Artificial intelligence in personalized cardiovascular medicine and cardiovascular imaging
Why we need artificial intelligence (AI) in cardiovascular medicine
Healthcare systems around the world are facing a plethora of new pressures; most notably, an increase in chronic and more complex diseases such as cardiovascular disease (CVD), the leading cause of mortality globally (1). As healthcare shifts away from population-based care to more patient-centered approaches, there is also a concomitant shift in the management of disease processes. One aspect is a greater emphasis on precision medicine: an emerging healthcare model that factors in individual variability in genes, environment and lifestyles, of particular relevance in CVD (2,3).
This is important given the current climate in cardiovascular medicine where we generate enormous amounts of heterogenous data, including ‘omics’ data (genomics, proteomics etc.), high resolution medical imaging data, biosensors, wearables, continuous physiologic metrics and electronic health records (EHR) (4) (Figure 1). This ‘big data’ holds immense potential for the use of sophisticated analysis by AI and machine learning (ML) in three aspects of cardiovascular medicine (5). Firstly, for clinicians it holds promise for more accurate, streamlined and standardised image interpretation, improved diagnosis, risk prediction and better guidance on best treatment options for different stages of disease processes. Secondly, for health systems AI has potential for improved workflow, reduction in medical errors and better patient outcomes. Thirdly, for patients who increasingly process more of their own data, an opportunity to further educate and promote primary and secondary cardiovascular health prevention (6,7).
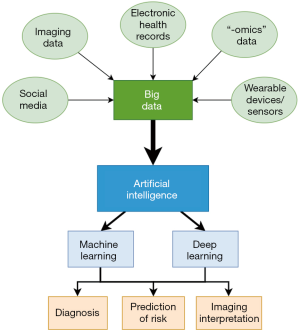
Understanding AI
Despite its widespread use, AI remains a broadly defined term in the literature. AI can be categorised into narrow and general AI. While we are still far from attaining general AI, which is the ability for machines to be self-sufficient and have cognitive function on par with human ability, narrow AI is being increasingly employed in various industries with increased uptake in healthcare, pharmaceuticals and biotechnology (8). Notable examples include using this technology in identifying new drugs and repurposing existing medications (9). Narrow AI can perform specific, applied tasks, and includes techniques such as ML to enable pattern recognition, generate automated predictions and interpret imaging data (10) (Figure 2).
ML
ML is part of applied AI, which is able to discover patterns within data by linking inputs to outputs, learning from the data using a learning model (11). It encompasses most AI applications within medicine. ML can be categorised into unsupervised, supervised and reinforcement learning (8). Supervised learning involves using labelled data to generate outcomes using new data (predictive); unsupervised learning involves using unstructured data to generate relationships between variables using deep learning (DL) (12). Reinforcement learning focuses on reward-based learning with positive and negative feedback and decision making based on prior experiences (7) (Table 1).
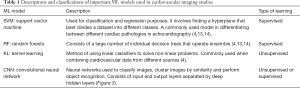
Full table
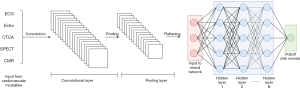
DL
DL is a sub-category of unsupervised learning where data is inputted into multilayer neural networks that yield final outputs (12). Neural networks can detect non-linear relationships between the input variables and outcomes using hidden layer combinations of functions. While in ML, an algorithm must be programmed before analysing data, DL can analyse datasets without prior human programming. Because DL is an extension of these neural networks, it enables greater abstraction and prediction with the discovery of more complex relationships between variables (13,14). Neural networks are often evaluated through receiver operating characteristic (ROC) curves (a plot of true positives rates against false positive rates) where the Area Under the Curve (AUC) is used to express accuracy (Table 1).
Applying AI to cardiovascular medicine
Of the many different aspects of AI in cardiovascular medicine, its application in non-imaging and imaging modalities routinely used to investigate disease holds the greatest potential (4). AI attempts to address issues relating to timing, efficiency, missed diagnosis and poor workflow (5). It offers the potential for earlier detection of disease, improved diagnostic accuracy and more accurate prediction of prognosis and disease severity to better guide optimal management (2). The democratisation of AI into every day wearable technology is further adding a new wave of lower cost, more accurate and easily accessible screening tools (15,16). Moreover, patterns and relationships previously not appreciated without ML could offer new insight into known disease processes and uncover new treatment therapies. The application of AI to imaging and non-imaging modalities in cardiovascular medicine are explored.
Electrocardiography
The electrocardiogram (ECG) has proved a valuable data source for ML studies, with algorithms dating back to the 1960s (15). By applying various ML tools, we are beginning to gather new insights into structural heart disease, rhythm disturbances, phenotypic demographics and electrolyte disturbances from low-cost, routine ECGs (Table 2). Attia et al. used 12 lead ECG data from 44,959 patients to develop a convolutional neural network (CNN) to detect patients with ventricular dysfunction; the algorithm was tested on 52,870 patients and the results were promising, achieving an area under the curve (AUC) of 0.93 (17). However, the limitations of the study include the low positive predictive value of 33.8%, owing to the threshold of an ejection fraction of less than 30% (17). Another study used 12 lead ECG data from 126,526 patients to develop a CNN to recognize patients with atrial fibrillation whilst still in sinus rhythm. When multiple ECGs for each patient were analysed, the algorithm was able to identify atrial fibrillation with an AUC of 0.90, a sensitivity of 82.3% and specificity of 83.4% (18). It is hypothesised structural heart changes such as myocyte hypertrophy, fibrosis and dilatation often precede atrial fibrillation and it was these structural changes that the CNN were able to identify in the ECG. However, the study is not without its limitations; the neural network was trained via retrospective classification on a population with higher incidences of atrial fibrillation so there may be a risk of over-fitting and the patient group with no atrial fibrillation may have undetected atrial fibrillation, and therefore those patients could have been labelled incorrectly, affecting algorithm accuracy. Nevertheless, the study did highlight that a low-cost, accessible and non-invasive test could be used to screen for patients with possible atrial fibrillation as a preventative and diagnostic tool (18). Further studies have explored this notion of detecting structural heart changes from routine ECGs, including detecting left ventricular hypertrophy using AI algorithms demonstrating an AUC of 0.87 when tested on over 5,000 patients (19).
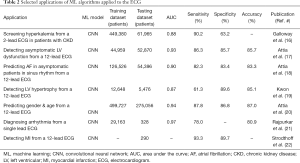
Full table
Attia et al. further found sex and age estimate could be elicited from a 12-lead ECG, where the estimated age may in fact serve as a physiological marker for a patient’s overall health status. The development of a CNN on data from 499,727 patients had an AUC of 0.94 and accuracy of 87.0% for classifying sex. When estimating age, the CNN overpredicted age when certain co-morbidities such as a low ejection fraction, hypertension or coronary artery disease were present (20). However, the study highlights the shortcomings of neural networks and ML, including the concept of the ‘black box’, where there is an inability to explain how and which features of the ECG caused the algorithm to produce certain outputs. This is often due to the relationships between multiple variables being non-linear and intricate that makes precise analysis difficult (20).
Studies demonstrating algorithmic performance that exceed a cardiologist are particularly interesting. A 2017 study by Rajpurkar et al. trained a 34-layer CNN to detect 12 arrhythmia classifications from 29,163 patients, and was tested on 336 records against six board-certified cardiologists, yielding an aggregate positive predictive value of 0.80 compared to 0.72 in cardiologists and aggregate sensitivity of 0.78 compared to 0.72 in cardiologists (21). More recently, Hannun et al. in 2019 developed a deep neural network to detect 12 arrythmia classifications from a single-lead ECG using a training dataset from 53,549 patients, which was compared against the gold standard cardiologist consensus committee diagnoses with an AUC of 0.91 or higher in all rhythm classifications, not only outperforming the cardiologists but achieving higher AUCs for arrhythmias such as atrial fibrillation, atrioventricular block and ventricular tachycardia (23). Both studies only investigated single-lead ECGs, therefore limiting the applicability of the algorithm to 12-lead ECGs. Moreover, the labelling of the data beforehand by a technician and a cardiologist may have affected the reproducibility of the findings given that there was still some degree of uncertainty in the correct label. Any future application of an algorithm must undergo further levels of training and pre-processing (23). Further exploratory studies have demonstrated the ability of DL algorithms to detect myocardial infarctions with a sensitivity of 93.3% and specificity of 89.7%, a similar level of performance when compared to human cardiologists (22). Other studies have also shown the utility of applying deep convolutional neural network models to ECGs in noninvasively screening for hyperkalaemia in patient with chronic kidney disease through wearable technology (16) (Table 2).
Echocardiography
Echocardiography aids diagnosis and management of a multitude of cardiovascular conditions; the process however is resource intensive and requires interpretation by a trained professional (24). The data generated by echocardiograms are often complex and are usually sieved by the interpreter into what is immediately clinically useful. There are other additional features within the echocardiographic data, such as quantification of cardiac structures with diagnostic or prognostic value, which could be unearthed using ML techniques (25). The evidence base for the use of ML within echocardiography remains encouraging (Table 3).
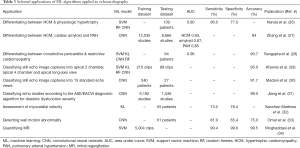
Full table
The use of ML to automate the classification of functional and morphological cardiac features using two-dimensional (2D) echocardiography has been promising. Narula et al. in 2016, used a ML learning framework to differentiate between hypertrophic cardiomyopathy and physiological hypertrophy in 139 athletes using speckle-tracking echocardiographic data (26). The ML model showed sensitivity of 96.0% and specificity of 77.0% and AUC of 0.80 with highest diagnostic value seen at end systole (26). Given the small and specific sample size used, further studies on more heterogeneous populations would need to be conducted on larger samples to improve generalisability (26). A further large retrospective study of 8,666 echocardiograms showed a high accuracy in using convolutional neural networks to identify hypertrophic cardiomyopathy (AUC, 0.93), cardiac amyloid (AUC, 0.87) and pulmonary arterial hypertension (AUC, 0.85) (27). Moreover, Sengupta et al. used an associative memory-based ML algorithm to distinguish between constrictive pericarditis and restrictive cardiomyopathy using speckle-tracking echocardiography data from 94 patients, achieving an AUC of 96.2% (28). With a small sample size and without separate training and testing datasets, the algorithm runs the risk of over-fitting. Therefore, it would be difficult to apply these algorithms beyond a research setting; more validation studies with larger training and testing datasets would be needed (28).
Automated functional assessment of echocardiograms with discrimination between the three apical views: apical two-chamber, apical four-chamber and apical long-axis, can be challenging. A 2017 study by Khamis et al. used spatio-temporal feature extraction and supervised learning to distinguish these different views using 309 clinical clips; accuracies of 97%, 91% and 97% for apical two-chamber, apical four-chamber and apical long-axis, respectively, were achieved (29). The use of DL with the development of a CNN by Madani et al. in 2018 to classify 15 standard views on echocardiography based on images and videos from 267 transthoracic echocardiograms capturing real world variation, yielded 91.7% test accuracy without over-fitting (30). One can argue methods such as occlusion testing and saliency mapping used by Madani et al. can help mitigate the limitations of ‘black-box’ algorithms. Moreover, the issue of clinical implications such as cost-effectiveness is addressed, with the study showing that cost-savings were made by down-sampling (reducing image size without losing accuracy) (30).
A recent prospective study by Jiang et al. in 2019 evaluated the use of 4-layer neural network to classify 7,728 studies according to the 2016 American Society of Echocardiography (ASE)/European Association of Cardiovascular Imaging (EACVI) diagnostic algorithm for diastolic dysfunction severity by echocardiography, to then create a single neural network to output a continuous diastolic function score (31). The neural network reclassified the studies with 99% accuracy (31).
Computed tomography coronary angiography (CTCA)
The use of CTCA imaging to investigate coronary artery disease (CAD) proves an accurate, non-invasive tool in clinical decision making. The use of CTCA in conjunction with ML could prove useful in improving diagnostic accuracy and predicting prognostic events (35). The use of ML in prognostic risk assessment was evaluated by Motwani et al. to predict 5-year all-cause mortality in 10,030 patients, who underwent CTCA with 25 clinical and 44 CTCA parameters assessed and compared against the Framingham risk score (FRS) and CTCA severity scores (36). The model consisted of feature selection before generating predictive classifiers using an ensemble classification approach and 10-fold cross validation; the ML technique had an AUC of 0.79, higher than the FRS (0.61) and CTCA severity scores (0.63) for prediction of 5-year all-cause mortality (36). However, limitations include the manual selection of variables for the ML performance, possible selection bias when using observational data and not all variables determining 5-year all-cause mortality were used (36).
A later study by van Rosendael et al. also utilised data from The Coronoary CT Angiography Evaluation For Clinical Outcomes International Multicenter (CONFIRM) registry, a multicenter, observational cohort prospectively collecting clinical, procedural and follow-up data, to create a ML risk score for CAD using standard 16 coronary segment sclerosis and composition information from CTCA readings (37). A boosted ensemble algorithm was used with discrimination of events better for the ML algorithm achieving an AUC of 0.77 compared to 0.69–0.70 in other scores (37). However, the data derived from the CONFIRM registry may introduce selection bias; the algorithm should be tested on an external study population to limit overfitting and to validate it further (37).
The use of ML, using feature selection then model building using a boosted ensemble algorithm and ten-fold cross validation, to predict lesion-specific ischaemia by invasive fractional flow reserve (FFR) was conducted by Dey at el. from quantitative plaque measurements from CTCA, on 254 patients (38). They attained an AUC of 0.84 which was higher than individual CTCA measurements (38). A recent study in 2019 by von Knebel Doeberitz et al. assessed the use of CTCA derived plaque markers in conjunction with ML derived CT-derived fractional flow reserve (CT-FFR), a validated alternative to invasive FFR, to predict major cardiac adverse events (MACE) compared to CTCA derived plaque markers alone; the use of additional ML derived CT-FFR had an AUC of 0.94 with higher discriminatory power for MACE prediction (39). The use of ML derived FFR could allow rapid functional assessment of ischemic lesions and risk assessment, but it still needs to be validated on external cohorts using larger sample sizes to achieve clinical implementation (39).
Mannil et al. investigated the use of texture analysis, a method objectively quantifying texture of radiological images using interpixel relationships, and ML to detect myocardial infarction (MI) on non-contrast enhanced low radiation dose CTCA images on 87 patients (40). Two models were used; model I distinguished between controls, acute MI and chronic MI, and model II between controls and cases (40). Model I achieved a sensitivity of 69.0% and specificity of 85.0%, model II achieved a sensitivity of 86.0%, specificity of 81% and an AUC of 0.78 (40). These models were compared to two independent readers with 4 years’ experience in cardiology, and they were unable to detect any signs of MI on the imaging (40). This does suggest the ability for ML to surpass a radiologist’s ability to detect abnormalities hidden from the trained eye. Despite this possibility, akin to most cases of ML, overfitting of data remains a large obstacle with selection bias, and in this particular study, the small study sample is a major limitation (40).
Cardiac nuclear imaging
Nuclear imaging such as myocardial perfusion single-photon emission computed tomography (SPECT) is a common non-invasive stress imaging for coronary artery disease (CAD). Because of variation in clinical presentations of patients with CAD who often present with multiple comorbidities, interpretation of SPECT imaging is challenging (41). ML is being used to help improve the accuracy of diagnosing CAD. Betancur et al. used 1,638 patients without known CAD to develop CNNs for predicting CAD (DL) compared to total perfusion deficit (TPD), achieving an AUC of 0.80 (DL) compared to 0.78 (TPD), per-patient sensitivity of 82.3% (DL) compared to 79.8% (TPD), and per-vessel sensitivity of 69.8% (DL) compared to 64.4% (TPD). However, improvements in the model could be made by using a larger training dataset, and limitations of the study include the visual interpretation of the degree of stenosis rather than quantitative assessment (42).
Improving detection of CAD was also investigated by Arsanjani et al., by combining quantitative perfusion and functional variables from SPECT, using a ML algorithm on data collected from 957 patients (43). The performance of the algorithm was compared to visual segmentation scoring by two experienced readers, the diagnostic accuracy achieved by the algorithm was 86.0% with AUC of 0.92, better than TPD (accuracy of 81.0% and AUC of 0.90) and interpretation by two readers (accuracy of 84% and AUC of 0.87 and 0.88) (43). The study highlighted that the diagnostic accuracy of MPS may be employing ML (43). The population had suspected but not known CAD; in medicine, diagnoses may be made with an element of uncertainty depending on clinical evaluation, but when developing a ML algorithm, this ambiguity is not taken into account. Moreover, the studies had limited number of feature classifications, limiting the algorithms diagnostic accuracy in different patient populations.
Cardiac magnetic resonance (CMR) imaging
In CMR, ML has been applied to functional indices, particularly measuring left ventricular function, relevant for conditions such as myocardial hypertrophy, myocardial ischemia and heart failure (44). A study by Luo et al. utilised a DL convolutional neural network for 1140 patients for left ventricle volume, end-diastolic volume (EDV), end-systolic volume (ESV) and ejection fraction (EF) estimation (44). Accuracy was tested using a linear regression fit (y=ax+b, ideally a=1, b=0), with accuracies of y=0.91x+11.7 for ESV, y=0.97x+9.5 for EF and y=0.87+0.2 for EDV (44). The use of automated and ML-powered left ventricle volume prediction shows promise in being used clinically following, albeit after more studies on larger datasets (44).
A study by Bernard et al. used the “Automatic Cardiac Diagnosis Challenge” dataset (ACDC), the largest publicly available and fully annotated dataset for CMR, to assess how well DL algorithms from 10 prior studies can carry out CMR assessment via segmentation and classification of pathologies (45). Results showed that DL attained a mean correlation score of 0.97 for extraction of clinical indices and an accuracy of 0.96 for automatic diagnosis (45). The results do suggest that automated CMR analysis could be achieved and implemented into clinical practice. However, the study does highlight the concept of a ‘diagnostic black-box’ where there is doubt regarding how the algorithm makes decisions, which would be an obstacle in clinical practice as a diagnostic summary should be accompanied with a report detailing the clinical reasoning (45).
Not only can CMR data be used for automated functional assessment, but a study by Dawes et al. shows that data on cardiac motion can be been used to predict patient survival via features of right ventricular failure in pulmonary hypertension (46). The study used CMR scans from 256 patients with recently diagnosed pulmonary hypertension and supervised learning was used to estimate which 3-dimensional systolic motion patterns correlated best with prediction of survival. It achieved an AUC of 0.73 compared to 0.60 in conventional imaging and haemodynamic, functional and clinical markers alone (46).
The role of the EHR
The transition to EHR has resulted in an increase in the volume of complex patient data. The datasets collected, although large and patient-specific, are often scattered and potentially disorganized, including multiple variables ranging from medications, laboratory values, imaging results, physiological measurements and history notes. Therefore, generating a predictive algorithm may prove difficult using traditional models, such as logistical regression. However, ML may thus, offer a way to understand the complex relationships between the complex variables within EHR datasets (7) (Table 4).
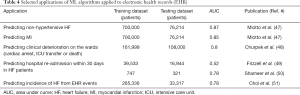
Full table
Miotto et al. used unsupervised deep feature learning to capture relationships in aggregated EHR datasets of 700,000 patients. The ML algorithm had an AUC of 0.87 in predicting which patients would go on to develop non-hypertensive heart failure and an AUC of 0.85 in predicting which patients who had a MI (47). The algorithms’ major limitations where it could not process laboratory test results and the ‘black box’ phenomenon. A 2016 multicentre trial compared ML models with logistical regression models in predicting adverse outcomes in hospitals, such as cardiac arrest, intensive care unit transfer, or death (48). Using demographic variables, laboratory values and vital signs from the EHR of 269,999 patients, the ML algorithm was able to detect adverse events with an AUC of 0.80 compared to 0.77 in linear predictors and the Modified Early Warning Score (MEWS) of 0.70 (48). However, the data was collected from five Illinois hospitals, which limits generalisability to other demographic groups. Additionally, a standardised framework was used and not all available ML methods were evaluated (48).
The use of EHR to predict the risk of readmission within 30 days in patients with heart failure was evaluated by Frizzell et al., using ML (least-absolute shrinkage and selection operator, random forest and gradient boosted) compared with traditional logistical regression, but only yielded a C-statistic ranging from 0.59 to 0.62 (49). The study suggested limited applicability of ML algorithms for the predictive value of 30-day readmission for patients with heart failure (49). However, a more recent study by Shameer et al. also used ML to predict 30-day readmission rates in a single centre cohort of 1,068 patients using variables from EHR, such as diagnosis codes, medications, laboratory measurements, surgical procedures and vital signs (50). The first step involved classification of cases into ‘re-admitted’ and ‘not re-admitted’ before using a correlation-based feature selection method to combine features predictive of re-admission; after training and testing, an AUC of 0.78 was achieved with accuracy of 83.2%. Replication of similar methods on larger datasets could have promising results in using ML for risk prediction (50). Similar to other studies, the algorithm would benefit from being used in multiple sites and applying the study to a larger cohort and using a systematic method for feature selection could improve robustness.
Choi et al. investigated the use of events in EHR to generate a recurrent neural network to predict the incidence of heart failure in patients with data from 3,884 heart failure cases and 28,903 controls, compared against regularized logistic regression, neural network, support vector machine, and K-nearest neighbor classifier approaches (51). A 12-month observation window was used and yielded an AUC 0.78 compared to logistical regression AUC of 0.75; this increased further to an AUC of 0.833 when using an 18-month observation (51).
Limitations and challenges
Despite the promise of AI in cardiovascular medicine, there remain many challenges to date. These can be divided into limitations relating to existing studies, pitfalls with the technology and challenges in the clinical translational ability of current AI techniques.
Many studies thus far employ ‘narrow’ AI use-cases, where the context of utilising ML is clearly defined with variables pre-selected by clinicians. Hence, much of the ML is based on past medical data generated using training datasets and runs the risk of overfitting and selection bias. Overfitting refers to the phenomenon that models developed on training sets do not generalise well to unseen data. This is more of a problem in studies applying ML algorithms to echocardiography, CTCA, SPECT and CMR. This is reflected in the majority of these studies not using separate training and testing sets, likely because of less mature ML algorithms (26,28,32,33,36,44-46). Furthermore, algorithm development requires massive data sets and minimising inherent biases in the data is a challenge. A lack of diversity in training datasets leads to downstream consequences of algorithms being inherently biased and skewed to those types of patients most contributing to model development (4).
Albeit not unique to ML, algorithm development is not immune from issues of privacy, security and data breaches. Moreover, the lack of existing frameworks and guidelines governing how to develop algorithms with sensitive patient data, cross-collaborate between institutions and ownership of patient data derived algorithms remain obscure. Another challenge of applying ML algorithms to patient care is the concept of the ‘black-box’ (52). It is the notion that for ML decision support tools, the outputs generated from the input variables occur in a fashion where the rationale for decision making remains unclear to clinicians. For example, it is not clear how exactly CNN are able to detect patients with atrial fibrillation whilst still in sinus rhythm but instead it is hypothesised structural heart changes detected from the ECG is what CNN are using (18). Thus, research must be rigorously conducted to allow steps and rationale behind each step to be evaluated (53).
Another limitation to consider when thinking about the implementation of AI/ML within clinical practice is that the added value of the technology within hospitals has yet to be established in the literature. Studies pertaining to the clinical, patient and economic outcomes of such ML algorithms are lacking (54). These factors are a major lever in determining technology adoption into the clinical workplace, because the buy-in from clinicians relies on data and a strong evidence base that the technology can deliver high-quality care. Therefore, current ML algorithms require multiple future validation studies, necessitating sharing of data, which brings about important patient confidentiality and regulation issues (55).
Future work
The majority of studies thus far have focused on previously generated data in silico. The next steps involve multi-centre randomized control trials prospectively investigating ML algorithms using unseen data sets to further validate the utility and reproducibility in the clinical setting. Moreover, such trials will need to evaluate the downstream impact of ML algorithms on patient outcomes and treatment pathways, an area sparsely studied to date (4). This involves centres prospectively labelling and annotating data to create multicentre data sets on which ML algorithms can be trained. These algorithms built on diverse populations at low risk of overfitting, then be tested on data sets from multicentre populations not participating in training the ML model. Such trials will need a multidisciplinary approach between centres and regulatory bodies in creating shared frameworks for algorithm standards, data set protocols, ML transparency and issues pertaining to data ownership.
It is important to note the interdisciplinary and cross-industry collaboration needed to further this field. Data scientists are at the heart of building ML algorithms and large technology companies are a rich source of data scientists and AI innovation (15). With such a vested interest in this domain, technology companies will begin to be more involved in prospective multi-centre clinical trial. One example is the Apple Heart Study: a large prospective clinical trial estimating the proportion of participants with atrial fibrillation from an irregular heart rate notification on a wearable technology passively monitoring participants (15). However, parallels, lessons and frameworks developed from pharmaceutical industry involvement in large clinical trials for breakthrough drugs must be correlated to technology company’s involvement in clinical trials for breakthrough AI algorithms, especially the ethical nuances of managing conflicts of interests between researchers, data scientists and algorithm intellectual property owners.
Conclusions
AI is potentially changing much of our life, but is only just beginning its journey in cardiovascular medicine and imaging. Studies to date have shown great promise in classifying disease phenotypes, improving diagnostic accuracy, screening for disease processes and better predicting disease progression, risk and prognosis in multiple imaging and non-imaging modalities (Tables 2,3,4). Leveraging AI in the context of such studies holds promise for clinicians, health systems and patients. However, there remain considerable limitations with respect to existing studies, underlying technology and the clinical translation of such techniques. Prospective, multi-centre randomized controlled trials utilizing interdisciplinary and cross-industry collaboration are needed to further validate the utility and reproducibility of AI in cardiovascular medicine.
Acknowledgments
Funding: None.
Footnote
Provenance and Peer Review: This article was commissioned by the editorial office, Cardiovascular Diagnosis and Therapy for the series “Heart Valve Disease”. The article has undergone external peer review.
Conflicts of Interest: All authors have completed the ICMJE uniform disclosure form (available at http://dx.doi.org/10.21037/cdt.2020.03.09). The series “Heart Valve Disease” was commissioned by the editorial office without any funding or sponsorship. BX served as the unpaid Guest Editor of the series. The authors have no other conflicts of interest to declare.
Ethical Statement: The authors are accountable for all aspects of the work in ensuring that questions related to the accuracy or integrity of any part of the work are appropriately investigated and resolved.
Open Access Statement: This is an Open Access article distributed in accordance with the Creative Commons Attribution-NonCommercial-NoDerivs 4.0 International License (CC BY-NC-ND 4.0), which permits the non-commercial replication and distribution of the article with the strict proviso that no changes or edits are made and the original work is properly cited (including links to both the formal publication through the relevant DOI and the license). See: https://creativecommons.org/licenses/by-nc-nd/4.0/.
References
- Yusuf S, Reddy S, Ôunpuu S, et al. Global burden of cardiovascular diseases: part I: general considerations, the epidemiologic transition, risk factors, and impact of urbanization. Circulation 2001;104:2746-53. [Crossref] [PubMed]
- Mesko B. The role of artificial intelligence in precision medicine. Expert Rev Precis Med Drug Dev 2017;2:239-41. [Crossref]
- Silverio A, Cavallo P, De Rosa R, et al. Big health data and cardiovascular diseases: a challenge for research, an opportunity for clinical care. Front Med (Lausanne) 2019;6:36. [Crossref] [PubMed]
- Dey D, Slomka PJ, Leeson P, et al. Artificial intelligence in cardiovascular imaging: JACC state-of-the-art review. J Am Coll Cardiol 2019;73:1317-35. [Crossref] [PubMed]
- Shah RU, Rumsfeld JS. Big Data in Cardiology. Eur Heart J 2017;38:1865-67. [Crossref] [PubMed]
- Krittanawong C, Zhang H, Wang Z, et al. Artificial intelligence in precision cardiovascular medicine. J Am Coll Cardiol 2017;69:2657-64. [Crossref] [PubMed]
- Johnson KW, Soto JT, Glicksberg BS, et al. Artificial intelligence in cardiology. J Am Coll Cardiol 2018;71:2668-79. [Crossref] [PubMed]
- Kagiyama N, Shrestha S, Farjo PD, et al. Artificial Intelligence: Practical Primer for Clinical Research in Cardiovascular Disease. J Am Heart Assoc 2019;8:e012788 [Crossref] [PubMed]
- Bali J, Garg R, Bali RT. Artificial intelligence (AI) in healthcare and biomedical research: Why a strong computational/AI bioethics framework is required? Indian J Ophthalmol 2019;67:3. [Crossref] [PubMed]
- Meskó B, Hetényi G, Győrffy Z. Will artificial intelligence solve the human resource crisis in healthcare? BMC Health Serv Res 2018;18:545. [Crossref] [PubMed]
- Rajkomar A, Dean J, Kohane I. Machine learning in medicine. N Engl J Med 2019;380:1347-58. [Crossref] [PubMed]
- LeCun Y, Bengio Y, Hinton G. Deep learning. Nature 2015;521:436-44. [Crossref] [PubMed]
- Wiens J, Shenoy ES. Machine learning for healthcare: on the verge of a major shift in healthcare epidemiology. Clin Infect Dis 2018;66:149-53. [Crossref] [PubMed]
- Esteva A, Robicquet A, Ramsundar B, et al. A guide to deep learning in healthcare. Nat Med 2019;25:24. [Crossref] [PubMed]
- Perez MV, Mahaffey KW, Hedlin H, et al. Large-Scale Assessment of a Smartwatch to Identify Atrial Fibrillation. N Engl J Med 2019;381:1909-17. [Crossref] [PubMed]
- Galloway CD, Valys AV, Shreibati JB, et al. Development and Validation of a Deep-Learning Model to Screen for Hyperkalemia From the Electrocardiogram. JAMA Cardiol 2019;4:428-36. [Crossref] [PubMed]
- Attia ZI, Kapa S, Lopez-Jimenez F, et al. Screening for cardiac contractile dysfunction using an artificial intelligence-enabled electrocardiogram. Nat Med 2019;25:70. [Crossref] [PubMed]
- Attia ZI, Noseworthy PA, Lopez-Jimenez F, et al. An artificial intelligence-enabled ECG algorithm for the identification of patients with atrial fibrillation during sinus rhythm: a retrospective analysis of outcome prediction. Lancet 2019;394:861-7. [Crossref] [PubMed]
- Kwon JM, Jeon KH, Kim HM, et al. Comparing the performance of artificial intelligence and conventional diagnosis criteria for detecting left ventricular hypertrophy using electrocardiography. Europace 2020;22:412-9. [Crossref] [PubMed]
- Attia ZI, Friedman PA, Noseworthy PA, et al. Age and sex estimation using artificial intelligence from standard 12-lead ECGs. Circulation 2019;12:e007284 [PubMed]
- Rajpurkar P, Hannun AY, Haghpanahi M, et al. Cardiologist-level arrhythmia detection with convolutional neural networks. Available online: https://stanfordmlgroup.github.io/projects/ecg/
- Strodthoff N, Strodthoff C. Detecting and interpreting myocardial infarction using fully convolutional neural networks. Physiol Meas 2019;40:015001 [Crossref] [PubMed]
- Hannun AY, Rajpurkar P, Haghpanahi M, et al. Cardiologist-level arrhythmia detection and classification in ambulatory electrocardiograms using a deep neural network. Nat Med 2019;25:65. [Crossref] [PubMed]
- Al’Aref SJ, Anchouche K, Singh G, et al. Clinical applications of machine learning in cardiovascular disease and its relevance to cardiac imaging. Eur Heart J 2019;40:1975-86. [Crossref] [PubMed]
- Alsharqi M, Upton R, Mumith A, et al. Artificial intelligence: a new clinical support tool for stress echocardiography. Expert Rev Med Devices 2018;15:513-5. [Crossref] [PubMed]
- Narula S, Shameer K, Omar AMS, et al. Machine-learning algorithms to automate morphological and functional assessments in 2D echocardiography. J Am Coll Cardiol 2016;68:2287-95. [Crossref] [PubMed]
- Zhang J, Gajjala S, Agrawal P, et al. Fully Automated Echocardiogram Interpretation in Clinical Practice. Circulation 2018;138:1623-35. [Crossref] [PubMed]
- Sengupta PP, Huang Y, Bansal M, et al. Cognitive machine-learning algorithm for cardiac imaging: a pilot study for differentiating constrictive pericarditis from restrictive cardiomyopathy. Circulation 2016;9:e004330 [PubMed]
- Khamis H, Zurakhov G, Azar V, et al. Automatic apical view classification of echocardiograms using a discriminative learning dictionary. Med Image Anal 2017;36:15-21. [Crossref] [PubMed]
- Madani A, Arnaout R, Mofrad M, et al. Fast and accurate view classification of echocardiograms using deep learning. NPJ Digit Med 2018;1:6. [Crossref] [PubMed]
- Jiang R, Yeung D, Behnami D, et al. Machine learning to facilitate assessment of diastolic function by echocardiography. Can J Cardiol 2019;35:S4-5. [Crossref]
- Sanchez-Martinez S, Duchateau N, Erdei T, et al. Characterization of myocardial motion patterns by unsupervised multiple kernel learning. Med Image Anal 2017;35:70-82. [Crossref] [PubMed]
- Omar HA, Domingos JS, Patra A, et al. Quantification of cardiac bull's-eye map based on principal strain analysis for myocardial wall motion assessment in stress echocardiograph. Echo Res Pract 2018;5:115-25.
- Moghaddasi H, Nourian S. Automatic assessment of mitral regurgitation severity based on extensive textural features on 2D echocardiography videos. Comput Biol Med 2016;73:47-55. [Crossref] [PubMed]
- Budoff MJ, Dowe D, Jollis JG, et al. Diagnostic performance of 64-multidetector row coronary computed tomographic angiography for evaluation of coronary artery stenosis in individuals without known coronary artery disease: results from the prospective multicenter ACCURACY (Assessment by Coronary Computed Tomographic Angiography of Individuals Undergoing Invasive Coronary Angiography) trial. J Am Coll Cardiol 2008;52:1724-32. [Crossref] [PubMed]
- Motwani M, Dey D, Berman DS, et al. Machine learning for prediction of all-cause mortality in patients with suspected coronary artery disease: a 5-year multicentre prospective registry analysis. Eur Heart J 2017;38:500-7. [PubMed]
- van Rosendael AR, Maliakal G, Kolli KK, et al. Maximization of the usage of coronary CTA derived plaque information using a machine learning based algorithm to improve risk stratification; insights from the CONFIRM registry. J Cardiovasc Comput Tomogr 2018;12:204-9. [Crossref] [PubMed]
- Dey D, Gaur S, Ovrehus KA, et al. Integrated prediction of lesion-specific ischaemia from quantitative coronary CT angiography using machine learning: a multicentre study. Eur Radiol 2018;28:2655-64. [Crossref] [PubMed]
- von Knebel Doeberitz PL, De Cecco CN, Schoepf UJ, et al. Coronary CT angiography-derived plaque quantification with artificial intelligence CT fractional flow reserve for the identification of lesion-specific ischemia. Eur Radiol 2019;29:2378-87. [Crossref] [PubMed]
- Mannil M, von Spiczak J, Manka R, et al. Texture analysis and machine learning for detecting myocardial infarction in noncontrast low-dose computed tomography: unveiling the invisible. Invest Radiol 2018;53:338-43. [Crossref] [PubMed]
- Box LC, Angiolillo DJ, Suzuki N, et al. Heterogeneity of atherosclerotic plaque characteristics in human coronary artery disease: A three‐dimensional intravascular ultrasound study. Catheter Cardiovasc Interv 2007;70:349-56. [Crossref] [PubMed]
- Betancur J, Commandeur F, Motlagh M, et al. Deep learning for prediction of obstructive disease from fast myocardial perfusion SPECT: a multicenter study. JACC Cardiovasc Imaging 2018;11:1654-63. [Crossref] [PubMed]
- Arsanjani R, Xu Y, Dey D, et al. Improved accuracy of myocardial perfusion SPECT for the detection of coronary artery disease using a support vector machine algorithm. J Nucl Med 2013;54:549-55. [Crossref] [PubMed]
- Luo G, Sun G, Wang K, et al. A novel left ventricular volumes prediction method based on deep learning network in cardiac MRI 2016 computing in cardiology conference (CinC). Available online: https://ieeexplore.ieee.org/document/7868686
- Bernard O, Lalande A, Zotti C, et al. Deep learning techniques for automatic MRI cardiac multi-structures segmentation and diagnosis: Is the problem solved? IEEE Trans Med Imaging 2018;37:2514-25. [Crossref] [PubMed]
- Dawes TJ, de Marvao A, Shi W, et al. Machine learning of three-dimensional right ventricular motion enables outcome prediction in pulmonary hypertension: a cardiac MR imaging study. Radiology 2017;283:381-90. [Crossref] [PubMed]
- Miotto R, Li L, Kidd BA, et al. Deep patient: an unsupervised representation to predict the future of patients from the electronic health records. Sci Rep 2016;6:26094. [Crossref] [PubMed]
- Churpek MM, Yuen TC, Winslow C, et al. Multicenter comparison of machine learning methods and conventional regression for predicting clinical deterioration on the wards. Crit Care Med 2016;44:368. [Crossref] [PubMed]
- Frizzell JD, Liang L, Schulte PJ, et al. Prediction of 30-day all-cause readmissions in patients hospitalized for heart failure: comparison of machine learning and other statistical approaches. JAMA Cardiol 2017;2:204-9. [Crossref] [PubMed]
- Shameer K, Johnson KW, Yahi A, et al. Predictive modeling of hospital readmission rates using electronic medical record-wide machine learning: a case-study using Mount Sinai heart failure cohort. Pacific symposium on biocomputing 2017. World Scientific, 2017.
- Choi E, Schuetz A, Stewart WF, et al. Using recurrent neural network models for early detection of heart failure onset. J Am Med Inform Assoc 2017;24:361-70. [Crossref] [PubMed]
- London AJ. Artificial Intelligence and Black‐Box Medical Decisions: Accuracy versus Explainability. Hastings Cent Rep 2019;49:15-21. [Crossref] [PubMed]
- Cabitza F, Rasoini R, Gensini GF. Unintended consequences of machine learning in medicine. JAMA 2017;318:517-8. [Crossref] [PubMed]
- Rumsfeld JS, Joynt KE, Maddox TM. Big data analytics to improve cardiovascular care: promise and challenges. Nat Rev Cardiol 2016;13:350. [Crossref] [PubMed]
- Xu B, Kocyigit D, Grimm R, Griffin B, Cheng F. Applications of artificial intelligence in multimodality cardiovascular imaging: A state-of-the-art review. Prog Cardiovasc Dis 2020;63:367-76. [Crossref] [PubMed]