Recent progress in metabolomic analysis of acute coronary syndrome: a narrative review
Introduction
Acute coronary syndrome (ACS) is a clinical syndrome caused by acute myocardial ischemia, and is one of the leading causes of death worldwide (1). The main pathogenesis of ACS is the sudden rupture and superficial erosion of atherosclerotic plaque in the process of occurrence and development, which causes thrombosis and eventually leads to insufficient blood supply or necrosis of myocardium (2-4). ACS subtypes include unstable angina (UA), non-ST-segment elevation myocardial infarction (NSTEMI) and ST-segment elevation myocardial infarction (STEMI) (5). Currently, the diagnosis of ACS requires a combination of clinical and electrocardiographic (ECG) signals, and myocardial injury markers are necessary to distinguish the various subtypes of ACS. However, not only may biomarkers such as troponin be increased in other diseases, but the identification of different subtypes also requires ongoing detection of ECG and biomarkers of myocardial damage in conjunction with clinical manifestations (6). There is undoubtedly an urgent need for more low-cost but highly sensitive and specific biomarkers.
With the continuous advancement of science and technology in the field of systems biology, after genomics and proteomics, metabolomics has also entered everyone’s field of vision (7). Metabolomics can qualitatively and quantitatively analyze all small molecule metabolites in an organism, providing an avenue for studying the relative relationships between physiological and pathological changes. Since dynamic perturbations of the genome, transcriptome, and proteome can all be reflected by changes in an organism’s metabolites, metabolites can efficiently link genotype to phenotype (8). For this reason, metabolomics can provide information about organisms under normal or pathological conditions, and reflect changes in organisms in response to metabolites stimulated by external stimuli (9). Metabolomics has been widely used to discover novel diagnostic and prognostic biomarkers and more therapeutic targets to improve disease outcomes (10).
In previous studies, researchers have also reviewed the metabolomic-related studies of ACS. In 2019, Pouralijan Amiri et al. (10) provided a comprehensive review of the pathogenesis of ACS, metabolomics techniques, and several studies on the application of metabolomics for detecting ACS. Their work highlights that metabolomics is a robust method for characterizing metabolic disturbances associated with various cardiovascular diseases. In 2021 and 2023, researchers augmented the existing technical methodologies in metabolomics, elucidated the experimental protocols for detecting diseases through metabolomics, and synthesized findings from previously identified altered metabolic pathways associated with ACS (1,11). This paper focuses more on the potential diagnostic and prognostic biomarkers and their diagnostic and prognostic abilities found in previous studies based on metabolomics, and summarizes the types they belong to, so as to find the metabolic pathways that are most prone to change when diseases occur. We present this article in accordance with the Narrative Review reporting checklist (available at https://cdt.amegroups.com/article/view/10.21037/cdt-24-431/rc).
Methods
This study is a narrative review aimed at identifying and searching current literature on studies related to the metabolomics of ACS. A combination of “acute coronary syndrome”, “unstable angina”, “NSTEMI, STEMI” and “metabolomics” was used to search PubMed literature in English from January 2014 to December 2024. We included articles that identified potential diagnostic and prognostic markers for ACS and their subtypes, as well as articles that identified mechanisms of drug treatment for the disease through metabolomics. The main objective of our review was to summarize the potential diagnostic and prognostic markers identified by metabolomics over the last decade and to explore the changes in metabolic pathways that most commonly occur in ACS. Table 1 summarizes the search strategies used in our review, the full electronic search strategy can be seen in Table S1.
Table 1
Items | Specification |
---|---|
Date of search | 03/15/2022–27/08/2024 |
Database searched | PubMed |
Search terms used | (“acute coronary syndrome” OR “unstable angina” OR “NSTEMI” OR “STEMI” ) AND “metabolomics” |
Timeframe | 01/01/2014–12/03/2024 |
Inclusion and exclusion criteria | Inclusion: primary investigational, clinical trial |
Exclusion: Review etc. | |
Selection process | Articles were selected and reviewed by J.L. and T.L. |
Any additional considerations, if applicable | Additional relevant references were included during the primary literature search |
NSTEMI, non-ST-segment elevation myocardial infarction; STEMI, ST-segment elevation myocardial infarction.
Techniques applied to metabolomics analysis
Currently, nuclear magnetic resonance (NMR) spectroscopy and mass spectrometry are the two most common analytical tools used in metabolomics studies (12). NMR is a pioneering platform for metabolomics that combines non-destructiveness, unbiasedness, high stability and reproducibility. Its main advantages are the small sample size required and simple pre-treatment, which can provide accurate molecular structures of compounds (13). However, the greatest problem is that it is not as sensitive as mass spectrometry and is prone to signal overlap, leading to inaccurate results (14). Mass spectrometry is generally combined with gas chromatography (GC-MS) and liquid chromatography (LC-MS), the overall sensitivity of mass spectrometry is higher than that of NMR, and the application of LC-MS is more widespread (15). LC-MS detection of metabolites is not limited by difficult volatility and thermal instability, and sample preparation is simpler than GC-MS as no derivatization is needed (8). GC-MS can be used for the detection of volatile or non-polar metabolites but sample preparation is complex. And it is very difficult to detect non-volatile or thermally unstable, easily degradable substances (12). The main advantages and disadvantages of NMR, LC-MS and GC-MS techniques are summarized in Table 2. In general, it is difficult to detect all metabolites with a single detection technique alone, so an increasing number of groups are now combining NMR and MS, using their complementary functions to improve the quality of detection, and to identify more metabolic compounds (16).
Table 2
Technique | Advantages | Disadvantages |
---|---|---|
NMR | • Simple sample pre-processing | • Low sensitivity |
• High stability and reproducibility | • Resonance has multiplicity, noise will repeat with the signal, low resolution | |
• Non-destructive to samples | ||
• Detects liquids as well as solids, gases, and tissue samples | ||
• Simulates physiological conditions detection | ||
• Provides accurate information on the molecular structure and concentration about compounds | ||
GC-MS | • Ultra-high column efficiency and peak capacity, better separation efficiency | • Complex sample preparation |
• Available for analysis of volatile and non-polar metabolites | • No thermally unstable substances detection | |
• Database available for identification provides relatively qualitative results | • No polar metabolites detection | |
LC-MS | • Smaller sample size required | • Low reproducibility |
• Proven data processing platform available | • Ion suppression | |
• Suitable for analysis of substances with highly repetitive structures | • No gas mixture detection | |
• Not limited by difficult volatility and thermal instability |
GC-MS, gas chromatography mass spectrometry; LC-MS, liquid chromatography mass spectrometry; NMR, nuclear magnetic resonance.
Application of metabolomics to discover potential biomarkers of ACS
The development of disease is often associated with genetic or phenotypic changes in the organism, so we have been able to diagnose and predict the occurrence of disease through changes in genes, proteins and metabolites. In contrast, most researchers believe that metabolites are better suited for clinical biomarker development and research (17). The number of biomarkers has been increasing in recent years, and over the past decade, many researchers have conducted extensive research on diagnostic and prognostic biomarkers associated with ACS. A comprehensive list of metabolomics studies on ACS focusing on diagnostic biomarkers and prognostic biomarkers is presented in Tables 3,4.
Table 3
No. | First author, year | Disease | Sample size | Source | Technique | Biomarker |
---|---|---|---|---|---|---|
1 | Yin et al. (18), 2017 | ACS | 20 ACS; 20 non-ACS | Plasma | LC-MS | 7 LysoPCs, 2 LysoPEs, 2 glycerophosphocholines, 3 Sphingomyelin, L-tryptophan, glucose, caffeine, paraxanthine, palmitoleic acid, indoleacrylic acid, oleic acid |
2 | Zhong et al. (19), 2021 | ACS | 284 ACS; 130 HCs | Plasma | LC-MS | Lysophosphatidylcholine (20:4), lysophosphatidylcholine ((16:0), 5-oxo-D-proline, creatinine |
3 | Song et al. (20), 2021 | ACS | 45ACS; 29 HCs | Serum | UPLC-MS | lysoPC(20:4(8Z,11Z,14Z,17Z)/0:0), sphingomyelin (d18:0/16:0), Sphingomyelin (d18:1/14:0) |
4 | Wei et al. (21), 2021 | ACS | 21 ACS anxiety; 26 ACS | Plasma | LC-MS | Serotonin; oleoylcarnitine; aminoethoxyacetic acid; tryptophan, argininosuccinic acid; homoserine,17-hydroxypregnenolone sulfate; 19-hydroxyandrost-4-ene-3,17-dione; 3-alpha-androstanediol glucuronide; 5a-tetrahydrocorticosterone; androsterone sulfate; LysoPC(15:0); LysoPC(16:0); LysoPC(18:1(11Z)); LysoPC(20:5(5Z,8Z,11Z,14Z,17Z)); LysoPC(22:5(4Z,7Z,10Z,13Z,16Z)); LysoPC(22:6(4Z,7Z,10Z,13Z,16Z,19Z)); 4-hydroxynonenal; thromboxane B2; triglyceride; 4-hydroxycyclohexylcarboxylic acid; Trans-aconitic acid; 7-methylguanine; 11-Oxo-androsterone glucuronide; deoxycholic acid 3-glucuronide; 5-androstenetriol; tetrahydrocortisone; 25-Hydroxyvitamin D2; alpha-carboxyethyl; hydroxychroman; trigonelline; 3-carbamoyl-2-phenylpropionaldehyde; phosphorylcholine; isobutyryl-L-carnitine; glycerophosphocholine; LysoPC(18:0); hippuric acid; alanine |
5 | Fu et al. (22), 2023 | ACS | 44 UA; 77 AMI; 29 HCs | Serum | LC-MS/MS | Octanoylcarnitine; cyclic GMP; LysoPC(18:2(9Z,12Z)); 2-ketobutyric acid; indoxyl sulfate |
6 | Qiu et al. (23), 2023 | ACS | 500 ACS; 500 HCs | Serum | LC-MS/MS | 1,5-anhydro-D-glucitol; tetracosanoic acid; aspartylphenylalanine |
7 | Shibata et al. (24), 2024 | ACS | 18 ACS; 24 HCs | Serum | UPLC-Q-TOF/MS | Isocitrate; lysine; tryptophan |
8 | Fan et al. (25), 2016 | AMI | 2,019 center 1; 71 center 2; 68 center 3; 166 center 4 | Plasma | LC-Q-TOF/MS | LysoPC 16:1; glycodeoxycholic acid LysoPC 18:2; LysoPC 16:0; palmitoylethanolamide; choline 5-hydroxy; tryptamine; threonine; acetylcarnitine; LysoPC 14:0; tryptophan; 2α-methyl-5α-androstane-3, 17-dione; trimethylamine 3-Octanone; decenyl acetate; fumaric acid; MG 18:0/0:0/0:0; phosphatidylcholine; CerP d18:0/16:0; N-methylisoleucine; LysoPE 22:6; Undecan-3-ol; ethylchenodeoxycholic acid; LysoPE 20:4; LysoPE 22:5; succinic acid; Valine; LysoPC 20:3; Indole-3-ethanol; LysoPC 24:0; LysoPC 22:5; LysoPC 22:6; LysoPE 16:0; LysoPE 18:0; LysoPE 20:1; 3-methyl-2-butene-1-thiol; PE 12:0/22:6; LysoPC 20:5; PG 13:0/14:0; LysoPE 18:3; PG 15:0/14:0; glycerophosphocholine; LysoPE 22:1 PE 20:0/0:0 PE 18:0/0:0 PI 18:2/0:0 2-Nonynoic acid; LysoPC 20:4; PE 21:0/0:0; octadecadienoic acid; CerP d18:1/8:0; LysoPC 10:0; LysoPC 18:0 LysoPE 18:1; PI 18:3/0:0; 2-octenoylcarnitine; phosphocholine; docosahexaenoic acid; LysoPC 18:1; glycocholic acid; γ-aminobutyric acid |
9 | Aa et al. (26), 2021 | AMI | 230 chest pain patients (85 MI; 61 non-MI); 84 HC | Plasma | LC-MS; GC-MS | Methionine; L-phenylalanine; deoxyuridine |
10 | Martin-Lorenzo et al. (27), 2015 | AMI | 7 experimental group rabbits; 7 control group rabbits | Urinary | 1H-NMR; LC-MS/MS | Kynurenine; N-acetyl neuraminic acid; scyllo-inositol |
11 | Xu et al. (28), 2016 | AMI | 38 SA; 34 AMI; 71 HCs | Serum | UPLC-Q-TOF/MS | Ceramide (d18:0/12:0); ceramide (t18:0/16:0); dehydroepiandrosterone sulfate |
12 | Jiang et al. (29), 2020 | AMI | 33 SPs; 32 VPs | Serum | LC-MS | Betaine; 1-heptadecanoyl-sn-glycero-3-phosphocholine; methylmalonate; hippurate; cis-aconitate; paraxanthine; acetylcarnitine; undecylic acid; pyruvate |
13 | Guo et al. (30), 2021 | AMI | 21 young AMI; 15 older AMI; 9 young HCs | Plasma | UPLC-MS/MS | Arachidonic acid; alpha-linolenic acid; 8,11,14-eicosatrienoic; adrenic acid; gamma-linolenic acid; linoleic acid |
14 | Petras et al. (31), 2020 | AMI | 30 AMI; 30 HCs | Plasma | 1H-NMR | 3-hydroxybutyrate; glutamine; alanine; inosine; myo-inositol; glycerol; pyruvate; tryptophan |
15 | Li et al. (32), 2020 | AMI | 136 NOCAD; 118 AMI | Serum | UPLC-Q-TOF/MS | 5-hydroxydantrolene; acetylglycine; glycerophospholipid; fatty acid; glutarylglycine; threoninyl-glycine; acylcarnitines |
16 | Deng et al. (33), 2018 | AMI | 45 AMI; 45 CPC | Serum | 1H-NMR | Phosphorylcholine; histidine; glycerophosphorylcholine; unsaturated fatty acids; citrate; gamma-glutamylthreonine; hypoxanthine; α-glucose; choline; isoleucine; valine; lysine; leucine; glutamate; tyrosine; lactate; alanine; phenylalanine; glutamine |
17 | Wang et al. (34), 2017 | AMI | CAL-rats, 34 infarcted myocardia; 34 non-infarcted myocardia | Tissue | GC-MS | Histidine; lactate; glutamate |
18 | Zhang et al. (35), 2018 | AMI | 2,019 center 1; 71 center 2; 68 center 3; 166 center 4 | Plasma | LC-Q-TOF/MS | N-acetylneuraminic acid |
19 | Park et al. (36), 2015 | AMI | 70 angina; 70 MI; 70 HCs | Serum | UPLC-Q-TOF/MS | Ceramide; palmitic acid; diacylglycerol; plasmalogen; sphingomyelin |
20 | DeFilippis et al. (37), 2017 | AMI | 11 thrombotic MI; 12 non-thrombotic MI | Plasma | UPLC-MS/MS GC-MS | Corticosterone; 1-palmitoylglycerol; 2-linoleoylglycerol; 2-oleoylglycerol; 2-palmitoylglycerol; 1-linoleoylglycerol; androsterone glucuronide; cortolone-3-glucuronide; dihydrocortisol; pregnenolone sulfate; tetrahydroaldosterone-3-glucuronide; 1-arachidonylglycerol; glutamine |
21 | Zhu et al. (38), 2018 | AMI | 30 MI; 30 HCs | Plasma | UPLC-Q-TOF/MS | Phosphatidylserine; lysoPC(16:0); lysoPC(18:2); lyso-PC (C18:1); C16-sphingosine; N-methyl arachidonic amide; linoleamidoglycerophosphate choline; N-(2-methoxyethyl) arachidonic amide; arachidonic acid; linoleic acid |
22 | Lu et al. (39), 2017 | AMI | 10 HCs; 10 SA; 8 MI | Plasma | UHPLC-Q-TOF/MS | Isoprostanes; oxidized phospholipid; oxidized PL; glycerophospholipid; arachidonic acid; linoleic acid |
23 | Lee et al. (40), 2015 | AMI | 68 MI; 68 HCs | Serum | UPLC-Q-TOF/MS | Proline; arginine; uridine; 2-hydroxyisobutyric acid; 9-decenoylcarnitine; decanoylcarnitine; suberic acid; malic acid; 3-indolepropionic acid; citric acid; azelaic acid; phenylalanyl-phenylalanine; phenylalanyltryptophan; glycocholic acid; cholic acid; L-acetylcarnitine; 2-hydroxy-3-metylbutyric acid; betaine; choline; Inosine; uric acid; hypoxanthine; isoleucine; creatine; phenylalanine |
24 | Holmes et al. (41), 2018 | AMI | 912 MI; 1,146 IS; 1,138 ICH; 1,466 HCs | Plasma | NMR | Trimethylamine; carnosine; glycoprotein acetyls; indol-3-acetate; β-hydroxybutyrate; creatine; docosahexaenoic acid; leucine; histidine; isoleucine; glutamine |
25 | Ward-Caviness et al. (42), 2017 | AMI | 2,257 AMI | Serum | LC-MS | Lysophosphatidylcholine 17:0; lysophosphatidylcholine 18:2; arginine |
26 | McKirnan et al. (43), 2019 | AMI | 10 surgical groups; 6 sham groups | Serum, tissue | GC-TOF/MS | Phosphoethanolamine; Beta-alanine; aminomalonate; stearic acid; 2-hydroxyvaleric acid; palmitoleic; benzoic Acid; succinic acid; threonic acid; glutathione; ethanolamine; cholesterol; dehydroascorbic acid; nicotinamide; taurine; conduritol-beta-epoxide; hydroxycarbamate; isothreitol; methylphosphate; sulfuric acid (Sulfate); phosphate; methionine; alpha-linolenic acid; malic acid; hypoxanthine; urea; phenylalanine; alanine |
27 | Khan et al. (44), 2020 | AMI | 112 AMI; 89 non-risk controls | Serum | HR-MS | Cysteic acid; L-cysteine; t-methylhistidine; L-homocysteine; sulfinic acid; L-cysteine; sulfinic acid; tryptophan |
28 | Huang et al. (45), 2023 | AMI | 103 AMI; 84 STEMI; 19 NSTEMI | Plasma | LC-MS/MS | Betaine; trimethylamine N-oxide |
29 | Lim et al. (46), 2022 | AMI | 101 AMI; 66 HCs | Plasma | LC-MS/MS | Glycerophospholipid |
30 | Xia et al. (47), 2023 | AMI | 59 diabetes AMI; 59 non-diabetes-AMI | Plasma | LC-MS/MS | N-lactoyl-phenylalanine; lysophosphatidylcholines |
31 | Naz et al. (48), 2015 | STEMI | 16 STEMI; 16 NSTEMI 20 STEMI; 26 NSTEMI |
Serum | CE-MS; HILIC-MS | C02-carnitine |
32 | Huang et al. (49), 2018 | STEMI | 44 STEMI (22 LMCAD; 22 non-LMCAD); 22 HCs | Plasma | UPLC-MS/MS | Dehydrophytosphingosine; 9-cis-retinoic acid; 1H-Indole-3-carboxaldehyde; lysophosphatidylcholines |
33 | Gundogdu et al. (50), 2020 | STEMI | 20 STEMI; 15 HCs | Serum | LC-Q-TOF/MS | L-proline; glycerol; lysoPC (18:2(9Z)); propionic acid; butyric acid; succinate; fumaric acid; L-lactic acid; caffeine; phosphatidylethanolamine; glycine; palmitic acid; oleic acid; citrate; taurine; urea; alanine; leucine; isoleucine |
34 | Goulart et al. (51), 2019 | STEMI | 15 STEMI; 15 HCs | Plasma | UPLC-MS/MS and FIA-MS | Phosphatidylcholines; sphingomyelin; lysophosphatidylcholines |
35 | Luo et al. (52), 2022 | STEMI | 42 STEMI patients (21 consecutive VF and 21 non-VF) | Plasma | UPLC-MS/MS | Dehydrophytosphingosine; 9-cis-retinoic acid |
36 | Ali et al. (53), 2016 | STEMI | 30 STEMI;15 UA; 15 HCs | Serum | GC-MS; SPME-GC/MS; 1H-NMR | Pyruvate; lactic acid; maleic acid; uric acid; urea; H2S.; β-hydroxybutyric acid; choline; betaine; citrulline; glycerol |
37 | Chorell et al. (54), 2020 | STEMI | 50 STEMI; 50 NSTEMI; 100 controls | Serum | GC-TOF/MS; LC-TOF/MS | Aspartate; branched-chain amino acids; sphingosine 1-phosphate; lysophospholipids; C02-carnitine; C5iso-carnitine; acylcarnitines; valine; isoleucine |
38 | Luo et al. (55), 2023 | STEMI | STEMI (36 PR; 36 PE) | Plasma | LC-MS | Salicylic acid; proline; docosahexaenoic acid |
39 | Laborde et al. (56), 2014 | NSTEMI | 35 NSTEACS; 35 HCs | Plasma | GC-MS | 5-OH-tryptophan; 2-OH-butyric acid; 3-OH-butyric acid |
40 | Ameta et al. (57), 2016 | UA | 65 UA; 62 HCs | Serum | 1H NMR | Glutamine; alanine; inosine; adenine; valine |
41 | Wang et al. (58), 2019 | UA | 39 UA; 40 HCs | Plasma | UPLC-Q-TOF/MS | Lysophosphatidylcholines; LysoPE |
42 | Yao et al. (59), 2017 | UA | 101 UA;132 HCs | Serum | 1H-NMR | Trimethylamine N-oxide; phosphocholine; isoleucine; valine; lysine; leucine; creatine; glutamate; threonine; 1-methylhistidine; myo-inositol; lactate; high-density lipoprotein; low density lipoprotein; very low-density lipoprotein; total cholesterol; choline; phenylalanine; glycerophosphocholine; 3-hydroxybutyrate; glutamine |
43 | Li et al. (60), 2015 | UA | 27 UA; 20 HCs | Urinary | 1H-NMR | Choline; phenylalanine; citrulline; L-alanine; N-methylnicotinamide; tryptophan; 3-hydroxybutyrate; creatinine; glutamine; lysine |
44 | PouralijanAmiri et al. (61), 2020 | UA | 94 UA; 32 HCs | Plasma | 1H-NMR | 17-hydroxyprogesterone; 2-hydroxyestrone; 2-methoxyestradiol; androstenedione; deoxycorticosterone; etiocholanolone; 2-methoxyestrone; 2-hydroxyestradiol; estradiol |
45 | Sun et al. (62), 2013 | UA | 45 UA; 43 AS | Plasma | RRLC-Q-TOF/MS | Glutamic acid; deoxyribose 1-phosphate; PG(16:0/16:0); PG(20:5(5Z,8Z,11Z,14Z,17Z)/20:1(11Z)); decenedioic acid; MG(0:0/18:2(9Z,12Z)/0:0); MG(0:0/18:3(9Z,12Z,15Z)/0:0); MG(20:5(5Z,8Z,11Z,14Z,17Z)/0:0/0:0); 7,8-dihydropteroic acid; indoxylsulfuric acid; Histidinyl-valine; 18-hydroxycorticosterone; 3b,16a-dihydroxyandrostenone sulfate; choline; phytosphingosine; lactate |
46 | Liu et al. (63), 2022 | UA | 10 UA;10 HCs | Serum | LC-MS | p-Cresol; sulfate; ceramide |
47 | Hao et al. (64), 2024 | UA | 33 UA; 38 MI; 24 HCs | Serum | UPLC-Q-TOF/MS | 2-hydroxyhexanoic acid; medronic acid; butylbenzene sulfonamide; phosphocholine |
ACS, acute coronary syndrome; AMI, acute myocardial infarction; AS, atherosclerosis; CAL, coronary artery ligation; CE-MS, capillary electrophoresis-mass spectrometry; CerP, ceramide phosphate; CPC, chest pain controls; FIA-MS, flow injection analysis-mass spectrometry; GC-MS, gas chromatography mass spectrometry; GC-TOF/MS, gas chromatography-time-of-flight mass spectrometry; HC, health control; HILIC-MS, hydrophilic interaction chromatography-mass spectrometry; HR-MS, high-resolution mass spectrometry; ICH, intracerebral hemorrhage; IS, ischemic stroke; LC-MS, liquid chromatography mass spectrometry; LC-MS/MS, liquid chromatography tandem mass spectrometry; LC-Q-TOF/MS, liquid chromatography-quadrupole time-of-flight mass spectrometry; LMCAD, left main coronary artery disease; lysoPC, lysophosphatidylcholine; lysoPE, lysophosphatidylethanolamine; MG, monoglyceride; MI, myocardial infarction; NMR, nuclear magnetic resonance; NOCAD, non-obstructive coronary artery disease; NSTEACS, Non-ST-segment elevation acute coronary syndrome; NSTEMI, non-ST-segment elevation myocardial infarction; PE, plaque erosion; PG, phosphatidylglycerol; PI, phosphatidylinositol; PL, phospholipid; PR, plaque rupture; RRLC-Q-TOF/MS, rapid resolution liquid chromatography-quadrupole time-of-flight mass spectrometry; SA, stable angina; SP, stable plaque; SPME-GC/MS, solid phase microextraction-gas chromatography/mass spectrometry; STEMI, ST-segment elevation myocardial infarction; UA, unstable angina; UHPLC-Q-TOF/MS, ultra-high performance liquid chromatography-quadrupole time-of-flight mass spectrometry; UPLC-MS, ultra-performance liquid chromatography mass spectrometry; UPLC-MS/MS, ultra-performance liquid chromatography tandem mass spectrometry; UPLC-Q-TOF/MS, ultra-performance liquid chromatography-quadrupole time-of-flight mass spectrometry; VF, ventricular fibrillation; VP, vulnerable plaques.
Table 4
No. | First author, year | Disease | Sample size | Source | Technique | Biomarker |
---|---|---|---|---|---|---|
1 | Vignoli et al. (65), 2019 | AMI | AMI 832 survivors; 146 deceased | Serum | 1H NMR | Valine; 3-hydroxybutyrate; formate; mannose; creatinine; histidine; proline; acetone |
2 | Liu et al. (66), 2022 | AMI | 445 HCs; 347 AMI; 79 AMICVD | Serum | 1H NMR | Glutamine; creatine; threonine; N-acetylphosphinothricin; inosine; myo-inositol; histidine; acetone; alanine; trimethylamine N-oxide |
3 | Vignoli et al. (67), 2020 | AMI | AMI: 702 survivors; 123 deceased | Serum | 1H NMR | Mannose; creatinine |
4 | Surendran et al. (68), 2019 | STEMI | 108 plasma samples from 27 STEMI patients | Plasma | LC-Q-TOF-MS | Linoleoyl carnitine; 1-linoleoylglycerophosphocholine; pentadecanoic acid |
5 | Liu et al. (69), 2021 | STEMI | 48 survivors; 48 non-survivors | Plasma | UPLC-MS | N-acetyl-leukotriene E4; 3-methyl-2-butene-1-thiol; 4-hydroxy-6-docosanone |
6 | Laaksonen et al. (70), 2016 | ACS | Corogene; SPUM-ACS; BECAC | Plasma | Cohort analysis | Ceramide |
7 | Kraler et al. (71), 2023 | ACS | 2,619 ACS | Plasma | Cohort analysis | LDL electronegativity |
8 | Lozhkina et al. (72), 2024 | ACS | 73 ACS; favorable outcome; fatal outcome | Plasma | HPLC-MS/MS | Phosphoribosyl pyrophosphate; 3-hydroxycapric acid; beta-alanine |
9 | Usova et al. (73), 2024 | ACS | 110 ACS | Plasma | UPLC-MS | Ceramide |
ACS, acute coronary syndrome; AMI, acute myocardial infarction; AMICVD, acute myocardial infarction-cardiovascular disease; BECAC, Bergen Coronary Angiography Cohort; HC, health control; HPLC-MS/MS, high performance liquid chromatography-tandem mass spectrometry; LC-Q-TOF-MS, liquid chromatography-quadrupole time-of-flight mass spectrometry; NMR, nuclear magnetic resonance; SPUM-ACS, Special Program University Medicine-Inflammation in Acute Coronary Syndromes; STEMI, ST-segment elevation myocardial infarction; UPLC-MS, ultra-performance liquid chromatography tandem mass spectrometry.
Metabolomics-based diagnostic biomarker analysis of ACS
Currently, the diagnosis of ACS relies on comprehensive analysis of clinical manifestations, electrocardiograms, and myocardial biomarkers of myocardial injury (74). Although the continuous advancement of technology has greatly improved the accuracy of diagnosis, there are still insufficient and certain limitations. Although markers of myocardial injury have good sensitivity, the specificity of creatine kinase-MB (CK-MB) is not strong, and an increase in troponin reflects only myocardial injury (75). If there is no clinical evidence that the injury is related to coronary ischemia, the myocardial injury may be caused by other causes (76). Metabolomics studies can detect a large number of metabolites, and metabolites can also be effective new biomarkers.
For ACS, there have been a number of articles in which valuable diagnostic biomarkers have been identified. In 2017, Yin et al. (18) used a non-targeted metabolomics approach (LC-MS) to identify novel biomarkers from plasma samples from 20 ACS patients and 20 non-ACS patients. Researchers found that phospholipid, glycolysis, and amino acid metabolism are altered in ACS patients compared with non-ACS patients. In 2021, Zhong et al. (19) applied a non-targeted LC-MS approach to plasma metabolomic analysis of 284 ACS patients and 130 healthy controls (HCs) to identify 28 metabolites that could be used to differentiate between the two groups. The receiver operating characteristic (ROC) curve showed that the combination of two metabolites, phosphatidylethanolamine lyso (16:0), and LPC (20:4), into one panel had an area under the curve (AUC) value of 0.905. In the same year, applying ultra-performance liquid chromatography coupled with the time of flight (UPLC-Q-TOF/MS) technique, Song et al. (20) examined serum samples from 45 ACS patients and 29 HCs and identified 46 differentially abundant metabolites. ROC curve analysis revealed that the three metabolites were used as diagnostic biomarkers with AUC values of 0.92–0.93. The diagnostic model constructed with metabolic biomarkers combined with cTNI had a high AUC value of 0.96. In 2024, Shibata et al. (24) also analyzed the metabolomic characteristics of 18 patients with ACS and 24 age-matched healthy volunteers by UPLC-Q-TOF/MS. A multiple logistic regression model for the diagnosis of ACS was established, in which the area under the ROC curve value of the lysine, isocitrate and tryptophan metabolite panel for distinguishing ACS patients from HCs was 1.00.
UA is the earliest type of disease process in ACS. In 2016, Ameta et al. (57) used 1H NMR to analyze serum samples from 65 patients with UA and 62 HCs. It was found that the group of five metabolites, valine, alanine, glutamine, inosine, and adenine, was sufficient to achieve the maximum area under the ROC curve (AUC =0.99). In 2019, Wang et al. (58) examined serum samples of UA, unstable angina-diabetes mellitus (UA-DM), and HCs using the UPLC-Q-TOF/MS technique. First, 27 differential metabolites were identified by comparison of UA and HC. Pathway analysis showed that arginine and proline metabolism, glycerophospholipid metabolism, and purine metabolism were affected in patients with UA. Then, the authors applied metabolomics to detect UA and UA-DM metabolites and identified 22 differential metabolites. ROC analysis showed that six had high specificity and sensitivity for distinguishing UA from UA-DM, with AUC values above 0.85. Pathway analysis suggested that tryptophan metabolism is a key metabolic pathway in patients with UA combined with DM. In 2024, Hao et al. (64) used metabolomics to analyze serum samples from 33 UA, 38 myocardial infarction (MI) and 24 normal controls. The results revealed significant differences between MI and UA in xylene, hydroxycaproic acid, butylbenzene sulfonamide, octanetriol, phosphocholine and medronic acid. Pathway analysis showed that it was related to myocardial hypertrophy, Wnt signaling and fatty acid oxidation.
AMI is a serious threat to human health, because of persistent ischemia and hypoxia in the coronary arteries, which can cause myocardial necrosis and even death. In 2016, Fan et al. (25) applied the liquid chromatography-quadrupole time-of-flight mass spectrometry (LC-Q-TOF/MS) technique to 1,086 blood samples [116 normal coronary artery (NCA), 276 non-obstructive coronary atherosclerosis (NOCA), 63 stable angina (SA), 307 UA, 324 acute myocardial infarction (AMI)] from center 1 for metabolomics. The results of the training set were validated by applying 933 blood samples from center 1 and 71, 68, and 166 blood samples from center 2, center 3, and center 4. The authors focused on the effects of NOCA versus NCA on plaque formation, SA versus NOCA on plaque growth, UA versus SA on the transition from stable to unstable coronary arteries, and AMI versus UA. A total of 89 differential metabolites were identified. Phospholipid catabolism was reduced, amino acid metabolism was increased, short-chain acylcarnitines were increased, the tricarboxylic acid cycle was reduced, and primary bile acid biosynthesis was reduced. The AUC values for the 12 metabolomics-specific biomarker-based experimental groups ranged from 0.938 to 0.996, providing 89.2% to 96.0% predictive values in the test phase and 85.3% to 96.4% predictive values in the 3-center outer set. In 2021, Aa et al. (26) applied GC-MS and LC-MS techniques to identify patients with AMI after the onset of chest pain versus patients with other cardiogenic chest pain (UA, myocarditis, heart valve disease) and combined the results of both methods and found that deoxyuridine (dU), homoserine and methionine could be potential biomarkers for AMI (AUC >0.91).
In the clinical setting, AMI is further classified into STEMI and NSTEMI based on the changes in the ST segment of the electrocardiogram. In 2015, Naz et al. (48) analyzed serum samples from 16 NSTEMI patients and 16 STEMI patients using CE-MS and identified 32 significant metabolites. Then, 13 carnitine-derived compounds and 13 amino acids were targeted and quantified in the serum of 20 STEMI patients and 28 NSTEMI patients using the HILIC-MS technique, and the c02-carnitine levels differed significantly between the two groups with an AUC of 0.85. Because it is crucial to examine left main coronary artery disease (LMCAD) before the occurrence of STEMI or sudden infarction death, in 2018, Huang et al. (49) examined serum samples including 44 STEMI patients (22 consecutive LMCAD and 22 non-LMCAD) using UPLC-MS technology. Fourteen metabolites were significantly different between LMCAD and non-LMCAD. ROC curve analysis showed that 9-cis-retinoic acid (9cRA) was the most important biomarker distinguishing the two groups with an AUC value of 0.888. Subsequently, the authors established a panel of 10 metabolites including 9cRA, dehydrophytosphingosine, 1H-indole-3-carbaldehyde, and 7 variants of lysophosphatidylcholine (LysoPC) with the largest AUC (0.933). In 2023, Luo et al. (55) applied a combination of metabolomics and OCT to detect the difference between plaque rupture (PR) and plaque erosion (PE). The results showed that docosahexaenoic acid (DHA), salicylic acid and proline were identified in both tests. ROC analysis showed that the areas under the curve of these metabolites in the training samples were 0.81, 0.70 and 0.67 respectively. The AUC values of 0.75, 0.73 and 0.74 were verified in the test samples.
Metabolomics-based prognostic biomarker analysis of ACS
The discovery of more novel biomarkers for early prognostic assessment of disease is essential to provide patients with more appropriate treatment strategies and further management (77). The 2023 ESC Management Guidelines emphasize the role of serial measurements of high sensitivity cardiac troponin (hs-cTn) and consideration of changes in plasma brain natriuretic peptide (BNP) or N-terminal pro-B-type natriuretic peptide (NT-proBNP) concentrations in the prognosis of ACS (78). One study found a substantial increase in the maximum chance of death in patients with elevated cardiac troponin T (cTnT) and cardiac troponin I (cTnI) (79). Another study showed that the NT-proBNP level at 6 weeks after ACS diagnosis was an independent predictor of future adverse outcomes. However, the predictive power of these biomarkers currently varies widely across disease endpoints, and the exploration of new prognostic biomarkers with high sensitivity and specificity is urgently needed (80).
Among the retrieved AMI studies, in 2019, Vignoli et al. (65) performed metabolomic analysis of serum samples from 80 surviving patients and 40 deceased patients, and for each serum sample, a nuclear Overhauser effect spectrum (NOESY) one-dimensional 1H-NMR spectrum was obtained. For each patient, a NOESY RF score was derived from the obtained NOESY spectrum, with the “RF risk score” indicating the extent to which the serum metabolomic profile appeared to be similar to that of one of the deceased patients. The metabolic profiles of 80 patients who survived and 40 patients who died were classified using the radio frequency (RF) classifier. The performance of the RF risk scores was compared with the actual results. The ROC analysis revealed an AUC of 0.859 for the NOESY spectrum, and the validation set (106 patients who died and 752 who survived) using the optimized NOESY RF risk score derived from the training set, the AUC value was 0.801. In 2022, Liu et al. (66) collected serum samples from 79 patients with AMI with cardiovascular disease (AMICVD), 28 of whom died within 1 year as the AMI with cardiovascular death (AMICVDD) group. A biomarker panel identifying three metabolites namely TMAO, OAG, and histidine was found to discriminate AMICVDD from AMICVD, and the AUC of this biomarker panel was 0.85 in the validation phase, which was the same as that in the discovery phase.
For NSTEMI and STEMI, there are also a number of previous articles that have identified valuable prognostic biomarkers. In Surendran et al.’s study (68) in 2019, a total of 108 plasma samples from 27 patients with STEMI were examined to identify changes in plasma metabolites during human ischemia/reperfusion (I/R) injury and to find potential plasma biomarkers to assess the extent of myocardial injury after PPCI. The researchers identified a panel of pentanoic acids, carnitine linoleate and choline 1-linoleate glycerophosphate as plasma biomarkers of severity with AUC values of 0.86. In 2021, Liu et al. (69) applied UPLC-MS technology to perform a metabolomic analysis of serum collected from 48 survivors (SSTEMI) and 48 non-survivors (DSTEMI). Twenty-six differential metabolites were identified, seven of which were strongly associated with the occurrence and different outcomes of STEMI. ROC curve analysis revealed multivariate linear support vector machine (SVM) algorithm and generalized estimating equation (GEE) model analysis of the panel consisting of seven metabolites with AUC values of 0.998 and 0.981, respectively.
For prognostic markers of ACS, many other researchers have applied cohort analysis. In 2016, Laaksonen et al. (70) studied the prognostic value of plasma ceramide (Cer) as a marker of cardiovascular death (CV death) in three independent coronary artery disease (CAD) cohorts. This finding was ultimately validated in a prospective Norwegian cohort study of patients with stable CAD, which demonstrated that ceramides, especially when used in proportion, are significantly associated with cardiovascular death. In the Corogene, SPUM-ACS, and BECAC studies, different plasma ceramide ratios were found to be important predictors of cardiovascular death in patients with stable coronary heart disease (CHD) and ACS. In 2023, Kraler et al. (71) recruited data from 2,619 ACS patients, follow-up for 30 days and 1 year, respectively, and mortality endpoints to observe whether changes in low-density lipoprotein (LDL) electronegativity are associated with adverse outcomes in patients with ACS. The results showed that changes in LDL electronegativity were associated with 30-day and 1-year all-cause mortality. It has replaced several risk factors that predict 1-year death.
Application of metabolomics to discover drugs for the prevention and treatment of ACS
ACS is most often caused by obstruction and requires the establishment of acute in-perfusion of blood flow. Currently, reperfusion is mostly achieved clinically with thrombolytic agents or percutaneous coronary intervention (PCI). Despite advances in treatment, the treatment and prognosis of ACS remain unsatisfactory, so new therapeutic approaches and medication targets are urgently needed (81). A list of the therapeutic effects and mechanisms of drugs for ACS are presented in Table 5.
Table 5
No. | First author, year | Disease | Sample size | Source | Medicine | Mechanisms |
---|---|---|---|---|---|---|
1 | Hu et al. (82), 2021 | ACS | 36 ACS-statins; 67 ACS | Serum | Statins | Statins modulated the gut microbiome of ACS patients towards a healthier status, reducing potentially pathogenic bacteria but increasing beneficial bacteria and specific changes in bacterial taxa were associated with disease severity or outcomes either directly or by mediating metabolites such as fatty acids and prenol lipids |
2 | Lam et al. (83), 2016 | AMI | Antibiotic-treated rats; control | Plasma | Antibiotic | Researchers found that antibiotic treatment reduced intestinal microbial metabolites of the amino acids phenylalanine, tryptophan and tyrosine were associated with reduced severity of myocardial infarction |
3 | Liu et al. (84), 2014 | AMI | 6 control; 6 model; 6 positive; 6 XKS | Plasma | XKS | The results suggested that pretreatment of XKS protected metabolic perturbations in rats with MI, major via lipid pathways, amino acid metabolism and purine metabolism |
4 | Wu et al. (85), 2020 | AMI | 11 Sham; 11 Mod; 11 SJP-L; 11 SJP-H | Serum; tissue; urinary | SJP | Researchers indicated that the protective effect of SJP on cardiovascular disease was associated with systemic metabolic modulation, in particular regulation of amino acid and fatty acid metabolism |
5 | Wu et al. (86), 2020 | AMI | 11 Sham; 11 Mod; 11 SBP-L; 11 SBP-H | Serum; tissue; urinary | SBP | This study demonstrated that SBP was effective for protecting cardiac dysfunction by regulating amino acid, lipid and energy metabolisms |
6 | Tan et al. (87), 2012 | AMI | 7 Sham; 6 SND-treated | Urinary | SND | With the altered metabolism pathways as possible drug targets, we systematically analyze the therapeutic effect of SND, which demonstrated that SND administration could provide satisfactory effect on MI through partially regulating the perturbed myocardial energy metabolism |
7 | Yuan et al. (88), 2021 | AMI | 30 ω-3 therapy; 30 usual therapy | Plasma | ω-3 | ω-3 PUFA supplementation may improve lipid metabolism and endothelial function possibly by affecting eicosanoid metabolic status at a systemic level during convalescent healing after AMI |
ACS, acute coronary syndrome; AMI, acute myocardial infarction; PUFA, polyunsaturated fatty acid; SBP-H, Shexiang Baoxin Pill-high; SBP-L, Shexiang Baoxin Pill-low; SJP-H, Sukei Jiuxin Pill-high; SJP-L, Sukei Jiuxin Pill-low; SND, Sini decoction; XKS, Xin-Ke-Su.
Metabolomics-based study of ACS prevention through herbal medicine influenced metabolites
For the treatment of ACS, in addition to some therapeutic drugs, there are also some traditional Chinese medicines that play preventive roles in this disease. Xin-Ke-Su (XKS) is a patented drug that is extensively used in the treatment of ACS. In 2014, Liu et al. (84) established a myocardial infarction model after pretreating mice with different levels of XKS, and metabolomic analysis of serum samples from each group showed that XKS could protect rats from metabolic disorders through lipid pathways, amino acid metabolism, and purine metabolism thus protecting the cardiovascular system.
Using HPLC-Q-TOF-MS/MS technique, Wu et al. (85) examined body fluid samples from different groups of rats, which were rat models of acute myocardial infarction established by ligation of the left anterior descending branch of the coronary artery. This study demonstrated that Sukei Jiuxin Pills (SJP) achieve cardiovascular protection by regulating the metabolism of amino acids and fatty acids in the body. In the same year, Wu’s team applied the same technique to study the action and analysis mechanism of Shexiang Baoxin Pill (SBP), and the results revealed that SBP protects the heart by regulating amino acid, lipid, and energy metabolism (86).
Metabolomics-based study to treat ACS with drug-influenced gut microbial metabolites
According to previous studies, the microbiota and derived microbial compounds of the gut might play a positive role in human metabolism and may be relevant to the pathogenesis of some common metabolic diseases (89). Meanwhile, metabolites associated with gut microbes make important contributions to cardiovascular disease.
Hu et al. (82) applied 16SrRNA and untargeted UPLC-Q-TOF/MS techniques to samples from ACS patients with and without statin therapy to explore the mechanisms by which statins affect key gut microbes and metabolites for cardiovascular protection in ACS patients, and reported that statins modulate bacteria in the gut, and that gut bacteria modulate metabolites such as fatty acids and acrolein lipids to regulate disease severity.
On the other hand, Lam et al. (83) established a rat model in which multiple antibiotics were acted on rats after which feces, blood heart tissue were collected for testing, and the researchers found that antibiotic treatment reduced intestinal microbial metabolites of the amino acids phenylalanine, tryptophan and tyrosine were associated with a reduced severity of myocardial infarction.
Metabolomics-based metabolic pathway analysis of the major differential metabolites of ACS
Metabolic pathway analysis of ACS diagnostic biomarkers
We reviewed 50 articles applying metabolomics to detect diagnostic biomarkers of ACS in the last decade, of which 304 metabolites have been applied as diagnostic biomarkers. These metabolites can be categorized into ten groups, including amino acids, lipids, organic acids, hormones, carbohydrates, hydrocarbons, vitamins, purines, and amines. Lipids accounted for 29% of the metabolites, and can be subdivided into fatty acids (13%), glycerophospholipids (12%), and sphingolipids (4%). Amino acids account for 18% of which peptides account for approximately 4%. Organic acids accounted for 11%. Hormones account for approximately 10% of which steroids account for 6%. The percentages of other types of metabolites are shown in Figure 1. Table 6 shows the metabolites found in ACS-related articles according to metabolite categorization ranked by the number of times they were mentioned in different articles.
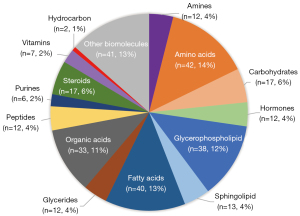
Table 6
Classification | Metabolite | Disease | Reference |
---|---|---|---|
Amines | Choline | AMI, UA | (33,40,53,59,60,62) |
Betaine | AMI, ACS | (29,40,45,53) | |
Trimethylamine oxide | UA, AMI | (45,59) | |
Phosphorylcholine | AMI, ACS | (21,33) | |
Isobutyryl-l-carnitine | ACS, AMI | (21,84) | |
Amino acids | Tryptophan | ACS, UA, AMI | (18,21,24,31,44,60,83,84,87) |
Glutamine | AMI, UA, ACS | (31,33,37,41,57,59,60) | |
Alanin | AMI, STEMI | (21,31,33,43,50,57,87) | |
Isoleucine/leucine | UA, AMI, STEMI | (33,40,41,50,54,59) | |
Phenylalanine | UA, AMI | (33,40,43,59,60) | |
Lysine | AMI, UA | (24,33,59,60) | |
Valine | AMI, UA, STEMI | (33,54,57,59) | |
Histidine | AMI | (33,34,41) | |
Creatine | AMI, UA | (40,41,59) | |
Glutamate | AMI, UA | (33,34,59) | |
Proline | AMI | (40,55) | |
Methionine | AMI | (26,43) | |
Arginine | AMI | (40,42) | |
Glycine | AMI | (50,87) | |
Tyrosine | AMI | (33,83) | |
Carbohydrates | Inosine | STEMI, AMI | (31,40,57) |
Glycerol | AMI, STEMI | (31,50,53) | |
myo-inositol | UA, AMI | (31,59) | |
Organic acids | Lactate | UA, AMI | (33,34,59,87) |
3-hydroxybutyrate | UA, ACS, AMI | (31,59,60) | |
Citrate | AMI | (33,50,87) | |
Pyruvate | AMI | (29,31,53) | |
Citrulline | AMI, UA | (53,60) | |
Hippuric acid | ACS, AMI | (21,87) | |
Malic acid | AMI | (40,43) | |
Succinate | AMI | (50,87) | |
Glycerophospholipid | Lysophosphatidylcholines | STEMI, AMI | (47,49,51,58) |
Glycerophosphocholine | AMI, UA, STEMI | (21,59,86) | |
LysoPC(18:0) | AMI, ACS | (21,69,84) | |
Glycerophospholipid | AMI | (32,39,46) | |
LysoPC(16:0) | AMI | (38,84) | |
LysoPC(18:2) | AMI | (38,84) | |
Sphingolipid | Dehydrophytosphingosine | STEMI, AMI | (49,52,69) |
Sphingomyelin | STEMI, AMI | (36,51) | |
Phytosphingosine | AMI, UA | (62,84) | |
Purines | Hypoxanthine | AMI | (33,40,43) |
Uric acid | AMI | (40,53,84) | |
Caffeine | AMI, ACS | (18,50) | |
Fatty acids | Linoleic acid | AMI | (30,38,39,84) |
Arachidonic acid | AMI | (30,38,39,84) | |
Docosahexaenoic acid | AMI | (41,55,84) | |
Palmitic acid | AMI | (36,50) | |
Oleic acid | ACS, AMI | (18,50) | |
α-linolenic acid | AMI | (30,43) |
ACS, acute coronary syndrome; AMI, acute myocardial infarction; STEMI, ST-segment elevation myocardial infarction; UA, unstable angina.
There are 42 amino acid metabolites of which tryptophan (21%), glutamine (17%), isoleucine (14%), and alanine (17%) have been mentioned several times in several articles. Amino acid metabolites mainly regulate amino acid-related metabolic pathways in the body. Some also regulate the tricarboxylic acid cycle in the body. Tryptophan is associated with UA mainly regulates the tryptophan metabolic pathway in the body, which plays an important role in protein synthesis, endothelium-derived blood pressure control in stroke vessels, and microvascular inflammatory response (31). However, in addition to UA glutamine, isoleucine, and alanine have been associated with STEMI. Patients with AMI have increased serum levels of alanine, glutamine, histidine, valine, and isoleucine, suggesting that the tricarboxylic acid cycle (TCA) cycle is facilitated and may be supplied by these amino acids. Elevated levels of amino acids also indicate disruption of lipoprotein degradation and energy metabolism in patients with acute myocardial infarction (33). A total of 11 metabolites that can be categorized as peptides, including carnosine, histidinyl-valine, glutathione, and phenylalanyl-phenylalanine are mentioned in the article. Among them, phenylalanyl-phenylalanine and phenylalanyl-tryptophan are associated with the lipid peroxidation pathway of AMI (40).
Lipids can be classified as fatty acids, glycerophospholipids, or sphingolipids. Among fatty acids linoleic acid (10%) and arachidonic acid (10%) are mentioned in several articles. Both metabolites are associated with acute infarction and are involved in the fatty acid metabolic pathway of the body. The level of unsaturated fatty acids in the blood of AMI patients suggests that the large amount of lipids accumulating in the blood vessels may increase the risk of atherosclerosis (30). Some pharmacologic studies suggest that omega-3 polyunsaturated fatty acid supplementation during rehabilitation after AMI may improve lipid metabolism and endothelial function by modulating the arachidonic acid metabolic status (88). In addition, LysoPC has been suggested in several articles to be associated with ACS, and LysoPC activates a wide range of cell types in the vascular system, accelerating the evolution and progression of atherosclerosis by inducing cell proliferation, and increasing lymphocyte. Plaque formation and thrombus rupture, which are also related mainly to the ratio of LysoPC to lysophosphatidylethanolamine (LysoPE) in the blood (36). The most mentioned metabolite of sphingosine is dehydrophytosphingosine (17%), which can be used as a diagnostic and prognostic marker for AMI. It attenuates vascular inflammation or oxidative stress through different mechanisms, such as the activation of G protein-coupled receptors or peroxisome proliferator-activated receptors (49).
Thirty metabolites were classified as organic acids, and lactate (12%) and 3-hydroxybutyrate (9%) were identified several times in previous articles. As better diagnostic biomarkers for AMI and STEMI, elevated levels of 3-hydroxybutyrate and lactate consistently indicate impaired fatty acid oxidation, tissue hypoxia, and increased anaerobic glycolysis, and elevated plasma lactate levels in patients with acute myocardial infarction can vary according to the extent of injury (60). The carbohydrate group includes 18 metabolites, of which inosine, Myo-inositol, glucose have been identified in several articles, inositol, and glucose have been associated with AMI and can be used as diagnostic biomarkers of AMI. Serum glucose levels are higher in AMI patients than in control subjects, suggesting accelerated gluconeogenesis and altered anaerobic glycolysis. In addition, the serum levels of inosine, a purine degradation product involved in anaerobic glycolysis, are increased in AMI patients, further confirming the observation of altered anaerobic glycolysis and energy metabolism (33).
Metabolic pathway analysis of ACS prognostic biomarkers
A total of 9 prognostic articles were mentioned in the article, and a total of 25 metabolites were found to be prognostic biomarkers of ACS. These prognostic metabolites can also be categorized into eight groups, including amino acids, lipid, organic acids, carbohydrates, hydrocarbons, and amines. Lipids accounted for 20% of the metabolites. Amino acids accounted for 28%, and organic acids accounted for 12%. The percentages of other types of metabolites are shown in Figure 2. Table 7 shows the metabolites found in ACS-related articles. Among the prognostic markers, the amino acid metabolites with the best proportions were mentioned, and histidine (29%) was mentioned in several articles. Previous study has found that histidine metabolism is closely related to the death outcome of AMI (66). L-histidine (HIS) can be synthesized by histidine decarboxylase. HIS also regulates the expression of histidases, produces uric acid, converts it to imidazoline propionate and hydrolyzes it to formiminoglutamate (FIGLU). The glutamate produced by FIGLU can be used to synthesize glutamine, which is eventually released into the blood. Researchers have reported elevated levels of histidine, glutamate, and glutamine in the event of eventual death from AMI (66).
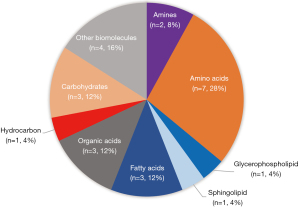
Table 7
Classification | Metabolite | Disease | Reference |
---|---|---|---|
Amines | Linoleoyl carnitine | STEMI | (68) |
TMAO | AMI | (66) | |
Amino acids | Histidine | AMI | (65,66) |
Glutamine | AMI | (66) | |
Alanine | AMI | (66) | |
Valine | AMI | (65) | |
Creatine | AMI | (66) | |
Proline | AMI | (65) | |
Threonine | AMI | (66) | |
Lipids/fatty acids | Acetone | AMI | (65,66) |
Pentadecanoic acid | STEMI | (68) | |
N-Acetyl-leukotriene E4 | STEMI | (69) | |
Organic acids | N-acetylphosphinothricin | AMI | (66) |
3-hydroxybutyrate | AMI | (65) | |
Formate | AMI | (65) | |
Carbohydrates | Mannose | AMI | (65,67) |
Inosine | AMI | (66) | |
Myo-inositol | AMI | (66) | |
Lipid/sphingolipid | Ceramide | ACS | (70,72,73) |
ACS, acute coronary syndrome; AMI, acute myocardial infarction; STEMI, ST-segment elevation myocardial infarction; TMAO, trimethylamine oxide.
The carbohydrate group included 3 prognostic metabolites, and two AMI prognosis-related articles identified mannitol as a potential prognostic marker. Mannose (67%) is responsible for maintaining the steady state of the blood and plays a central role in the glycosylation of lipoproteins involved in the development and progression of atherosclerosis. N-glycans are upregulated in a pro-inflammatory milieu and are found on the surface of endothelial cells during the early stages of atherosclerotic plaque formation. Authors reported that patients at high risk for AMI relatively high levels of mannose, suggesting that mannose binds relatively little to mannose-binding lectin (MBL), which reduces the activation of the lecithin pathway, one of the three modes of complement system activation (67).
Ceramide, a sphingomyelin metabolite among lipid metabolites, was mentioned in three articles as a prognostic marker for ACS. Researchers have reported that ceramides can promote a number of central atherosclerotic processes, including lipoprotein aggregation and uptake, inflammation, superoxide ion production, and apoptosis (70). High ceramide levels have also been found to be associated with cardiac cell death in a mouse model of myocardial infarction. In addition, ceramides can cause vascular dysfunction by inactivating endothelial nitrogen monoxide (NO) synthase (72).
Conclusions and future expectations
In conclusion, ACS is a common CAD, and although researchers have conducted metabolomic studies on ACS and successfully identified potential biomarkers for diagnosis, prognosis, and some pharmacological treatments, current studies have various shortcomings that need improvement.
In current studies on ACS, the maximum sample size collected in experiments is around 1,000 cases, but very few can reach this number, and most experiments have a sample size of a few hundred cases or even a few dozen cases. Therefore, in future studies, we can expand the sample size and collect multicenter samples, which will make the results more convincing. In addition, most of the current studies collected validation groups to verify the specificity and sensitivity of diagnostic and prognostic biomarkers, and several studies on AMI performed targeted metabolomics assays, however, they also targeted only one class of metabolites and did not target previously identified metabolite biomarkers. Therefore, in future studies, targeted metabolomics should be used more over to quantify previously identified valuable metabolites and to provide a more powerful account of the previous studies. Finally, we can also improve the accuracy of metabolite discovery by integrating metabolic data generated by different metabolic technologies, and we can also combine genomics and proteomics technologies to use multi-omics to better understand the regulatory networks among substances, to further understand the biological pathways and mechanisms of diseases.
It is believed that with the continuous advancement of technology and the efforts of millions of researchers, more accurate and specific markers will continue to emerge, leading to greater breakthroughs in the diagnosis and prevention of ACS, thus improving the current status of ACS diagnosis and treatment.
Acknowledgments
We express our gratitude to Wei Sun for his valuable assistance in the selection and writing of this article.
Footnote
Reporting Checklist: The authors have completed the Narrative Review reporting checklist. Available at https://cdt.amegroups.com/article/view/10.21037/cdt-24-431/rc
Peer Review File: Available at https://cdt.amegroups.com/article/view/10.21037/cdt-24-431/prf
Funding: This work was supported by
Conflicts of Interest: All authors have completed the ICMJE uniform disclosure form (available at https://cdt.amegroups.com/article/view/10.21037/cdt-24-431/coif). The authors have no conflicts of interest to declare.
Ethical Statement: The authors are accountable for all aspects of the work in ensuring that questions related to the accuracy or integrity of any part of the work are appropriately investigated and resolved.
Open Access Statement: This is an Open Access article distributed in accordance with the Creative Commons Attribution-NonCommercial-NoDerivs 4.0 International License (CC BY-NC-ND 4.0), which permits the non-commercial replication and distribution of the article with the strict proviso that no changes or edits are made and the original work is properly cited (including links to both the formal publication through the relevant DOI and the license). See: https://creativecommons.org/licenses/by-nc-nd/4.0/.
References
- Surendran A, Atefi N, Zhang H, et al. Defining Acute Coronary Syndrome through Metabolomics. Metabolites 2021;11:685. [Crossref] [PubMed]
- Bergmark BA, Mathenge N, Merlini PA, et al. Acute coronary syndromes. Lancet 2022;399:1347-58. [Crossref] [PubMed]
- Libby P, Pasterkamp G, Crea F, et al. Reassessing the Mechanisms of Acute Coronary Syndromes. Circ Res 2019;124:150-60. [Crossref] [PubMed]
- Vergallo R, Crea F. Atherosclerotic Plaque Healing. N Engl J Med 2020;383:846-57. [Crossref] [PubMed]
- Anderson JL, Morrow DA. Acute Myocardial Infarction. N Engl J Med 2017;376:2053-64. [Crossref] [PubMed]
- Wang L, Jin Y. Noncoding RNAs as Biomarkers for Acute Coronary Syndrome. Biomed Res Int 2020;2020:3298696. [Crossref] [PubMed]
- Olivier M, Asmis R, Hawkins GA, et al. The Need for Multi-Omics Biomarker Signatures in Precision Medicine. Int J Mol Sci 2019;20:4781. [Crossref] [PubMed]
- Bujak R, Struck-Lewicka W, Markuszewski MJ, et al. Metabolomics for laboratory diagnostics. J Pharm Biomed Anal 2015;113:108-20. [Crossref] [PubMed]
- Rosano GM, Fini M, Caminiti G, et al. Cardiac metabolism in myocardial ischemia. Curr Pharm Des 2008;14:2551-62. [Crossref] [PubMed]
- Pouralijan Amiri M, Khoshkam M, Salek RM, et al. Metabolomics in early detection and prognosis of acute coronary syndrome. Clin Chim Acta 2019;495:43-53. [Crossref] [PubMed]
- Zhang F, Li B, Su H, et al. Progress in the Metabolomics of Acute Coronary Syndrome. Rev Cardiovasc Med 2023;24:204. [Crossref] [PubMed]
- Laíns I, Gantner M, Murinello S, et al. Metabolomics in the study of retinal health and disease. Prog Retin Eye Res 2019;69:57-79. [Crossref] [PubMed]
- Wilson I. Methods and techniques for metabolic phenotyping. Bioanalysis 2017;9:1-3. [Crossref] [PubMed]
- Markley JL, Brüschweiler R, Edison AS, et al. The future of NMR-based metabolomics. Curr Opin Biotechnol 2017;43:34-40. [Crossref] [PubMed]
- Gika HG, Theodoridis GA, Plumb RS, et al. Current practice of liquid chromatography-mass spectrometry in metabolomics and metabonomics. J Pharm Biomed Anal 2014;87:12-25. [Crossref] [PubMed]
- Marshall DD, Powers R. Beyond the paradigm: Combining mass spectrometry and nuclear magnetic resonance for metabolomics. Prog Nucl Magn Reson Spectrosc 2017;100:1-16. [Crossref] [PubMed]
- Frédérich M, Pirotte B, Fillet M, et al. Metabolomics as a Challenging Approach for Medicinal Chemistry and Personalized Medicine. J Med Chem 2016;59:8649-66. [Crossref] [PubMed]
- Yin X, de Carvalho LP, Chan MY, et al. Integrated metabolomics and metallomics analyses in acute coronary syndrome patients. Metallomics 2017;9:734-43. [Crossref] [PubMed]
- Zhong W, Deng Q, Deng X, et al. Plasma Metabolomics of Acute Coronary Syndrome Patients Based on Untargeted Liquid Chromatography-Mass Spectrometry. Front Cardiovasc Med 2021;8:616081. [Crossref] [PubMed]
- Song L, Zhang Z, Qiu Z, et al. Serum Metabonomic Study of Patients With Acute Coronary Syndrome Using Ultra-Performance Liquid Chromatography Orbitrap Mass Spectrometer. Front Cardiovasc Med 2021;8:637621. [Crossref] [PubMed]
- Wei H, Gu J, Jiang X, et al. Anxiety disturbs the blood plasma metabolome in acute coronary syndrome patients. Sci Rep 2021;11:12897. [Crossref] [PubMed]
- Fu M, He R, Zhang Z, et al. Multinomial machine learning identifies independent biomarkers by integrated metabolic analysis of acute coronary syndrome. Sci Rep 2023;13:20535. [Crossref] [PubMed]
- Qiu G, Lin Y, Ouyang Y, et al. Nontargeted Metabolomics Revealed Novel Association Between Serum Metabolites and Incident Acute Coronary Syndrome: A Mendelian Randomization Study. J Am Heart Assoc 2023;12:e028540. [Crossref] [PubMed]
- Shibata M, Sugimoto M, Watanabe N, et al. Exploring Novel Biomarkers for an Acute Coronary Syndrome Diagnosis Utilizing Plasma Metabolomics. Int J Mol Sci 2024;25:6674. [Crossref] [PubMed]
- Fan Y, Li Y, Chen Y, et al. Comprehensive Metabolomic Characterization of Coronary Artery Diseases. J Am Coll Cardiol 2016;68:1281-93. [Crossref] [PubMed]
- Aa N, Lu Y, Yu M, et al. Plasma Metabolites Alert Patients With Chest Pain to Occurrence of Myocardial Infarction. Front Cardiovasc Med 2021;8:652746. [Crossref] [PubMed]
- Martin-Lorenzo M, Zubiri I, Maroto AS, et al. KLK1 and ZG16B proteins and arginine-proline metabolism identified as novel targets to monitor atherosclerosis, acute coronary syndrome and recovery. Metabolomics 2015;11:1056-67. [Crossref] [PubMed]
- Xu X, Gao B, Guan Q, et al. Metabolomic profile for the early detection of coronary artery disease by using UPLC-QTOF/MS. J Pharm Biomed Anal 2016;129:34-42. [Crossref] [PubMed]
- Jiang H, Jiang H, Zhang J, et al. The Serum Metabolic Biomarkers in Early Diagnosis and Risk Stratification of Acute Coronary Syndrome. Front Physiol 2020;11:776. [Crossref] [PubMed]
- Guo M, Fan X, Tuerhongjiang G, et al. Targeted metabolomic analysis of plasma fatty acids in acute myocardial infarction in young adults. Nutr Metab Cardiovasc Dis 2021;31:3131-41. [Crossref] [PubMed]
- Petras M, Kalenska D, Samos M, et al. NMR plasma metabolomics study of patients overcoming acute myocardial infarction: in the first 12 h after onset of chest pain with statistical discrimination towards metabolomic biomarkers. Physiol Res 2020;69:823-34. [Crossref] [PubMed]
- Li J, Duan W, Wang L, et al. Metabolomics Study Revealing the Potential Risk and Predictive Value of Fragmented QRS for Acute Myocardial Infarction. J Proteome Res 2020;19:3386-95. [Crossref] [PubMed]
- Deng D, Liu L, Xu G, et al. Epidemiology and Serum Metabolic Characteristics of Acute Myocardial Infarction Patients in Chest Pain Centers. Iran J Public Health 2018;47:1017-29. [PubMed]
- Wang X, Wang D, Wu J, et al. Metabolic Characterization of Myocardial Infarction Using GC-MS-Based Tissue Metabolomics. Int Heart J 2017;58:441-6. [Crossref] [PubMed]
- Zhang L, Wei TT, Li Y, et al. Functional Metabolomics Characterizes a Key Role for N-Acetylneuraminic Acid in Coronary Artery Diseases. Circulation 2018;137:1374-90. [Crossref] [PubMed]
- Park JY, Lee SH, Shin MJ, et al. Alteration in metabolic signature and lipid metabolism in patients with angina pectoris and myocardial infarction. PLoS One 2015;10:e0135228. [Crossref] [PubMed]
- DeFilippis AP, Trainor PJ, Hill BG, et al. Identification of a plasma metabolomic signature of thrombotic myocardial infarction that is distinct from non-thrombotic myocardial infarction and stable coronary artery disease. PLoS One 2017;12:e0175591. [Crossref] [PubMed]
- Zhu M, Han Y, Zhang Y, et al. Metabolomics Study of the Biochemical Changes in the Plasma of Myocardial Infarction Patients. Front Physiol 2018;9:1017. [Crossref] [PubMed]
- Lu J, Chen B, Chen T, et al. Comprehensive metabolomics identified lipid peroxidation as a prominent feature in human plasma of patients with coronary heart diseases. Redox Biol 2017;12:899-907. [Crossref] [PubMed]
- Lee J, Jung Y, Park JY, et al. LC/MS-based polar metabolite profiling reveals gender differences in serum from patients with myocardial infarction. J Pharm Biomed Anal 2015;115:475-86. [Crossref] [PubMed]
- Holmes MV, Millwood IY, Kartsonaki C, et al. Lipids, Lipoproteins, and Metabolites and Risk of Myocardial Infarction and Stroke. J Am Coll Cardiol 2018;71:620-32. [Crossref] [PubMed]
- Ward-Caviness CK, Xu T, Aspelund T, et al. Improvement of myocardial infarction risk prediction via inflammation-associated metabolite biomarkers. Heart 2017;103:1278-85. [Crossref] [PubMed]
- McKirnan MD, Ichikawa Y, Zhang Z, et al. Metabolomic analysis of serum and myocardium in compensated heart failure after myocardial infarction. Life Sci 2019;221:212-23. [Crossref] [PubMed]
- Khan A, Choi Y, Back JH, et al. High-resolution metabolomics study revealing l-homocysteine sulfinic acid, cysteic acid, and carnitine as novel biomarkers for high acute myocardial infarction risk. Metabolism 2020;104:154051. [Crossref] [PubMed]
- Huang S, Lim SY, Tan SH, et al. Targeted Plasma Metabolomics Reveals Association of Acute Myocardial Infarction Risk with the Dynamic Balance between Trimethylamine-N-oxide, Betaine, and Choline. J Agric Food Chem 2023;71:15097-105. [Crossref] [PubMed]
- Lim SY, Lim FLS, Criado-Navarro I, et al. Multi-Omics Investigation into Acute Myocardial Infarction: An Integrative Method Revealing Interconnections amongst the Metabolome, Lipidome, Glycome, and Metallome. Metabolites 2022;12:1080. [Crossref] [PubMed]
- Xia JG, Li B, Zhang H, et al. Precise Metabolomics Defines Systemic Metabolic Dysregulation Distinct to Acute Myocardial Infarction Associated With Diabetes. Arterioscler Thromb Vasc Biol 2023;43:581-96. [Crossref] [PubMed]
- Naz S, Calderón ÁA, García A, et al. Unveiling differences between patients with acute coronary syndrome with and without ST elevation through fingerprinting with CE-MS and HILIC-MS targeted analysis. Electrophoresis 2015;36:2303-13. [Crossref] [PubMed]
- Huang L, Zhang L, Li T, et al. Human Plasma Metabolomics Implicates Modified 9-cis-Retinoic Acid in the Phenotype of Left Main Artery Lesions in Acute ST-Segment Elevated Myocardial Infarction. Sci Rep 2018;8:12958. [Crossref] [PubMed]
- Gundogdu G, Senol O, Demirkaya Miloglu F, et al. Serum metabolite profiling of ST-segment elevation myocardial infarction using liquid chromatography quadrupole time-of-flight mass spectrometry. Biomed Chromatogr 2020;34:e4738. [Crossref] [PubMed]
- Goulart VAM, Santos AK, Sandrim VC, et al. Metabolic Disturbances Identified in Plasma Samples from ST-Segment Elevation Myocardial Infarction Patients. Dis Markers 2019;2019:7676189. [Crossref] [PubMed]
- Luo J, Shaikh JA, Huang L, et al. Human Plasma Metabolomics Identify 9-cis-retinoic Acid and Dehydrophytosphingosine Levels as Novel biomarkers for Early Ventricular Fibrillation after ST-elevated Myocardial Infarction. Bioengineered 2022;13:3334-50. [Crossref] [PubMed]
- Ali SE, Farag MA, Holvoet P, et al. A Comparative Metabolomics Approach Reveals Early Biomarkers for Metabolic Response to Acute Myocardial Infarction. Sci Rep 2016;6:36359. [Crossref] [PubMed]
- Chorell E, Olsson T, Jansson JH, et al. Lysophospholipids as Predictive Markers of ST-Elevation Myocardial Infarction (STEMI) and Non-ST-Elevation Myocardial Infarction (NSTEMI). Metabolites 2020;11:25. [Crossref] [PubMed]
- Luo X, Liu M, Wang S, et al. Combining metabolomics and OCT to reveal plasma metabolic profiling and biomarkers of plaque erosion and plaque rupture in STEMI patients. Int J Cardiol 2023;390:131223. [Crossref] [PubMed]
- Laborde CM, Mourino-Alvarez L, Posada-Ayala M, et al. Plasma metabolomics reveals a potential panel of biomarkers for early diagnosis in acute coronary syndrome. Metabolomics 2014;10:414-24. [Crossref] [PubMed]
- Ameta K, Gupta A, Ameta D, et al. 1H NMR-derived metabolomics of filtered serum of myocardial ischemia in unstable angina patients. Clin Chim Acta 2016;456:56-62. [Crossref] [PubMed]
- Wang J, Xu W, Zhao H, et al. Identification of potential plasma biomarkers and metabolic dysfunction for unstable angina pectoris and its complication based on global metabolomics. Biosci Rep 2019;39:BSR20181658. [Crossref] [PubMed]
- Yao W, Gao Y, Wan Z. Serum Metabolomics Profiling to Identify Biomarkers for Unstable Angina. Biomed Res Int 2017;2017:7657306. [Crossref] [PubMed]
- Li Z, Liu X, Wang J, et al. Analysis of urinary metabolomic profiling for unstable angina pectoris disease based on nuclear magnetic resonance spectroscopy. Mol Biosyst 2015;11:3387-96. [Crossref] [PubMed]
- PouralijanAmiri M. NMR-based plasma metabolic profiling in patients with unstable angina. Iran J Basic Med Sci 2020;23:311-20. [PubMed]
- Sun M, Gao X, Zhang D, et al. Identification of biomarkers for unstable angina by plasma metabolomic profiling. Mol Biosyst 2013;9:3059-67. [Crossref] [PubMed]
- Liu L, Luo F. Alterations in the fecal microbiota and serum metabolome in unstable angina pectoris patients. Front Biosci (Landmark Ed) 2022;27:100. [Crossref] [PubMed]
- Hao Y, Wen W, Gao Y, et al. Distinct metabolic profiles and pathway alterations in myocardial infarction and unstable angina revealed by metabolomics. Clin Chim Acta 2024;562:119853. [Crossref] [PubMed]
- Vignoli A, Tenori L, Giusti B, et al. NMR-based metabolomics identifies patients at high risk of death within two years after acute myocardial infarction in the AMI-Florence II cohort. BMC Med 2019;17:3. [Crossref] [PubMed]
- Liu W, Zhang L, Shi X, et al. Cross-comparative metabolomics reveal sex-age specific metabolic fingerprints and metabolic interactions in acute myocardial infarction. Free Radic Biol Med 2022;183:25-34. [Crossref] [PubMed]
- Vignoli A, Tenori L, Giusti B, et al. Differential Network Analysis Reveals Metabolic Determinants Associated with Mortality in Acute Myocardial Infarction Patients and Suggests Potential Mechanisms Underlying Different Clinical Scores Used To Predict Death. J Proteome Res 2020;19:949-61. [Crossref] [PubMed]
- Surendran A, Aliani M, Ravandi A. Metabolomic characterization of myocardial ischemia-reperfusion injury in ST-segment elevation myocardial infarction patients undergoing percutaneous coronary intervention. Sci Rep 2019;9:11742. [Crossref] [PubMed]
- Liu J, Huang L, Shi X, et al. Clinical Parameters and Metabolomic Biomarkers That Predict Inhospital Outcomes in Patients With ST-Segment Elevated Myocardial Infarctions. Front Physiol 2021;12:820240. [Crossref] [PubMed]
- Laaksonen R, Ekroos K, Sysi-Aho M, et al. Plasma ceramides predict cardiovascular death in patients with stable coronary artery disease and acute coronary syndromes beyond LDL-cholesterol. Eur Heart J 2016;37:1967-76. [Crossref] [PubMed]
- Kraler S, Wenzl FA, Vykoukal J, et al. Low-density lipoprotein electronegativity and risk of death after acute coronary syndromes: A case-cohort analysis. Atherosclerosis 2023;376:43-52. [Crossref] [PubMed]
- Lozhkina NG, Gushchina OI, Basov NV, et al. Ceramides As Potential New Predictors of the Severity of Acute Coronary Syndrome in Conjunction with SARS-CoV-2 Infection. Acta Naturae 2024;16:53-60. [Crossref] [PubMed]
- Usova E, Yakovlev A, Kopanitsa G, et al. Prognostic Value of Ceramide Dynamics in Patients with Acute Coronary Syndrome. Stud Health Technol Inform 2024;321:175-9. [Crossref] [PubMed]
- Bhatt DL, Lopes RD, Harrington RA. Diagnosis and Treatment of Acute Coronary Syndromes: A Review. JAMA 2022;327:662-75. Erratum in: JAMA 2022;327:1710. [Crossref] [PubMed]
- Collet JP, Thiele H, Barbato E, et al. 2020 ESC Guidelines for the management of acute coronary syndromes in patients presenting without persistent ST-segment elevation. Eur Heart J 2021;42:1289-367. [Crossref] [PubMed]
- Babuin L, Jaffe AS. Troponin: the biomarker of choice for the detection of cardiac injury. CMAJ 2005;173:1191-202. [Crossref] [PubMed]
- Lindahl B. Acute coronary syndrome - the present and future role of biomarkers. Clin Chem Lab Med 2013;51:1699-706. [Crossref] [PubMed]
- Byrne RA, Rossello X, Coughlan JJ, et al. 2023 ESC Guidelines for the management of acute coronary syndromes. Eur Heart J 2023;44:3720-826. [Crossref] [PubMed]
- Heidenreich PA, Alloggiamento T, Melsop K, et al. The prognostic value of troponin in patients with non-ST elevation acute coronary syndromes: a meta-analysis. J Am Coll Cardiol 2001;38:478-85. [Crossref] [PubMed]
- Eggers KM, Lagerqvist B, Venge P, et al. Prognostic value of biomarkers during and after non-ST-segment elevation acute coronary syndrome. J Am Coll Cardiol 2009;54:357-64. [Crossref] [PubMed]
- Eisen A, Giugliano RP, Braunwald E. Updates on Acute Coronary Syndrome: A Review. JAMA Cardiol 2016;1:718-30. [Crossref] [PubMed]
- Hu X, Li H, Zhao X, et al. Multi-omics study reveals that statin therapy is associated with restoration of gut microbiota homeostasis and improvement in outcomes in patients with acute coronary syndrome. Theranostics 2021;11:5778-93. [Crossref] [PubMed]
- Lam V, Su J, Hsu A, et al. Intestinal Microbial Metabolites Are Linked to Severity of Myocardial Infarction in Rats. PLoS One 2016;11:e0160840. [Crossref] [PubMed]
- Liu YT, Jia HM, Chang X, et al. Metabolic pathways involved in Xin-Ke-Shu protecting against myocardial infarction in rats using ultra high-performance liquid chromatography coupled with quadrupole time-of-flight mass spectrometry. J Pharm Biomed Anal 2014;90:35-44. [Crossref] [PubMed]
- Wu G, Zhong J, Chen L, et al. Effects of the Suxiao Jiuxin pill on acute myocardial infarction assessed by comprehensive metabolomics. Phytomedicine 2020;77:153291. [Crossref] [PubMed]
- Wu G, Chen L, Gu Y, et al. Exploring the mechanism underlying the cardioprotective effect of shexiang baoxin pill on acute myocardial infarction rats by comprehensive metabolomics. J Ethnopharmacol 2020;259:113001. [Crossref] [PubMed]
- Tan G, Liao W, Dong X, et al. Metabonomic profiles delineate the effect of traditional Chinese medicine sini decoction on myocardial infarction in rats. PLoS One 2012;7:e34157. [Crossref] [PubMed]
- Yuan M, Zhang Y, Hua T, et al. Omega-3 polyunsaturated fatty acid supplementation improves lipid metabolism and endothelial function by providing a beneficial eicosanoid-pattern in patients with acute myocardial infarction: A randomized, controlled trial. Clin Nutr 2021;40:445-59. [Crossref] [PubMed]
- Fan Y, Pedersen O. Gut microbiota in human metabolic health and disease. Nat Rev Microbiol 2021;19:55-71. [Crossref] [PubMed]