Cardiovascular/stroke risk predictive calculators: a comparison between statistical and machine learning models
Introduction
Cardiovascular disease (CVD) is the leading cause of mortalities around the world which has accounted for the global deaths of 17.9 million people (1). Out of these 17.9 million, 85% of the deaths were attributed to myocardial infarction and stroke (1). Prevention of CVD/stroke events is an important challenge in front of the medical community, which is causing a higher global economic burden (2,3). Thus, there an increasing demand to provide accurate and time-efficient preventive tools that can provide a long-term prognosis of CVD/stroke events at an affordable cost to the patients. Atherosclerosis is one of the dominant causes of CVD/stroke events (4-10). One way to prevent the growth of atherosclerosis is to treat the risk factors which are responsible for the initiation and progression of atherosclerotic plaque. Besides controlling the risk factors, treating the arteries by measuring the atherosclerotic plaque extent and making the plans for its regression is also an established way of reducing the risk of CVD (11).
According to INTERHEART* and INTERSTROKE* studies [*as it is taken from the Yusuf et al. and O’Donnell et al. (12-14)], conventional cardiovascular risk factors (CCVRF) such as diabetes mellitus, hypertension, hyperlipidemia, smoking, alcohol consumption, physical inactivity, and body mass index are attributed to ~90% risk of CVD/stroke events (12-14). Current guidelines also suggest the treatment of all these modifiable risk factors for CVD prevention (15-19). Most of these guidelines take the help of statistically derived conventional cardiovascular risk calculators (CVRC) for initiation of CVD/stroke treatment plans (e.g., for initiation of statins) (15,17-21). Some commonly used statistically derived CVRC (CVRCStat) are the Framingham Risk Score (FRS), the UK Prospective Diabetes Score (UKPDS), the Reynold Risk Score (RRS), the Systematic Coronary Risk Evaluation Chart, the QRISK3 calculator, and the World Health Organization (WHO) are the commonly used CVD risk prediction models. All such CVRCStat reported a modest performance while providing a 10-year risk assessment especially in patients suffering from diabetes mellitus (22,23) and rheumatoid arthritis (24,25). Furthermore, it has been observed that most of CVRCStat provide overestimation or underestimation of CVD risk (26,27).
The reason for such sub-optimal performance of CVRCStat lies in their design. These CVRCStat were designed using statistical regression-based techniques that consider only a limited number of risk factors (28). These regression-based methods assume a linear relationship between the input risk factors and the outcome of interest (for example the CVD events) (26,29,30). However, in practice, the risk due to CVD depends upon the complex interaction between conventional cardiovascular risk factors (CCVRF) (31). Furthermore, these risk prediction models assume a limited or no interaction between the CCVRF itself, thus, this assumption ends-up in providing an oversimplified and approximate risk assessment model (26,29,30). Another important factor that may lead to the poor performance of CVRCStat is the exclusion of image-based phenotypes. The CCVRF may note provide the complete information about the atherosclerotic plaque components, which, however, can easily be captured using imaging modalities (32,33). Thus, there is a need to refine the search of risk prediction models that can provide more robust risk estimation by considering the non-linear interaction between a large number of a diverse set of input risk factors (33). Lastly, these models for CVRCStat never use cohort knowledge in their persistence layer (database layer) or to embed intelligence in their designs, which is so important for an accurate prediction of 10-year risk (34). Machine learning (ML)-has been actively pursued in medical imaging recently covering several anatomic areas such as liver cancer (35,36), lung cancer (37,38), prostate cancer (39,40) more recently in carotid plaque characterization (32-36). These techniques are generally the data-driven algorithms, which identify the complex interactions among the CCVRF to provide accurate CVD risk estimation (26,33). The ML-based techniques have plenty amount of applications in the medical domain including the CVD/stroke risk assessment (41-47). However, there were a few attempts were made to investigate the performance of ML-based CVD/Stroke risk stratification against to the CVRCStat (26,30,48-52). In this study, a Support Vector Machine (SVM) algorithm was adapted for ML-based CVD/stroke risk calculator (CVRCML). The proposed study had two main hypotheses for this study: (I) CVRCML can provide a better risk stratification compared to CVRCStat and (II) the inclusion of carotid ultrasound image-based phenotypes (CUSIP) into the CCVRF (so-called integrated systems) can provide a better predictive ability compared to the CCVRF alone, irrespective of the type of risk calculator (CVRCML or CVRCStat).
In order to prove these hypotheses, this study has three unique objectives. These are: To compare the performance of the 12 types of CVRCStat (conventional) and CVRCML (conventional); To compare the performance of the CVRCStat (integrated) and the CVRCML (integrated); To compare the performance of the CVRCML (conventional) and the CVRCML (integrated).
Definitions of CVRCStat (conventional), CVRCStat (integrated), CVRCML (conventional), and CVRCML (integrated)
It should be noted that (I) 12 types of CVRCStat (conventional) means statistically derived CVRCStat that used 14 CCVRF. These 12 types of CVRCStat (conventional) are: (i) the FRS, (ii) the UKPDS56, (iii) the UKPDS60, (iv) the RRS, (v) Atherosclerosis CVD (ASCVD) calculator, (vi) the NIPPON risk chart, (vii) the SCORE risk chart, (viii) the QRISK3, (ix) the WHO risk chart, (x) the PROCAM, (xi) FRS (Stroke), and (xii) my_stroke risk calculator (MSRC). (II) CVRCStat (integrated) means CVRCStat that used integrated risk factors, which were the combination of 14 CCVRF and 5 CUSIP. AtheroEdge Composite Risk Score version 1 (AECRS 1.0) (53-55) was the recently developed type of CVRCStat (integrated). In this study, we extended the AECRS 1.0 to AECRS 2.0 by further adding the status of chronic kidney disease (CKD). This will allow the physicians to perform the CVD/stroke risk stratification of patients suffering from CKD. In this study, AECRS 2.0 will be referred as CVRCStat (integrated). (III) CVRCML (conventional) means CVRCML that used 14 CCVRF, and (IV) CVRCML (integrated) means CVRCML that used integrated risk factors. Hereafter, throughout this study, the above definitions will be followed. The definition various 14 CCVRF and 5 CUSIP are provided in the “Method” section of this article.
Figure 1 shows the overall architecture of the proposed system that compares the CVD/stroke risk stratification performed using the ML-based approach against the risk stratification performed using 13 different types of statistically derived CVRC (12 types of CVRCStat (conventional) and CVRCStat (integrated)]. In this study, a combination of glycated hemoglobin (HbA1c) and maximum carotid intima-media thickness has been used as the event-equivalence gold standard (EEGS). Description of such type of combinational EEGS has been given in the “Discussion” section of this study.
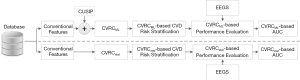
Methods
Study participants
This retrospective study recruited a Japanese ethnicity cohort of 202 patients between July 2009 and December 2010. All the patients were approved by the institutional review board of the Ohashi Medical Center, Toho University Japan, and informed consent was obtained from all the study participants. The proposed study is unique in its concept compared to all our previous studies that used a similar Japanese cohort (53-60). In total, 404 CUS scans (2 CUS scans × 202 patients) were collected from both the left and right common carotid arteries of 202 patients, out of which nine scans were excluded from this study due to the poor visualization. Thus, a total of 395 CUS scans were used in this study. All the CUS scans were retrospectively analyzed by two experts who had 15 years of experience in the field of radiology.
Carotid ultrasound image acquisition
The ultrasound image of the common carotid artery (CCA) was obtained by using a B-mode ultrasound scanner (Aplio XG, Xario, Aplio XV, Toshiba Inc., Tokyo, Japan) equipped with 7.5 MHz linear arrays of the transducer. In order to capture the ultrasound image and to measure the image-based phenotypes, a standardized protocol of the American Society of Echocardiography (ASE) was followed (61). Initially, the patients were examined in a supine position with their head tilted backward. At first, the carotid artery of the patients was identified by the transverse scan and then, once identified; the probe was rotated by 90º to capture the longitudinal image of walls of the carotid artery. The complete protocol for image acquisition was discussed in detail in our previous studies (53-60). The average image resolution was 0.0529 mm-per-pixel.
Conventional cardiovascular risk factors
In this study, 14 CCVRF including demographics and blood biomarkers were collected for each patient. These 14 CCVRF are (I) age, (II) gender, (III) systolic blood pressure, (IV) diastolic blood pressure, (V) status of hypertension, (VI) glycated hemoglobin (HbA1c), (VII) low-density lipoprotein cholesterol (LDL-C), (VIII) high-density lipoprotein cholesterol (HDL-C), (IX) total cholesterol (TC), (X) triglyceride (TG), (XI) a ratio of total cholesterol and high-density lipoprotein cholesterol (TC/HDL), (XII) smoking status, (XIII) family history of CVD in first-degree relative, and (XIV) estimated glomerular filtration rate (eGFR). The baseline characteristics of these conventional cardiovascular risk factors have been presented in the “Baseline characteristics” section.
Current image phenotype measurements and 10-year CUSIP prediction
Current CUSIP measurements
Besides the CCVRF, this study also measures five CUSIP such as (I) average cIMT (cIMTavecurr) (62), (II) maximum cIMT (cIMTmaxcurr) (62), (III) minimum cIMT (cIMTmincurr) (62), (IV) variability in cIMT (cIMTVcurr) (63,64), and (V) carotid total plaque area (cTPAcurr) (56,60,62,65-67). The subscript “curr” indicates the measurement of the corresponding CUSIP at the baseline. Two operators (experienced and novice) each having 15 years of experience in radiology measured all these five CUSIP from the 395 CUS scans using automated software (AtheroEdge, AtheroPointTM, Roseville, California, USA) (62,63,66,68,69). This software works in three stages: (I) automatic detection of the region-of-interest, (II) identification of far wall of the CCA followed by a delineation of the lumen-intima (LI) and media-adventitia (MA) interfaces, and (III) measurement of current CUSIP throughout the length of CUS scan. Such type of measurement is as the full-length measurement (62,63,66,68-70). Note that our cIMT measurements consider both basic cIMT and plaque burden if any.
The cIMTavecurr was then measured as the mean polyline distance between the 100 connected set of points taken on LI and MA interfaces (71,72). Polyline distance metric was used to measure the perpendicular distance between the vertex on the LI interface and the corresponding polyline segment on the ML interfaces. Since the plaque growth in bidirectional [so-called Glagov’s Phenomenon (73)], the distance was also measured between the vertex on the MA interface and the corresponding polyline segment on the LI interface (71,72). All the distances were then averaged together to obtain the cIMTavecurr. Similarly, cIMTmaxcurr and cIMTmincurr were computed as the maximum and minimum distance, respectively, between LI and MA borders. The cIMTVcurr is a recently developed marker of variations cIMT (63,64). The procedure for measuring cIMTVcurr has been discussed in our recent study (63,64). In this study, we automatically measured the carotid plaque area as the sum of all pixels encapsulated within the envelope of LI and MA interfaces that spanned all along the length of the CUS scan (56,60). Note that since this plaque follows the morphology of the atherosclerotic plaque variation, it was also termed as morphological total plaque area (mTPA) (60) or cTPAcurr (56,60). It must be noted that the cTPAcurr is comprised of the focal thickening region which is more than 50% of the surrounding IMT (61,74,75) or the region where cIMTmax >1.5 mm (61,74-78).
10-year CUSIP measurements
The five types of 10-year CUSIP (cIMTave10-year, cIMTmax10-year, cIMTmin10-year, cIMTV10-year, and cTPA10-year) are predicted using the standardized annual progression rates of cIMT and carotid plaque area (53,54,58,79) when applied on the original five types of image-based risk factors. The subscript ‘10-year’ indicates the 10-year prediction of the phenotype. These five types of 10-year CUSIP are computed by integrating the five types of current CUSIP (cIMTavecurr, cIMTmaxcurr, cIMTmincurr, cIMTVcurr, and cITPAcurr) with the 11 CCVRF (age, ethnicity, gender, carotid artery type, HbA1c, LDL-C, total cholesterol (TC), SBP, smoking, BMI, and estimated glomerular filtration rate or eGFR). Given the annual progression rates of cIMT due to all 11 CCVRF, an overall increase in cIMT from the current value, in 10-years, was computed. In the current study, the eGFR blood biomarker was used for the 10-year CVD/stroke risk prediction.
Statistically derived 10-year CVD/stroke risk calculators
In this study, the 10-year CVD/stroke risk was computed using 13 types of CVRCStat. These 13 types of CVRCStat are: (I) FRS (80), (II) UKPDS56 (81), (III) UKPDS60 (82), (IV) RRS (83), (V) Pooled Cohort Risk Score (PCRS) (15), (VI) NIPPON risk chart (84), (VII) SCORE risk chart (85), (VIII) QRISK3 (86), (IX) World Health Organization (WHO) risk chart (87,88)), (X) PROCAM (89), (XI) FRS (Stroke) (90), (XII) MSRC (91), and (XIII) AECRS2.0. Out of 13, the first 12 types of CVRCStat (conventional) are purely based on the 14 CCVRF and they do not consider image-based biomarkers of CVD/stroke (for example cIMT and cTPA) into their risk estimation model. Recently, Khanna et al. (54,55) made a first-ever attempt to bridge this gap between CCVRF and image-based phenotypes by developing an integrated 10-year risk calculator called AECRS1.0. Khanna et al. (53) further evaluated the performance of AECRS1.0 against the 10 types of CVRCStat (conventional) and reported a higher predictive ability (AUC 0.927, P<0.001) of the AECRS1.0 compared to other 10 types of CVRCStat (conventional).
In our proposed study, the AECRS1.0 was extended by adding an eGFR into the risk prediction model (so-called AECRS2.0). Earlier studies have reported a strong link between CVD/stroke and CKD (92,93). Hence, the inclusion of eGFR in the risk prediction model of AECRS2.0 provided an additional benefit to the physicians, while evaluating the 10-year CVD/stroke risk in patients suffering from CKD. The AECRS2.0 is considered as the 13th type of CVRCStat. Since the AECRS2.0 considers the integrated risk factors (CCVRF + CUSIP), we refer to it as CVRCStat (integrated). Although a set of input risk factors used for the development of all these 13 types of CVRCStat (12 types of CVRCStat (conventional) and one type of CVRCStat (integrated)] is different, a common thread links all these CVRCStat under one category. This common thread is the use of statistics or the statistical methods for the development of this CVRCStat. In our proposed study we are comparing the CVD/stroke risk stratification of patients performed using 13 different types of CVRCStat and the automated CVRCML. In short, this study compared the ML-based method vs. the statistical-based methods of CVD/stroke risk stratification.
Machine learning-based risk stratification
The generalized architecture of the ML-based risk stratification system is shown in Figure 2. The overall system architecture is divided into three parts: (I) offline system (or training model), (II) online system (testing model), and (III) performance evaluation. At first, the database was partitioned using a 10-fold cross-validation protocol (so-called K10). The details about the working of the K10 protocol have already been discussed in our previous studies (47,94-96).
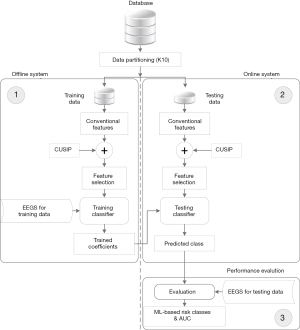
The offline ML-based system then extracts 14 conventional features (gender, age, HbA1c, LDL, HDL, TC, TG, LR, HT, SBP, DBP, smoking FH, and eGFR) from the training database. It should be noted that in order to investigate the effect of current CUSIP on the 10-year risk stratification of patients, a dotted plug-and-play block of CUSIP has been shown in Figure 2. The training features along with EEGS will then be used to train the SVM-based ML classifier. Once the SVM-based ML classifier is trained, the offline training coefficients are then used to transform the online test features into the high-risk or low-risk category for the patients using the online SVM classifier. Such an online ML system can then be utilized in routine preventive CVD/stroke prognosis systems without the requirement of EEGS. The performance of the proposed ML-based risk stratification system can be evaluated using the area-under-the-curve (AUC) metric, which is most commonly employed in medical studies (97-99).
Experimental protocol
The hypothesis of the proposed study is based on three types of experiments shown in Figure 3.
Experiment 1: 12 types of CVRCStat against CVRCML utilizing 14 types of CCVRF
In this experiment (Figure 3A), the performance of the ML-based calculator [CVRCML (conventional)] was compared against the 12 types of CVRCStat (conventional) using 14 CCVRF. Here, we hypothesize that, given the same 14 CCVRF, ML-based calculator [CVRCML (conventional)] provides a better CVD/stroke risk stratification compared to the 12 types of statistically derived conventional calculators [CVRCStat (conventional)].
Experiment 2: CVRCStat (integrated) vs. CVRCML (integrated) with integrated risk factors
In this experiment (Figure 3B), the performance of ML-based calculator [CVRCML (integrated)] was compared against the CVRCStat (integrated) using integrated risk factors (14 CCVRF + 5 CUSIP). Here we hypothesize that, given the same integrated risk factors, ML-based calculator [CVRCML (integrated)] provides a better CVD/stroke risk stratification compared to the statistically derived CVRC Stat (integrated). Note that as explained in the “Introduction” section, CVRCStat (integrated) means the AECRS2.0 calculator.
Experiment 3: CVRCML (conventional) with 14 CCVRF vs. CVRCML (integrated) with 19 integrated risk factors
In this experiment (Figure 3C), we hypothesize that ML-based calculator with integrated risk factors CVRCML (integrated) can provide a better CVD/stroke risk compared to the ML-based calculator with 14 CCVRF [CVRCML (conventional)]. This experiment showed the higher predictive ability of the integrated risk factors compared to the CCVRF alone.
Statistical analysis
All types of statistical analyses were performed using SPSS23.0 and MATLAB2017b. The baseline characteristics shown in Table 1 are expressed in mean ± standard deviation for continuous variable and as a percentage for the categorical variable. Two sample students’ t-test and chi-square tests were used for continuous variable and categorical variables, respectively. The receiver operating characteristics (ROC) curve was used to compare the performance of CVD/stroke risk stratification performed using the CVRCML and the 13 types of CVRCStat [12 types of CVRCStat (conventional) and one CVRCStat (integrated)]. Area-under-the-curve (AUC) was computed for all the 13 types of CVRCStat and the ML-based system. In order to obtain the ROC curve and to compute the AUC value, a combination of HbA1c and the cIMTmaxcurr was used as a response variable. Such type of response variable, which is also called an event-equivalence gold standard (EEGS), has already been studied in our previous publications (33,53,55,58,59,70,79). This is because this EEGS is associated with the atherosclerosis process and mimics the characteristics of the true endpoint such as CVD/stroke events (70,79). Although AUC was used as a primary performance evaluation (PE) metric, additional PE metrics like sensitivity, specificity, positive predictive value (PPV), negative predictive value (NPV), and accuracy were also computed using the same EEGS. The level of statistical significance was tested for P<0.05.
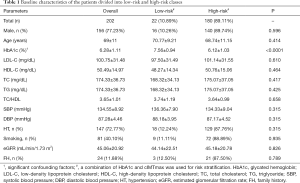
Full table
Results
Baseline characteristics
The baseline characteristics of the study participants are shown in Table 1. A cohort of 202 Japanese ethnicity patients was recruited in this study with a mean age of 69±11years (range, 29–88 years). Out of 202 patients, 156 (77.23%) were males and 46 (22.77%) were females. The average value of HbA1c was 6.28±1.11% (range, 4.80–13%), LDL-C was 100.75±31.48 mg/dL (range, 24–193 mg/dL), HDL-C was 50.49±14.97 mg/dL (range, 18–115 mg/dL), TC was 174.33±36.73 mg/dL (range, 61–255 mg/dL), TG was 117.10±56.69 mg/dL (range, 32–255 mg/dL), and eGFR was 45.06±20.92 mL/min/1.73 m2 (range, 3–110 mL/min/1.73 m2). Out of 202 patients, 49 (24.26%) were suffering from type 2 diabetes mellitus, 162 (80.20%) were suffering from chronic kidney disease, 147 (72.77%) were hypertensive, 81 (40.10%) were smokers, and 24 (11.88%) patients had a family history of coronary heart disease in the first degree relatives. The criteria for type 2 diabetes mellitus was HbA1c ≥6.5% or treatment for hyperglycemia (100), criteria for hypertension was SBP ≥140 mmHg or DBP ≥90 mmHg or treatment with antihypertensive medications (101), and the criteria for chronic kidney disease was EGFR <60 mL/min/1.73 m2 (102). As shown in Table 1, all the patients were further classified into two risk categories using an event equivalence gold standard (a combination of HbA1c ≥6.5% and cIMTmax ≥1.5 mm). The risk of category-based baseline characteristics is presented in Table 1.
10-year CVD/stroke risk stratification: 12 types of CVRCStat (conventional)vs. CVRCML (conventional)
The ROC curves are shown in Figure 4A compared to the performance of the 12 types of CVRCStat (conventional) and CVRCML (conventional) using the 14 types of CCVRF. The CVD/stroke risk stratification performed using CVRCML (conventional) reported the higher AUC value (AUC 0.70, P=0.11) compared to the mean AUC value (AUC 0.62, P<0.05) computed from 12 types of CVRCStat (conventional), indicating an overall improvement by ~13%. From Figure 4A, it is clear that the CVRCML (conventional) provides higher predictive power and a better or comparable risk stratification compared to the 12 types of CVRCStat (conventional).
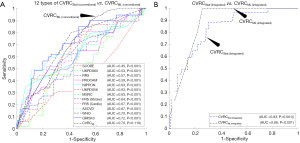
10-year CVD/stroke risk stratification: CVRCStat (integrated)vs. CVRCML (integrated)
The ROC curves are shown in Figure 4B compared to the performance of the CVRCStat (integrated) against CVRCML (integrated) using the integrated risk factors (14 CCVRF + 5 CUSIP). The CVD/stroke risk stratification performed using CVRCML (integrated) reported the higher AUC value (AUC 0.88, P<0.001) compared to the AUC of CVRCStat (integrated) (AUC 0.83, P<0.001), indicating an overall improvement by ~6%. It should be noted that the CVRCStat (integrated) with integrated risk factors is nothing but the AECRS2.0 calculator. Figure 4B further validated our hypothesis of better risk stratification using CVRCML (integrated) compared to the CVRCStat (integrated).
Comparison between CVRCML (conventional) and CVRCML (integrated): effect of image-phenotypes
Figure 5 explicitly compares the performance of two types of ML-based CVRC: (I) CVRCML (conventional) and (II) CVRCML (integrated). The inclusion of integrated risk factors into the ML-based risk calculator has resulted in a higher AUC value (AUC 0.88, P<0.001) compared to the ML-based risk calculator with conventional risk factors alone (AUC 0.70, P=0.11), indicating an overall improvement of ~26%. The improvement in AUC value is in line with a recent study presented by our group (79). This experiment showed that the integrated risk factors have higher predictive ability, not only in statistically derived risk calculators but also with the ML-based risk calculators. The reason for the higher predictive ability of the integrated risk factors is due to the integration of CUSIP with the CCVRF. The CUSIP truly reflect the carotid atherosclerotic plaque burden of the patients and thus, provide better risk estimation of the patients.
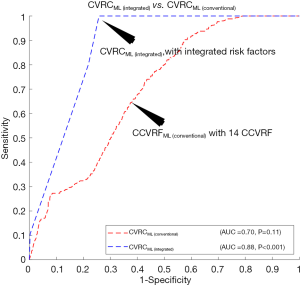
Sensitivity analysis and performance evaluation
In this study, the 10-year CVD/stroke risk was computed using 13 types of CVRCStat which were either derived using proportional hazard or regression-based methods (15,80-84,86,89,91,103), it is important to investigate the sensitivity of 10-year risk if the coefficients are varied. In our study, we have varied the coefficients of these risk calculators by ±2% and recorded the variation in AUC values. It should be noted that all 13 types of CVRCStat showed a variation of less than 5% in the overall AUC value. The average sensitivity, specificity, PPV, NPV, and accuracy for the 10-fold cross-validation (I) for the 12 types of CVRCStat (conventional) were 34%, 80.47%, 14.72%, 93.38%, and 76.74%, respectively, (II) for the CVRCML (conventional) were 23.86%, 93.01%, 23.08%, 93.29%, and 87.43%, respectively, (III) for the CVRCStat (integrated) were 69.89%, 75.60%, 20.10%, 96.62%, and 75.14%, respectively, and (IV) for the CVRCML (integrated) were 10.60%, 99.72%, 76.59%, 92.70%, and 92.52%, respectively. It should be noted sensitivity and PPV for all the CVRC is less than the specificity and NPV. This is because of the unbalanced nature of the database with only ~8% of samples were categorized into high-risk class. Due to the lower sensitivity values, it can be interpreted that the predictive ability of all the CVRC to correctly identify low-risk patients is more than the high-risk patients. The similar kind of observations of lower sensitivity values compared to septicity values was previously reported in multiple studies (26,51,104). Another observation from these PE metrics is that the overall accuracy for CVRCML (integrated) is highest amongst all the other CVRC (CVRCStat (conventional) or CVRCStat (integrated) or CVRCML (conventional)]. This has again validated our hypothesis of higher predictive ability of the integrated risk calculators compared to the CCVRF alone.
Discussion
Claims and summary
There were two hypotheses for this study: (I) the ML-based CVD/stroke risk calculator (CVRCML) can provide a better CVD/stroke risk stratification compared to statistically derived risk calculators (CVRCStat) and (II) the inclusion of CUSIP into the CCVRF can provide a higher predictive ability compared to the CCVRF alone, irrespective of the type of risk calculator (CVRCML or CVRCStat). The results are shown in Figure 4A,B, confirmed the first hypothesis of this proposed study. Given the 14 CCVRF (Figure 4A), CVRCML (conventional) provided a higher AUC value (AUC 0.70, P=0.11) compared to mean AUC of all the 12 types of CVRCStat (conventional), with an overall improvement of ~13%. Similarly, given the integrated risk factors (Figure 4B), CVRCML (integrated) provided a higher AUC value (AUC 0.88, P<0.001) compared to the AUC value of CVRCStat (integrated) (AUC 0.83, P<0.001), with an overall improvement of ~6%. The reason for this improved performance in ML-based risk calculators is their ability to consider a non-linear and complex relationship between the risk factors, which is, however, ignored by the conventional CVRCStat (conventional) (28,33). The results shown in Figure 5, confirmed the second hypothesis of this proposed study. The ML-based CVD/stroke risk calculator with integrated risk factors provided a higher AUC value (AUC 0.88, P<0.001) compared to an ML-based risk calculator with CCVRF (AUC 0.70, P=0.11), demonstrating an overall improvement of ~26%. This is because integrated risk factors combine the 5 CUSIP that provides additional information about the morphological variations in atherosclerotic plaque, which is, however, not well-captured by the CCVRF, alone (32,33,53,54,57-59,79). Such type of comparison between CVRCML with two different sets of input risk factors has been recently studied by our group (79). In conclusion, there is an improvement in risk stratification with CVRCML (integrated) (AUC 0.88, P<0.001) compared to all the CVRCStat (conventional) (AUC 0.62, P<0.05) with an improvement in an AUC value of ~42%. The improvement in CVD/stroke risk stratification is depicted in Figure 6. The overall sensitivity, specificity, PPV, NPV, and accuracy for the CVRCML (integrated) were 10.60%, 99.72%, 76.59%, 92.70%, and 92.52%, respectively.
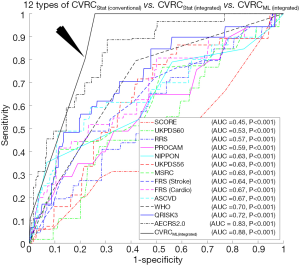
Benchmarking
Table 2 compares eight studies that compare the ML-based risk stratification against statistical calculator-based CVD/stroke risk assessment. As shown in Table 2, there are 12 attributes from column C1 to column C12 for each of the 8 studies (row R1 to row R8). Out of the eight studies, only three studies (row R4, row R7, and row R8) consider a combination of CCVRF and the CUSIP as input phenotypes. Two studies out of these three have used the CUS-based image phenotypes. The SVM is the most commonly used ML algorithm. It should be noted that, among all the eight studies, only the proposed study (row R8) compares the ML-based risk stratification results against the 13 types of statistically derived CVRC. The remaining seven studies (row R1 to row R7) either use FRS or ASCVD or UKPDS risk calculator for the comparison. The proposed study uses the integrated risk factors as the input to the ML-based algorithm. The inclusion of integrated risk factors is the main cause of an increase in the AUC value from 0.93 to 0.99, much stronger to other studies.
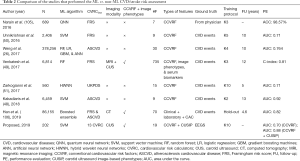
Full table
Use of event-equivalence gold standard for the risk stratification
Cerebrovascular and cardiovascular events are generally considered as the primary endpoints or gold standards for the CVD/stroke risk assessment and these are expensive and time-consuming (107-109). In order to provide an affordable CVD/stroke risk assessment solution to the physicians, low-cost preventive surrogate biomarkers need to be explored and refined (79). Surrogate biomarkers mimic the characteristics of the primary endpoints and thus can be considered as alternative gold standards. Furthermore, surrogate biomarkers (I) exhibit a link with the primary endpoints, (II) are clinically relevant, and (III) reproducible (110). Since such surrogate biomarkers provide equivalence to the primary gold standards, they are termed as EEGS (70,79). This EEGS needs to be evaluated using a small sample size, with lower-cost, and for short duration (107,108). The Food and Drug Administration of the USA has defined some of the commonly used surrogate biomarkers of CVD/stroke (111). Mancini et al. (112) also presented a study that reported a list of surrogate biomarkers of CVD. Since the patients are diabetic and have a moderate-to-high CVD risk profile, we have selected a combination of HbA1c and cIMTmax as the EEGS. Both the HbA1c and cIMTmax have a strong association with CVD/stroke events. Such types of EEGS were already been tested and evaluated in recent studies (53,54,58,59,79).
Therapeutic implications of risk stratification
Risk stratification assists the physicians while making the prognostic decision about the prevention of CVD/stroke events. The value of screening using AECRS2.0 or ML-based calculators can be more effective when computing 10-year risk, unlike the current risk as one can compute the contribution of individual risk factors and their joint associations which influences the overall CVD/stroke risk. For example, if the patients are suffering from diabetes, hyperlipidemia, hypertension, or CKD, then an appropriate combination of medications need to be prescribed for preventing the onset of CVD/stroke events. This is purely based on the contribution of individual risk category in which the patients belong. This is the point where the CVRC comes into the picture. These CVRC estimate the 10-year risk of CVD/stroke of the patients and further categorize them into appropriate risk profiles using predefined thresholds. Certain guidelines also take the assistance of these CVRC to recommend the initiation of statin therapy. The recent guidelines for the assessment of cardiovascular risk by the American College of Cardiology (ACC) and American Heart Association (AHA) recommended the use of low-to-moderate intensity statins if the 10-year risk computed using atherosclerotic CVD (ASCVD) calculator is ≥7.5% (15,113). The National Institute for Health and Care Excellence (NICE) guidelines recommended the use of 20 mg Atorvastatin if the 10-year risk computed using the QRISK2 calculator is ≥10% (20,21,113). Canadian guidelines recommended the statin initiation if the FRS is ≥10% (113-115). Since the CVRCML provides a better risk assessment of the patients compared to the statistically derived CVRCStat, they can accurately determine the statin eligibility of the patients (48,116). The ML-based risk stratification further prevents the excessive or unnecessary statins recommended by the CVRCStat to patients (48). This has been recently observed by the study presented by Kakadiaris et al. (48) who reported the use of statins to 46% (AUC 0.76) of the study population using the ACC/AHA calculator, while the SVM-based CVRCML recommended the statins to only 11.1% of their study population (AUC 0.92). Thus, compared to the statistically derived CVRC, the ML-based risk calculators can be employed for routine CVD/stroke risk assessment in clinical settings (116).
From the baseline characteristics, it is clear that the selected Japanese cohort belongs to the low-to-moderate risk category, with 49 patients (24.26%) was suffering from type 2 diabetes mellitus, 162 (80.20%) were suffering from chronic kidney disease, 147 (72.77%) were hypertensive. As discussed earlier, NICE (20,21), Canadian (115), and the recent ACC/AHA guidelines (15,16) favor the use of low-to-moderate intensity of statins for high-risk patients. This is also applicable for the patients stratified into the high-risk category using the ML-based CVD risk calculator. In cohorts with similar baseline characteristics, a treatment plan can further be modified based on the presence of risk factors like type 2 diabetes mellitus, hypertensive, dyslipidemia, and chronic kidney disease. For example besides statins therapy, high-risk patients suffering from type 2 diabetes mellitus can be treated with Metformin, Sulfonylureas (117,118). Similarly, high-risk patients suffering from hypertension can be treated angiotensin-converting enzyme (AEC), Angiotensin II receptor blockers (119), Renin-Angiotensin-Aldosterone System (RAAS) Blockade (120). Initial lipid-lowering medications like Atorvastatin, Simvastatin, and Pitavastatin can be used for controlling hyperlipidemia (121-124). However, the decision of an appropriate choice of medications is strictly based on the risk category of patients and the judgment made by physicians.
A note on sample size
In general, the selected data should not be identical. In our proposed study, samples were taken from both the left and right carotid artery of the same person. Although both left and right carotid arteries follow a similar genetic makeup and functionality of supplying oxygen-rich blood to the brain tissues, they work independently in two different pathways. Furthermore, due to the multi-focal and random nature of the atherosclerotic disease, the deposition of the plaque can be different in both the left and right carotid arteries. Thus, initially, total samples of 404 (202 patients × 2 CUS scans) non-identical samples were selected to perform various investigations in this study. Due to the poor image quality, nine CUS scans were omitted from this study and thus a total of 395 ultrasound B-mode carotid scans were finally selected for further statistical analysis. To investigate the validity of the selected sample size (i.e., 395 CUS scans), a power analysis was performed with confidence level of 95%, the margin of error (MoE) of 5%, and data proportion () of 0.5. The power analysis results in the desired sample size of 384. This means the selected sample size 395 samples is ~3% higher than the required sample size of 384. This validated the claim that the sample size of 395 was enough to perform this study. The detailed discussion on power analysis provided in the Supplementary files.
Strength, limitations, and future scope
Although we reported a robust and superior performance of the SVM-based CVRCML to risk stratify the patients, we intend to improvise the study in the following areas: (I) the proposed study needs to be validated in the database with varying ethnicities. This will help the physicians to understand the effect of ethnicity on the ML-based risk stratification of the patients. Except for QRISK3, nearly all the 13 types of CVRCStat were developed for specific types of ethnic groups. Thus, it would be interesting to know the behavior of the ML-based system and CVRCStat on the diverse ethnic cohorts. Besides ethnicity, it is also important to consider inflammatory markers such as erythrocyte sedimentation rate and the high-sensitivity C-reactive protein into the risk prediction algorithm (125-130). This is because these inflammatory markers have a strong association with CVD/stroke (125-130). Besides these intended improvisations, the proposed study is novel in its concept and perhaps the first study that compares the ML-based risk stratification with 13 types of CVRCStat. An influential effect of integrated risk factors has also been investigated in this study. Due to the inclusion of CUS scans and limited (but most common) number of risk factors, the proposed ML-based can be deployed in routine preventive CVD/stroke risk stratification applications at low-cost (79). The proposed study is a pilot investigation of the ML system in an environment of 13 types of CVRCStat. Further validation of this study can be tried to upgrade the current system having more robustness and independent of ethnicity. This retrospective pilot study can also be tested on a longitudinal dataset that actual cardiovascular or cerebrovascular events instead of EEGS. Lastly, hybrid classifiers can be built for better training design (35,131,132).
Conclusions
This study is a first-ever attempt for comparing the ML-based risk calculator against the 13 types of statistical CVRCs. The study hypothesized that machine learning in has better learning ability due to its ability to adjust the nonlinearity in risk factors and further, morphological plaque-based inclusions are representations of risk factors unlike the blood biomarkers alone. Based on these hypotheses, this study showed fulfillment of three objectives: ML-based calculators are more powerful than: (I) statistical calculators without integrated factors (~13% improvement) and (II) with integrated risk factors (6% improvement) such as AtheroEdge (AtheroPoint, Roseville, CA, USA) composite risk calculator, and (III) ML-based conventional risk calculators (26% improvement), respectively. Furthermore, the ML-based integrated calculator showed higher predictive power (42% improvement) compared to the mean predictive power of the 12 types of the conventional risk calculator. The overall risk-stratification accuracy for the ML-based integrated risk calculator was 92.52% which was higher than all other statistical calculators. Even though the system was designed on a particular ethnic group, it is generalized enough to be extended to other ethnicities, classification paradigms, and other event equivalent gold standards.
Supplementary
Power analysis
The primary aim of the power analysis is to determine a sample size which is essential to prove the hypothesis of the study. In this study, we hypothesized that (I) ML-based calculator can provide a better risk stratification compared to the statically derived conventional risk calculators and (II) inclusion of integrated risk factors into the risk prediction model can further improve the risk stratification ability, irrespective of the type of CVD risk calculator (statistical or ML-based). This study was designed for patients with Japanese ethnicity, thus, the population in power analysis refers to the samples derived from the Japanese patients (202 patients or i.e., 395 CUS scans) who need to be risk-stratified. The reason for considering the 395 CUS scans as samples have been discussed in the “A note on Sample Size” section. In order to perform the power analysis, we considered the 95% CI, 5% of the margin of error (MoE), and 0.5 as data proportion (
Acknowledgments
AtheroEdge™ and AtheroEdge Composite Risk Score (AECRS)™ are trademarks of AtheroPoint™, California, USA. The authors of this manuscript would like to thank the Ministry of Human Resource and Development, Government of India to provide financial support to conduct this study as a part of a PhD dissertation at Visvesvaraya National Institute of Technology, Nagpur, India.
Funding: None.
Footnote
Provenance and Peer Review: This article was commissioned by the Guest Editor (Luca Saba) for the series “Advanced Imaging in The Diagnosis of Cardiovascular Diseases” published in Cardiovascular Diagnosis and Therapy. The article was sent for external peer review organized by the Guest Editor and the editorial office.
Conflicts of Interest: All authors have completed the ICMJE uniform disclosure form (available at http://dx.doi.org/10.21037/cdt.2020.01.07). The series “Advanced Imaging in The Diagnosis of Cardiovascular Diseases” was commissioned by the editorial office without any funding or sponsorship. LS served as the unpaid Guest Editor of the series and serves as an unpaid editorial board member of Cardiovascular Diagnosis and Therapy from July 2019 to June 2021. JSS is affiliated to AtheroPointTM, focused in the area of stroke and cardiovascular imaging. The authors have no other conflicts of interest to declare.
Ethical Statement: The authors are accountable for all aspects of the work in ensuring that questions related to the accuracy or integrity of any part of the work are appropriately investigated and resolved. The study was conducted in accordance with the Declaration of Helsinki (as revised in 2013). The patients were approved by the institutional review board of Toho University, Japan and written consent was obtained from all the study participants. The study was approved by Toho University Japan (Ohashi Ethics Committee, Authorization No. 13-56).
Open Access Statement: This is an Open Access article distributed in accordance with the Creative Commons Attribution-NonCommercial-NoDerivs 4.0 International License (CC BY-NC-ND 4.0), which permits the non-commercial replication and distribution of the article with the strict proviso that no changes or edits are made and the original work is properly cited (including links to both the formal publication through the relevant DOI and the license). See: https://creativecommons.org/licenses/by-nc-nd/4.0/.
References
- Organization WH. WHO Cardiovascular disease. Available online: http://www.who.int/mediacentre/factsheets/fs317/en/
- Writing GM, Mozaffarian D, Benjamin E, et al. Heart Disease and Stroke Statistics-2016 Update: A report from the American Heart Association. Circulation 2016;133:e38. [PubMed]
- Benjamin Emelia J, Virani Salim S, Callaway Clifton W, et al. Heart Disease and Stroke Statistics—2018 Update: A Report From the American Heart Association. Circulation 2018;137:e67-e492. [PubMed]
- Libby P, Clinton SK. The role of macrophages in atherogenesis. Curr Opin Lipidol 1993;4:355-63. [Crossref]
- Libby P, Ridker PM, Maseri A. Inflammation and atherosclerosis. Circulation 2002;105:1135-43. [Crossref] [PubMed]
- Libby P. Vascular biology of atherosclerosis: overview and state of the art. Am J Cardiol 2003;91:3-6. [Crossref] [PubMed]
- Libby P, Ridker PM, Hansson GK. Progress and challenges in translating the biology of atherosclerosis. Nature 2011;473:317-25. [Crossref] [PubMed]
- Suri JS, Kathuria C, Molinari F. Atherosclerosis disease management. Springer: Science & Business Media, 2010.
- Trivedi R, Saba L, Suri JS. 3D Imaging Technologies in Atherosclerosis. New York: Springer, 2015.
- Saba L, Sanches JM, Pedro LM, et al. Multi-modality atherosclerosis imaging and diagnosis. New York: Springer, 2014.
- Spence JD, Hackam DG. Treating arteries instead of risk factors: a paradigm change in management of atherosclerosis. Stroke 2010;41:1193-9. [Crossref] [PubMed]
- Yusuf S, Hawken S, Ounpuu S, et al. Effect of potentially modifiable risk factors associated with myocardial infarction in 52 countries (the INTERHEART study): case-control study. Lancet 2004;364:937-52. [Crossref] [PubMed]
- O'Donnell MJ, Xavier D, Liu L, et al. Risk factors for ischaemic and intracerebral haemorrhagic stroke in 22 countries (the INTERSTROKE study): a case-control study. Lancet 2010;376:112-23. [Crossref] [PubMed]
- O'Donnell MJ, Chin SL, Rangarajan S, et al. Global and regional effects of potentially modifiable risk factors associated with acute stroke in 32 countries (INTERSTROKE): a case-control study. Lancet 2016;388:761-75. [Crossref] [PubMed]
- Goff DC, Lloyd-Jones DM, Bennett G, et al. 2013 ACC/AHA guideline on the assessment of cardiovascular risk: a report of the American College of Cardiology/American Heart Association Task Force on Practice Guidelines. J Am Coll Cardiol 2014;63:2935-59. [Crossref] [PubMed]
- Whelton PK, Carey RM, Aronow WS, et al. 2017 ACC/AHA/AAPA/ABC/ACPM/AGS/APhA/ASH/ASPC/NMA/PCNA Guideline for the Prevention, Detection, Evaluation, and Management of High Blood Pressure in Adults: Executive Summary: A Report of the American College of Cardiology/American Heart Association Task Force on Clinical Practice Guidelines. Hypertension 2018;71:1269-324. [Crossref] [PubMed]
- Expert Panel on Detection E. Executive summary of the Third Report of the National Cholesterol Education Program (NCEP) expert panel on detection, evaluation, and treatment of high blood cholesterol in adults (Adult Treatment Panel III). JAMA 2001;285:2486. [Crossref] [PubMed]
- Greenland P, Alpert JS, Beller GA, et al. 2010 ACCF/AHA guideline for assessment of cardiovascular risk in asymptomatic adults: a report of the American College of Cardiology Foundation/American Heart Association task force on practice guidelines developed in collaboration with the American Society of Echocardiography, American Society of Nuclear Cardiology, Society of Atherosclerosis Imaging and Prevention, Society for Cardiovascular Angiography and Interventions, Society of Cardiovascular Computed Tomography, and Society for Cardiovascular Magnetic Resonance. J Am Coll Cardiol 2010;56:e50-e103. [Crossref] [PubMed]
- Piepoli MF, Hoes AW, Agewall S, et al. 2016 European Guidelines on cardiovascular disease prevention in clinical practice: The Sixth Joint Task Force of the European Society of Cardiology and Other Societies on Cardiovascular Disease Prevention in Clinical Practice (constituted by representatives of 10 societies and by invited experts)Developed with the special contribution of the European Association for Cardiovascular Prevention & Rehabilitation (EACPR). Eur Heart J 2016;37:2315-81. [Crossref] [PubMed]
- National Institute for Health and Care Excellence. Surveillance report 2018 – Cardiovascular disease: risk assessment and reduction, including lipid modification (2014) NICE guideline CG181. London: National Institute for Health and Care Excellence (UK), 2018.
- Duerden M, O’Flynn N, Qureshi N. Cardiovascular risk assessment and lipid modification: NICE guideline. Br J Gen Pract 2015;65:378-80. [Crossref] [PubMed]
- Coleman RL, Stevens RJ, Retnakaran R, et al. Framingham, SCORE, and DECODE Risk Equations Do Not Provide Reliable Cardiovascular Risk Estimates in Type 2 Diabetes. Diabetes Care 2007;30:1292. [Crossref] [PubMed]
- McEwan P, Williams JE, Griffiths JD, et al. Evaluating the performance of the Framingham risk equations in a population with diabetes. Diabet Med 2004;21:318-23. [Crossref] [PubMed]
- Wahlin B, Innala L, Magnusson S, et al. Performance of the expanded cardiovascular risk prediction score for rheumatoid arthritis is not superior to the ACC/AHA Risk calculator. J Rheumatol 2019;46:130-7. [Crossref] [PubMed]
- Kremers HM, Crowson CS, Therneau TM, et al. High ten-year risk of cardiovascular disease in newly diagnosed rheumatoid arthritis patients: a population-based cohort study. Arthritis Rheum 2008;58:2268-74. [Crossref] [PubMed]
- Weng SF, Reps J, Kai J, et al. Can machine-learning improve cardiovascular risk prediction using routine clinical data? PLoS One 2017;12:e0174944. [Crossref] [PubMed]
- Taggar JS, Lip GY. The QRISK was less likely to overestimate cardiovascular risk than the Framingham or ASSIGN equations. ACP J Club 2008;148:25. [PubMed]
- Goldstein BA, Navar AM, Carter RE. Moving beyond regression techniques in cardiovascular risk prediction: applying machine learning to address analytic challenges. Eur Heart J 2017;38:1805-14. [PubMed]
- Suzuki S, Yamashita T, Sakama T, et al. Comparison of risk models for mortality and cardiovascular events between machine learning and conventional logistic regression analysis. PLoS One 2019;14:e0221911. [Crossref] [PubMed]
- Alaa AM, Bolton T, Di Angelantonio E, et al. Cardiovascular disease risk prediction using automated machine learning: A prospective study of 423,604 UK Biobank participants. PLoS One 2019;14:e0213653. [Crossref] [PubMed]
- Vishram JK. Prognostic interactions between cardiovascular risk factors. Dan Med J 2014;61:B4892. [PubMed]
- Boi A, Jamthikar AD, Saba L, et al. A Survey on Coronary Atherosclerotic Plaque Tissue Characterization in Intravascular Optical Coherence Tomography. Curr Atheroscler Rep 2018;20:33. [Crossref] [PubMed]
- Jamthikar A, Gupta D, Khanna NN, et al. A Special Report on Changing Trends in Preventive Stroke/Cardiovascular Risk Assessment Via B-Mode Ultrasonography. Curr Atheroscler Rep 2019;21:25. [Crossref] [PubMed]
- Suri JS. System and method for creating and using intelligent databases for assisting in intima-media thickness (IMT). Available online: https://patents.justia.com/patent/8805043
- Acharya UR, Sree SV, Ribeiro R, et al. Data mining framework for fatty liver disease classification in ultrasound: a hybrid feature extraction paradigm. Med Phys 2012;39:4255-64. [Crossref] [PubMed]
- Saba L, Dey N, Ashour AS, et al. Automated stratification of liver disease in ultrasound: An online accurate feature classification paradigm. Comput Methods Programs Biomed 2016;130:118-34. [Crossref] [PubMed]
- Than JC, Saba L, Noor NM, et al. Lung disease stratification using amalgamation of Riesz and Gabor transforms in machine learning framework. Comput Biol Med 2017;89:197-211. [Crossref] [PubMed]
- El-Baz A, Suri JS. Lung imaging and computer aided diagnosis. Boca Raton (FL): CRC Press, 2011.
- Pareek G, Acharya UR, Sree SV, et al. Prostate tissue characterization/classification in 144 patient population using wavelet and higher order spectra features from transrectal ultrasound images. Technol Cancer Res Treat 2013;12:545-57. [Crossref] [PubMed]
- El-Baz A, Pareek G, Suri JS. Prostate Cancer Imaging: An Engineering and Clinical Perspective. Boca Raton (FL): CRC Press, 2018.
- Acharya UR, Faust O, Sree SV, et al. An accurate and generalized approach to plaque characterization in 346 carotid ultrasound scans. IEEE Trans Instrum Meas 2012;61:1045-53. [Crossref]
- Molinari F, Krishnamurthi G, Acharya UR, et al. Hypothesis validation of far-wall brightness in carotid-artery ultrasound for feature-based IMT measurement using a combination of level-set segmentation and registration. IEEE Trans Instrum Meas 2012;61:1054-63. [Crossref]
- Acharya UR, Sree SV, Krishnan MMR, et al. Atherosclerotic risk stratification strategy for carotid arteries using texture-based features. Ultrasound Med Biol 2012;38:899-915. [Crossref] [PubMed]
- Acharya UR, Mookiah MRK, Sree SV, et al. Atherosclerotic plaque tissue characterization in 2D ultrasound longitudinal carotid scans for automated classification: a paradigm for stroke risk assessment. Med Biol Eng Comput 2013;51:513-23. [Crossref] [PubMed]
- Acharya UR, Krishnan MMR, Sree SV, et al. Plaque tissue characterization and classification in ultrasound carotid scans: A paradigm for vascular feature amalgamation. IEEE Trans Instrum Meas 2013;62:392-400. [Crossref]
- Biswas M, Kuppili V, Edla DR, et al. Symtosis: A Liver Ultrasound Tissue Characterization and Risk Stratification in Optimized Deep Learning Paradigm. Comput Methods Programs Biomed 2018;155:165-77. [Crossref] [PubMed]
- Kuppili V, Biswas M, Sreekumar A, et al. Extreme Learning Machine Framework for Risk Stratification of Fatty Liver Disease Using Ultrasound Tissue Characterization. J Med Syst 2017;41:152. [Crossref] [PubMed]
- Kakadiaris IA, Vrigkas M, Yen AA, et al. Machine Learning Outperforms ACC/AHA CVD Risk Calculator in MESA. J Am Heart Assoc 2018;7:e009476. [Crossref] [PubMed]
- Ambale-Venkatesh B, Wu CO, Liu K, et al. Cardiovascular event prediction by machine learning: the Multi-Ethnic Study of Atherosclerosis. Circ Res 2017;121:1092-101. [Crossref] [PubMed]
- Unnikrishnan P, Kumar DK, Poosapadi Arjunan S, et al. Development of health parameter model for risk prediction of CVD using SVM. Comput Math Methods Med 2016;2016:3016245.
- Zarkogianni K, Athanasiou M, Thanopoulou AC, et al. Comparison of Machine Learning Approaches Toward Assessing the Risk of Developing Cardiovascular Disease as a Long-Term Diabetes Complication. IEEE J Biomed Health Inform 2018;22:1637-47. [Crossref] [PubMed]
- Laine A, Sanches JM, Suri JS. Ultrasound Imaging: Advances and Applications. New York: Springer, 2012.
- Khanna NN, Jamthikar AD, Gupta D, et al. Performance evaluation of 10-year ultrasound image-based stroke/cardiovascular (CV) risk calculator by comparing against ten conventional CV risk calculators: A diabetic study. Comput Biol Med 2019;105:125-43. [Crossref] [PubMed]
- Khanna NN, Jamthikar AD, Gupta D, et al. Effect of carotid image-based phenotypes on cardiovascular risk calculator: AECRS1. 0. Med Biol Eng Comput 2019;57:1553-66. [Crossref] [PubMed]
- Suri JS, Turk M, Jamthikar AD, et al. Performance evaluation of AECRS1. 0 using stroke risk calculators. Eur J Neurol 2019;26:280-1.
- Cuadrado-Godia E, Srivastava SK, Saba L, et al. Geometric Total Plaque Area Is an Equally Powerful Phenotype Compared With Carotid Intima-Media Thickness for Stroke Risk Assessment: A Deep Learning Approach. Available online: https://journals.sagepub.com/doi/abs/10.1177/1544316718806421?journalCode=jvua
- Kotsis V, Jamthikar AD, Araki T, et al. Echolucency-based phenotype in carotid atherosclerosis disease for risk stratification of diabetes patients. Diabetes Res Clin Pract 2018;143:322-31. [Crossref] [PubMed]
- Khanna NN, Jamthikar AD, Araki T, et al. Nonlinear model for the carotid artery disease 10-year risk prediction by fusing conventional cardiovascular factors to carotid ultrasound image phenotypes: A Japanese diabetes cohort study. Echocardiography 2019;36:345-61. [Crossref] [PubMed]
- Cuadrado-Godia E, Jamthikar AD, Gupta D, et al. Ranking of stroke and cardiovascular risk factors for an optimal risk calculator design: Logistic regression approach. Comput Biol Med 2019;108:182-95. [Crossref] [PubMed]
- Cuadrado-Godia E, Maniruzzaman M, Araki T, et al. Morphologic TPA (mTPA) and composite risk score for moderate carotid atherosclerotic plaque is strongly associated with HbA1c in diabetes cohort. Comput Biol Med 2018;101:128-45. [Crossref] [PubMed]
- Stein JH, Korcarz CE, Hurst RT, et al. Use of carotid ultrasound to identify subclinical vascular disease and evaluate cardiovascular disease risk: a consensus statement from the American Society of Echocardiography Carotid Intima-Media Thickness Task Force endorsed by the Society for Vascular Medicine. J Am Soc Echocardiogr 2008;21:93-111. [Crossref] [PubMed]
- Molinari F, Pattichis CS, Zeng G, et al. Completely automated multiresolution edge snapper—a new technique for an accurate carotid ultrasound IMT measurement: clinical validation and benchmarking on a multi-institutional database. IEEE Trans Image Process 2012;21:1211-22. [Crossref] [PubMed]
- Saba L, Mallarini G, Sanfilippo R, et al. Intima Media Thickness Variability (IMTV) and its association with cerebrovascular events: a novel marker of carotid therosclerosis? Cardiovasc Diagn Ther 2012;2:10. [PubMed]
- Saba L, Meiburger KM, Molinari F, et al. Carotid IMT variability (IMTV) and its validation in symptomatic versus asymptomatic Italian population: can this be a useful index for studying symptomaticity? Echocardiography 2012;29:1111-9. [Crossref] [PubMed]
- Molinari F, Zeng G, Suri JS. A state of the art review on intima–media thickness (IMT) measurement and wall segmentation techniques for carotid ultrasound. Comput Methods Programs Biomed 2010;100:201-21. [Crossref] [PubMed]
- Molinari F, Zeng G, Suri JS. Intima-media thickness: setting a standard for a completely automated method of ultrasound measurement. IEEE Trans Ultrason Ferroelectr Freq Control 2010;57:1112-24. [Crossref] [PubMed]
- Molinari F, Meiburger KM, Saba L, et al. Ultrasound IMT measurement on a multi-ethnic and multi-institutional database: our review and experience using four fully automated and one semi-automated methods. Comput Methods Programs Biomed 2012;108:946-60. [Crossref] [PubMed]
- Molinari F, Meiburger KM, Zeng G, et al. Automated carotid IMT measurement and its validation in low contrast ultrasound database of 885 patient Indian population epidemiological study: results of AtheroEdge™ Software. Int Angiol 2012;31:42-53. [PubMed]
- Molinari F, Meiburger KM, Saba L, et al. Automated Carotid IMT Measurement and Its Validation in Low Contrast Ultrasound Database of 885 Patient Indian Population Epidemiological Study: Results of AtheroEdge® Software. Multi-Modality Atherosclerosis Imaging and Diagnosis. New York: Springer, 2014:209-19.
- Saba L, Jamthikar A, Gupta D, et al. Global perspective on carotid intima-media thickness and plaque: should the current measurement guidelines be revisited? Int Angiol 2019;38:451-65. [PubMed]
- Saba L, Molinari F, Meiburger K, et al. What is the correct distance measurement metric when measuring carotid ultrasound intima-media thickness automatically? Int Angiol 2012;31:483-9. [PubMed]
- Saba L, Banchhor SK, Suri HS, et al. Accurate cloud-based smart IMT measurement, its validation and stroke risk stratification in carotid ultrasound: A web-based point-of-care tool for multicenter clinical trial. Comput Biol Med 2016;75:217-34. [Crossref] [PubMed]
- Korshunov VA, Schwartz SM, Berk BC. Vascular remodeling: hemodynamic and biochemical mechanisms underlying Glagov’s phenomenon. Arterioscler Thromb Vasc Biol 2007;27:1722-8. [Crossref] [PubMed]
- Touboul PJ, Hennerici M, Meairs S, et al. Mannheim carotid intima-media thickness consensus (2004-2006). An update on behalf of the Advisory Board of the 3rd and 4th Watching the Risk Symposium, 13th and 15th European Stroke Conferences, Mannheim, Germany, 2004, and Brussels, Belgium, 2006. Cerebrovasc Dis 2007;23:75-80. [Crossref] [PubMed]
- Touboul PJ, Hennerici M, Meairs S, et al. Mannheim carotid intima-media thickness and plaque consensus (2004-2006-2011). An update on behalf of the advisory board of the 3rd, 4th and 5th watching the risk symposia, at the 13th, 15th and 20th European Stroke Conferences, Mannheim, Germany, 2004, Brussels, Belgium, 2006, and Hamburg, Germany, 2011. Cerebrovasc Dis 2012;34:290-6. [Crossref] [PubMed]
- Spence JD, Eliasziw M, DiCicco M, et al. Carotid plaque area: a tool for targeting and evaluating vascular preventive therapy. Stroke 2002;33:2916-22. [Crossref] [PubMed]
- Spence JD, Solo K. Resistant atherosclerosis: The need for monitoring of plaque burden. Stroke 2017;48:1624-9. [Crossref] [PubMed]
- Rundek T, Gardener H, Della-Morte D, et al. The relationship between carotid intima-media thickness and carotid plaque in the Northern Manhattan Study. Atherosclerosis 2015;241:364-70. [Crossref] [PubMed]
- Jamthikar A, Gupta D, Khanna NN, et al. A low-cost machine learning-based cardiovascular/stroke risk assessment system: integration of conventional factors with image phenotypes. Cardiovasc Diagn Ther 2019;9:420-30. [Crossref] [PubMed]
- D’agostino RB, Vasan RS, Pencina MJ, et al. General cardiovascular risk profile for use in primary care: the Framingham Heart Study. Circulation 2008;117:743-53. [Crossref] [PubMed]
- Stevens RJ, Kothari V, Adler AI, et al. The UKPDS risk engine: a model for the risk of coronary heart disease in Type II diabetes (UKPDS 56). Clin Sci (Lond) 2001;101:671-9. [Crossref] [PubMed]
- Kothari V, Stevens RJ, Adler AI, et al. UKPDS 60: risk of stroke in type 2 diabetes estimated by the UK Prospective Diabetes Study risk engine. Stroke 2002;33:1776-81. [Crossref] [PubMed]
- Ridker PM, Buring JE, Rifai N, et al. Development and validation of improved algorithms for the assessment of global cardiovascular risk in women: the Reynolds Risk Score. JAMA 2007;297:611-9. [Crossref] [PubMed]
- NIPPON DATA80 Research Group. Risk assessment chart for death from cardiovascular disease based on a 19-year follow-up study of a Japanese representative population. Circ J 2006;70:1249-55. [Crossref] [PubMed]
- Conroy RM. Estimation of ten-year risk of fatal cardiovascular disease in Europe: the SCORE project. Eur Heart J 2003;24:987-1003. [Crossref] [PubMed]
- Hippisley-Cox J, Coupland C, Brindle P. Development and validation of QRISK3 risk prediction algorithms to estimate future risk of cardiovascular disease: prospective cohort study. BMJ 2017;357:j2099. [Crossref] [PubMed]
- Organization WH. WHO/ISH cardiovascular risk prediction charts. Prevention of cardiovascular disease: guideline for assessment and management of cardiovascular risk. Geneva: WHO, 2007.
- Mendis S, Lindholm LH, Mancia G, et al. World Health Organization (WHO) and International Society of Hypertension (ISH) risk prediction charts: assessment of cardiovascular risk for prevention and control of cardiovascular disease in low and middle-income countries. J Hypertens 2007;25:1578-82. [Crossref] [PubMed]
- Assmann G, Cullen P, Schulte H. Simple scoring scheme for calculating the risk of acute coronary events based on the 10-year follow-up of the prospective cardiovascular Münster (PROCAM) study. Circulation 2002;105:310-5. [Crossref] [PubMed]
- D'agostino RB, Wolf PA, Belanger AJ, et al. Stroke risk profile: adjustment for antihypertensive medication. The Framingham Study. Stroke 1994;25:40-3. [Crossref] [PubMed]
- Nobel L, Mayo NE, Hanley J, et al. MyRisk_stroke calculator: a personalized stroke risk assessment tool for the general population. J Clin Neurol 2014;10:1-9. [Crossref] [PubMed]
- Go AS, Chertow GM, Fan D, et al. Chronic kidney disease and the risks of death, cardiovascular events, and hospitalization. N Engl J Med 2004;351:1296-305. [Crossref] [PubMed]
- Lee M, Saver JL, Chang K-H, et al. Low glomerular filtration rate and risk of stroke: meta-analysis. BMJ 2010;341:c4249. [Crossref] [PubMed]
- Maniruzzaman M, Rahman MJ, Al-MehediHasan M, et al. Accurate diabetes risk stratification using machine learning: role of missing value and outliers. J Med Syst 2018;42:92. [Crossref] [PubMed]
- Araki T, Jain PK, Suri HS, et al. Stroke risk stratification and its validation using ultrasonic Echolucent Carotid Wall plaque morphology: a machine learning paradigm. Comput Biol Med 2017;80:77-96. [Crossref] [PubMed]
- Saba L, Jain PK, Suri HS, et al. Plaque Tissue Morphology-Based Stroke Risk Stratification Using Carotid Ultrasound: A Polling-Based PCA Learning Paradigm. J Med Syst 2017;41:98. [Crossref] [PubMed]
- Saba L, Biswas M, Suri HS, et al. Ultrasound-based carotid stenosis measurement and risk stratification in diabetic cohort: a deep learning paradigm. Cardiovasc Diagn Ther 2019;9:439-61. [Crossref] [PubMed]
- Maniruzzaman M, Rahman MJ, Ahammed B, et al. Statistical characterization and classification of colon microarray gene expression data using multiple machine learning paradigms. Comput Methods Programs Biomed 2019;176:173-93. [Crossref] [PubMed]
- Biswas M, Kuppili V, Saba L, et al. Deep learning fully convolution network for lumen characterization in diabetic patients using carotid ultrasound: a tool for stroke risk. Med Biol Eng Comput 2019;57:543-64. [Crossref] [PubMed]
- Association AD. Standards of medical care in diabetes—2019 abridged for primary care providers. Clin Diabetes 2019;37:11-34. [Crossref] [PubMed]
- Whelton PK, Carey RM, Aronow WS, et al. 2017 ACC/AHA/AAPA/ABC/ACPM/AGS/APhA/ASH/ASPC/NMA/PCNA guideline for the prevention, detection, evaluation, and management of high blood pressure in adults: a report of the American College of Cardiology/American Heart Association Task Force on Clinical Practice Guidelines. J Am Coll Cardiol 2018;71:e127-e248. [Crossref] [PubMed]
- National Kidney Foundation. KDOQI Clinical Practice Guideline for Diabetes and CKD: 2012 Update. Am J Kidney Dis 2012;60:850-86. [Crossref] [PubMed]
- Conroy R, Pyörälä K. Estimation of ten-year risk of fatal cardiovascular disease in Europe: the SCORE project. Eur Heart J 2003;24:987-1003. [Crossref] [PubMed]
- Quesada JA, Lopez-Pineda A, Gil-Guillén VF, et al. Machine learning to predict cardiovascular risk. Int J Clin Pract 2019;73:e13389. [Crossref] [PubMed]
- Narain R, Saxena S, Goyal AK. Cardiovascular risk prediction: a comparative study of Framingham and quantum neural network based approach. Patient Prefer Adherence 2016;10:1259. [Crossref] [PubMed]
- Han D, Kolli KK, Gransar H, et al. Machine learning based risk prediction model for asymptomatic individuals who underwent coronary artery calcium score: Comparison with traditional risk prediction approaches. J Cardiovasc Comput Tomogr 2020;14:168-76. [Crossref] [PubMed]
- Revkin JH, Shear CL, Pouleur HG, et al. Biomarkers in the prevention and treatment of atherosclerosis: need, validation, and future. Pharmacol Rev 2007;59:40-53. [Crossref] [PubMed]
- Bots ML, Evans GW, Tegeler CH, et al. Carotid intima-media thickness measurements: relations with atherosclerosis, risk of cardiovascular disease and application in randomized controlled trials. Chin Med J (Engl) 2016;129:215. [Crossref] [PubMed]
- Thompson JB, Blaha M, Resar JR, et al. Strategies to reverse atherosclerosis: an imaging perspective. Curr Treat Options Cardiovasc Med 2008;10:283-93. [Crossref] [PubMed]
- Boissel JP, Collet JP, Moleur P, et al. Surrogate endpoints: a basis for a rational approach. Eur J Clin Pharmacol 1992;43:235-44. [Crossref] [PubMed]
- Administration UFaD. Table of Surrogate Endpoints That Were the Basis of Drug Approval or Licensure. 2019. . Accessed 28 November 2019.https://www.fda.gov/drugs/development-resources/table-surrogate-endpoints-were-basis-drug-approval-or-licensure
- Mancini GJ. Dahlöf Br, Diez J. Surrogate markers for cardiovascular disease: structural markers. Circulation 2004;109:IV-22-IV-30. [Crossref]
- Garg N, Muduli SK, Kapoor A, et al. Comparison of different cardiovascular risk score calculators for cardiovascular risk prediction and guideline recommended statin uses. Indian Heart Journal 2017;69:458-63. [Crossref] [PubMed]
- Anderson TJ, Grégoire J, Hegele RA, et al. 2012 update of the Canadian Cardiovascular Society guidelines for the diagnosis and treatment of dyslipidemia for the prevention of cardiovascular disease in the adult. Can J Cardiol 2013;29:151-67. [Crossref] [PubMed]
- Anderson TJ, Grégoire J, Pearson GJ, et al. 2016 Canadian Cardiovascular Society Guidelines for the Management of Dyslipidemia for the Prevention of Cardiovascular Disease in the Adult. Can J Cardiol 2016;32:1263-82. [Crossref] [PubMed]
- Jamthikar AD, Gupta D, Khanna NN, et al. A Special Report on Changing Trends in Preventive Stroke/Cardiovascular Risk Assessment via B-Mode Ultrasonography. Curr Atheroscler Rep 2019;21:25. [Crossref] [PubMed]
- Berns JS, Glickman JD, Golper TA, et al. Management of hyperglycemia in patients with type 2 diabetes and pre-dialysis chronic kidney disease or end-stage renal disease. Golper T, Nathan D (ed). Waltham, MA: UpToDate, 2018.
- Hahr AJ, Molitch ME. Management of diabetes mellitus in patients with chronic kidney disease. Clin Diabetes Endocrinol 2015;1:2. [Crossref] [PubMed]
- Kalaitzidis RG, Elisaf MS. Treatment of Hypertension in Chronic Kidney Disease. Curr Hypertens Rep 2018;20:64. [Crossref] [PubMed]
- Pugh D, Gallacher PJ, Dhaun N. Management of Hypertension in Chronic Kidney Disease. Drugs 2019;79:365-79. [Crossref] [PubMed]
- Eknoyan G, Lameire N, Eckardt K, et al. KDIGO 2012 clinical practice guideline for the evaluation and management of chronic kidney disease. Kidney Int 2013;3:5-14. [PubMed]
- Kovell L. Lipid Management Guidelines for Adults with Chronic Kidney Disease. American College of Cardiology. 2016. Available online: https://www.acc.org/latest-in-cardiology/articles/2016/05/31/13/00/lipid-management-guidelines-for-adults-with-chronic-kidney-disease
- Tannock L. Dyslipidemia in chronic kidney disease. South Dartmouth (MA): MDText.com, Inc.: 2000-2018 Jan 22.
- Major RW, Cheung CK, Gray LJ, et al. Statins and cardiovascular primary prevention in CKD: a meta-analysis. Clin J Am Soc Nephrol 2015;10:732-9. [Crossref] [PubMed]
- Rafnsson V, Bengtsson C. Erythrocyte sedimentation rate and cardiovascular disease: Results from a population study of women in Göteborg, Sweden. Atherosclerosis 1982;42:97-107. [Crossref] [PubMed]
- Erikssen G, Liestøl K, Bjørnholt J, et al. Erythrocyte sedimentation rate: a possible marker of atherosclerosis and a strong predictor of coronary heart disease mortality. Eur Heart J 2000;21:1614-20. [Crossref] [PubMed]
- Andresdottir MB, Sigfusson N, Sigvaldason H, et al. Erythrocyte Sedimentation Rate, an Independent Predictor of Coronary Heart Disease in Men and Women: The Reykjavik Study. Am J Epidemiol 2003;158:844-51. [Crossref] [PubMed]
- Harrison M. Erythrocyte sedimentation rate and C-reactive protein. Aust Prescr 2015;38:93-4. [Crossref] [PubMed]
- Winbeck K, Kukla C, Poppert H, et al. Elevated C-reactive protein is associated with an increased intima to media thickness of the common carotid artery. Cerebrovasc Dis 2002;13:57-63. [Crossref] [PubMed]
- Eltoft A, Arntzen KA, Wilsgaard T, et al. Joint Effect of Carotid Plaque and C-Reactive Protein on First-Ever Ischemic Stroke and Myocardial Infarction? J Am Heart Assoc 2018;7:e008951. [Crossref] [PubMed]
- Saba L, Tiwari A, Biswas M, et al. Wilson's disease: A new perspective review on its genetics, diagnosis and treatment. Front Biosci (Elite Ed) 2019;11:166-85. [Crossref] [PubMed]
- Molinari F, Zeng G, Suri JS. Inter-greedy technique for fusion of different segmentation strategies leading to high-performance carotid IMT measurement in ultrasound images. Atherosclerosis Disease Management. New York: Springer, 2011:253-79.
- Qualtrics. Determining Sample Size: How to Ensure You Get the Correct Sample Size. 2019. Available online: https://www.qualtrics.com/experience-management/research/determine-sample-size/