Machine learning prediction of no reflow in patients with ST-segment elevation myocardial infarction undergoing primary percutaneous coronary intervention
Highlight box
Key findings
• A logistic regression (LR) model was successfully developed through machine learning (ML) to forecast no-reflow (NRF) phenomena in ST-segment elevation myocardial infarction (STEMI) patients undergoing primary percutaneous coronary intervention (pPCI).
• The logistic regression model encompassed seven meaningful features.
What is known and what is new?
• While previous research has explored risk factors for NRF, few studies have employed ML approaches.
• Our study utilized various ML algorithms for data cleaning, feature selection, and model construction, conducting a comprehensive evaluation of model performance.
• Thrombolysis in myocardial infarction flow after initial balloon dilation (TFAID) was the most impactful predictor in all models.
• Serum potassium levels were identified as a primary predictor, consistently ranking within the top three variables in all models.
What is the implication, and what should change now?
• A web-based calculator, crafted from the LR model, facilitates clinicians in assessing the risk of NRF in STEMI patients undergoing pPCI and aids in the timely adjustment of intraoperative intervention strategies.
Introduction
Acute ST-segment elevation myocardial infarction (STEMI) ranks as one of the leading killers globally, and primary percutaneous coronary intervention (pPCI) has been established as the most effective treatment modality, which evidently shrinks the area compromised by the myocardial infarction and improves the long-term prognosis of patients (1,2). Unfortunately, approximately 10–30% of patients undergoing PCI have been confirmed to suffer from no-reflow (NRF) (2,3). NRF refers to a condition where, despite the restoration of blood flow, the myocardial tissue in the previously occluded coronary artery region exhibits reduced or absent reperfusion, which can lead to exacerbation of ischemic myocardial injury and increase the risk of adverse cardiovascular events (3). Post NRF, conventional medications often fail to reach the distal vasculature, and currently there is a paucity of corresponding effective interventions (4). Therefore, emphasis should be laid on the early prevention of NRF and selection of intraoperative strategies.
In spite of the identified factors such as age, history of diabetes, smoking history, total ischemia time, Killip classification, inflammatory markers, stent length and stent diameter as potential predictors of NRF (3,5,6), these studies widely utilized traditional statistical methods, like logistic regression (LR) and meta-analysis, which may have limitations in handling large complex datasets, potentially overlooking crucial variables. With the advancement of machine learning (ML) technology, mounting medical researchers are committed to exploring the application of ML in the medical field, yet its use in NRF research attracts relatively insufficient attention. A few studies have demonstrated the potential of ML methods such as random forest (RF) and nomogram-based LR models in predicting NRF in STEMI patients during pPCI (7,8). However, challenges in obtaining timely data before pPCI, ignoring intraoperative interventions and handling imbalanced data should not be neglected.
On this basis, our study aims to analyze preoperative historical data and laboratory indices of STEMI patients, along with the imaging features of coronary angiography and intraoperative treatment strategies based on various ML methods. This study is devoted to identifying risk and protective factors for NRF during pPCI, dissecting the relationships between these factors and NRF, and establishing a rapid, robust predictive model. The model is designed to guide pre- and intra-operative decision-making, contributing to the potential reduction of NRF incidence and thereby improving patients’ outcomes in future. We present this article in accordance with the TRIPOD reporting checklist (available at https://cdt.amegroups.com/article/view/10.21037/cdt-24-83/rc) (9).
Methods
Study participants
A retrospective analysis was conducted on 321 STEMI patients who underwent pPCI at The Second Affiliated Hospital of Anhui Medical University between January 2022 and May 2023. The pPCI procedures conformed strictly to the latest 2023 European ACS treatment guidelines (1). Inclusion criteria were as follows: (I) patients diagnosed with STEMI based on clinical symptoms, electrocardiographic changes, and elevated cardiac biomarkers; (II) patients undergoing primary pPCI within 48 hours of symptom onset. Exclusion criteria were as follows: (I) onset of symptoms exceeding 48 hours; (II) poor angiographic image quality precluding accurate analysis; (III) acute occlusion of the left main coronary artery; (IV) inability to achieve revascularization during the procedure; (V) multi-vessel treatment during the procedure; (VI) occlusions of branches other than left anterior descending artery (LAD), left circumflex artery (LCX) and right coronary artery (RCA), including bridge vessels. All eligible patients during the study period were consecutively included to avoid selection bias. After screening, a total of 261 patients were enrolled in the study and categorized based on the occurrence of NRF into a normal flow group and an NRF group. Subsequently, patients were randomly divided into a training set (183 patients) and an internal validation set (78 patients) in a 7:3 ratio. The flowchart outlining patient enrollment and study design is shown in Figure 1. The study was conducted in accordance with the Declaration of Helsinki (as revised in 2013). The study was approved by the institutional review board of The Second Affiliated Hospital of Anhui Medical University (YX2022-001) and individual consent for this retrospective analysis was waived.
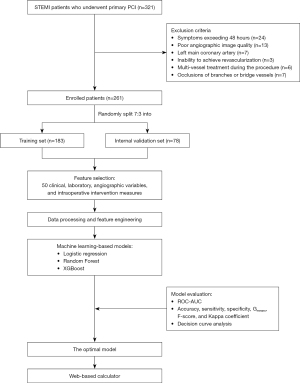
Definitions and predictive variables
The criteria for STEMI are defined as follows: (I) persistent typical chest pain over 20 minutes, and unresponsive to nitroglycerin; (II) new ST-segment elevation at the J-point in at least two contiguous leads: ≥2.5 mm in men under 40 years old, ≥2 mm in men 40 years old or older, or ≥1.5 mm in women of any age in leads V2–V3, and/or ≥1 mm in other leads, provided there is no left ventricular hypertrophy or left bundle branch block; (III) elevated cardiac biomarkers indicating myocardial injury (1). NRF is identified as a predictive variable, defined as post-PCI thrombolysis and thrombolysis in myocardial infarction (TIMI) flow grade ≤2 in the infarct-related artery, in the absence of dissection, spasm, apparent thrombus, or residual stenosis (7).
pPCI
The pPCI was uniformly performed in each patient using standard techniques. All primary operators were interventional cardiologists with over 10 years of experience in coronary interventions and held certification in interventional procedures. The radial artery (right or left) was the preferred access route, with the femoral artery used only if radial access was unsuccessful bilaterally. All intraoperative strategies were selected and implemented by the primary operators based on coronary angiography findings, their clinical experience, and the patient’s condition.
Feature collection and definition
Initially, 50 features were incorporated (Table 1). Killip classification was divided into Killip class I and Killip class II–IV. Off-hours were defined as pPCI procedures performed between 6:00 PM and 7:59 AM daily, including weekends and holidays. A diffuse lesion signified a total lesion length ≥30 mm in a single vessel (10). Prophylactic tirofiban referred to the intracoronary administration of tirofiban prior to stent implantation or in the event of NRF. Based on the TIMI thrombus grading (TTG) criteria (3,4), thrombus grades were classified as TTG <4 and TTG ≥4. Thrombolysis in myocardial infarction flow after initial balloon dilation (TFAID) was delineated as the TIMI flow grade quantified in the target vessel subsequent to the initial balloon dilation, which may encompass either a single or multiple inflation(s). This parameter was stratified into two distinct categories: TFAID 0–2, denoting suboptimal perfusion, and TFAID 3, indicating optimal perfusion.
Table 1
Variable | Assignment | Missing value | All (n=261) | Normal flow (n=212) | No reflow (n=49) | P |
---|---|---|---|---|---|---|
Demographic | ||||||
Gender (male) | gender | 0 | 212 (81.226) | 174 (82.075) | 38 (77.551) | 0.47 |
Smoke (yes) | smoke | 0 | 149 (57.088) | 119 (56.132) | 30 (61.224) | 0.52 |
Age (years) | age | 0 | 59.000 [50.000–70.000] | 58.500 [50.000–69.000] | 60.000 [52.000–72.000] | 0.21 |
Symptom to wire (min) | S2W | 0 | 278.000 [177.000–574.000] | 273.000 [175.000–575.500] | 316.000 [189.000–538.000] | 0.56 |
Off-hours (yes) | Off_hours | 0 | 128 (49.042) | 98 (46.226) | 30 (61.224) | 0.06 |
Tests | ||||||
Neutrophil (103/μL) | Neu | 1 (0.38) | 7.890 [6.000–10.598] | 7.790 [5.490–10.495] | 8.810 [6.630–10.820] | 0.13 |
Lymphocyte (103/μL) | lym | 1 (0.38) | 1.455 [0.958–2.393] | 1.510 [0.955–2.385] | 1.370 [1.010–2.400] | 0.73 |
Ratio1 | ratio1 | 1 (0.38) | 5.569 [2.657–9.600] | 5.642 [2.541–9.523] | 5.169 [2.986–10.126] | 0.50 |
Hemoglobin (g/dL) | Hgb | 1 (0.38) | 144.500 [129.000–155.000] | 144.000 [128.500–156.000] | 146.000 [134.000–152.000] | 0.74 |
Mean corpuscular volume (fL) | MCV | 1 (0.38) | 0.910 [0.880–0.950] | 0.910 [0.880–0.950] | 0.920 [0.890–0.960] | 0.33 |
Platelet (103/μL) | PLT | 1 (0.38) | 212.500 [174.000–258.000] | 212.000 [169.500–257.500] | 218.000 [179.000–275.000] | 0.47 |
Plateletcrit (%) | PCT | 4 (1.53) | 0.220 [0.190–0.260] | 0.220 [0.190–0.260] | 0.230 [0.200–0.270] | 0.38 |
Troponin I (ng/mL) | TnI | 12 (4.6) | 0.440 [0.010–4.400] | 0.400 [0.010–3.700] | 0.560 [0.010–11.635] | 0.31 |
Myoglobin (ng/mL) | MYO | 12 (4.6) | 71.900 [30.000–228.000] | 70.600 [30.000–210.275] | 123.700 [30.450–307.100] | 0.22 |
CK-MB (mg/mL) | CK_MB | 12 (4.6) | 5.910 [2.500–28.130] | 5.680 [2.500–25.328] | 9.270 [2.515–36.920] | 0.21 |
Creatinine (μmol/L) | CREN | 1 (0.38) | 77.500 [63.750–94.250] | 77.000 [63.000–91.500] | 79.000 [68.000–99.000] | 0.24 |
Uric acid (μmol/L) | UA | 1 (0.38) | 346.500 [298.000–415.250] | 343.000 [297.500–415.500] | 367.000 [303.000–415.000] | 0.29 |
Potassium (mmol/L) | Potassium | 1 (0.38) | 3.935 [3.648–4.210] | 3.930 [3.660–4.200] | 3.950 [3.370–4.260] | 0.52 |
D-dimer (mg/L) | D_D | 30 (11.49) | 0.170 [0.100–0.390] | 0.170 [0.100–0.370] | 0.175 [0.115–0.512] | 0.30 |
NT-proBNP (pg/mL) | nt_BNP | 43 (16.48) | 1,521.839±4,955.617 | 1,318.011±4,579.102 | 2,428.875±6,359.820 | 0.20 |
C-reactive protein (mg/L) | CRP | 40 (15.33) | 1.500 [0.500–5.200] | 1.400 [0.500–5.300] | 2.400 [0.575–5.125] | 0.19 |
ST segment elevation value (mm) | ST_seg_ele | 0 | 2.000 [1.000–3.000] | 2.000 [1.000–3.000] | 2.000 [1.000–3.000] | 0.31 |
Comorbidities | ||||||
Hypertension (yes) | HTN | 0 | 138 (52.874) | 114 (53.774) | 24 (48.980) | 0.55 |
Diabetes mellitus (yes) | DM | 0 | 82 (31.418) | 63 (29.717) | 19 (38.776) | 0.22 |
Previous PCI (yes) | Prior_PCI | 0 | 4 (1.533) | 3 (1.415) | 1 (2.041) | 0.75 |
Hyperlipidemia (yes) | HLP | 0 | 122 (46.743) | 100 (47.170) | 22 (44.898) | 0.77 |
Clinic status | ||||||
Killip class | Killip | |||||
I | Killip.I | 0 | 205 (78.544) | 173 (81.604) | 32 (65.306) | 0.01 |
II–IV | Killip.II–IV | 56 (21.456) | 39 (18.396) | 17 (34.694) | ||
Preoperative systolic blood pressure (mmHg) | SBP | 1 (0.38) | 120.000 [108.750–135.000] | 120.000 [108.500–135.000] | 120.000 [109.000–132.000] | 0.96 |
Preoperative diastolic blood pressure (mmHg) | DBP | 1 (0.38) | 78.000 [70.000–89.000] | 78.000 [70.000–89.000] | 78.000 [70.000–89.000] | 0.73 |
Angiography | ||||||
Infarct-related artery | IRA | 0 | ||||
LAD | IRA.LAD | 142 (54.406) | 113 (53.302) | 29 (59.184) | 0.34 | |
LCX | IRA.LCX | 25 (9.579) | 23 (10.849) | 2 (4.082) | ||
RCA | IRA.RCA | 94 (36.015) | 76 (35.849) | 18 (36.735) | ||
Culprit lesion | culprit_lesion | 0 | ||||
Proximal | culprit_lesion.P | 115 (44.061) | 88 (41.509) | 27 (55.102) | 0.22 | |
Middle | culprit_lesion.M | 102 (39.080) | 87 (41.038) | 15 (30.612) | ||
Distal | culprit_lesion.D | 44 (16.858) | 37 (17.453) | 7 (14.286) | ||
Diffuse (yes) | diffuse | 0 | 76 (29.119) | 65 (30.660) | 11 (22.449) | 0.25 |
Multiple lesion (yes) | multi_vessel | 0 | 153 (58.621) | 125 (58.962) | 28 (57.143) | 0.82 |
Bifurcation (yes) | bifurcation | 0 | 140 (53.640) | 114 (53.774) | 26 (53.061) | 0.93 |
In-stent restenosis (yes) | ISR | 0 | 4 (1.533) | 4 (1.887) | 0 (0.000) | 0.33 |
TIMI thrombus grade (≥4) | TTG.4_5 | 0 | 116 (44.444) | 91 (42.925) | 25 (51.020) | 0.30 |
Initial TIMI flow (≥2) | initial_TIMI.2_3 | 0 | 78 (29.885) | 69 (32.547) | 9 (18.367) | 0.051 |
TFAID 3 | TFAID.3 | 0 | 161 (61.686) | 149 (70.283) | 12 (24.490) | <0.001 |
Intraoperative intervention measures | ||||||
Radial approach (yes) | Radial_Approach | 0 | 256 (98.084) | 208 (98.113) | 48 (97.959) | 0.94 |
Anticoagulation | ||||||
Heparin | anticoagulation.1 | 0 | 205 (78.544) | 169 (79.717) | 36 (73.469) | 0.34 |
Bivalirudin | anticoagulation.2 | 56 (21.456) | 43 (20.283) | 13 (26.531) | ||
Prophylactic tirofiban (yes) | Pro_tirofiban | 0 | 91 (34.866) | 80 (37.736) | 11 (22.449) | 0.04 |
Intracoronary thrombolysis (yes) | thrombolysis | 0 | 100 (38.314) | 81 (38.208) | 19 (38.776) | 0.94 |
Thrombotic aspiration (yes) | aspiration | 0 | 56 (21.456) | 39 (18.396) | 17 (34.694) | 0.01 |
Using of stent (yes) | stent | 0 | 218 (83.525) | 174 (82.075) | 44 (89.796) | 0.19 |
Number of stents | num_of_stent | 0 | 1.000 [1.000–1.000] | 1.000 [1.000–1.000] | 1.000 [1.000–1.000] | 0.31 |
Stent diameter (mm) | stent_diameter | 0 | 3.000 [2.500–3.500] | 3.000 [2.500–3.500] | 3.000 [2.750–3.500] | 0.08 |
Stent length (mm) | stent_length | 0 | 23.000 [18.000–33.000] | 24.000 [18.000–33.000] | 23.000 [18.000–29.000] | 0.91 |
Post dilatation (yes) | post_dilatation | 0 | 140 (53.640) | 112 (52.830) | 28 (57.143) | 0.59 |
Post-dilatation balloon diameter (mm) | balloon_diameter | 0 | 2.750 [0.000–3.500] | 2.750 [0.000–3.500] | 3.000 [0.000–3.500] | 0.31 |
Ratio2 | ratio2 | 0 | 1.000 [0.000–1.091] | 1.000 [0.000–1.083] | 1.000 [0.000–1.100] | 0.34 |
Data are presented as median [interquartile range], mean ± standard deviation or number (%). NRF, no-reflow; CK-MB, creatine kinase myocardial band isoenzyme; NT-proBNP, N-terminal pro B-type natriuretic peptide; PCI, percutaneous coronary intervention; LAD, left anterior descending artery; LCX, left circumflexus artery; RCA, right coronary artery; TIMI, thrombolysis in myocardial infarction; TFAID, TIMI flow after initial balloon dilation; Ratio1, neutrophil/lymphocyte; Ratio2, post-dilation balloon diameter/stent diameter.
Data processing
Data processing using R software (version 4.22, R Foundation for Statistical Computing, Vienna, Austria) involved streamlined steps for data optimization. Features with minimal variance were removed by the ‘nearZeroVar’ function, and those with over 10% missing values were excluded. Remaining missing data were subjected to multiple imputation via the ‘mice’ package. Skewed continuous variables, like cTnI and CK-MB, which exhibited high peak and long-tailed distributions, were normalized through log-transformation and standardization. This process was essential to address skewness, reduce the impact of outliers, and improve the model’s performance and interpretability. Collinearity, known to distort model estimations and predictions (11), was assessed using Pearson’s correlation coefficient, with variables of |r| ≥0.7 excluded in line with clinical relevance and prior research. Further feature refinement was then performed with the least absolute shrinkage and selection operator (LASSO) to select variables with non-zero LASSO coefficients. Outliers significantly affecting model accuracy were identified and removed via the ‘outliers.ranking’ function in the ‘DMwR2’ package. The dataset exhibited an imbalance due to NRF samples constituting only 18.77% of the total, and accordingly, the synthetic minority over-sampling technique (SMOTE) was applied in the training set, thereby enhancing data representation and reducing the risk of overfitting (12).
Prediction models
This study examined three algorithms: LR, RF, and eXtreme Gradient Boosting (XGBoost). These models are widely adopted in binary classification problems and possess excellent interpretability to assist clinicians in understanding and intervening in high-risk factors for NRF. Due to the risk of overfitting from excessive features and affecting performance on other datasets, variable selection was essential prior to model construction (13). Three methods were employed for variable selection:
- Conventional univariate LR analysis was conducted, with the retention of variables demonstrating P values less than 0.1.
- For the RF algorithm, recursive feature elimination (RFE) was employed, starting with the full feature set and progressively removing the least important features. ‘TrainControl’ was set for 10-fold repeated cross-validation and conducted three times for evaluating test results based on accuracy, accuracy standard deviation (SD), Kappa, and Kappa SD. Optimal variable count was identified as eight, where accuracy and Kappa were maximized, and accuracy SD and Kappa SD were minimized (Figure S1A). The top 8 variables were then confirmed based on the MeanDecreaseGINI index.
- In the XGBoost algorithm, RFE based on SHapley Additive exPlanations (SHAP) values was utilized. SHAP values helped interpret the impact of each feature on the model’s predictions by assigning an importance value to each feature, indicating how much each feature contributes to the model’s output. Following 10-fold cross-validation repeated thrice, the average SHAP values were computed and employed to rank the variables. Similar to the second method, variables with lower SHAP values were progressively eliminated. The optimal variable count was determined as 10, where Kappa peaked, and accuracy SD and Kappa SD were minimized (Figure S1B). The top 10 variables based on SHAP values were selected for further analysis.
In the LR framework, variables sourced from three distinct methodologies were harnessed for the construction of models, a process that involved forward and backward stepwise regression techniques, culminating in the development of three models, specifically designated as LR-classic, LR-RF, and LR-XGB. The superior model, exemplifying the efficacy of LR algorithm, was ascertained by a comparative analysis of the area under the curve (AUC) values, derived from the internal validation datasets. For the RF and XGBoost models, variables acquired according to the RFE-based methods were employed. The identification of the optimal models ensued after a rigorous process involving 10-fold cross-validation and meticulous hyperparameter tuning.
Performance evaluation
By means of internal validation sets, all models were evaluated, with comparison performed on key performance metrics, including AUC, accuracy, sensitivity (also known as Recall), specificity, Gmeans, F-Score, and Kappa Coefficient. Furthermore, the net benefit of each model was assessed using decision curve analysis (DCA) which accounts for the trade-off between the predicted benefits and the expected risk associated with NRF. Upon determining the optimal model, a calibration curve was exploited to examine the congruence between the calculated likelihood and the observed NRF prevalence in the population.
Statistical analysis
In the descriptive analysis, categorical variables were delineated as frequencies and percentages, and comparatively dissected through chi-square testing. Continuous variables were expressed by mean (SD) or median (interquartile range), and subjected to Independent-sample t-tests or Wilcoxon U tests based on their distribution characteristics. Statistical significance of AUC differences between models was determined using the DeLong test. All statistical analyses were performed using R software (version 4.2.2, R Foundation for Statistical Computing, Vienna, Austria). The statistical significance level was defined by P<0.05.
Results
Baseline characteristics
As per the inclusion and exclusion criteria, 261 patients were enrolled for subsequent analyses, and distributed into the normal flow group (212 patients) and the NRF group (49 patients). More specific data were depicted in Table 1, which not only provides information covering demographic data, test results, comorbidities, clinical conditions, coronary angiography, and intraoperative interventions in these groups, but also details the assignment of variables and the status of missing values.
Features
Following data processing, features demonstrating near-zero variance (Previous PCI, in-stent restenosis and radial approach) were removed, and three features (D-dimer, N-terminal pro B-type natriuretic peptide, and C-reactive protein) were excluded as missing values exceeded 10%. Correlation tests revealed multicollinearity among several features, according to which six features were excluded: CK-MB, Plateletcrit, Using of Stent, Number of Stents, Diameter of Post-Dilatation Balloon, and Ratio2 (Figure 2). The LASSO method and 10-fold cross-validation further identified thirteen eliminable features with zero coefficients, including gender, age, symptom to wire, lymphocyte, ratio1, hemoglobin, creatinine, hyperlipidemia, preoperative systolic blood pressure, culprit lesion, diffuse, intracoronary thrombolysis, and TIMI thrombus grade (Figure 3A,3B), after which 25 features were chosen for final analysis. Through traditional univariate LR, together with forward and backward methods, 15 variables were retained (Figure 3C). The RF algorithm, based on RFE, highlighted 9 variables (Figure 3D). Finally, the XGBoost algorithm, combined with SHAP and RFE, preserved 10 variables, with their SHAP values and rankings illustrated in Figure 3E.
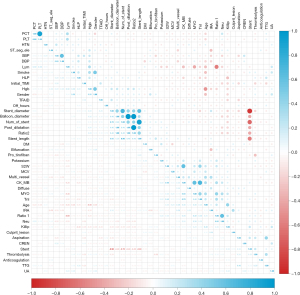
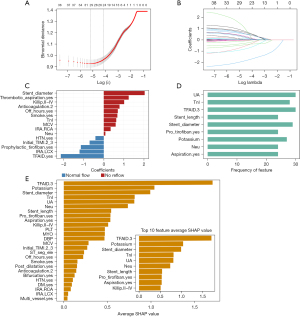
Model performance
Among the LR models evaluated, the LR-XGB model displayed superior predictive performance with an AUC of 0.829 [95% confidence interval (CI): 0.779–0.880], outperforming the LR-classic and LR-RF models that achieved AUCs of 0.728 (95% CI: 0.663–0.793) and 0.770 (95% CI: 0.712–0.827), respectively (Figure 4A). The statistically significant differences in AUC between LR-XGB and LR-classic, and between LR-XGB and LR-RF, were confirmed by the DeLong test (LR-XGB vs. LR-classic: P=0.02; LR-XGB vs. LR-RF: P<0.001). Thus, the LR-XGB model was perceived as the optimal LR model. Comparatively, the RF and XGBoost models yielded AUCs of 0.731 (95% CI: 0.660–0.802) and 0.835 (95% CI: 0.781–0.889) (Figure 4A), respectively. Internal validation highlighted distinct strengths across models: the LR model led in accuracy, F-score, and Kappa; XGBoost model in sensitivity, Gmeans, and AUC; and RF model in specificity (Table 2 and Figure 4B). DCA indicated the LR model had a superior clinical net benefit within the relevant threshold range (Figure 4C). The calibration curve affirmed the favorable calibration of LR model (Figure 4D). In this model, Killip class II–IV, TnI levels, thrombotic aspiration, and stent diameter were recognized as independent risk factors for NRF, whereas potassium levels, prophylactic tirofiban, and TFAID 3 emerged as protective factors (Figure 5A). Furthermore, TFAID was consistently the most influential feature across all models, and serum potassium frequently ranked in the top three (Figure 5B,5C).
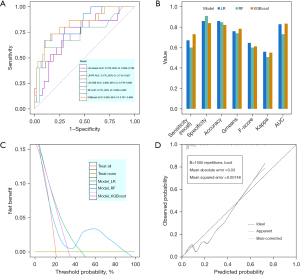
Table 2
Model | Sensitivity (recall) | Specificity | Accuracy | Gmeans | F-score | Kappa | AUC |
---|---|---|---|---|---|---|---|
LR | 0.670 | 0.860 | 0.860 | 0.759 | 0.645 | 0.559 | 0.829 |
RF | 0.600 | 0.910 | 0.848 | 0.739 | 0.600 | 0.506 | 0.731 |
XGBoost | 0.730 | 0.840 | 0.820 | 0.783 | 0.611 | 0.550 | 0.835 |
LR, logistic regression; RF, random forest; XGBoost, eXtreme Gradient Boosting; AUC, area under the receiver operating characteristic curve.

Optimal web-based calculator
In view of the LR model as the optimal model in this study, a web-based calculator was further developed for predicting NRF in pPCI using this model in the “Shiny” application. To facilitate user access, the calculator was set up with three distinct panels: one for selecting and entering model parameters, one for obtaining the predicted NRF occurrence rates, and the other for offering an overview of the model alongside a forest plot illustrating the OR for each feature (Figure 6). This calculator was available at https://l7173o-wang-lyn.shinyapps.io/shiny-1/.
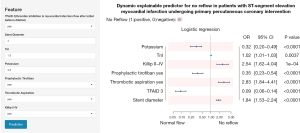
Discussion
In this study, we developed a sophisticated predictive model to assess the risk of NRF during pPCI in patients with STEMI, and employed three advanced ML algorithms for rigorous data analysis. Additionally, to enhance the clinical utility of the model, we created an intuitive, web-based application grounded in the most effective classification model.
First, to identify features influencing NRF as comprehensively as possible, we selected a total of 50 features for analysis, encompassing demographic data, test results, comorbidities, clinical conditions, coronary angiography, and intraoperative interventions. Some of these features have been identified in previous studies as potential predictors of NRF occurrence (3,6-8,14-20). Furthermore, specific factors such as pPCI performed during off-hours and operators’ limited experience in femoral access have been confirmed to be linked to poorer clinical outcomes (21,22). Consequently, these factors were included in our analysis to investigate their association with NRF.
Among all evaluated classification models, our LR model outperformed the RF and XGBoost models in terms of predictive accuracy for NRF, aligning with the results of previous studies that have utilized traditional LR for NRF prediction (5,8). However, a key advantage in our study lies in a comprehensive and innovative approach for data preparation and feature engineering prior to the final construction of the LR model. This approach, unlike the methodologies employed in earlier research, entailed a multifaceted strategy encompassing advanced techniques such as RF-based multiple imputation for handling missing data, SMOTE for addressing data imbalance, and RFE with RF and XGBoost models for selecting sophisticated feature. These advanced methodologies not only enhanced the predictive power of our LR model but also contributed to a more nuanced and robust analysis, distinguishing our research in the field of NRF prediction studies. By dint of these methods, the AUC of the LR model has markedly improved from 0.728 to 0.770 and 0.829.
In our model evaluation process, a suite of metrics was utilized to assess the efficacy of different classification models. The AUC values of both LR and XGBoost models were well matched and prominently exceeded that of the RF model. To further refine our assessment, we incorporated supplementary performance evaluation indicators, including accuracy, specificity (recall), sensitivity, F1-score, Gmean, and Kappa coefficient. Notably, neither LR nor XGBoost model consistently outperformed in terms of all these metrics. To determine the most optimal model, we employed DCA, a method that is superior to traditional performance measures by evaluating the clinical decision-making utility of models (23). DCA plots the net benefit across a range of plausible clinical risk thresholds. The DCA demonstrated that the LR model had a greater clinical net benefit than XGBoost, a vital determinant in its selection as the preferred model.
The LR model featured seven clinically accessible variables, and highlighted TFAID as a novel and vital predictor of NRF, which have not been extensively documented in prior research. Factors such as residual stenosis, local obstructions (like coronary artery dissection or hematoma), ischemia-reperfusion injury, endothelial cell swelling, and microvascular obstruction (MVO) make a profound impact upon post-dilation blood flow (16). While stent deployment can mitigate local blockages, strategies to address MVO are still under exploration (4). A TFAID score of 3 decreased the risk of intraoperative NRF by 91% [odds ratio (OR) 0.09 (95% CI: 0.06–0.14); P<0.0001], implying that subsequent intervention strategies warrant prudent selection to avoid NRF when TFAID is below 3.
In our study, intraoperative tactics, for addressing issues such as the prophylactic administration of tirofiban, thrombus aspiration, and stent diameter, are pivotal factors influencing the occurrence of NRF. Tirofiban is notably effective in NRF prevention and treatment, with its intracoronary delivery acting better than intravenous administration in reducing MVO (24,25). Evidence from two large randomized trials suggests that aspiration thrombectomy fails to improve microcirculation or clinical outcomes in STEMI patients undergoing pPCI, leading to recommendations against its routine use (26,27). Our analysis indicated thrombus aspiration may inadvertently raise NRF risk, possibly attributed to mechanical effects post thrombus detachment. Furthermore, the stent with an inappropriate diameter, especially larger and overexpanded stents, is a vital inducement for NRF (19,20,28). Large vessels often have lower flow velocities and contain substantial plaques and thrombi, which can break and extrude through stent struts, thus forming microemboli that lead to distal microvascular embolism and increased resistance. Additionally, previous studies also have identified stent length as a significant risk factor for NRF, possibly due to a higher burden of plaque and thrombus (7,15,20). Although this variable did not feature in the optimal model of our study, it ranked moderately in the RF and XGBoost models. Hence, stent placement decisions, particularly under high NRF risk, necessitate careful consideration. The strategy of deferred stenting, which delays stent placement following initial reperfusion, shows promise in reducing NRF incidence (16).
Intriguingly, our findings accentuated serum potassium levels as a prime predictor consistently among the top three variables in all models. Hypokalemia enhances cell membrane hyperpolarization, causing sustained vascular contraction and increased distal vessel resistance. Normalizing extracellular potassium can reduce free radicals in macrophages and endothelial cells, inhibit vascular smooth muscle cell proliferation, and attenuate platelet responsiveness (29). There is evidence supporting the use of nicorandil, a potassium channel opener, to prevent and mitigate NRF, despite the undefined impact of potassium supplementation on reducing NRF in STEMI patients (30,31), which, however, deserves investigation as a potential research target. Furthermore, elevated troponin I (TnI) levels at admission and a Killip classification above 2 are identified as risk factors for NRF, congruent with findings from previous studies (3,7,8). Fortunately, these variables can be rapidly available in the emergency room setting.
More importantly, a web-based calculator, developed using the LR model and incorporating the aforementioned seven variables, enabled clinicians to estimate the likelihood of NRF in STEMI patients undergoing pPCI. The tool was designed to predict the risk of NFR in future randomized controlled studies, as well as to reduce NFR by adapting strategies. Despite difficulties in modifying some of the parameters included in this calculator, such as TnI levels, Killip classification and TFAID, certain intraoperative decisions by clinicians may potentially reduce the incidence of NRF. These strategies included the prophylactic intracoronary administration of tirofiban, correction of hypokalemia or intraoperative use of nicorandil, avoidance of routine thrombus aspiration, careful selection of stent diameter, and deferred stenting in high-risk patients.
Limitation
However, there is also room for improvement in our research. First, there is a paucity of the real-world application results regarding this tool. In the next phase of our research, we plan to use this model and its online calculator in a multi-center, prospective randomized controlled trial to confirm whether this tool can influence intraoperative decisions during pPCI to reduce the incidence of NRF and improve patients’ outcomes. The pre-procedural interventions and adjustments to interventional strategies based on instantaneous computational outcomes will be evaluated to validate the efficacy of the predictive model in a clinical setting. Second, as a single-center retrospective analysis with a constrained sample size, the generalizability of our LR model is limited due to the lack of external validation across larger and multi-centric cohorts. Future efforts will be made to expand our dataset from multiple centers to enhance the robustness and applicability of the model. Third our study excludes blockages in the left main artery and other branches. Left main lesions often involve true bifurcations that necessitate more complex intraoperative maneuvers, differing prominently from interventions in other vessels. Branch vessels, often narrower, are less likely to have stents implanted compared to main vessel occlusions owing to their small size. Future studies will seek to incorporate data on NRF occurrence in left main and branch vessels to provide a more comprehensive understanding.
Conclusions
In summary, this research utilizes a range of ML algorithms to construct a LR model that can predict the incidence of NRF during pPCI in patients with STEMI. Leveraging the insights derived from this model, a web-based tool has been developed to assist in the intraoperative decision-making. Future advancements in the field, combining NRF prevention with the capabilities of artificial intelligence, hold promise for significantly improving the outcomes of STEMI patients.
Acknowledgments
We express our profound gratitude to Kai Wang, Yu Fang, Hao Zhang, Ran Chen, and Wanting Zhou for their invaluable assistance in providing patient data and facilitating data collection. Our thanks also extend to Yixiang Zhou for deploying the model to the web and enhancing the interface’s aesthetic appeal. We would like to thank Yu Xiao (Wie-Biotech Company) for her help in language editing. Special acknowledgment goes to Xin Su for offering critical suggestions on optimizing machine learning algorithms. L.W.’s deepest appreciation is reserved for his wife, whose meticulous care for their family has allowed him to dedicate himself fully to this research.
Funding: This study was supported by
Footnote
Reporting Checklist: The authors have completed the TRIPOD reporting checklist. Available at https://cdt.amegroups.com/article/view/10.21037/cdt-24-83/rc
Data Sharing Statement: Available at https://cdt.amegroups.com/article/view/10.21037/cdt-24-83/dss
Peer Review File: Available at https://cdt.amegroups.com/article/view/10.21037/cdt-24-83/prf
Conflicts of Interest: All authors have completed the ICMJE uniform disclosure form (available at https://cdt.amegroups.com/article/view/10.21037/cdt-24-83/coif). The authors have no conflicts of interest to declare.
Ethical Statement: The authors are accountable for all aspects of the work in ensuring that questions related to the accuracy or integrity of any part of the work are appropriately investigated and resolved. The study was conducted in accordance with the Declaration of Helsinki (as revised in 2013). The study was approved by the institutional review board of The Second Affiliated Hospital of Anhui Medical University (No. YX2022-001) and individual consent for this retrospective analysis was waived.
Open Access Statement: This is an Open Access article distributed in accordance with the Creative Commons Attribution-NonCommercial-NoDerivs 4.0 International License (CC BY-NC-ND 4.0), which permits the non-commercial replication and distribution of the article with the strict proviso that no changes or edits are made and the original work is properly cited (including links to both the formal publication through the relevant DOI and the license). See: https://creativecommons.org/licenses/by-nc-nd/4.0/.
References
- Byrne RA, Rossello X, Coughlan JJ, et al. 2023 ESC Guidelines for the management of acute coronary syndromes. Eur Heart J 2023;44:3720-826. [Crossref] [PubMed]
- Chandrashekhar Y, Alexander T, Mullasari A, et al. Resource and Infrastructure-Appropriate Management of ST-Segment Elevation Myocardial Infarction in Low- and Middle-Income Countries. Circulation 2020;141:2004-25. [Crossref] [PubMed]
- d'Entremont MA, Alazzoni A, Dzavik V, et al. No-reflow after primary percutaneous coronary intervention in patients with ST-elevation myocardial infarction: an angiographic core laboratory analysis of the TOTAL Trial. EuroIntervention 2023;19:e394-401. [Crossref] [PubMed]
- Rezkalla SH, Stankowski RV, Hanna J, et al. Management of No-Reflow Phenomenon in the Catheterization Laboratory. JACC Cardiovasc Interv 2017;10:215-23. [Crossref] [PubMed]
- Celik T, Balta S, Ozturk C, et al. Predictors of No-Reflow Phenomenon in Young Patients With Acute ST-Segment Elevation Myocardial Infarction Undergoing Primary Percutaneous Coronary Intervention. Angiology 2016;67:683-9. [Crossref] [PubMed]
- Fajar JK, Heriansyah T, Rohman MS. The predictors of no reflow phenomenon after percutaneous coronary intervention in patients with ST elevation myocardial infarction: A meta-analysis. Indian Heart J 2018;70:S406-18. [Crossref] [PubMed]
- Deng L, Zhao X, Su X, et al. Machine learning to predict no reflow and in-hospital mortality in patients with ST-segment elevation myocardial infarction that underwent primary percutaneous coronary intervention. BMC Med Inform Decis Mak 2022;22:109. [Crossref] [PubMed]
- Liu Y, Ye T, Chen K, et al. A nomogram risk prediction model for no-reflow after primary percutaneous coronary intervention based on rapidly accessible patient data among patients with ST-segment elevation myocardial infarction and its relationship with prognosis. Front Cardiovasc Med 2022;9:966299. [Crossref] [PubMed]
- Collins GS, Reitsma JB, Altman DG, et al. Transparent reporting of a multivariable prediction model for individual prognosis or diagnosis (TRIPOD): the TRIPOD statement. BMJ 2015;350:g7594. [Crossref] [PubMed]
- Paszek E, Zajdel W, Musiałek P, et al. Percutaneous management of long and diffused coronary lesions using newer generation drug-eluting stents in routine clinical practice: long-term outcomes and complication predictors. Pol Arch Intern Med 2019;129:392-8. [Crossref] [PubMed]
- Dormann, Carsten & Elith, Jane & Bacher, et al. Collinearity: A review of methods to deal with it and a simulation study evaluating their performance. Ecography. 2013. (36): 027–046.
- Wang X, Ren H, Ren J, et al. Machine learning-enabled risk prediction of chronic obstructive pulmonary disease with unbalanced data. Comput Methods Programs Biomed 2023;230:107340. [Crossref] [PubMed]
- Chowdhury MZI, Turin TC. Variable selection strategies and its importance in clinical prediction modelling. Fam Med Community Health 2020;8:e000262. [Crossref] [PubMed]
- Wang JW, Zhou ZQ, Chen YD, et al. A risk score for no reflow in patients with ST-segment elevation myocardial infarction after primary percutaneous coronary intervention. Clin Cardiol 2015;38:208-15. [Crossref] [PubMed]
- Bayramoğlu A, Taşolar H, Kaya A, et al. Prediction of no-reflow and major adverse cardiovascular events with a new scoring system in STEMI patients. J Interv Cardiol 2018;31:144-9. [Crossref] [PubMed]
- Ndrepepa G, Kastrati A. Coronary No-Reflow after Primary Percutaneous Coronary Intervention-Current Knowledge on Pathophysiology, Diagnosis, Clinical Impact and Therapy. J Clin Med 2023;12:5592. [Crossref] [PubMed]
- Hu X, Wang W, Ye J, et al. Effect of GP IIb/IIIa inhibitor duration on the clinical prognosis of primary percutaneous coronary intervention in ST-segment elevation myocardial infarction with no-/slow-reflow phenomenon. Biomed Pharmacother 2021;143:112196. [Crossref] [PubMed]
- Yang L, Cong H, Lu Y, et al. A nomogram for predicting the risk of no-reflow after primary percutaneous coronary intervention in elderly patients with ST-segment elevation myocardial infarction. Ann Transl Med 2021;9:126. [Crossref] [PubMed]
- Aggarwal P, Rekwal L, Sinha SK, et al. Predictors of no-reflow phenomenon following percutaneous coronary intervention for ST-segment elevation myocardial infarction. Ann Cardiol Angeiol (Paris) 2021;70:136-42. [Crossref] [PubMed]
- Chen Y, Gao YF, Wang YF, et al. Influence of Stent Length on Periprocedural Outcomes After Primary Percutaneous Coronary Intervention in Patients with ST Segment Elevation Myocardial Infarction. Clin Interv Aging 2022;17:1687-95. [Crossref] [PubMed]
- Tokarek T, Dziewierz A, Plens K, et al. Percutaneous coronary intervention during on- and off-hours in patients with ST-segment elevation myocardial infarction. Hellenic J Cardiol 2021;62:212-8. [Crossref] [PubMed]
- Tokarek T, Dziewierz A, Plens K, et al. Radial Approach Expertise and Clinical Outcomes of Percutanous Coronary Interventions Performed Using Femoral Approach. J Clin Med 2019;8:1484. [Crossref] [PubMed]
- Li R, Lin S, Tu J, et al. Establishment and evaluation of a novel practical tool for the diagnosis of pre-sarcopenia in young people with diabetes mellitus. J Transl Med 2023;21:393. [Crossref] [PubMed]
- Akpek M, Sahin O, Sarli B, et al. Acute Effects of Intracoronary Tirofiban on No-Reflow Phenomena in Patients With ST-Segment Elevated Myocardial Infarction Undergoing Primary Percutaneous Coronary Intervention. Angiology 2015;66:560-7. [Crossref] [PubMed]
- Ma Q, Ma Y, Wang X, et al. Intracoronary compared with intravenous bolus tirofiban on the microvascular obstruction in patients with STEMI undergoing PCI: a cardiac MR study. Int J Cardiovasc Imaging 2020;36:1121-32. [Crossref] [PubMed]
- Fröbert O, Lagerqvist B, Olivecrona GK, et al. Thrombus aspiration during ST-segment elevation myocardial infarction. N Engl J Med 2013;369:1587-97. [Crossref] [PubMed]
- Jolly SS, Cairns JA, Yusuf S, et al. Randomized trial of primary PCI with or without routine manual thrombectomy. N Engl J Med 2015;372:1389-98. [Crossref] [PubMed]
- Li X, Sun S, Luo D, et al. Microvascular and Prognostic Effect in Lesions With Different Stent Expansion During Primary PCI for STEMI: Insights From Coronary Physiology and Intravascular Ultrasound. Front Cardiovasc Med 2022;9:816387. [Crossref] [PubMed]
- Young DB, Ma G. Vascular protective effects of potassium. Semin Nephrol 1999;19:477-86. [PubMed]
- Gupta H, Parihar S, Tripathi VD. Assessment of the Efficacy and Safety of Early Intracoronary Nicorandil Administration in Patients With ST-Elevation Myocardial Infarction Undergoing Primary Percutaneous Coronary Intervention. Cureus 2022;14:e25349. [Crossref] [PubMed]
- Qi Q, Niu J, Chen T, et al. Intracoronary Nicorandil and the Prevention of the No-Reflow Phenomenon During Primary Percutaneous Coronary Intervention in Patients with Acute ST-Segment Elevation Myocardial Infarction. Med Sci Monit 2018;24:2767-76. [Crossref] [PubMed]