Impact of coronary plaque morphology on the precision of computational fractional flow reserve derived from optical coherence tomography imaging
Introduction
Fractional flow reserve (FFR) has emerged as a useful tool to guide revascularization in patients with coronary artery disease (1,2). FFR-guided percutaneous coronary intervention (PCI) leads to lower rates of repeat urgent revascularization and non-fatal myocardial infarction (MI) than optimal medical treatment and angiography-guided PCI (3,4). During recent years, the image-based FFR was developed to expand the use of physiology-guided PCI. Both angiography and intracoronary imaging-based computational methods have a good agreement with FFR (5-7). Nevertheless, current methods do not account for plaque composition in the computation of FFR. It remains unknown whether the numerical precision of computational FFR is impacted by the plaque composition in the interrogated vessels. Recently, a novel deep learning algorithm was proposed for automatic plaque characterization on intracoronary optical coherence tomography (OCT) images (8). We aimed to investigate the impact of coronary plaque composition on the numerical precision of OCT-derived FFR with invasive FFR as reference. We present the following article in accordance with the STROBE reporting checklist (available at https://cdt.amegroups.com/article/view/10.21037/cdt-21-505/rc).
Methods
Study design and patient population
This study was a post-hoc analysis of an observational, retrospective, cross-sectional single-center study (9). Main results including details on patient flow, exclusion/inclusion criteria, acquisition of invasive coronary angiography (ICA), FFR and OCT were previously described (9). In short, all patients who underwent ICA, OCT-imaging and FFR assessment between August 2011 and October 2018 at Wakayama Medical University Hospital (Wakayama, Japan) were enrolled. Vessels were excluded from OCT plaque analysis if they were not suitable for paired comparison between optical flow ratio (OFR) and FFR. Briefly, vessels were excluded if (I) the balloon predilatation was performed prior to OCT imaging, (II) the spasm or injury during OCT imaging was present, (III) OCT pullback not covering the entire lesion, (IV) there was a myocardial bridge in the interrogated vessel, (V) the presence of bypass graft, (VI) the quality of FFR pressure tracings was unacceptable, or (VII) the presence of substantial thrombosis identified by OCT (9). OCT imaging was performed by frequency-domain OCT systems (ILUMIEN™ or OPTIS™; Abbott, St. Paul, MN, USA) at 100 or 180 frames/s, with the Dragonfly or Dragonfly DUO catheter (9). All vessels with paired OFR, and FFR (193 patients and 230 vessels) were included in the present study. The study was conducted in accordance with the Declaration of Helsinki (as revised in 2013). The institutional review board of Wakayama Medical University approved the post hoc analysis of the data for this study (No. 2876) and patients’ informed consent was waived due to the retrospective nature of the study.
OFR
Computation of OFR was performed by two observers blinded to FFR using the OctPlus software (version 1.0, Pulse Medical Imaging Technology, Shanghai, China). In short, lumen contours of the interrogated vessels were automatically delineated and reconstructed in three-dimension (3D). The side branches ostia were reconstructed and the reference lumen size incorporating the step-down phenomenon across bifurcations was quantified (7,9). The hypothesized maximum hyperemic flow rate was calculated and fluid dynamics equations were used to calculate OFR value at each position along the interrogated vessel. The OFR value at the distal position of the analyzed vessel matching with the pressure sensor position was used to compare with FFR (9). The numerical difference between OFR and FFR (NDOF) was evaluated at vessel level and categorized in three groups; (I) overestimation where OFR was at least 0.05 larger than FFR, i.e., NDOF >0.05, (II) reference group where −0.05≤ NDOF ≤0.05, and (III) underestimation group where OFR was at least 0.05 smaller than FFR, i.e., NDOF <−0.05. The value of 0.05 was chosen because it was the standard deviation of NDOF.
Plaque morphology
Plaque composition was automatically characterized as fibrotic, lipid or calcified using a recently developed software package (OctPlus, Pulse Medical Imaging Technology, Shanghai, China, version 2.0) with a convolution neural network (CNN) model (8), which has been externally validated with high diagnostic accuracy. For cross-sections with invisible media due to the intense attenuation of the signal through the lipid-rich pool, the contour will be extrapolated based on the circular geometry of the arterial structures in the cross-section and the information from continuous frames (8). Thin-cap fibroatheroma (TCFA) was defined as a plaque with maximal lipid arc >90° in three consecutive OCT image frames and thinnest fibrous cap thickness <65 µm in at least one image frame (10). All OCT image frames in the interrogated vessel were analyzed during the same computational procedure. Total plaque volume (TPV), fibrous plaque volume (FPV), lipidic plaque volume (LPV) and calcific plaque volume (CPV) were quantified. Other parameters of interest were minimal lumen area (MLA) and quantitative coronary angiography (QCA)-derived percentage diameter stenosis. Diffusely diseased vessels were defined as vessels with an OCT pullback with <10% of the frames with normal tri-laminar structure in the entire circumference. Reference diameter for calculating OFR was only analyzed in frames without plaque. Diagnostic accuracy is not influenced by the presence of diffuse disease (9).
Statistical analysis
Descriptive results were reported as mean ± SD or median [inter-quartile range (IQR)] according to data distribution. Correlation coefficients were analyzed using Pearson’s correlation or Spearman’s correlation. Kruskal-Wallis test or Chi-square test was used to test for statistical significance of plaque parameters between different groups categorized by NDOF as appropriate. A multilevel linear mixed regression model was constructed to evaluate if plaque features among LPV, CPV, FPV and TCFA were associated with NDOF using patient ID as random effect to account for multiple lesion per patient. The model was adjusted for factors known to influence FFR and computational approaches (age, sex, diabetes, hypertension, smoking, previous MI, and hyperlipidemia). Sensitivity analysis further adjusting for MLA, FFR and acute coronary syndrome (ACS)/non-ACS were then performed. The cases with missing data will be excluded from statistically analysis. A 2-sided P value <0.05 was considered significant. MedCalc version 14.12 (Medcalc Software, Ostend, Belgium) and STATA version 16.0 was used for analysis.
Results
A total of 339 vessels from 277 consecutive patients undergoing both OCT imaging and pressure-derived FFR measurement were screened and 230 vessels from 193 patients paired with OFR and FFR were enrolled for plaque analysis finally (Figure 1). Deep learning-based plaque characterization was performed on all the enrolled cases. Most of them were excluded due to the OCT image pullbacks not covering the entire lesion (n=59). Details were reported in the previous study (9). The baseline demographic and lesion characteristics are reported stratified by NDOF in Table 1. Patient characteristics are presented at vessel level. In case one patient has multiple vessels enrolled, results would be reported separately. In the current study, 30 out of 193 (16%) patients have more than one vessel enrolled. Percent diameter stenosis derived by 3D-QCA of the interrogated vessel was (48.5±11.8)%. Six (3.1%) lesions were located in NSTEMI patients with the rest being stable angina (25.9%), silent ischemia (51.3%), unstable angina (13.5%) and other (6.2%). One patient was excluded due to an excessive amount of thrombus and no plaque ruptures were observed in the pullbacks. The MLA was 2.08±1.09 mm2, the minimum lumen diameter was 1.57±0.40 mm, and the reference diameter was 3.07±0.96 mm by QCA. The CNN was able to quantify plaque composition in all patients with paired OFR and FFR (230 vessels from 193 patients). In total, 138 (59.9%) vessels interrogated were the left anterior descending artery (LAD), 39 (16.9%) were left circumflex artery and 53 (23.0%) were right coronary artery. A total of 79 (34.3%) vessels had a diffuse disease in the region of interest and the mean lesion length was 22.75±13.23 mm. Figure 2 shows a representative example of plaque characterization in a study vessel. Mean NODF was 0.00±0.05 at vessel level. According to the defined threshold of 0.05 for NODF, 21, 179, and 30 vessels were in the overestimation, reference and underestimation group, respectively. Patients tended to have a worse cardiac risk profile in the overestimation group than the reference and underestimation groups indicated by a higher proportion of patients with previous MI, hypertension, diabetes mellitus and hyperlipidemia.
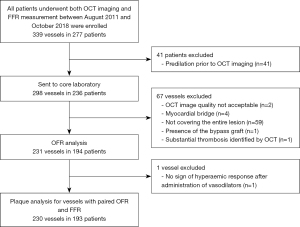
Table 1
Vessels (n=230) | Overestimation (n=21) | Reference (n=179) | Underestimation (n=30) |
---|---|---|---|
Patient characteristics* | |||
Male | 16 (76.1%) | 137 (76.5%) | 17 (56.7%) |
Age | 66±11.2 | 68±11.0 | 69±8.9 |
Diabetes | 12 (57.1%) | 72 (40.2%) | 10 (33.3%) |
Hypertension | 20 (95.2%) | 146 (81.6%) | 26 (86.7%) |
Hyperlipidemia | 17 (80.9%) | 131 (73.2%) | 21 (70.0%) |
Smoking | 1 (4.8%) | 40 (22.3%) | 4 (13.3%) |
Previous MI | 12 (57.1%) | 86 (48.0%) | 11 (36.7%) |
ACS | 2 (9.5%) | 28 (15.6%) | 5 (16.7%) |
Plaque parameters | |||
MLA (mm2) | 2.92±3.53 | 1.92±1.40 | 1.92±1.48 |
DS% | 46.1±28.7 | 49.1±13.4 | 48.9±11.6 |
TPV (mm3) | 261.74±113.79 | 298.32±138.96 | 323.20±154.70 |
LPV (mm3) | 34.18±44.66 | 59.42±59.38 | 85.96±61.64 |
FPV (mm3) | 192.40±80.37 | 188.34±87.66 | 189.99±61.37 |
CPV (mm3) | 5.63±16.32 | 7.52±15.34 | 6.48±19.13 |
TCFA | 4 (19.0%) | 65 (36.3%) | 18 (60.0%) |
Data are presented as mean ± SD or n (%). *, patient characteristics are presented at vessel level, i.e., for patients who have more than one vessel enrolled, results were reported separately. MI, myocardial infarction; ACS, acute coronary syndrome; MLA, minimal lumen area; DS%, percentage of diameters stenosis; TPV, total plaque volume; LPV, lipidic plaque volume; FPV, fibrous plaque volume; CPV, calcific plaque volume; TCFA, thin-cap fibroatheroma.
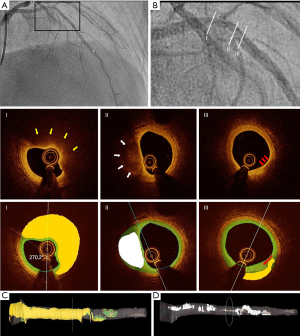
Association of plaque composition and OFR-FFR disagreement
The correlations of NODF with MLA, LPV, FPV, and CPV are plotted in Figure 3. NODF showed a weak negative correlation with LPV (r=−0.189; P=0.004) while the remaining parameters did not correlate with NODF (Figure 3). LPV was larger in the underestimation group (85.96 mm3) than the overestimation (34.18 mm3, P<0.001) and reference group (59.42 mm3, P=0.015) (Figure 4). LPV was the only independent predictor of OFR-FFR disagreement (Table 2). In the unadjusted and adjusted models, OFR tended to underestimate FFR with an average of −0.028 [95% confidence interval (CI): −0.048, −0.009] and −0.028 (95% CI: −0.047, −0.009) for every 100 mm3 increase in LPV (Table 2).
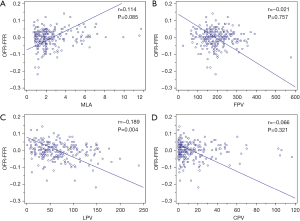
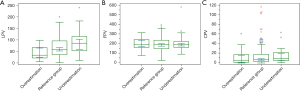
Table 2
Parameter | Unadjusted (95% CI) | P | Adjusted* (95% CI) | P |
---|---|---|---|---|
LPV/100 (mm3) | −0.028 (−0.048, −0.009) | 0.004 | −0.028 (−0.047, −0.009) | 0.004 |
CPV/100 (mm3) | 0.025 (−0.013, 0.062) | 0.201 | 0.026 (−0.013, 0.065) | 0.194 |
FPV/100 (mm3) | 0.002 (−0.007, 0.012) | 0.621 | −0.002 (−0.012, 0.008) | 0.679 |
TCFA | −0.008 (−0.022, 0.007) | 0.288 | −0.007 (−0.023, 0.008) | 0.366 |
*, the model was adjusted by patients’ characteristics including age, sex, diabetes, hypertension, smoking, previous MI, and hyperlipidemia. OFR, optical flow ratio; FFR, fractional flow reserve; CI, confidence interval; LPV, lipid plaque volume; CPV, calcific plaque volume; FPV, fibrotic plaque volume; TCFA, thin-cap fibroatheroma; MI, myocardial infarction.
Plaque vulnerability as a predictor of OFR-FFR disagreement
The occurrence of TCFA was numerically more frequent in the underestimation group (60%) as compared to the overestimation (19%) and reference group (36.3%). TCFA was not associated with NDOF in the unadjusted [−0.008 (95% CI: −0.022, 0.007); P=0.288] nor the adjusted [−0.007 (95% CI: −0.023, 0.008); P=0.366] regression models.
Sensitivity analysis
Sensitivity analysis further adjusting for MLA, FFR and ACS/non-ACS were performed, these variables did not change the associations found in Table 2 (Table 3).
Table 3
Parameter | Adjusted for MLA (95% CI) | P | Adjusted for ACS/non-ACS (95% CI) | P | Adjusted for FFR (95% CI) | P |
---|---|---|---|---|---|---|
LPV/100 (mm3) | −0.026 (−0.045, −0.007) | 0.008 | −0.031 (−0.050, −0.011) | 0.002 | −0.036 (−0.055, −0.018) | <0.001 |
CPV/100 (mm3) | 0.023 (−0.016, 0.062) | 0.243 | 0.027 (−0.012, 0.066) | 0.171 | 0.015 (−0.022, 0.052) | 0.437 |
FPV/100 (mm3) | −0.004 (−0.014, 0.006) | 0.445 | 0.002 (−0.012, 0.008) | 0.726 | 0.003 (−0.006, 0.013) | 0.478 |
TCFA | −0.006 (−0.021, 0.010) | 0.477 | −0.007 (−0.020, 0.010) | 0.492 | −0.006 (−0.021, 0.008) | 0.396 |
MLA, minimal lumen area; FFR, fractional flow reserve; ACS, acute coronary syndrome; CI, confidence interval; LPV, lipid plaque volume; CPV, calcific plaque volume; FPV, fibrotic plaque volume; TCFA, thin-cap fibroatheroma.
Discussion
The main findings of the present study can be summarized as follows: (I) atherosclerotic plaque burden from lipid but not from calcific or fibrotic plaque influenced the numerical precision of computational OFR when using FFR as the reference standard. (II) Increasing lipid plaque burden was found to yield lower OFR than FFR with an NDOF of −0.028 (−0.047, −0.009) for every 100 mm3 increase in lipid plaque volume. (III) Occurrence of TCFA was more pronounced when OFR underestimated FFR but TCFA was not an independent predictor of the NDOF. Finally, in sensitivity analysis it was observed that adjusting for MLA, FFR and ACS/non-ACS did not change the association between plaque composition and NDOF.
The relationship observed between coronary physiology and atherosclerotic plaque in the present study is largely in line with prior fluid-structure reports (11). Pressure-derived FFR is mainly determined by the epicardial pressure gradient during vasodilator-induced hyperemia. The vasodilatory capacity is dependent on the integrity of the internal elastic lamina and changes following the progression of atherosclerosis (12). This was previously exemplified in a bench study where it was found that plaques consisting of stiff materials required more external work to reach the same degree of deformation as compared to soft plaque models (13). Hence, lipidic plaque would have a larger deformation than fibrous plaques and calcific plaques with identical stenosis geometry and fibrous cap thickness, which may result in different FFR values. This is supported by a fluid-structure interaction analysis showing a greater cyclic lumen dilatation and larger strain in deformable lipid-rich plaques than in rigid fibrous and calcific plaques, which lead to different FFR values (11). It is therefore plausible that our findings are explained by the alterations in the elasticity of the intima/internal elastic membrane. High LPV may result in larger luminal deformation and thereby enlargement of the vessel capacity, leading to numerical higher FFR values (13), whereas OFR is underestimated due to the assumption of rigid vessel wall in the computation. An increasing interest in quantifying LPV in relation to FFR measurements has evolved. Previous studies documented a negative association between LPV and FFR using non-invasive and invasive methods (14,15). These findings were further confirmed by Driessen and colleagues who found low attenuating plaques to be an independent predictor of abnormal FFR following adjustment for stenosis severity (16). Importantly, we did not assess the direct association between plaque composition and FFR and therefore, our findings are not directly relatable to previous studies. Furthermore, it is important to recognize the differences in evaluating plaque morphology using coronary computed tomography angiography (CTA) vs. OCT. Paired coronary CTA, OCT and physiology measurements should be obtained before concluding on this matter. Our findings add to the existing literature illustrating that lipidic burden must be considered when evaluating physiological indices. However, the correlation between LPV and NDOF was weak and underlines that the discrepancy between OFR and FFR is multifactorial. In clinical practice, FFR may be affected by factors including pharmacological treatment, left ventricular hypertrophy, microvascular disease or tachycardia that cannot be fully reflected by OFR (17-19). Despite the risk of other factors affecting NDOF, we only saw a minor correction in the adjusted analysis indicating little residual confounding for the point estimate. However, we were not able to adjust for all relevant factors.
High-risk plague features
In addition to a correlation of high LPV and NDOF, a larger frequency of TCFA was observed in the underestimation group. However, following adjusted multivariable analysis, the point estimate for NDOF changed from −0.008 [(95% CI: −0.022; 0.007); P=0.288] to −0.007 [95% CI: −0.023, 0.008); P=0.366] and therefore the present analysis does not support TCFA as an independent predictor of OFR-FFR discrepancy. This may be explained by potential collinearity between high LPV and TCFA, as TCFA is defined from the presence of widespread lipidic plaques (10,20). Therefore, we cannot formally exclude that the overrepresentation of TCFAs is due to a larger lipid pool. On the other hand, a true overrepresentation of TCFAs was observed and the characteristics of this plaque type may be the actual cause of OFRs underestimation of FFR in vessels with lipid plaques. The driver behind this could potentially be endothelial dysfunction as is often seen in large necrotic cores causing more pronounced vasoconstriction (21,22). Some degree of vasoconstriction is apparent even in relatively normal sections in vessels with TCFA because of the systemic effects caused by endothelial dysfunction (23). The downstream vasoconstriction due to these systemic effects may result in numerical higher FFR values and this may give a mismatch with OFR because rigid vessel wall was assumed in OFR computation. Therefore, we cannot rule out that OFR may reflect a truer lower FFR value than wire-based FFR. In addition, vessels with TCFA have thin cap thickness which increases wall strain during the cardiac cycle and thereby enlargement of the vessel capacity (13), which leads theoretically to higher FFR values compared with normal vessels without TCFA.
Micro- and collateral circulation
In the presented data some cases with low LPV still had OFR values that underestimated FFR. A possible explanation for these cases could be coronary microvascular disease (CMD) by OFR and FFR. Inducible ischemia may be caused by CMD in the absence of a severe epicardial coronary artery stenosis. The computational algorithm of OFR ignores the microcirculatory status because an empirical hyperemic flow velocity is incorporated in the computational algorithm (7). Hence, OFR may provide a precise measure of the epicardial disease but not reflect FFR in cases with CMD (19). Another factor that may explain our findings is the presence of collateral circulation. The formation of collateral circulation increases the flow in severely stenotic vessels as “natural bypasses from normal coronary arteries” (24). The OFR algorithm accounts for side branch areas (25), but cannot adjust for altered flow caused by competing collateral circulation. Despite accounting for factors potentially associated with CMD (diabetes, previous MI) (26), we were not able to adjust our regression models for true CMD and collateral circulation status. Hence, both CMD and increased collateral flow may have influenced the OFR-FFR disagreement towards null in the underestimation group and therefore there is a possibility that the actual contribution of plaque volumes is underestimated.
Clinical implications
Despite that the overall agreement is good, FFR and OFR have different pros and cons (27). We demonstrated that plaque composition may explain some of the variations between the two methods. However, the clinical impact is limited with large increases in e.g., LPV giving rise to minor changes in NDOF. Recent reports have shown that diagnosing and treating high-risk plaques could be a key element in predicting outcomes in patients with coronary artery disease—even in patients with non-obstructive disease as defined by FFR (28,29). Hence, OFR measurement, combined with plaque characterization, may provide a valuable instrument for the clinician when performing stent planning since it provides both anatomical and functional information about what, where and how to treat. OFR may be of particular interest in those patients who are currently treated with optimal medical therapy due to a negative FFR but with high-risk plaques identified by intravascular imaging. In summary, we might not have to dichotomize the use of the two tools for the same situations but accept that they co-exist and could be of use in different kinds of situations. Future studies should focus on how to implement both anatomy and physiology in one simple guiding strategy.
Limitations
The retrospective design of the study should be acknowledged as a potential source of bias, however, all patients in the original study were included in this sub-analysis. Due to the retrospective nature of the study, there is a risk for residual confounding despite adjustment for relevant factors in the multivariate analysis. Furthermore, the patients were not consecutively enrolled and therefore there is a risk of a selection bias. ACS patients were enrolled in the trial which may give a risk of misclassification between thrombus and lipidic plaque on OCT. However, a validation between the software and international OCT experts was performed previously. Secondly, the relationship between coronary flow and plaque characteristics is unclear. In addition, although the plaque morphology was derived from an externally validated method (8), the estimated LPV might still be deviated from the real situation due to the limited penetration depth of infrared. Besides, the vessel-level instead of lesion-level plaque morphology were investigated in this study considering the fact that no lesion-level FFR data were available. However, only the OCT images covering the whole lesion were included in the current study, thus the impact of un-imaged proximal lesion could be neglected. Further lesion level-based research is warranted to assess the deformation and coronary flow change by plaque components and morphologic features in the local stenosis segment.
Conclusions
High lipid burden leads to a minor underestimation of FFR when OFR is used to assess the hemodynamic importance of intermediate coronary artery stenosis. TCFA, calcific and fibrotic plaques were not independent variables explaining differences between OFR and FFR. Future studies should focus on how to merge the anatomical and physiological data that can be acquired by a single OFR pullback into a simplified treatment strategy.
Originality and clinical impact
NDOF was low in the present study and only very large lipidic plaques provided a minor numerical difference. OFR incorporating plaque morphology is capable of simultaneously physiological and anatomical assessment and provides a novel valuable guiding tool for the physician to use in coronary stent implantation.
Acknowledgments
Funding: This work was supported by the National Key Research and Development Program of China; and the Science and Technology Commission of Shanghai Municipality (grant number 19DZ1930600 to ST); and the Natural Science Foundation of China (grant number 82020108015 and 81871460 to ST); and the Science Foundation Ireland (15/RP/2765 to WW and JH).
Footnote
Reporting Checklist: The authors have completed the STROBE reporting checklist. Available at https://cdt.amegroups.com/article/view/10.21037/cdt-21-505/rc
Data Sharing Statement: Available at https://cdt.amegroups.com/article/view/10.21037/cdt-21-505/dss
Conflicts of Interest: All authors have completed the ICMJE uniform disclosure form (available at https://cdt.amegroups.com/article/view/10.21037/cdt-21-505/coif). ST serves as an unpaid editorial board member of Cardiovascular Diagnosis and Therapy and reports grants from Pulse medical imaging technology during the conduct of the study. WW reports grants and personal fees from MicroPort, other from Rede Optrimus Research, other from Argonauts, outside the submitted work. The other authors have no conflicts of interest to declare.
Ethical Statement: The authors are accountable for all aspects of the work in ensuring that questions related to the accuracy or integrity of any part of the work are appropriately investigated and resolved. The study was conducted in accordance with the Declaration of Helsinki (as revised in 2013). The institutional review board of Wakayama Medical University approved the post hoc analysis of the data for this study (No. 2876) and patients’ informed consent was waived due to the retrospective nature of the study.
Open Access Statement: This is an Open Access article distributed in accordance with the Creative Commons Attribution-NonCommercial-NoDerivs 4.0 International License (CC BY-NC-ND 4.0), which permits the non-commercial replication and distribution of the article with the strict proviso that no changes or edits are made and the original work is properly cited (including links to both the formal publication through the relevant DOI and the license). See: https://creativecommons.org/licenses/by-nc-nd/4.0/.
References
- Neumann FJ, Sousa-Uva M, Ahlsson A, et al. 2018 ESC/EACTS Guidelines on myocardial revascularization. Eur Heart J 2019;40:87-165. [Crossref] [PubMed]
- Zimmermann FM, Omerovic E, Fournier S, et al. Fractional flow reserve-guided percutaneous coronary intervention vs. medical therapy for patients with stable coronary lesions: meta-analysis of individual patient data. Eur Heart J 2019;40:180-6. [Crossref] [PubMed]
- Tonino PA, De Bruyne B, Pijls NH, et al. Fractional flow reserve versus angiography for guiding percutaneous coronary intervention. N Engl J Med 2009;360:213-24. [Crossref] [PubMed]
- Pijls NH, Fearon WF, Tonino PA, et al. Fractional flow reserve versus angiography for guiding percutaneous coronary intervention in patients with multivessel coronary artery disease: 2-year follow-up of the FAME (Fractional Flow Reserve Versus Angiography for Multivessel Evaluation) study. J Am Coll Cardiol 2010;56:177-84. [Crossref] [PubMed]
- Xu B, Tu S, Qiao S, et al. Diagnostic Accuracy of Angiography-Based Quantitative Flow Ratio Measurements for Online Assessment of Coronary Stenosis. J Am Coll Cardiol 2017;70:3077-87. [Crossref] [PubMed]
- Westra J, Tu S. Overview of Quantitative Flow Ratio and Optical Flow Ratio in the Assessment of Intermediate Coronary Lesions. US Cardiology Review 2020;14:e09. [Crossref]
- Yu W, Huang J, Jia D, et al. Diagnostic accuracy of intracoronary optical coherence tomography-derived fractional flow reserve for assessment of coronary stenosis severity. EuroIntervention 2019;15:189-97. [Crossref] [PubMed]
- Chu M, Jia H, Gutiérrez-Chico JL, et al. Artificial intelligence and optical coherence tomography for the automatic characterisation of human atherosclerotic plaques. EuroIntervention 2021;17:41-50. [Crossref] [PubMed]
- Huang J, Emori H, Ding D, et al. Diagnostic performance of intracoronary optical coherence tomography-based versus angiography-based fractional flow reserve for the evaluation of coronary lesions. EuroIntervention 2020;16:568-76. [Crossref] [PubMed]
- Araki M, Yonetsu T, Kurihara O, et al. Predictors of Rapid Plaque Progression: An Optical Coherence Tomography Study. JACC Cardiovasc Imaging 2021;14:1628-38. [Crossref] [PubMed]
- Wu X, von Birgelen C, Zhang S, et al. Simultaneous evaluation of plaque stability and ischemic potential of coronary lesions in a fluid-structure interaction analysis. Int J Cardiovasc Imaging 2019;35:1563-72. [Crossref] [PubMed]
- Wang JC, Bennett M. Aging and atherosclerosis: mechanisms, functional consequences, and potential therapeutics for cellular senescence. Circ Res 2012;111:245-59. [Crossref] [PubMed]
- Wu X, von Birgelen C, Li Z, et al. Assessment of superficial coronary vessel wall deformation and stress: validation of in silico models and human coronary arteries in vivo. Int J Cardiovasc Imaging 2018;34:849-61. [Crossref] [PubMed]
- Ahmadi A, Leipsic J, Øvrehus KA, et al. Lesion-Specific and Vessel-Related Determinants of Fractional Flow Reserve Beyond Coronary Artery Stenosis. JACC Cardiovasc Imaging 2018;11:521-30. [Crossref] [PubMed]
- Murai K, Kataoka Y, Nakaoku Y, et al. The association between the extent of lipidic burden and delta-fractional flow reserve: analysis from coronary physiological and near-infrared spectroscopic measures. Cardiovasc Diagn Ther 2021;11:362-72. [Crossref] [PubMed]
- Driessen RS, Stuijfzand WJ, Raijmakers PG, et al. Effect of Plaque Burden and Morphology on Myocardial Blood Flow and Fractional Flow Reserve. J Am Coll Cardiol 2018;71:499-509. [Crossref] [PubMed]
- Götberg M, Cook CM, Sen S, et al. The Evolving Future of Instantaneous Wave-Free Ratio and Fractional Flow Reserve. J Am Coll Cardiol 2017;70:1379-402. [Crossref] [PubMed]
- Kwasiborski PJ, Czerwiński W, Kowalczyk P, et al. Influence of heart rate on FFR measurements: An experimental and clinical validation study. Int J Cardiol 2020;317:13-7. [Crossref] [PubMed]
- Elgendy IY, Conti CR, Bavry AA. Fractional flow reserve: an updated review. Clin Cardiol 2014;37:371-80. [Crossref] [PubMed]
- Moreno PR. The high-risk thin-cap fibroatheroma: a new kid on the block. Circ Cardiovasc Interv 2009;2:500-2. [Crossref] [PubMed]
- Naghavi M, Libby P, Falk E, et al. From vulnerable plaque to vulnerable patient: a call for new definitions and risk assessment strategies: Part I. Circulation 2003;108:1664-72. [Crossref] [PubMed]
- Lavi S, Yang EH, Prasad A, et al. The interaction between coronary endothelial dysfunction, local oxidative stress, and endogenous nitric oxide in humans. Hypertension 2008;51:127-33. [Crossref] [PubMed]
- Lavi S, Bae JH, Rihal CS, et al. Segmental coronary endothelial dysfunction in patients with minimal atherosclerosis is associated with necrotic core plaques. Heart 2009;95:1525-30. [Crossref] [PubMed]
- Seiler C. The human coronary collateral circulation. Eur J Clin Invest 2010;40:465-76. [Crossref] [PubMed]
- Karanasos A, Tu S, van Ditzhuijzen NS, et al. A novel method to assess coronary artery bifurcations by OCT: cut-plane analysis for side-branch ostial assessment from a main-vessel pullback. Eur Heart J Cardiovasc Imaging 2015;16:177-89. [Crossref] [PubMed]
- Heusch G. Coronary microvascular obstruction: the new frontier in cardioprotection. Basic Res Cardiol 2019;114:45. [Crossref] [PubMed]
- De Maria GL, Garcia-Garcia HM, Scarsini R, et al. Novel Indices of Coronary Physiology: Do We Need Alternatives to Fractional Flow Reserve? Circ Cardiovasc Interv 2020;13:e008487. [Crossref] [PubMed]
- Stone GW, Maehara A, Ali ZA, et al. Percutaneous Coronary Intervention for Vulnerable Coronary Atherosclerotic Plaque. J Am Coll Cardiol 2020;76:2289-301. [Crossref] [PubMed]
- Pinilla-Echeverri N, Mehta SR, Wang J, et al. Nonculprit Lesion Plaque Morphology in Patients With ST-Segment-Elevation Myocardial Infarction: Results From the COMPLETE Trial Optical Coherence Tomography Substudys. Circ Cardiovasc Interv 2020;13:e008768. [Crossref] [PubMed]