Non-fasting lipid profile for cardiovascular risk assessments using China ASCVD risk estimator and Europe SCORE risk charts in Chinese participants
Introduction
Cardiovascular disease (CVD) is the leading cause of death worldwide (1) and accounts for more than 40% of deaths in China (2). CVD is caused by multiple invariable and variable factors, the former includes age, gender, genetic heritage, and the latter includes smoking, physical inactivity, obesity, hypertension, hyperglycemia, and hyperlipidemia, which can be modified (3). Dyslipidemia is the strongest modifiable risk factor for CVD, and blood lipids measurement is an integral part of overall CVD risk assessment (4). For decades, lots of guidelines recommended fasting blood lipids (usually at least 8 h or even overnight after the last meal) to be used in lipids measurement, which poses obvious barriers and inconvenience to lipids detection, such as poor compliance, time-consuming, losing visit and missed cases.
However, fasting is not routinely required for lipids assessment (5). Large population studies performed in Copenhagen and Calgary showed that maximal mean changes in lipid profiles as measured in non-fasting samples versus fasting ones were minor, with triglyceride (TG) level increased by 26 mg/dL (0.3 mmol/L), remnant cholesterol (RC) level increased by 8 mg/dL (0.2 mmol/L), total cholesterol (TC) level decreased by 8 mg/dL (0.2 mmol/L), low density lipoprotein cholesterol (LDL-C) level decreased by 8 mg/dL (0.2 mmol/L), non-high-density-lipoprotein cholesterol (non-HDL-C) level decreased by 8 mg/dL (0.2 mmol/L), whereas high density lipoprotein cholesterol (HDL-C), lipoprotein(a), apolipoprotein B, and apolipoprotein A1 were largely unaffected (6,7). Non-fasting level of TG or RC as well as LDL-C has been demonstrated as an independent risk factor for CVD and ischemic stroke in western countries (8-10). Besides, a randomized, cross-over study conducted by Klop et al. (11) demonstrated that non-fasting direct LDL-C (not calculated LDL-C with Friedewald equation), non-HDL-C and apolipoprotein B could be used as treatment targets in secondary cardiovascular prevention. In general, non-fasting lipids have been supported by multiple societies, guidelines, and statements (5,12-16).
As we know, there are few studies about using non-fasting lipids to evaluate cardiovascular risk for primary prevention in China (17), especially in comparison between fasting and non-fasting lipids in the same patients on the same day (18). The aim of this study was to use fasting and non-fasting lipid profiles to estimate CVD risk in Chinese participants through two established risk assessment tools: China atherosclerotic CVD (ASCVD) risk estimator and Europe Systemic Coronary Risk Estimation (SCORE) risk charts, and compare the consistency between two estimators.
We present the following article in accordance with the MDAR reporting checklist (available at https://dx.doi.org/10.21037/cdt-20-1012).
Methods
Study participants
From March 2018 to September 2018, 127 participants (76 men, 51 women) without ASCVD were enrolled at the Second Xiangya Hospital of Central South University. All participants were hospitalized due to supraventricular tachycardia (SVT) without acute attacks waiting for radiofrequency ablation, and had no history of thyroid diseases, liver and kidney diseases, autoimmune disease, cancer, or other severe medical illnesses, and no one took hypolipidemic agents. We chose these participants because there were no absolutely healthy participants in the cardiovascular medicine ward. Besides, SVT patients without any underlying CVDs would be closest to the “general participants”. Acute attacks of supraventricular tachycardia include atrial fibrillation, paroxysmal supraventricular tachycardia, atrial flutter, and atrial tachycardia. We excluded these acute attacks because possible changes in lipids levels due to acute stress. Meanwhile, all participants included in this study without history of CVDs, including coronary artery disease, heart failure, stroke, peripheral arterial disease, etc. The study was approved by the Ethics Committee of the Second Xiangya Hospital of Central South University (No. ChiCTR1900020873) and informed consent was gained from all participants. The study was conducted in accordance with the Declaration of Helsinki (as revised in 2013).
Sample collection
All enrolled participants took breakfast according to their daily diet habits. Venous blood samples were collected before and at 2 h, 4 h after breakfast. During the 4 h period, participants were only allowed to drink water and prohibited to smoke, drink wine, eat any food or strenuously exercising. Fasting blood lipids usually at least 8 h or even overnight after the last meal. In this study, we collected fasting blood samples before breakfast, because there is no need to wait 8 hours after breakfast which it is not easy for participants to keep fasting during the day time.
Laboratory examinations
The concentrations of serum TC and TG were measured by enzyme method, HDL-C and LDL-C were measured by chemical masking method (Wako, Japan) based on Hitachi 7170A automatic biochemical analyzer (19-21). RC and non-HDL-C level were calculated by the two following formulas, RC = TC − (HDL-C) − (LDL-C), and non-HDL-C = TC − (HDL-C).
Risk estimator
China ASCVD risk estimator was based on China's first risk prediction model with ASCVD as the endpoint event to validate the 10-year ASCVD risk in Chinese participants, which included age, BP, TC level, LDL-C level, HDL-C level, current smoking, and diabetes mellitus (22,23). Europe SCORE risk charts were based on large, representative European cohort datasets, which included age, gender, smoking, systolic blood pressure and total cholesterol level (24).
Statistical analyses
All data were analyzed using Social Sciences (SPSS) version 22.0 and Graph Pad prism7.0 software. Continuous variables and categorical parameters were compared with the Students t-test and chi-square test, respectively. Quantitative variables were expressed as mean ± standard deviation (SD) unless specifically explained, and qualitative variables were expressed as numbers and percentages. Reliability analysis was performed with the Kappa statistic to determine consistency among estimators. Two estimators were said to be in poor agreement if the Kappa statistic was <0, in slight agreement if 0.0 to 0.20, fair agreement if 0.21 to 0.40, moderate agreement if 0.41 to 0.60, substantial agreement if 0.61 to 0.80, and in perfect agreement if it was 0.81 to 1.00 (25). All tests were considered to be statistically significant at P value <0.05.
Results
Basic clinical features of study participants
Total participants in this study were 127, including 76 men (59.8%) and 51 (40.2%) women. There were no significant differences in age, systolic or diastolic blood pressure, TC level, LDL-C level, non-HDL-C level, the percentages of cases with hypertension and diabetes between male and female participants, except male participants had significantly higher body mass index (BMI), levels of TG and RC, while lower HDL-C level. Besides, smokers incorporated in this study were all male (Table 1).
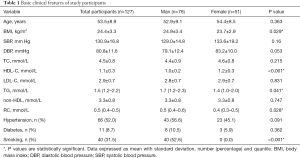
Full table
Changes between fasting and non-fasting lipid levels after a daily breakfast
Taking all participants as a whole, the serum levels of TC and non-HDL-C mildly decreased at 2 h, but slightly recovered at 4 h. In the meantime, both TG and RC levels increased significantly and reached their peak at 4 h while LDL-C level reached the lowest value at 4 h (P<0.05). Besides, postprandial change in HDL-C level was negligible (Figure 1).
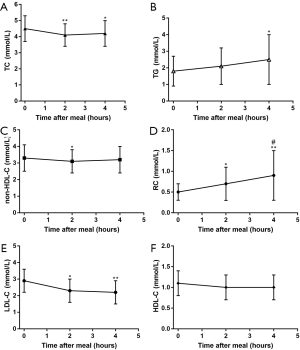
To be specific, TC level decreased from 4.5 mmol/L at baseline to 4.1 mmol/L at 2 h and increased to 4.2 mmol/L at 4 h, non-HDL-C level decreased from 3.3 mmol/L to 3.1 mmol/L at 2 h and increased to 3.2 mmol/L at 4 h, and LDL-C level decreased from 2.9 to 2.2 mmol/L at 4 h. Besides, TG and RC levels respectively varied from 1.5 mmol/L at baseline to 2.1 mmol/L, 0.5 mmol/L to 0.7 mmol/L at 4 h after a daily breakfast.
Cardiovascular risk classifications based on fasting and non-fasting lipid profiles using different risk estimators
All participants were underwent cardiovascular risk assessment according to China ASCVD risk estimator and European SCORE risk charts respectively (22,23,26). According to lipid profile between fasting and 2 h, 4 h after daily breakfast, China ASCVD risk estimator respectively classified approximately half of the participants as low-risk (0 h, 2 h, 4 h: 49.6%, 55.1%, 55.1%), less than one-fifth of them as moderate-risk (0 h, 2 h, 4 h: 18.9%, 17.3%, 15.7%), about one-fifth of them as high-risk (0 h, 2 h, 4 h: 22.8%, 18.9%, 20.5%) (Figure 2A). However, European SCORE system (low-and high-risk charts) showed a different result which classified less than one-third them as low-risk (low risk chart: 0 h, 2 h, 4 h: 25.2%, 25.2%, 24.4%; high risk chart: 0 h, 2 h, 4 h: 15.0%, 15.0%, 15.0%), more than half of the participants as moderate-risk (low risk chart: 0 h, 2 h, 4 h: 55.9%, 55.9%, 56.7%; high risk chart: 0 h, 2 h, 4 h: 53.5%, 55.9%, 55.1%), less one-fourth of them as high-risk (low risk chart: 0 h, 2 h, 4 h: 10.2%, 10.2%, 10.2%; high risk chart: 0 h, 2 h, 4 h: 22.8%, 20.4%, 21.2%) in fasting and non-fasting states. Besides, percentages of very high-risk in both two risk estimators are the same (8.7%) (Figure 2B,C). To sum up, using China ASCVD risk estimator with non-fasting samples has a reduction effect in CVD risk evaluation, which is not statistically significant (P=0.970, Tables S1,S2). Besides, that reduction trend is not present in SCORE charts (P=1.000, Tables S3,S4,S5,S6, Table 2). In addition, China ASCVD risk estimator assessed half of the participants as low risk, while European risk charts assessed half of the participants as moderate risk for the same participants (Figure 2A,B,C), which indicated European risk charts may overestimate risk assessment among Chinese patients compared with China ASCVD risk estimator.
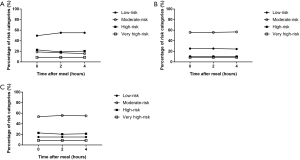
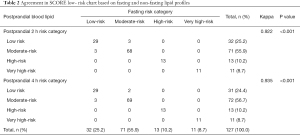
Full table
Moreover, there was substantial agreement between China ASCVD risk estimator based on fasting and non-fasting lipid profiles (Kappa =0.731 or 0.718, P<0.001) (Table 3). In addition, the agreement between SCORE system based on fasting and non-fasting lipid profiles in both low- and high- risk charts were high as well, (Kappa =0.922 or 0.935, P<0.001, Table 2) (Kappa =0.886 or 0.874, P<0.001, Table 4).

Full table
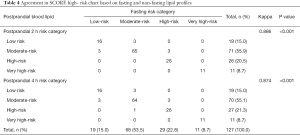
Full table
Before China ASCVD risk estimator came out in 2016, European SCORE risk charts were used to evaluate Chinese subjects as well. Moreover, to a certain extent, China ASCVD risk estimator was also established risk factors used in European SCORE risk charts. From this point of view, it is meaningful to compare the two evaluation systems. However, agreement between China ASCVD risk estimator and SCORE low- or high-risk chart was relatively poor no matter in fasting (Kappa =0.339 or 0.300, P<0.001) (Table 5) or non-fasting state (Kappa =0.364 or 0.286, P<0.001) (Table 6), which indicated China ASCVD risk estimator and SCORE risk charts are inconsistent in risk stratification in the same Chinese participants.
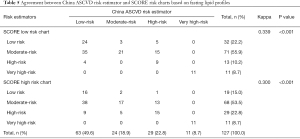
Full table
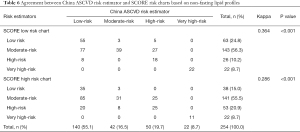
Full table
Discussion
Non-fasting lipids measurements are the new standard in European countries according to the latest guidelines. Large population studies have fully affirmed that non-fasting lipids are better than fasting lipids in predicting cardiovascular disease risk (8-10). Therefore, it is feasible to use non-fasting lipids as a routine blood lipid testing method for CVD risk assessment. However, due to lack of clinical data on non-fasting lipids, no reference values suitable for Chinese population to determine levels of non-fasting lipids, and also the concept of non-fasting lipids has not been extended to hospitals at all levels. Therefore, non-fasting lipids are not widely used in China.
In this study, we use fasting and non-fasting lipid profile to assess 10-year risk of ASCVD in the same Chinese general patients via China ASCVD risk estimator and Europe SCORE risk charts respectively. The big difference between two estimators is that China ASCVD risk estimator assessed half of the participants as low risk, while European risk charts assessed half of the participants as moderate risk in the same participants. Reliability analysis in China ASCVD risk estimator and Europe SCORE risk charts based on fasting and or non-fasting lipids profile were relatively high. However, agreement between two estimators were relatively poor in both fasting and non-fasting states. The above results indicated that non-fasting lipid profile could be considered to evaluate 10-year CVD risk via China ASCVD risk estimator and Europe SCORE risk charts in clinical practice, but, the latter one may overestimate CVD risk in Chinese general population.
Non-fasting lipid profiles changes in this study, especially LDL-C and TG, were relatively different from Denmark’s studies, which showed insignificant changes after a daily meal, and maximal mean changes between non-fasting versus fasting blood samples as measured in random are +0.3 mmol/L for TG level and −0.2 mmol/L for TC and LDL-C (5,6,27,28). However, TC and LDL-C levels decreased by 0.3 and 0.7 mmol/L, respectively, while TG level increased by 0.6 mmol/L at 4 h after a daily breakfast in this study. The potential causes for non-fasting reduction in LDL-C level and increment in TG level were complicated and controversial. In our previous study, we found that postprandial reduction in direct measured LDL-C level was more prominent than that in calculated LDL-C level by Friedewald formula at both 2 h and 4 h after a daily breakfast in Chinese subjects (29), and LDL-C level was detected by direct method in the present study. Additionally, some scholars believed that mildly reduction in LDL-C level after a daily meal was due to fluid intake, but changes could be corrected by adjustment for albumin levels (6,30). All participants had their breakfast according to their daily habits with similar type and amount of food. In addition, we will record their daily appetite, sleep quality and body weight during hospitalization. As participants were similar to the “general participants”, their physical condition and appetite remained good in this study. Compared with the western breakfast, traditional Chinese breakfast could have a higher carbohydrate content and fluid intake, such as porridge, noodles, vermicelli, soy milk and so on, but not breakfast rich in protein and/or fat and solid food such as cheese, sausage, ham, and bacon (31,32). Unfortunately, changes in albumin levels were not included in this study. Moreover, the potential role of difference in commercial lipid test kits and race between different studies could also be considered.
In addition, there is a reducing trend in CVD risk using China ASCVD risk estimator with non-fasting samples, which is not the case in European SCORE charts, even though that reducing trend due to habitual food intake was minimal and without clinical consequences (P=0.970, Tables S1,S2). In view of the decreases of TC, LDL-C and HDL-C levels after a daily meal, the risk of ASCVD assessed by non-fasting blood lipids would be underestimated rather than overestimated. Changes in risk categories paralleled with lipids changes that TC, LDL-C and non-HDL-C levels decreased after a daily breakfast (Figure 1). Moreover, participants will reclassify into different risk categories based on non-fasting lipids after a daily meal when compared with corresponding fasting lipids. In addition, number of participants in this study was relatively small, and it is possible if we include more samples, there is no reducing trend.
China ASCVD risk estimator was primarily based on results of China-PAR (i.e., Prediction for ASCVD Risk in China) project which was the first study to develop and validate the 10-year ASCVD risk in four large, contemporary Chinese populations, and it was fasting lipid profiles used in that project (22). In addition, China-PAR project included age, BP, TC level, LDL-C level, HDL-C level, current smoking, and diabetes mellitus, had been proved excellent performance in ASCVD risk prediction with good internal consistency and external validation compared with western estimators (33,34).
SCORE system, which estimated 10-year cumulative risk of first fatal atherosclerotic events, had been recommended for risk stratification in European countries, and the corresponding risk estimator had been produced as charts for low- and high-risk regions (24). SCORE system was based on large, representative European cohort datasets, included several risk factors, such as age, gender, smoking, systolic blood pressure and total cholesterol level in risk charts. Agreement between fasting and non-fasting in risk stratification was high in both low- and high-risk charts, and it was TC level used in SCORE risk charts.
Agreement between SCORE low-and high-risk charts was high, whereas relatively poor with China ASCVD risk estimator as the former classified half of the participants as moderate risk, while China ASCVD risk estimator assessed half of the participants as low risk in the same participants, which indicated that SCORE risk charts may overestimate CVD risk in Chinese general participants. That is to say that SCORE system may not be appropriate for Chinese individuals to evaluate 10-year CVD risk. Substantial differences in prediction capability between China ASCVD risk estimator and SCORE risk charts could be due to ethnic heterogeneities, distinctive risk characteristics of CVD, as well as different treatment, control rates of risk factors (e.g., hyperlipidemia) (26,35-38). Since two established risk assessment systems above were based on research results or databases of their own respective populations, which caused big differences in genetic heritage, living, and eating habits and environment that are all matters to CVD risk stratification. Thus, it is more suitable for individuals to use specific and regional assessment tools when conducting cardiovascular risk assessments.
There were several limitations in this study. Firstly, the number of participants in this study was relatively small. Secondly, this is a cross-sectional study, thus it was impossible to verify occurrence of cardiovascular events. A prospective study with large sample size could be needed to further explore application of non-fasting lipids in CVD risk assessments in Chinese general population.
Conclusions
Our study suggested that non-fasting lipids could be applied in evaluation of 10-year CVD risk via China ASCVD risk estimator in Chinese general population, which make application of non-fasting lipids in CVD more widespread.
Acknowledgments
We would like to thank Alessandro Shapiro for his help in polishing our paper.
Funding: This work was supported by National Natural Science Foundation of China (No. 81902512 and 81470577), Fundamental Research Funds for the Central Universities of Central South University (No. 2018zzts047) and Hunan Provincial Innovation Foundation for Postgraduate (No. CX20200288).
Footnote
Reporting Checklist: The authors have completed the MDAR reporting checklist. Available at https://dx.doi.org/10.21037/cdt-20-1012
Data Sharing Statement: Available at https://dx.doi.org/10.21037/cdt-20-1012
Conflicts of Interest: All authors have completed the ICMJE uniform disclosure form (available at https://dx.doi.org/10.21037/cdt-20-1012). The authors declare no conflicts of interest.
Ethical Statement: The authors are accountable for all aspects of the work in ensuring that questions related to the accuracy or integrity of any part of the work are appropriately investigated and resolved. The study was conducted in accordance with the Declaration of Helsinki (as revised in 2013). The study was approved by the Ethics Committee of the Second Xiangya Hospital of Central South University (No. ChiCTR1900020873) and informed consent was gained from all participants.
Open Access Statement: This is an Open Access article distributed in accordance with the Creative Commons Attribution-NonCommercial-NoDerivs 4.0 International License (CC BY-NC-ND 4.0), which permits the non-commercial replication and distribution of the article with the strict proviso that no changes or edits are made and the original work is properly cited (including links to both the formal publication through the relevant DOI and the license). See: https://creativecommons.org/licenses/by-nc-nd/4.0/.
References
- Sacco RL, Roth GA, Reddy KS, et al. The Heart of 25 by 25: Achieving the Goal of Reducing Global and Regional Premature Deaths From Cardiovascular Diseases and Stroke: A Modeling Study From the American Heart Association and World Heart Federation. Circulation 2016;133:e674-90. [Crossref] [PubMed]
- Zhou M, Wang H, Zhu J, et al. Cause-specific mortality for 240 causes in China during 1990-2013: a systematic subnational analysis for the Global Burden of Disease Study 2013. Lancet 2016;387:251-72. [Crossref] [PubMed]
- European Association for Cardiovascular Prevention & Rehabilitation. ESC/EAS Guidelines for the management of dyslipidaemias: the Task Force for the management of dyslipidaemias of the European Society of Cardiology (ESC) and the European Atherosclerosis Society (EAS). Eur Heart J 2011;32:1769-818. [Crossref] [PubMed]
- Yusuf S, Hawken S, Ounpuu S, et al. Effect of potentially modifiable risk factors associated with myocardial infarction in 52 countries (the INTERHEART study): case-control study. Lancet 2004;364:937-52. [Crossref] [PubMed]
- Nordestgaard BG, Langsted A, Mora S, et al. Fasting is not routinely required for determination of a lipid profile: clinical and laboratory implications including flagging at desirable concentration cut-points-a joint consensus statement from the European Atherosclerosis Society and European Federation of Clinical Chemistry and Laboratory Medicine. Eur Heart J 2016;37:1944-58. [Crossref] [PubMed]
- Langsted A, Freiberg JJ, Nordestgaard BG. Fasting and nonfasting lipid levels: influence of normal food intake on lipids, lipoproteins, apolipoproteins, and cardiovascular risk prediction. Circulation 2008;118:2047-56. [Crossref] [PubMed]
- Sidhu D, Naugler C. Fasting time and lipid levels in a community-based population: a cross-sectional study. Arch Intern Med 2012;172:1707-10. [Crossref] [PubMed]
- Bansal S, Buring JE, Rifai N, et al. Fasting compared with nonfasting triglycerides and risk of cardiovascular events in women. JAMA 2007;298:309-16. [Crossref] [PubMed]
- Freiberg JJ, Tybjaerg-Hansen A, Jensen JS, et al. Nonfasting triglycerides and risk of ischemic stroke in the general population. JAMA 2008;300:2142-52. [Crossref] [PubMed]
- Doran B, Guo Y, Xu J, et al. Prognostic value of fasting versus nonfasting low-density lipoprotein cholesterol levels on long-term mortality: insight from the National Health and Nutrition Examination Survey III (NHANES-III). Circulation 2014;130:546-53. [Crossref] [PubMed]
- Klop B, Hartong SCC, Vermeer HJ, et al. Risk of misclassification with a non-fasting lipid profile in secondary cardiovascular prevention. Clin Chim Acta 2017;472:90-5. [Crossref] [PubMed]
- Catapano AL, Graham I, De Backer G, et al. 2016 ESC/EAS Guidelines for the Management of Dyslipidaemias. Eur Heart J 2016;37:2999-3058. [Crossref] [PubMed]
- Anderson TJ, Grégoire J, Pearson GJ, et al. 2016 Canadian Cardiovascular Society Guidelines for the Management of Dyslipidemia for the Prevention of Cardiovascular Disease in the Adult. Can J Cardiol 2016;32:1263-82. [Crossref] [PubMed]
- Scartezini M, Ferreira CEDS, Izar MCO, et al. Positioning about the Flexibility of Fasting for Lipid Profiling. Arq Bras Cardiol 2017;108:195-7. [Crossref] [PubMed]
- Miller M, Stone NJ, Ballantyne C, et al. Triglycerides and cardiovascular disease: a scientific statement from the American Heart Association. Circulation 2011;123:2292-333. [Crossref] [PubMed]
- Downs JR, O'Malley PG. Management of dyslipidemia for cardiovascular disease risk reduction: synopsis of the 2014 U.S. Department of Veterans Affairs and U.S. Department of Defense clinical practice guideline. Ann Intern Med 2015;163:291-7. [Crossref] [PubMed]
- Hong LF, Yan XN, Lu ZH, et al. Predictive value of non-fasting remnant cholesterol for short-term outcome of diabetics with new-onset stable coronary artery disease. Lipids Health Dis 2017;16:7. [Crossref] [PubMed]
- Tian F, Xiang QY, Zhang MY, et al. Changes in non-fasting concentrations of blood lipids after a daily Chinese breakfast in overweight subjects without fasting hypertriglyceridemia. Clin Chim Acta 2019;490:147-53. [Crossref] [PubMed]
- Zhao Y, Peng R, Zhao W, et al. Zhibitai and low-dose atorvastatin reduce blood lipids and inflammation in patients with coronary artery disease. Medicine (Baltimore) 2017;96:e6104 [Crossref] [PubMed]
- Tian F, Wu CL, Yu BL, et al. Apolipoprotein O expression in mouse liver enhances hepatic lipid accumulation by impairing mitochondrial function. Biochem Biophys Res Commun 2017;491:8-14. [Crossref] [PubMed]
- Xu J, Chen YQ, Zhao SP, et al. Determination of optimal cut-off points after a high-fat meal corresponding to fasting elevations of triglyceride and remnant cholesterol in Chinese subjects. Lipids Health Dis 2019;18:206. [Crossref] [PubMed]
- Yang X, Li J, Hu D, et al. Predicting the 10-Year Risks of Atherosclerotic Cardiovascular Disease in Chinese Population: The China-PAR Project (Prediction for ASCVD Risk in China). Circulation 2016;134:1430-40. [Crossref] [PubMed]
- Hu DY. New guidelines and evidence for the prevention and treatment of dyslipidemia and atherosclerotic cardiovascular disease in China. Zhonghua Xin Xue Guan Bing Za Zhi 2016;44:826-7. [PubMed]
- Perk J, De Backer G, Gohlke H, et al. European Guidelines on cardiovascular disease prevention in clinical practice (version 2012). The Fifth Joint Task Force of the European Society of Cardiology and Other Societies on Cardiovascular Disease Prevention in Clinical Practice (constituted by representatives of nine societies and by invited experts). Eur Heart J 2012;33:1635-701. [Crossref] [PubMed]
- Landis JR, Koch GG. The measurement of observer agreement for categorical data. Biometrics 1977;33:159-74. [Crossref] [PubMed]
- Conroy RM, Pyörälä K, Fitzgerald AP, et al. Estimation of ten-year risk of fatal cardiovascular disease in Europe: the SCORE project. Eur Heart J 2003;24:987-1003. [Crossref] [PubMed]
- Nordestgaard BG, Benn M. Fasting and nonfasting LDL cholesterol: to measure or calculate? Clin Chem 2009;55:845-7. [Crossref] [PubMed]
- Nordestgaard BG. A Test in Context: Lipid Profile, Fasting Versus Nonfasting. J Am Coll Cardiol 2017;70:1637-46. [Crossref] [PubMed]
- Lin QZ, Chen YQ, Guo LL, et al. Comparison of non-fasting LDL-C levels calculated by Friedewald formula with those directly measured in Chinese patients with coronary heart disease after a daily breakfast. Clin Chim Acta 2019;495:399-405. [Crossref] [PubMed]
- Langsted A, Nordestgaard BG. Nonfasting lipids, lipoproteins, and apolipoproteins in individuals with and without diabetes: 58 434 individuals from the Copenhagen General Population Study. Clin Chem 2011;57:482-9. [Crossref] [PubMed]
- Lee MM, Wu-Williams A, Whittemore AS, et al. Comparison of dietary habits, physical activity and body size among Chinese in North America and China. Int J Epidemiol 1994;23:984-90. [Crossref] [PubMed]
- Chen Z, Shu XO, Yang G, et al. Nutrient intake among Chinese women living in Shanghai, China. Br J Nutr 2006;96:393-9. [Crossref] [PubMed]
- D'Agostino RB Sr, Vasan RS, Pencina MJ, et al. General cardiovascular risk profile for use in primary care: the Framingham Heart Study. Circulation 2008;117:743-53. [Crossref] [PubMed]
- Goff DC Jr, Lloyd-Jones DM, Bennett G, et al. 2013 ACC/AHA guideline on the assessment of cardiovascular risk: a report of the American College of Cardiology/American Heart Association Task Force on Practice Guidelines. Circulation 2014;129:S49-73. [Crossref] [PubMed]
- De Backer G, Ambrosioni E, Borch-Johnsen K, et al. European guidelines on cardiovascular disease and prevention in clinical practice. Atherosclerosis 2003;171:145-55. [Crossref] [PubMed]
- Yang W, Xiao J, Yang Z, et al. Serum lipids and lipoproteins in Chinese men and women. Circulation 2012;125:2212-21. [Crossref] [PubMed]
- Chan F, Adamo S, Coxson P, et al. Projected impact of urbanization on cardiovascular disease in China. Int J Public Health 2012;57:849-54. [Crossref] [PubMed]
- Xu Y, Wang L, He J, et al. Prevalence and control of diabetes in Chinese adults. JAMA 2013;310:948-59. [Crossref] [PubMed]