Pathogenesis of thrombosis: cellular and pharmacogenetic contributions
Introduction
Thrombosis is defined as the formation of a blood clot (thrombus) within a blood vessel (1). This process is generally considered pathologic except in the case of traumatic injury, where thrombus formation may prevent loss of blood volume and protect against systemic infection. A rudimentary description of the factors contributing to thrombosis is attributed to Rudolf Virchow. A modern interpretation of this “Virchow’s triad” is that hemodynamic disruption, intrinsic hypercoagulability, and endothelial damage or dysfunction are the three broad categories that lead to thrombosis (Figure 1) (2).
When the balance of the body’s pro- and anti-thrombotic forces becomes disturbed, it is often necessary to treat pharmacologically in order to restore homeostasis. In the hemophiliac, this means administration of pro-thrombotics such as recombinant activated factor VII (rFVIIa), while in the patient with atrial fibrillation, an anti-thrombotic such as warfarin is required. These agents may act directly or indirectly on the coagulation cascade, platelets, or other components to achieve their effect. That effect, like in so much of pharmacology, may be influenced by the individual’s genetic make-up, and in some cases those genetics may seriously alter the drug’s effect. Here, we will discuss drugs with pro- and anti-thrombotic effects, their mechanisms of action, and the influence of genetic polymorphisms on their effect.
Pro- and anti-thrombotic pharmacology and genetic influences
Pro-thrombotics
Drugs that intentionally encourage coagulation are often concentrates of coagulation cascade proteins, such as rFVIIa, which contains activated factor VII; prothrombin complex concentrates (PCC), which contain factors II, IX, X and sometimes VII; and fresh frozen plasma (FFP), which contains all the coagulation factors (3). These may be administered to patients with a coagulation disorder or to patients suffering from massive bleeding due to trauma or an overdose of an anti-thrombotic. By their nature, these drugs increase the risk of thrombosis, but genetic variation in drug response has not been explored.
More common are the drugs that unintentionally encourage coagulation, which is often an undesirable side effect of the drug (Table 1). Such drugs are numerous, but classic examples are oral contraceptives (OCPs) and chemotherapy agents.
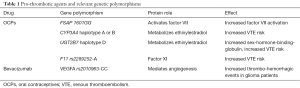
Full table
Oral contraceptives containing an estrogen and a progestogen increase the risk of venous thromboembolism (VTE) via increased levels of thrombin and fibrin, as well as the levels of almost all coagulation factors (4,5). Plasma from patients taking OCPs is also resistant to the anticoagulation effect of activated protein C, an independent risk factor for VTE (4). OCPs also increase the levels of factor VII-activating protease (FSAP), which activates factor VII, thus initiating the extrinsic path of the coagulation cascade (6). In OCP-taking individuals with the 1601GG genotype of the FSAP gene, FSAP levels and consequent factor VII activation were higher than in the 1601GA genotype (6). Genetic variation in the metabolism of a component of combined oral contraceptives, ethinylestradiol, has been linked with increased VTE risk. Possession of the A or B CYP3A4 haplotype or having two copies of the D UGT2B7 haplotype increased VTE risk in patients on OCPs but not in those not taking OCPs (5). The UGT2B7 haplotype D also increased the levels of sex-hormone-binding-globulin, a marker of VTE risk. Another study of OCPs found that possession of the A allele of the F11 rs2289252 polymorphism—a variant in factor XI associated with VTE in the general population—increased VTE risk (7).
Thalidomide has returned to the marketplace, this time for treatment of multiple myeloma. Though its precise mechanism of action remains unclear, it appears to exert its effect both on the malignant cell as well as the microenvironment (8). Unfortunately, it increases the risk of VTE, perhaps due to effects on COX-2 or cytokines. SNPs that may affect risk of VTE in thalidomide-treated patients include those involved in response to DNA damage, cytokine balance, and several genes involved in apoptosis (8). Polymorphisms in the coagulation cascade did not appear to be associated with thalidomide-associated VTE.
Bevacizumab is a recombinant humanized monoclonal neutralizing antibody targeting vascular endothelial growth factor (VEGF) and is used in a variety of malignancies (9). It has also been associated with an increased risk of thromboembolic events (9,10). Among glioma patients, those treated with bevacizumab had increased incidence of ischemic stroke, but the same rates of deep vein thrombosis (DVT) (10). Possession of the CC genotype of the rs2010963 SNP on the vascular endothelial growth factor A (VEGFA) gene in glioma patients was associated with an increase in thrombo-hemorrhagic events during treatment with bevacizumab (11). In a xenograft mouse model of human lung tumors, bevacizumab appeared to exert its pro-thrombotic effect through blocking VEGF’s inhibition of plasminogen activator inhibitor (PAI-1), thus increasing PAI-1 expression and increasing risk of thrombosis (9).
Anti-thrombotics
Pharmacologic anticoagulation is more commonly seen than pro-coagulation (Table 2), and foremost among the anticoagulants is warfarin. Despite increasing prevalence of the direct oral anticoagulants (DOACs), warfarin remains the most commonly prescribed anticoagulant. A classic example of pharmacogenetics is warfarin and its interactions with two genes: cytochrome P450 2C9 (CYP2C9) and vitamin K epoxide reductase (VKORC1) (12). Single nucleotide polymorphisms (SNPs) in these genes alter the effectiveness of warfarin. Warfarin acts by inhibiting VKORC1, which is responsible for the reduction of vitamin K (13). Without the reductase, vitamin K is unable to be utilized by the vitamin K-dependent clotting factors: factors II, VII, IX, and X. Warfarin thus indirectly inhibits the clotting cascade via its inhibition of the reductase, resulting in anticoagulation.
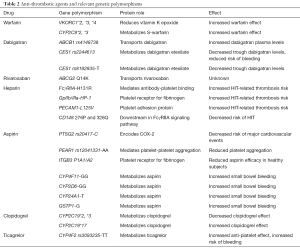
Full table
Warfarin is notorious for its dose variability, and depending on the patient population, genetic factors may account for up to 45% of this variability (14). There are two key genetic polymorphisms that influence warfarin’s effect. The first are polymorphisms in a gene encoding a subunit of vitamin K epoxide reductase, VKORC1. The second are polymorphisms in the cytochrome P450 (CYP) responsible for the metabolism of warfarin, CYP2C9. Polymorphisms in both these structures result in increased sensitivity to warfarin, and hence increased risk for severe bleeding (15). The VKORC1*2 allele is a marker of warfarin sensitivity in 93% of patients (16). Like other alleles *3 and *4, VKORC1*2 results in decreased VKORC1 expression, lower protein production, and thus an inflated effect of warfarin. Two common CYP2C9 variants, *2 and *3, are both the result of single amino acid substitutions and reduce the metabolism of the more potent enantiomer S-warfarin by 30–50% (*2) and 90% (*3), and thus increase the duration of warfarin’s effect (13,16). Those patients with VKORC1 and CYP2C9 polymorphisms that increased their sensitive to warfarin were shown to benefit more from edoxaban treatment as compared with warfarin than normal responders (17).
Direct oral anticoagulants
The DOACs are relatively new anticoagulants that act either by directly inhibiting thrombin in the case of dabigatran, or by directly inhibiting activated factor X, as in the case of rivaroxaban, apixaban, and edoxaban (15). Due to their recent introduction, there is less data on the influence of genetics on DOAC efficacy, but genetic variability does seem to play a role. All DOACs are substrates of the transport protein P-glycoprotein (P-gp), encoded by ABCB1 (18). Variations in P-gp expression have been seen in individuals with SNPs in exon 21 and exon 26 of ABCB1. Possession of the ABCB1 rs4148738 polymorphism (in linkage disequilibrium with rs1045642 on exon 26) resulted in higher peak dabigatran plasma concentrations than those without the polymorphism (18). Rivaroxaban requires both P-gp and breast cancer resistance protein (BCRP, encoded by ABCG2) for absorption and excretion (17). A polymorphism of ABCG2, Q141K, reduces activity of BCRP, resulting in reduced transport of its substrates, though this has not yet been studied in the case of rivaroxaban (17). However, in mouse models, lack of P-gp and BCRP greatly reduced clearance of rivaroxaban.
Dabigatran etexilate, a pro-drug, is converted to its active metabolite by esterases, including CES1. CYPs do not play a role. Individuals with the CES1 polymorphism rs2244613 had 15% lower trough dabigatran concentrations and reduced risk of bleeding (15,19). Possession of the T variant of the CES1 SNP rs8192935 also lowered trough dabigatran concentrations (20).
Less than 4% of edoxaban is metabolized by cytochrome enzymes, primarily hydrolysis via CES1 (17). Given that polymorphisms in CES1 affect dabigatran serum levels, these polymorphisms may also affect edoxaban, though such studies have not been conducted, and edoxaban does not require bioactivation.
Unlike dabigatran and edoxaban, rivaroxaban and apixaban are heavily metabolized by CYPs, and like many drugs, CYP3A4 plays a large role (14). Two-thirds of rivaroxaban is metabolized by CYPs, most prominently CYP3A4 and CYP2J2 (17). Although none of the 44 polymorphisms of CYP3A4 or the 10 polymorphisms of CYP2J2 have been studied in relation to rivaroxaban, these polymorphisms have been associated with variability in enzyme activity level, suggesting possible effect on rivaroxaban metabolism (17).
Like rivaroxaban, apixaban is also metabolized by CYP3A4, and CYP3A5 plays an important role too, as well as CYP1A2 and CYP2J2 to lesser extents (17). The conjugation of the inactive apixaban metabolite, O-demethyl apixaban, to another inactive product is performed by SUT1A1, of which three major genotypes with differing enzymatic activity exist (17). Again, no studies exist as to the possible influence of these polymorphisms on apixaban, but reduced activity of a downstream reaction has the potential to influence the metabolism of apixaban.
Heparin
The mainstay of inpatient anticoagulation is heparin, and few complications are as feared as heparin-induced thrombocytopenia (HIT). This mass activation of platelets and consequent widespread thrombosis is due to an immune response involving IgG antibodies targeted against complexes of platelet factor 4 and heparin, and can occur in patients receiving unfractionated or low molecular weight heparin (21,22). HIT has a mortality of greater than 30% and occurs in 0.5–5% of patients treated with heparin (21). Using genetic variability to predict patients likely to develop HIT is thus of great interest. Some promising genes include SNPs at HLA-DRA, an area already associated with several immune-mediated adverse drug reactions, and TDAG8, which plays a role in activation-induced apoptosis of T cells and attenuation of immune-mediated cytokine production (21). Alleles associated with an increased risk of thrombosis in HIT include the polymorphisms FcγRIIA-H131R, encoding an antibody binding protein; GpIIb/IIIa-HP-1, which encodes the platelet receptor for fibrinogen; and PECAM1-L125V, encoding a platelet adhesion protein (23). The development of thrombosis in individuals with HIT and the FcγRIIA-H131R polymorphism may be due to more efficient activation of platelets in homozygous RR individuals (22). Particularly key for the development of HIT is the FcγRIIA receptor, the only Fc receptor on platelets, which mediates the interaction between platelets and antibodies and when stimulated activates platelets (22). A membrane protein tyrosine phosphatase involved in downstream signaling of the FcγRIIA receptor, CD148 polymorphisms 276P and 326Q were associated with decreased risk of HIT, suggesting a less efficient FcγRIIA signaling pathway in individuals with these alleles (22).
Anti-platelet therapy
Another standard of anticoagulation is anti-platelet therapy, most commonly represented by aspirin and clopidogrel. One of the most popular drugs in the world, aspirin renders platelets non-functional by irreversibly inhibiting platelet cyclooxygenase (primarily COX-1), responsible for the ultimate production of thromboxane A2, an activator of the coagulation cascade (17). Despite exerting its primary effect on COX-1, aspirin also affects COX-2, and variations in the PTSG2 gene that encodes COX-2 may play a role in variability of response to aspirin. Possession of the rs20417 C allele of PTSG2 conferred a decreased risk of major cardiovascular events, which was particularly significant in aspirin users (17). Another clinically significant polymorphism is that on the platelet endothelial aggregation receptor 1 gene, PEAR1, important for signaling in platelet-platelet aggregation. Reduced aggregation in response to both collagen and epinephrine was observed in aspirin-taking patients with the rs12041331 minor AA allele, present in 8–28% of populations, as compared with GG or GA genotypes (17). Finally, possession of the P1A1/A2 SNP on the ITGB3 gene, which encodes the IIIa subunit of the GPIIb/IIIa receptor, has been linked with reduced efficacy of aspirin in healthy subjects, but not in those with cardiovascular disease (17).
A side effect of aspirin therapy is increased bleeding risk, particularly gastrointestinal. Small bowel bleeding associated with low dose aspirin therapy was found to be more common patients with the GG homo-genotypes of CYP4F11 or CYP2D6, those with the T allele of CYP24A1, or the G allele of GSTP1 (24).
Clopidogrel irreversibly inhibits the P2Y12 purinergic receptor on platelets, preventing stimulation by adenosine diphosphate, thus inhibiting platelet aggregation (25). Clopidogrel is a pro-drug, of which approximately 15% is metabolized by a variety of CYPs in a two-step process to form the active metabolite. CYP2C19 plays key roles in both steps, while CYP3A4 is essential for the second step. Polymorphisms in the genes encoding these enzymes may result in altered clopidogrel efficacy. CYP2C19 has two reduced function alleles—CYP2C19*2 and *3—formed via single nucleotide polymorphisms of guanine to adenine (25). The *17 variant is associated with increased gene transcription and thus increased enzyme activity. Clinically, presence of a *2 or *3 allele results in reduced metabolism of CYP2C19 drugs, including clopidogrel, while presence of a *17 allele results in enhanced metabolism. For a pro-drug such as clopidogrel, this means that individuals with a *2 or *3 allele will have lower plasma concentrations of the active metabolite, and hence require a higher dose for therapeutic effect, while those with a *17 allele will have higher plasma concentrations of the active metabolite, and require a lower dose of clopidogrel. Polymorphisms in a variety of other enzymes have been suggested to influence clopidogrel efficacy, but the CYP2C19 variants remain the most clinically relevant at the present time (25).
Alternatives to clopidogrel include ticagrelor, which binds reversibly to P2Y12 receptor on platelets, and is not a pro-drug, hence requiring no activation by hepatic enzymes. Like clopidogrel, however, ticagrelor also appears to have some genetic influencers, such as the CYP4F2 rs3093235 TT variant, which was associated with an increased anti-platelet effect and increased risk of bleeding (26).
Systems approaches to elucidating pharmacogenetics of thrombosis
We have discussed the current state of pharmacogenetics for pro- and anti-thrombotic agents. This field has become an indispensable part of the clinical toolkit in managing patients with coagulation disorders. The DNA sequence alone, however, does not explain the entire story and consequently does not fully predict patients’ responses. This is especially true for underrepresented populations in clinical trials, comorbid conditions, and polypharmacy (27). In other words, for the vast majority of drugs and patients, we receive little guidance from pharmacogenetics today.
To improve the state of the practice and move toward a precision medicine ideal, we must move beyond biological reductionism and pursue a systems approach—one where physiological networks of biomolecules are taken into account. Computational methods are now revealing the deep functional organization and emergent behavior of human biology (28,29). These advances are also opening doors to applying systems and network biology tools to pharmacology, providing novel insights into drug purposing and safety (30-32).
Some early work in this field has been applied to the pathogenesis of thrombosis. A topological analysis of platelet signaling, coagulation, and resolution has shed some light on the complex mechanisms of some traditional Chinese medicines (33). Comprehensive functional enrichment and pathway analysis has elucidated a link between thrombosis and myeloproliferative disorders, reinforcing Virchow’s triad model and indicating that some drugs approved for myeloproliferative disorders could be repurposed to treat thrombosis (34). Protein-protein interaction (PPI) network analysis followed by pathway enrichment analysis identified interleukin-6 and other immunoregulatory proteins as having a key role in inflammation-driven atherosclerosis (35). This approach could suggest potential drug targets for alleviating thrombosis due to endothelial dysfunction.
Going forward, we suggest expanding investigation of published datasets using newer network biology techniques. Tools like NetDecoder and P-Map could facilitate the development of new molecular entities, combination therapies, and repurposing of existing pharmaceuticals (36,37). Context-specific, prioritized biological networks and gene activities, as well as phenotype mapping, are essential for understanding the disease process deeper than Virchow ever imagined. We envision that these systems pharmacology methods could complement or even supplant current pharmacogenetics strategies to serve as complementary prognostics and diagnostics for therapeutic decision making.
Acknowledgements
Funding: This work was supported in part by an NIH/NIGMS training grant to the Medical Scientist Training Program at the Mayo Clinic (T32GM65841).
Footnote
Conflicts of Interest: The authors have no conflicts of interest to declare.
References
- Aird WC. Vascular bed-specific thrombosis. J Thromb Haemost 2007;5 Suppl 1:283-91. [Crossref] [PubMed]
- Esmon CT. Basic mechanisms and pathogenesis of venous thrombosis. Blood Rev 2009;23:225-9. [Crossref] [PubMed]
- Bertina RM. The role of procoagulants and anticoagulants in the development of venous thromboembolism. Thromb Res 2009;123 Suppl 4:S41-5. [Crossref] [PubMed]
- Ramot Y, Nyska A. Drug-induced thrombosis--experimental, clinical, and mechanistic considerations. Toxicol Pathol 2007;35:208-25. [Crossref] [PubMed]
- Stegeman BH, Vos HL, Helmerhorst FM, et al. Genetic variation in the first-pass metabolism of ethinylestradiol, sex hormone binding globulin levels and venous thrombosis risk. Eur J Intern Med 2017;42:54-60. [Crossref] [PubMed]
- Sidelmann JJ, Skouby SO, Kluft C, et al. Plasma factor VII-activating protease is increased by oral contraceptives and induces factor VII activation in-vivo. Thromb Res 2011;128:e67-72. [Crossref] [PubMed]
- Suchon P, Al Frouh F, Ibrahim M, et al. Genetic risk factors for venous thrombosis in women using combined oral contraceptives: update of the PILGRIM study. Clin Genet 2017;91:131-6. [Crossref] [PubMed]
- Johnson DC, Corthals S, Ramos C, et al. Genetic associations with thalidomide mediated venous thrombotic events in myeloma identified using targeted genotyping. Blood 2008;112:4924-34. [Crossref] [PubMed]
- Chen N, Ren M, Li R, et al. Bevacizumab promotes venous thromboembolism through the induction of PAI-1 in a mouse xenograft model of human lung carcinoma. Mol Cancer 2015;14:140. [Crossref] [PubMed]
- Seidel C, Hentschel B, Simon M, et al. A comprehensive analysis of vascular complications in 3,889 glioma patients from the German Glioma Network. J Neurol 2013;260:847-55. [Crossref] [PubMed]
- Di Stefano AL, Labussiere M, Lombardi G, et al. VEGFA SNP rs2010963 is associated with vascular toxicity in recurrent glioblastomas and longer response to bevacizumab. J Neurooncol 2015;121:499-504. [Crossref] [PubMed]
- Dean L. Warfarin Therapy and the Genotypes CYP2C9 and VKORC1. In: Pratt V MH, Dean L, et al. editors. Medical Genetics Summaries [Internet]. Bethesda (MD): National Center for Biotechnology Information (US), 2016.
- Mousa SA. Pharmacogenomics in thrombosis. Methods Mol Biol 2010;663:277-89. [Crossref] [PubMed]
- Lentz SR. Genetic testing to guide warfarin dosing: Impact of direct oral anticoagulants. Clin Pharmacol Ther 2016;100:128-30. [Crossref] [PubMed]
- Baker WL, Johnson SG. Pharmacogenetics and oral antithrombotic drugs. Curr Opin Pharmacol 2016;27:38-42. [Crossref] [PubMed]
- Mazur-Bialy AI, Zdebska K, Wypasek E, et al. Repeated bleeding complications during therapy with vitamin K antagonists in a patient with the VKORC1*2A and the CYP2C9*3/*3 alleles: genetic testing to support switching to new oral anticoagulants. Thromb Res 2013;131:279-80. [Crossref] [PubMed]
- O'Connor CT, Kiernan TJ, Yan BP. The genetic basis of antiplatelet and anticoagulant therapy: A pharmacogenetic review of newer antiplatelets (clopidogrel, prasugrel and ticagrelor) and anticoagulants (dabigatran, rivaroxaban, apixaban and edoxaban). Expert Opin Drug Metab Toxicol 2017;13:725-39. [Crossref] [PubMed]
- Gouin-Thibault I, Delavenne X, Blanchard A, et al. Interindividual variability in dabigatran and rivaroxaban exposure: contribution of ABCB1 genetic polymorphisms and interaction with clarithromycin. J Thromb Haemost 2017;15:273-83. [Crossref] [PubMed]
- Pare G, Eriksson N, Lehr T, et al. Genetic determinants of dabigatran plasma levels and their relation to bleeding. Circulation 2013;127:1404-12. [Crossref] [PubMed]
- Dimatteo C, D'Andrea G, Vecchione G, et al. Pharmacogenetics of dabigatran etexilate interindividual variability. Thromb Res 2016;144:1-5. [Crossref] [PubMed]
- Karnes JH, Cronin RM, Rollin J, et al. A genome-wide association study of heparin-induced thrombocytopenia using an electronic medical record. Thromb Haemost 2015;113:772-81. [Crossref] [PubMed]
- Rollin J, Pouplard C, Gruel Y. Risk factors for heparin-induced thrombocytopenia: Focus on Fcgamma receptors. Thromb Haemost 2016;116:799-805. [Crossref] [PubMed]
- Pamela S, Anna Maria L, Elena D, et al. Heparin-induced thrombocytopenia: the role of platelets genetic polymorphisms. Platelets 2013;24:362-8. [Crossref] [PubMed]
- Shiotani A, Murao T, Fujita Y, et al. Novel single nucleotide polymorphism markers for low dose aspirin-associated small bowel bleeding. PLoS One 2013;8:e84244. [Crossref] [PubMed]
- Amin AM, Sheau Chin L, Azri Mohamed Noor D, et al. The personalization of clopidogrel antiplatelet therapy: the role of integrative pharmacogenetics and pharmacometabolomics. Cardiol Res Pract 2017;2017:8062796. [PubMed]
- Tatarunas V, Kupstyte N, Zaliunas R, et al. The impact of clinical and genetic factors on ticagrelor and clopidogrel antiplatelet therapy. Pharmacogenomics 2017;18:969-79. [Crossref] [PubMed]
- Shah RR. Pharmacogenetics in drug regulation: promise, potential and pitfalls. Philos Trans R Soc Lond B Biol Sci 2005;360:1617-38. [Crossref] [PubMed]
- Barabasi AL, Oltvai ZN. Network biology: understanding the cell's functional organization. Nat Rev Genet 2004;5:101-13. [Crossref] [PubMed]
- Ma’ayan A. Introduction to network analysis in systems biology. Science signaling 2011;4:tr5. -tr. [Crossref] [PubMed]
- Zhao S, Iyengar R. Systems pharmacology: network analysis to identify multiscale mechanisms of drug action. Annu Rev Pharmacol Toxicol 2012;52:505-21. [Crossref] [PubMed]
- Danhof M. Systems pharmacology – Towards the modeling of network interactions. Eur J Pharm Sci 2016;94:4-14. [Crossref] [PubMed]
- Trame MN, Biliouris K, Lesko LJ, et al. Systems pharmacology to predict drug safety in drug development. Eur J Pharm Sci 2016;94:93-5. [Crossref] [PubMed]
- Kong X, Zhou W, Wan JB, et al. An integrative thrombosis network: visualization and topological analysis. Evid Based Complement Alternat Med 2015;2015:265303. [PubMed]
- Jha PK, Vijay A, Sahu A, et al. Comprehensive Gene expression meta-analysis and integrated bioinformatic approaches reveal shared signatures between thrombosis and myeloproliferative disorders. Sci Rep 2016;6:37099. [Crossref] [PubMed]
- Nair J, Ghatge M, Kakkar VV, et al. Network analysis of inflammatory genes and their transcriptional regulators in coronary artery disease. PLoS One 2014;9:e94328. [Crossref] [PubMed]
- da Rocha EL, Ung CY, McGehee CD, et al. NetDecoder: a network biology platform that decodes context-specific biological networks and gene activities. Nucleic Acids Res 2016;44:e100. [Crossref] [PubMed]
- Cairns J, Ung CY, da Rocha EL, et al. A network-based phenotype mapping approach to identify genes that modulate drug response phenotypes. Sci Rep 2016;6:37003. [Crossref] [PubMed]