolic parameters, including the E velocity, e' velocity, E/e' ratio, and LA volume. Validation of the E/A ratio and TR velocity were omitted, and most notably, the assessment of LVDF grade, based on the combination of the automatically derived measurements according to current guidelines, was missing. More recently, Chen et al. proposed and validated a workflow similar to ours, encompassing automated view classification, diastolic parameter measurement, and diastolic dysfunction grading following established guidelines (6). The concordance between automatically and manually derived grades was 92%, which is close to that in the present study. However, the study by Chen et al. (6). lacked outcome data, precluding an evaluation of the prognostic capabilities of their AI-based automatically-derived diastolic parameter measurement and the resulting diastolic dysfunction grading.
Explanation of findings
Evaluating LVDF is undeniably a crucial facet of echocardiography, yet it entails a complex procedure involving the assessment of various parameters to ensure accuracy (7-10). This complexity often leads to the omission of many elements of LVDF assessment in clinical practice, and the subjectivity of interpretation can escalate when diverse parameters yield conflicting outcomes (11). While efforts have been made to simplify guidelines for LVDF assessment, they are still perceived as intricate by many, and their practical implementation in clinical settings remains restricted. However, the results of the present study underscore the importance of adherence to these guidelines. Whether assessed manually or using AI-based automation, our findings show that guideline-based assessments provide superior prognostic capabilities compared to that with a conventional clinician’s assessment. Our AI-based framework not only accurately assists in these tasks but also saves healthcare professionals’ time and effort, while simultaneously providing excellent prognostic prediction capabilities.
Implications and action needed
The advancement of AI in automating the measurement of various echocardiographic parameters is evolving beyond simply automating manual measurements. It is ultimately progressing towards automating the evaluation of diverse cardiac functions intended in echocardiography. This study highlights the feasibility of automatically evaluating LVDF using AI-based framework, which has been largely overlooked in clinical practice due to practical limitations. By demonstrating the capability to automate LVDF assessment, it suggests a potential shift in the workflow of echocardiographic examinations. This could enable the measurement of a broader range of parameters without additional time and effort, allowing for more comprehensive and in-depth evaluations than previously feasible. However, a valid concern arises regarding whether we can accurately interpret and effectively utilize this wealth of provided metrics. Nevertheless, progress in AI is opening doors to solutions for effectively utilizing an abundance of parameters at our disposal. In recent studies, various efforts have been made to develop AI models for predicting LVDF by integrating echocardiography and clinical data (20-24). These models incorporate not only conventional echocardiography diastolic parameters but also strain values and novel parameters obtained from speckle tracking images and have shown promising performance in predicting increased LV filling pressure and identifying high-risk phenotypes associated with diastolic dysfunction (21,23,25). While there are still many challenges to address before AI-based LVDF assessment becomes widely used in clinical practice, these endeavours offer valuable insights. They also suggest that in the future, we may be able to utilize a more extensive array of parameters more efficiently and easily than previously possible.
Conclusions
The implementation of an AI-based fully automated system demonstrated feasibility for LVDF assessment on echocardiography. This approach holds potential in enhancing the diagnostic accuracy of diastolic dysfunction, thereby improving risk stratification for clinical outcomes. However, the findings of this pilot study necessitate further validation with a larger sample size and in a real-world setting. Specifically, an evaluation of whether our AI-based system can reduce human labour and time expenditures during echocardiography in routine clinical practice is required.
Acknowledgments
Funding: This work was supported by a grant from
Footnote
Reporting Checklist: The authors have completed the STROBE reporting checklist. Available at https://cdt.amegroups.com/article/view/10.21037/cdt-24-25/rc
Data Sharing Statement: Available at https://cdt.amegroups.com/article/view/10.21037/cdt-24-25/dss
Peer Review File: Available at https://cdt.amegroups.com/article/view/10.21037/cdt-24-25/prf
Conflicts of Interest: All authors have completed the ICMJE uniform disclosure form (available at https://cdt.amegroups.com/article/view/10.21037/cdt-24-25/coif). Y.E.Y. is Chief Medical Officer of Ontact Health, Inc. J.J., Y.J., Y.H., S.H., and S.A.L. are currently affiliated with Ontact Health, Inc. J.J. and J.K. are co-inventors on a patent related to this work filed by Ontact Health (Method And Device For Acquiring Information From Temporal Information Represented Medical Images; Method Of Acquiring Information From Temporal Information Represented Medical Images And Device For The Same). J.J., J.K., and S.H. are co-inventors on a patent related to this work filed by Ontact Health (Method And Device For Providing Information On Ultrasound Image View Based On Out-Of-Distribution Data Detection). Y.J., Y.H., and S.H. are co-inventors on a patent related to this work filed by Ontact Health (Method For Providing Information Of Organ Function And Device For Providing Information Of Organ Function Using The Same). H.J.C. holds stock in Ontact Health, Inc. The other authors have no conflicts of interest to declare.
Ethical Statement: The authors are accountable for all aspects of the work in ensuring that questions related to the accuracy or integrity of any part of the work are appropriately investigated and resolved. The study was conducted in accordance with the Declaration of Helsinki (as revised in 2013). The study was approved by institutional review boards of Seoul National University Bundang Hospital (No. B-2301-807-114) and Mayo Clinic in Arizona (No. 21-009795). The requirement for informed consent was waived because of the retrospective and observational nature of the study design. All clinical and echocardiographic data were fully anonymized before data analysis.
Open Access Statement: This is an Open Access article distributed in accordance with the Creative Commons Attribution-NonCommercial-NoDerivs 4.0 International License (CC BY-NC-ND 4.0), which permits the non-commercial replication and distribution of the article with the strict proviso that no changes or edits are made and the original work is properly cited (including links to both the formal publication through the relevant DOI and the license). See: https://creativecommons.org/licenses/by-nc-nd/4.0/.
References
- Zhang J, Gajjala S, Agrawal P, et al. Fully Automated Echocardiogram Interpretation in Clinical Practice. Circulation 2018;138:1623-35. [Crossref] [PubMed]
- Leclerc S, Smistad E, Pedrosa J, et al. Deep Learning for Segmentation Using an Open Large-Scale Dataset in 2D Echocardiography. IEEE Trans Med Imaging 2019;38:2198-210. [Crossref] [PubMed]
- Kusunose K, Haga A, Yamaguchi N, et al. Deep Learning for Assessment of Left Ventricular Ejection Fraction from Echocardiographic Images. J Am Soc Echocardiogr 2020;33:632-635.e1. [Crossref] [PubMed]
- Asch FM, Descamps T, Sarwar R, et al. Human versus Artificial Intelligence-Based Echocardiographic Analysis as a Predictor of Outcomes: An Analysis from the World Alliance Societies of Echocardiography COVID Study. J Am Soc Echocardiogr 2022;35:1226-1237.e7. [Crossref] [PubMed]
- Tromp J, Seekings PJ, Hung CL, et al. Automated interpretation of systolic and diastolic function on the echocardiogram: a multicohort study. Lancet Digit Health 2022;4:e46-54. [Crossref] [PubMed]
- Chen X, Yang F, Zhang P, et al. Artificial Intelligence-Assisted Left Ventricular Diastolic Function Assessment and Grading: Multiview Versus Single View. J Am Soc Echocardiogr 2023;36:1064-78. [Crossref] [PubMed]
- Nagueh SF, Appleton CP, Gillebert TC, et al. Recommendations for the evaluation of left ventricular diastolic function by echocardiography. Eur J Echocardiogr 2009;10:165-93. [Crossref] [PubMed]
- Nagueh SF, Smiseth OA, Appleton CP, et al. Recommendations for the Evaluation of Left Ventricular Diastolic Function by Echocardiography: An Update from the American Society of Echocardiography and the European Association of Cardiovascular Imaging. J Am Soc Echocardiogr 2016;29:277-314. [Crossref] [PubMed]
- Nagueh SF, Smiseth OA, Appleton CP, et al. Recommendations for the Evaluation of Left Ventricular Diastolic Function by Echocardiography: An Update from the American Society of Echocardiography and the European Association of Cardiovascular Imaging. Eur Heart J Cardiovasc Imaging 2016;17:1321-60. [Crossref] [PubMed]
- Silbiger JJ. Pathophysiology and Echocardiographic Diagnosis of Left Ventricular Diastolic Dysfunction. J Am Soc Echocardiogr 2019;32:216-232.e2. [Crossref] [PubMed]
- Sitges M, Ajmone Marsan N, Cameli M, et al. EACVI survey on the evaluation of left ventricular diastolic function. Eur Heart J Cardiovasc Imaging 2021;22:1098-105. [Crossref] [PubMed]
- National Information Society Agency. Open AI Dataset Project (AI-Hub). Available online: https://aihub.or.kr/
- Jiamsripong P, Honda T, Reuss CS, et al. Three methods for evaluation of left atrial volume. Eur J Echocardiogr 2008;9:351-5. [PubMed]
- Jeon J, Ha S, Yoon YE, et al. Echocardiographic View Classification with Integrated Out-of-Distribution Detection for Enhanced Automatic Echocardiographic Analysis. arXiv 2023. doi:
10.48550 /arXiv.2308.16483v1. - Yoo S, Lee KH, Lee HJ, et al. Seoul National University Bundang Hospital's Electronic System for Total Care. Healthc Inform Res 2012;18:145-52. [Crossref] [PubMed]
- Badano LP, Kolias TJ, Muraru D, et al. Standardization of left atrial, right ventricular, and right atrial deformation imaging using two-dimensional speckle tracking echocardiography: a consensus document of the EACVI/ASE/Industry Task Force to standardize deformation imaging. Eur Heart J Cardiovasc Imaging 2018;19:591-600. [Crossref] [PubMed]
- McLeod K, Šprem J. The Role of Artificial Intelligence in Streamlining Echocardiography Quantification. 2022. Available online: https://www.gehealthcare.com/-/jssmedia/gehc/us/files/products/ultrasound/vivid/redesign/resources/whitepaper_the-role-of-artificial-intelligence__jb20789xx.pdf
- Prater D, Vogel J. Automated Cardiac Motion QuantificationA.I. (aCMQA.I.). 2017. Available online: https://www.documents.philips.com/assets/20220225/bcaa0857d8b24ee5b736ae47008ffc98.pdf
- Chang HJ. HeartAssist: Automatic View Classification and Measurement Tool for Adult Transthoracic Echocardiography. 2022. Available online: https://samsunghealthcare.com/de/knowledge_hub/clinical_library/white_paper
- Fletcher AJ, Lapidaire W, Leeson P. Machine Learning Augmented Echocardiography for Diastolic Function Assessment. Front Cardiovasc Med 2021;8:711611. [Crossref] [PubMed]
- Cho JS, Shrestha S, Kagiyama N, et al. A Network-Based "Phenomics" Approach for Discovering Patient Subtypes From High-Throughput Cardiac Imaging Data. JACC Cardiovasc Imaging 2020;13:1655-70. [Crossref] [PubMed]
- Hubert A, Le Rolle V, Galli E, et al. New expectations for diastolic function assessment in transthoracic echocardiography based on a semi-automated computing of strain-volume loops. Eur Heart J Cardiovasc Imaging 2020;21:1366-71. [Crossref] [PubMed]
- Lancaster MC, Salem Omar AM, Narula S, et al. Phenotypic Clustering of Left Ventricular Diastolic Function Parameters: Patterns and Prognostic Relevance. JACC Cardiovasc Imaging 2019;12:1149-61. [Crossref] [PubMed]
- Salem Omar AM, Shameer K, Narula S, et al. Artificial Intelligence-Based Assessment of Left Ventricular Filling Pressures From 2-Dimensional Cardiac Ultrasound Images. JACC Cardiovasc Imaging 2018;11:509-10. [Crossref] [PubMed]
- Carluccio E, Cameli M, Rossi A, et al. Left Atrial Strain in the Assessment of Diastolic Function in Heart Failure: A Machine Learning Approach. Circ Cardiovasc Imaging 2023;16:e014605. [Crossref] [PubMed]