Protocol and rationale of the Australian multicentre registry for serial cardiac computed tomography angiography (ARISTOCRAT): a prospective observational study of the natural history of pericoronary adipose tissue attenuation and radiomics
Introduction
Coronary artery disease (CAD) continues to be the primary cause of mortality both in Australia and globally (1,2). Majority of the mortality and morbidity burden is driven by acute coronary syndrome (ACS) which is comprise of myocardial infarction (MI) and unstable angina. A substantial residual cardiovascular event risk persists despite improvements in primary and secondary prevention strategies (3). Vascular inflammation is a key factor in the development of atherogenesis and rupture of atherosclerotic plaque leading to ACS (4). Randomised studies demonstrate an ongoing inflammatory risk even when low-density lipoprotein (LDL) cholesterol levels are substantially reduced (5-7). The pivotal CANTOS demonstrated that using the monoclonal antibody canakinumab to target interleukin-1β decreased the rate of recurrent cardiovascular events, thereby confirming the inflammatory theory of atherosclerosis (8). This creates a need for non-invasive evaluation of vascular inflammation. Such assessment will significantly enhance cardiovascular risk stratification, leading to personalised use of inflammation-lowering therapies. However, it remains challenging to identify patients with elevated levels of inflammation who would benefit most from targeted therapy. Circulating inflammatory biomarkers such as high-sensitivity C-reactive protein and pro-inflammatory cytokines are not specific enough to identify coronary inflammation and do not directly corresponds to the process of atherogenesis (9,10). Furthermore, advanced imaging techniques such as sodium-fluoride positron emission tomography have limited practical clinical utility due to its high cost and lack of availability (11).
Coronary computed tomography angiography (CCTA) has been recommended by Australian and international guidelines as the first-line diagnostic test in the investigation of suspected CAD with high sensitivity and negative predictive value (12-15). Recent evidence has shown that inflammation in the coronary arteries can prevent the buildup of lipids in preadipocytes of pericoronary adipose tissue (PCAT) via paracrine signalling, and this effect can be observed on routine CCTA as an increase in computed tomography (CT) attenuation values of PCAT (16). PCAT encased the epicardial coronary artery and directly interacts with the vascular wall in a bidirectional manner. Through paracrine signalling, cytokines from PCAT directly diffuse into the vascular wall, directly influencing its biology and facilitating atherogenesis. Coronary inflammation leads to reduced lipid accumulation and impaired adipocyte differentiation in the adjacent PCAT (16). Consequently, pericoronary fat decreases its lipid content in reaction to inflammatory signals from the nearby coronary artery, serving as an indicator of coronary inflammation. The smaller adipocyte size induced by coronary inflammation is detected as an increase in PCAT attenuation on CCTA. In patients undergoing routine CCTA for suspected CAD, PCAT attenuation has been shown to independently predict plaque progression and cardiac mortality in some studies (17,18). Cholesterol crystal-induced coronary inflammation demonstrated on optical coherence tomography has been shown to be associated with elevated PCAT attenuation (19). The CRISP-CT study showed that high PCAT attenuation [cut-off ≥−70.1 Hounsfield unit (HU)] has additional predictive value in patients with stable CAD for cardiac mortality, above traditional cardiovascular risk factors, CT coronary calcium score, and presence of high-risk plaques (HRPs) (18). However, this study was limited to stable patients and did not evaluate the natural history of PCAT attenuation or the impact of statin therapy on PCAT.
Moreover, the method of determining PCAT attenuation relies solely on the absolute values of voxels, neglecting the intricate spatial interactions among them. Ongoing improvements with CT technology and availability of high-speed computing have paved the way for the emergence of cardiac CT radiomics (20). Radiomics involves the extraction of extensive quantitative features from a given region of interest, generating vast datasets where each anomaly is characterised by hundreds of parameters (20,21). These parameters are then examined through computational methods such as machine learning to uncover novel imaging patterns that hold significant clinical relevance but might remain undetected by the human eye (22). Analyses using CCTA-based radiomics have demonstrated enhanced capability in distinguishing vulnerable plaques beyond the scope of traditional qualitative plaque assessment (23-26).
It is crucial that we thoroughly understand the natural history of PCAT attenuation as CCTA becomes more commonly used as a non-invasive imaging tool for assessing the effect of medical therapies on coronary inflammation and plaque characteristics. The Australian natuRal hISTOry of periCoronary adipose tissue attenuation, RAdiomics and plaque by computed Tomographic angiography (ARISTOCRAT) registry aims to explore the natural history of PCAT attenuation and its association with changes in plaque characteristics in patients undergoing clinically indicated CCTA.
Methods
Study design
The ARISTOCRAT registry is a multi-centre, multi-vendor observational registry comprised of retrospective and prospective arms (Figure 1). The study is independent from industry funding. The study was retrospectively registered with the Australian and New Zealand clinical trials registry (ACTRN12621001018808).
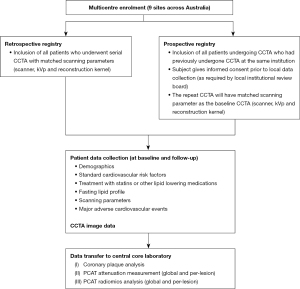
For the prospective registry, eligible patients being referred for clinically indicated CCTA by their treating specialists (e.g., evaluation of chest pain, dyspnoea, or exclusion of significant CAD in setting of newly diagnosed cardiomyopathy) are invited to participate in the study. Eligible patients are identified via periodic screening of the institution’s CCTA database. Eligibility is verified based on the inclusion and exclusion criteria, the study details are explained to the participants, and informed consent is then obtained.
Patients are included for the retrospective arm if they have undergone a least 2 CCTAs with the same CT scanner at the same institution with matched scanning parameters (scanner, tube voltage, and reconstruction kernel) on prior to 31st of December 2020. Clinical data (demographics, cardiovascular risk factors, fasting lipid profiles and CT scanning parameters) are to be collected from medical records. Patient consents are not required for the transfer of deidentified data.
Study population
All eligible patients will be enrolled from 9 large tertiary hospitals across Australia participating in the registry (Figure 2).
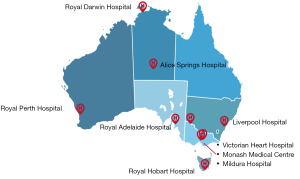
Inclusion criteria
- Underwent 2 or more CCTA imaging at the same institution, using the same CT scanner, and the same scanning tube voltage.
- At least 12 months interval between baseline and follow-up CCTAs.
- Male or female ≥18 years of age at screening.
Exclusion criteria
- Clinical or laboratory data not available within 3 months from the baseline or follow-up CCTA.
- Inadequate image quality as deemed by the core laboratory.
- Heart failure (New York Heart Association class IV) or left ventricular ejection fraction ≤35%.
- Inability to provide informed consent.
- Prior coronary artery bypass graft surgery or percutaneous revascularisation.
- Atrial fibrillation.
- Dialysis or estimated glomerular filtration rate (eGFR) <30 mL/min/1.73 m2.
Patient data collection
Patient demographic data and standard cardiovascular risk factors will be assessed from patient questionnaires at the time of CCTA scans and by review of electronic medical records. Total cholesterol, high-density lipoprotein (HDL) and LDL cholesterol, and triglycerides levels are measured either at the time of scanning or within 3 months of the CCTA studies. Diabetes is defined by the use of oral hypoglycaemic agents or insulin or fasting serum glucose ≥7 mmol/L. Hypertension is defined as systolic blood pressure ≥140 mmHg and/or diastolic blood pressure ≥90 mmHg or patients treated with antihypertensive medication. Current smoking is defined as use of cigarettes within the past 3 months, while ex-smoking are individuals who quit smoking ≥3 months prior to CCTA. Dyslipidaemia is defined as total cholesterol ≥5.5 mmol/L, LDL ≥3.5 mmol/L, HDL ≤1.0 mmol/L, triglycerides ≥2.0 mmol/L, or treatment with statins. The name and dosage of statin and all other lipid lower medication taken at the time of each CCTAs will be recorded. To estimate the pre-test probability of CAD for all patients, the pooled cohort atherosclerotic cardiovascular disease (ASCVD) risk score will be calculated (27).
Patient data will be entered into an online, centralised, secure, and encrypted databases in a deidentified format (Table 1) (28). Once enrolled, all patients are assigned a unique study identifier, which is used to link all imaging data and demographic information.
Table 1
Age (years), mean ± SD |
Male gender, n (%) |
Time period between baseline and follow-up CCTA (years), mean ± SD |
Diabetes mellitus, n (%) |
Dyslipidaemia, n (%) |
Current smoker, n (%) |
Ex-smoker, n (%) |
Family history of CAD, n (%) |
Obesity, n (%) |
Baseline and follow-up CCTA |
Tube voltage (kVp), mean ± SD |
Tube current (mA), mean ± SD |
Weight (kg), mean ± SD |
Height (m), mean ± SD |
CAD-RADS |
Segment Involvement Score, mean ± SD |
Segment Stenosis Score, mean ± SD |
Total cholesterol (mmol/L), mean ± SD |
Triglyceride (mmol/L), mean ± SD |
LDL (mmol/L), mean ± SD |
HDL (mmol/L), mean ± SD |
Statin name and dosage (mg) |
Other lipid lowering medication name and dosage (mg) |
SD, standard deviation; CCTA, coronary computed tomography angiography; CAD, coronary artery disease; kVp, kilovoltage peak; mA, milliampere; CAD-RADS, Coronary Artery Disease-Reporting and Data System; LDL, low-density lipoprotein; HDL, high-density lipoprotein.
CCTA data acquisition and repository
All CCTA testing, image acquisition, and image postprocessing in the ARISTOCRAT registry is conducted in strict compliance with the guidelines of the Society of Cardiovascular Computed Tomography (29,30). All CCTA scans are performed using a scanner with ≥64-detector rows. However, no restrictions are placed regarding the type of CT scanner or type of iodinated contrast. Data regarding the CCTA scan parameters including tube current (milliampere; mA), peak tube voltage (kVp), and dose-length product (DLP) are recorded.
All de-identified CCTA images will be sent to the core laboratory (Victorian Heart Institute, Monash University) in thin digital imaging and communication in medicine (DICOM) format and stored on a secured web-based server. The core laboratory will perform analyses for CCTA image quality, and the core laboratory interpretation will be used for post hoc analysis.
Image quality between the serial CTs will be assessed by measuring the signal-to-noise ratio (SNR) and the contrast-to-noise ratio (CNR). The SNR is calculated by dividing the average coronary luminal CT attenuation in HU adjacent to the plaque in a healthy segment by the standard deviation of the CT attenuation in the aorta. This measurement is taken within an area of interest that is at least 2 cm2, located at the level of the left main trunk. The CNR is determined by subtracting the perivascular HU at the plaque site from the mean luminal HU, and then dividing this difference by the standard deviation of the aortic HU.
Quantitative coronary plaque analysis
CCTA will be analysed as per the 18-segment model outlined by the Society of Cardiovascular Computed Tomography on a dedicated workstation using the standard anatomical arterial region (30). The presence of coronary atherosclerotic lesion is defined as any tissue structures larger than 1 mm2 located either within the coronary artery lumen or immediately adjacent to. These lesions are distinguishable from the surrounding pericardial tissue, epicardial fat, or the vessel lumen itself. Each coronary segment >1.5 mm in diameter will be analysed for the presence of plaque and diameter stenosis will be visualised graded and categorised as: no stenosis (0%), minimal stenosis (<25%), mild (25–49%), moderate (50–69%), severe (70–99%) and, occluded (100%).
Quantitative plaque analysis will be performed with semiautomated software (Autoplaque v2.5, Cedars-Sinai Medical Center, Los Angeles, CA, USA) with appropriate manual adjustment of the vessel lumen and wall contouring using multiplanar views. A circular region of interest is placed in the ascending aorta to define normal blood pool. The absolute plaque volumes (in mm3) were measured for the following plaque subtypes: total plaque, calcified plaque, non-calcified plaque (NCP), and low-attenuation plaque (defined by an attenuation of <30 HU) (31,32). Plaque burden (in %) is calculated by dividing the volume of plaque to the vessel volume of the region assessed (plaque volume/analysed vessel volume × 100%). The remodelling index is determined by comparing the maximum vessel area to the area at the proximal normal reference point, expressed as a ratio. To obtain the maximum diameter stenosis, we divide the narrowest diameter of the lumen by the average of two normal non-diseased reference points (33). Lesion length (in mm), is the length of the diseased vessel as determined by the Autoplaque software.
PCAT attenuation measurement
Following lesion-level quantitative plaque analysis, CT measurement of PCAT attenuation will be fully automated using Autoplaque at both per-patient and per-plaque lesion level (Figure 3). PCAT attenuation surrounding the proximal right coronary artery (10 mm distal to the ostium for a length of 40 mm), 40 mm proximal segments of the left anterior descending and the left circumflex coronary arteries will be used as a standardised patient-level measurement (16,34). PCAT is sampled in three-dimensional layers which expands radially from the outer vessel wall in 1 mm increments. All voxels with attenuation between −190 and −30 HU will be defined as adipose tissue. Subsequently, PCAT CT attenuation is determined by averaging the CT attenuation (in HU) of the adipose tissue within the defined volume of interest (16,18). Per-lesion PCAT attenuation analysis will be limited to the length of the plaque. The annualised rate of PCAT attenuation changes will be calculated to account for the different time intervals between the CCTAs among patients.
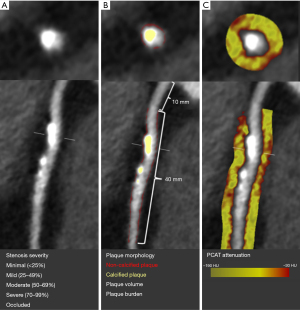
PCAT radiomics analysis
The PCAT volume data exported will be analysed using the radiomics image analysis (RIA) open-source software package within the R programming environment (35). Our analysis will incorporate four distinct categories of radiomic features. First-order statistics focuses on analysing the distribution of HU values without considering their spatial arrangement. Grey level co-occurrence matrices (GLCM) track the occurrence of adjacent voxels with similar values within a lesion, whereas grey level run length matrices (GLRLM) measure the frequency of consecutive voxels of the same value (36,37). For analysis, the images will be segmented into 8, 16, and 32 bins of equal size based on the range of HU values, creating three replicas of the image (Figure 4). All GLCM and GLRLM metrics are computed across all three binning types. Geometry-based statistics are calculated both on the original image and on each discretised component.
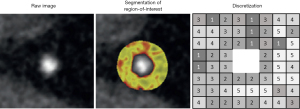
Previously, we calculated a total of 1,103 radiomic parameters for each PCAT segmentation (38). This included 44 first-order parameters, 342 statistics derived from GLCM, 33 statistics from GLRLM, and 684 geometrical parameters (24,25).
Sample size calculation
In our preliminary retrospective analysis of patients commenced on statin after their baseline CCTA, we have demonstrated an average reduction of PCAT attenuation of 3.1% per year (39). This finding is consistent with another study from Dai et al. (40). Assuming the PCAT attenuation reduction of 3% per year, enrolment of 354 patients commenced on statin after their baseline CCTA and another 354 patients who remains statin naïve at both CCTA timepoints would achieve 80% power at an α level of 0.05.
Statistical methods
Parametric variables will be reported as mean ± standard deviation, non-parametric variables will be reported as median with interquartile range (IQR), and categorical variables will be reported as percentages. For parametric data, group comparisons at baseline will utilize the t-test, while longitudinal changes will be analysed using the paired t-test. Non-parametric variables will be compared using the Wilcoxon signed-rank test for paired data and the Mann-Whitney test for independent groups. Categorical variables will be analyzed between groups using the Pearson χ2 test. To explore the effect of statin therapy on change in PCAT attenuation, a two-factor mixed analysis of variance will be conducted. This will include a treatment × time point interaction, after confirming the normality of the dependent variable with the Shapiro-Wilk test. To examine the association of changes in PCAT attenuation with changes in plaque volume/burden, we will perform multivariable linear regression, adjusting for confounding variables. All statistical analyses will be carried out on an individual patient basis using IBM SPSS Statistics for Windows (version 26.0, Armonk, NY, USA). P values <0.05 will be considered statistically significant.
For radiomic analysis, we will determine the set of unique radiomic features that explain 99.5% of the variability in our dataset by employing principal component analysis. To adjust for multiple comparisons and determine the threshold for statistical significance, we will apply the Bonferroni correction when comparing the two groups. The comparison of radiomic parameters across scans will be carried out using the symmetry permutation test available in the coin R package (41). Significant radiomic features will undergo linear regression analysis, with the R2 value serving as a measure of distance for hierarchical clustering between each pair of features. The optimal number of clusters will be identified using the elbow method, which considers the total within-cluster sum of squares. For every cluster, the radiomic feature demonstrating the lowest P value will be chosen as the key biomarker for that cluster.
Endpoints
The primary objective of the ARISTOCRAT registry is to delineate the natural history of PCAT attenuation and its relationship with plaque characteristics as observed through CCTA. In particular, our focus will be on examining the temporal changes in per-patient and per-lesion level PCAT attenuation.
The secondary objectives of the ARISTOCRAT registry will be to evaluate: (I) changes in PCAT attenuation and plaque characteristics in relation to major adverse cardiovascular events (MACEs); (II) impact of statin therapy on PCAT attenuation and plaque characteristics; and (III) changes in PCAT attenuation and plaque characteristics in specific subgroups according to sex and cardiovascular risk factors. MACEs will be defined by a composite of cardiac death and ACS (MI and unstable angina). Cardiac death refers to any fatality resulting from an acute MI. ACS is determined based on the criteria set forth in the fourth universal definition of MI and the classifications of angina severity by the Canadian Cardiovascular Society. This includes instances where patients exhibit classic symptoms of chest pain along with an increase in troponin levels, with or without changes in the electrocardiogram (ECG). Should there be no elevation in troponin, chest pain classified as Canadian Cardiovascular Society class 3 or 4 will also be deemed significant. This will be performed through examination of the Australian Death Registry, Victoria Cardiac Outcomes Registry (VCOR) registry as well as hospital electronic medical records.
Discussion
PCAT attenuation has been recognised as a non-invasive imaging biomarker that effectively reflects regional atherosclerotic burden and the extent of local inflammation (17,18,42). Numerous observational studies have investigated the ability of PCAT attenuation to monitor changes in vascular inflammation, including responses to treatment interventions. A prospective study involving 134 patients with psoriasis who underwent serial CCTA, has demonstrated a notable reduction in PCAT attenuation in those treated with biologic therapy after one year. Conversely, no significant change was detected in patients who did not undergo biologic treatment (43). A retrospective study of 108 statin-naïve patients who were commenced on statin treatment, has demonstrated significant reduction in lesion-specific PCAT attenuation for non-calcified and mixed plaques (40). To date, there are no data regarding the natural history of PCAT attenuation in patients not treated with statin or anti-inflammatory therapy.
The long-term natural history of PCAT in the general cohorts of patients with suspected CAD, most of whom undergo CCTA and are found to have non-obstructive CAD, remained unexplored (44). Given PCAT’s universality in human anatomy, it is important to examine the relationship between PCAT changes with atherosclerotic plaque progression in the context of cardiovascular risk factors. Such understanding will also be vital with interpreting findings from the present and future studies using PCAT attenuation as the dynamic non-invasive marker of coronary inflammation in response to therapeutic interventions. This registry will be the first to use serial CCTA to quantitively measure PCAT inflammation in populations with low-to-moderate risk of CAD. The registry will utilise its extensive database, collected from geographically diverse regions and is representative of individuals undergoing clinically indicated CCTA.
ARISTOCRAT will also be the first registry to integrate CCTA radiomics and machine learning to uncover novel imaging patterns of significant clinical relevance. This incorporates texture analysis to model the spatial distribution of voxel gray-level intensities and applies higher-order statistics to provide a measure of heterogeneity (21). Furthermore, the registry will analysis geometric radiomic parameters, facilitating the measurement of the size and shape of three-dimensional structures within the CCTA imaging dataset. PCAT radiomic analysis has been shown to improved prediction of major adverse cardiovascular events when added to traditional risk stratification that included CVD risk factors, coronary calcium score, coronary stenosis and CT HRP features (45).
Therefore, we have designed the ARISTOCRAT registry to be a prospective multicentre observational registry which aims to describe and characterise the natural history, and the determinants of PCAT inflammation. The anticipated results from ARISTOCRAT are expected to help personalise clinical decision making on the appropriate management of patients by using PCAT characteristics as a surrogate marker of atherosclerotic progression.
Study limitations
In this registry, enrolment will be limited to patients who have undergone at least two CCTA scans. According to existing guidelines and standard clinical practice, individuals identified with severe CAD based on their initial CCTA are typically recommended for invasive coronary angiography, potentially leading to revascularization (14,46). Conversely, for patients whose initial CCTA shows normal coronary anatomy, follow-up CCTA is generally not advised within such a brief timeframe (47). Consequently, given the nature of our study design, we expect that most patients with either severe disease or normal coronary anatomy based on their baseline CCTA will not be included in the registry.
Trial registration
This study has been prospectively registered with the Australia and New Zealand Clinical Trials Registry (ACTRN12621001018808).
Acknowledgments
Funding: This study was supported by
Footnote
Peer Review File: Available at https://cdt.amegroups.com/article/view/10.21037/cdt-23-392/prf
Conflicts of Interest: All authors have completed the ICMJE uniform disclosure form (available at https://cdt.amegroups.com/article/view/10.21037/cdt-23-392/coif). P.J.P. serves as an unpaid editorial board member of Cardiovascular Diagnosis and Therapy from July 2022 to June 2024. S.J.N. as an unpaid editorial board member of Cardiovascular Diagnosis and Therapy from September 2023 to August 2025. D.T.L.W. serves as an unpaid editorial board member of Cardiovascular Diagnosis and Therapy from February 2021 to January 2023. K.C. was supported by the National Health and Medical Research Council postgraduate scholarship (No. APP2002573), which covers a portion of the research-related expenses and stipend to help with living expenses, research-related travel costs and other expenses associated with research project. S.J.N. received consulting fees from Amgen, Akcea, AstraZeneca, Boehringer Ingelheim, CSL Behring, Eli Lilly, Esperion, Kowa, Merck, Takeda, Pfizer, Sanofi- Regeneron, Vaxxinity, CSL Sequiris, and Novo Nordisk; received grants from AstraZeneca, Amgen, Anthera, CSL Behring, Cerenis, Cyclarity, Eli Lilly, Esperion, Resverlogix, New Amsterdam Pharma, Novartis, InfraReDx and Sanofi-Regeneron. D.T.L.W. received honoraria for lectures from Eli-Lilly, Pfizer and Boehringer. The other authors have no conflicts of interest to declare.
Ethical Statement: The authors are accountable for all aspects of the work in ensuring that questions related to the accuracy or integrity of any part of the work are appropriately investigated and resolved. All procedures in this study will be conducted in accordance with the Declaration of Helsinki (as revised in 2013), International Conference on Harmonization, Good Clinical Practice guidelines, and applicable regulatory requirements. The final protocol was reviewed and approved by Ethics Committees and Institutional Review Boards at each study site and by the Monash Health Human Research Ethics Committee, Melbourne, Australia (HREC/75307/MonH/2021). All participating hospitals/centres were informed and agreed on the study. Informed consent is obtained from the participants in the prospective arm prior to enrolment, and the data and samples are deidentified and managed entirely anonymously.
Open Access Statement: This is an Open Access article distributed in accordance with the Creative Commons Attribution-NonCommercial-NoDerivs 4.0 International License (CC BY-NC-ND 4.0), which permits the non-commercial replication and distribution of the article with the strict proviso that no changes or edits are made and the original work is properly cited (including links to both the formal publication through the relevant DOI and the license). See: https://creativecommons.org/licenses/by-nc-nd/4.0/.
References
- Australian Bureau of Statistics. Causes of Death, Australia 2021 [updated 29 September 2021]. Available online: https://www.abs.gov.au/statistics/health/causes-death/causes-death-australia/latest-release#australia-s-leading-causes-of-death-2020
- Roth GA, Mensah GA, Johnson CO, et al. Global Burden of Cardiovascular Diseases and Risk Factors, 1990-2019: Update From the GBD 2019 Study. J Am Coll Cardiol 2020;76:2982-3021. [Crossref] [PubMed]
- Cholesterol Treatment Trialists’ (CTT) Collaboration. Efficacy and safety of more intensive lowering of LDL cholesterol: a meta-analysis of data from 170,000 participants in 26 randomised trials. Lancet 2010;376:1670-81. [Crossref] [PubMed]
- Libby P, Ridker PM, Maseri A. Inflammation and atherosclerosis. Circulation 2002;105:1135-43. [Crossref] [PubMed]
- Ridker PM, Cannon CP, Morrow D, et al. C-reactive protein levels and outcomes after statin therapy. N Engl J Med 2005;352:20-8. [Crossref] [PubMed]
- Bohula EA, Giugliano RP, Cannon CP, et al. Achievement of dual low-density lipoprotein cholesterol and high-sensitivity C-reactive protein targets more frequent with the addition of ezetimibe to simvastatin and associated with better outcomes in IMPROVE-IT. Circulation 2015;132:1224-33. [Crossref] [PubMed]
- Lu Y, Zhou S, Dreyer RP, et al. Sex Differences in Inflammatory Markers and Health Status Among Young Adults With Acute Myocardial Infarction: Results From the VIRGO (Variation in Recovery: Role of Gender on Outcomes of Young Acute Myocardial Infarction Patients) Study. Circ Cardiovasc Qual Outcomes 2017;10:e003470. [Crossref] [PubMed]
- Ridker PM, Everett BM, Thuren T, et al. Antiinflammatory Therapy with Canakinumab for Atherosclerotic Disease. N Engl J Med 2017;377:1119-31. [Crossref] [PubMed]
- Weintraub WS, Harrison DG. C-reactive protein, inflammation and atherosclerosis: do we really understand it yet? Eur Heart J 2000;21:958-60. [Crossref] [PubMed]
- Lee R, Margaritis M, Channon KM, et al. Evaluating oxidative stress in human cardiovascular disease: methodological aspects and considerations. Curr Med Chem 2012;19:2504-20. [Crossref] [PubMed]
- Camici PG, Rimoldi OE, Gaemperli O, et al. Non-invasive anatomic and functional imaging of vascular inflammation and unstable plaque. Eur Heart J 2012;33:1309-17. [Crossref] [PubMed]
- Moss AJ, Williams MC, Newby DE, et al. The Updated NICE Guidelines: Cardiac CT as the First-Line Test for Coronary Artery Disease. Curr Cardiovasc Imaging Rep 2017;10:15. [Crossref] [PubMed]
- Writing Committee Members. 2021 AHA/ACC/ASE/CHEST/SAEM/SCCT/SCMR Guideline for the Evaluation and Diagnosis of Chest Pain: A Report of the American College of Cardiology/American Heart Association Joint Committee on Clinical Practice Guidelines. J Am Coll Cardiol 2021;78:e187-285. [Crossref] [PubMed]
- Knuuti J, Wijns W, Saraste A, et al. 2019 ESC Guidelines for the diagnosis and management of chronic coronary syndromes. Eur Heart J 2020;41:407-77. [Crossref] [PubMed]
- Parsonage WA, Cullen L, Brieger D, et al. CSANZ Position Statement on the Evaluation of Patients Presenting With Suspected Acute Coronary Syndromes During the COVID-19 Pandemic. Heart Lung Circ 2020;29:e105-10. [Crossref] [PubMed]
- Antonopoulos AS, Sanna F, Sabharwal N, et al. Detecting human coronary inflammation by imaging perivascular fat. Sci Transl Med 2017;9:eaal2658. [Crossref] [PubMed]
- Goeller M, Tamarappoo BK, Kwan AC, et al. Relationship between changes in pericoronary adipose tissue attenuation and coronary plaque burden quantified from coronary computed tomography angiography. Eur Heart J Cardiovasc Imaging 2019;20:636-43. [Crossref] [PubMed]
- Goeller M, Achenbach S, Cadet S, et al. Pericoronary Adipose Tissue Computed Tomography Attenuation and High-Risk Plaque Characteristics in Acute Coronary Syndrome Compared With Stable Coronary Artery Disease. JAMA Cardiol 2018;3:858-63. [Crossref] [PubMed]
- Lin A, Nerlekar N, Munnur RK, et al. Cholesterol crystal-induced coronary inflammation: Insights from optical coherence tomography and pericoronary adipose tissue computed tomography attenuation. J Cardiovasc Comput Tomogr 2020;14:277-8. [Crossref] [PubMed]
- Cheng K, Lin A, Yuvaraj J, et al. Cardiac Computed Tomography Radiomics for the Non-Invasive Assessment of Coronary Inflammation. Cells 2021;10:879. [Crossref] [PubMed]
- Kolossváry M, Kellermayer M, Merkely B, et al. Cardiac Computed Tomography Radiomics: A Comprehensive Review on Radiomic Techniques. J Thorac Imaging 2018;33:26-34. [Crossref] [PubMed]
- Gillies RJ, Kinahan PE, Hricak H. Radiomics: Images Are More than Pictures, They Are Data. Radiology 2016;278:563-77. [Crossref] [PubMed]
- Kolossváry M, Karády J, Szilveszter B, et al. Radiomic Features Are Superior to Conventional Quantitative Computed Tomographic Metrics to Identify Coronary Plaques With Napkin-Ring Sign. Circ Cardiovasc Imaging 2017;10:e006843. [Crossref] [PubMed]
- Kolossváry M, Karády J, Kikuchi Y, et al. Radiomics versus Visual and Histogram-based Assessment to Identify Atheromatous Lesions at Coronary CT Angiography: An ex Vivo Study. Radiology 2019;293:89-96. [Crossref] [PubMed]
- Kolossváry M, Park J, Bang JI, et al. Identification of invasive and radionuclide imaging markers of coronary plaque vulnerability using radiomic analysis of coronary computed tomography angiography. Eur Heart J Cardiovasc Imaging 2019;20:1250-8. [Crossref] [PubMed]
- Kolossváry M, Gerstenblith G, Bluemke DA, et al. Contribution of Risk Factors to the Development of Coronary Atherosclerosis as Confirmed via Coronary CT Angiography: A Longitudinal Radiomics-based Study. Radiology 2021;299:97-106. [Crossref] [PubMed]
- Goff DC Jr, Lloyd-Jones DM, Bennett G, et al. 2013 ACC/AHA guideline on the assessment of cardiovascular risk: a report of the American College of Cardiology/American Heart Association Task Force on Practice Guidelines. Circulation 2014;129:S49-73. [Crossref] [PubMed]
- Harris PA, Taylor R, Thielke R, et al. Research electronic data capture (REDCap)--a metadata-driven methodology and workflow process for providing translational research informatics support. J Biomed Inform 2009;42:377-81. [Crossref] [PubMed]
- Abbara S, Blanke P, Maroules CD, et al. SCCT guidelines for the performance and acquisition of coronary computed tomographic angiography: A report of the society of Cardiovascular Computed Tomography Guidelines Committee: Endorsed by the North American Society for Cardiovascular Imaging (NASCI). J Cardiovasc Comput Tomogr 2016;10:435-49. [Crossref] [PubMed]
- Leipsic J, Abbara S, Achenbach S, et al. SCCT guidelines for the interpretation and reporting of coronary CT angiography: a report of the Society of Cardiovascular Computed Tomography Guidelines Committee. J Cardiovasc Comput Tomogr 2014;8:342-58. [Crossref] [PubMed]
- Dey D, Schepis T, Marwan M, et al. Automated three-dimensional quantification of noncalcified coronary plaque from coronary CT angiography: comparison with intravascular US. Radiology 2010;257:516-22. [Crossref] [PubMed]
- Motoyama S, Ito H, Sarai M, et al. Plaque Characterization by Coronary Computed Tomography Angiography and the Likelihood of Acute Coronary Events in Mid-Term Follow-Up. J Am Coll Cardiol 2015;66:337-46. [Crossref] [PubMed]
- Achenbach S, Ropers D, Hoffmann U, et al. Assessment of coronary remodeling in stenotic and nonstenotic coronary atherosclerotic lesions by multidetector spiral computed tomography. J Am Coll Cardiol 2004;43:842-7. [Crossref] [PubMed]
- Oikonomou EK, Marwan M, Desai MY, et al. Non-invasive detection of coronary inflammation using computed tomography and prediction of residual cardiovascular risk (the CRISP CT study): a post-hoc analysis of prospective outcome data. Lancet 2018;392:929-39. [Crossref] [PubMed]
- Kolossvary M. RIA: Radiomics Image Analysis Toolbox for Grayscale Images. 2017. Available online: https://cran.r-project.org/web/packages/RIA/index.html
- Haralick RM, Shanmugam K, Dinstein I. Textural Features for Image Classification. IEEE Transactions on Systems, Man, and Cybernetics 1973;SMC-3:610-21. [Crossref]
- Galloway MM. Texture analysis using gray level run lengths. Computer Graphics and Image Processing 1975;4:172-9. [Crossref]
- Lin A, Kolossváry M, Yuvaraj J, et al. Myocardial Infarction Associates With a Distinct Pericoronary Adipose Tissue Radiomic Phenotype: A Prospective Case-Control Study. JACC Cardiovasc Imaging 2020;13:2371-83. [Crossref] [PubMed]
- Cheng K, Hii R, Lim E, et al. Effect of Statin Therapy on Coronary Inflammation Assessed by Pericoronary Adipose Tissue Computed Tomography Attenuation: Insights From the Multicentre Observational Study. Heart, Lung and Circulation 2023;32:S116-7. [Crossref]
- Dai X, Yu L, Lu Z, et al. Serial change of perivascular fat attenuation index after statin treatment: Insights from a coronary CT angiography follow-up study. Int J Cardiol 2020;319:144-9. [Crossref] [PubMed]
- Hothorn T, Hornik K, van de Wiel MA, et al. Implementing a Class of Permutation Tests: The coin Package. Journal of Statistical Software 2008;28:1-23. [Crossref]
- Marwan M, Hell M, Schuhbäck A, et al. CT Attenuation of Pericoronary Adipose Tissue in Normal Versus Atherosclerotic Coronary Segments as Defined by Intravascular Ultrasound. J Comput Assist Tomogr 2017;41:762-7. [Crossref] [PubMed]
- Elnabawi YA, Oikonomou EK, Dey AK, et al. Association of Biologic Therapy With Coronary Inflammation in Patients With Psoriasis as Assessed by Perivascular Fat Attenuation Index. JAMA Cardiol 2019;4:885-91. [Crossref] [PubMed]
- Wang ZJ, Zhang LL, Elmariah S, et al. Prevalence and Prognosis of Nonobstructive Coronary Artery Disease in Patients Undergoing Coronary Angiography or Coronary Computed Tomography Angiography: A Meta-Analysis. Mayo Clin Proc 2017;92:329-46. [Crossref] [PubMed]
- Oikonomou EK, Williams MC, Kotanidis CP, et al. A novel machine learning-derived radiotranscriptomic signature of perivascular fat improves cardiac risk prediction using coronary CT angiography. Eur Heart J 2019;40:3529-43. [Crossref] [PubMed]
- Fihn SD, Blankenship JC, Alexander KP, et al. 2014 ACC/AHA/AATS/PCNA/SCAI/STS focused update of the guideline for the diagnosis and management of patients with stable ischemic heart disease: a report of the American College of Cardiology/American Heart Association Task Force on Practice Guidelines, and the American Association for Thoracic Surgery, Preventive Cardiovascular Nurses Association, Society for Cardiovascular Angiography and Interventions, and Society of Thoracic Surgeons. J Am Coll Cardiol 2014;64:1929-49. [Crossref] [PubMed]
- Min JK, Dunning A, Lin FY, et al. Age- and sex-related differences in all-cause mortality risk based on coronary computed tomography angiography findings results from the International Multicenter CONFIRM (Coronary CT Angiography Evaluation for Clinical Outcomes: An International Multicenter Registry) of 23,854 patients without known coronary artery disease. J Am Coll Cardiol 2011;58:849-60. [Crossref] [PubMed]