Dual-energy CT and coronary imaging
Introduction
Despite recent advancements in prevention and therapy, heart disease is still the leading cause of death in the United States (1). Coronary artery disease (CAD) alone was responsible for the death of 365,914 individuals in 2017 (2). Thus, it is imperative that current methods and strategies for diagnosing CAD continue to evolve. Noninvasive coronary imaging with computed tomography gained significant importance for diagnosing patients with CAD in the last decades. At the secondary prevention level, CT coronary artery calcium scoring (CTCACS) can provide guidance for statin therapy in certain individuals with 10-year atherosclerotic cardiovascular disease risk between 7.5% and 20% (3). In addition, current guidelines of the National Institute for Health and Care Excellence in the UK {NICE, clinical guideline [CG95], updated in 2016} (4) established CT coronary angiography (CTCA) as the first-line diagnostic tool in patients with typical or atypical anginal chest pain, or in those with non-anginal pain and abnormal 12-lead resting ECG. CTCA can also provide a unique opportunity to noninvasively evaluate features of coronary atherosclerotic plaques that were found to predispose to acute coronary events (5-9). More recently, CTCA-derived fractional flow reserve and CTCA myocardial perfusion have proven value in assessing the hemodynamic significance of coronary atherosclerotic plaques (10-13).
Despite the introduction of dual-energy CT (DECT) scanners more than a decade ago (14) and the many potential applications already described in cardiovascular imaging (15), there has been some latency in the full and widespread clinical incorporation of this technology, especially in practice guidelines. Several limiting factors could explain this phenomenon, including decreased availability of DECT scanners in comparison with single-energy CT (SECT) scanners, lack of extensive clinical validation, and paucity of outcome studies suggesting superiority over standard imaging methods. Notwithstanding, studies have shown the potential of DECT to improve many aspects of CCTA, such as optimizing image quality and luminal assessment of the coronary arteries, decreasing the amount of iodinated contrast load, eliminating the need for a non-enhanced phase for CTCACS or aortic valve calcium scoring purposes, characterizing atherosclerotic plaque, and evaluating myocardial ischemia. The fact that some scanners allow one to fully benefit from DECT data without the need for changing scanning parameters (e.g., dual-layer DECT) has allowed us to test some of these applications in clinical practice without departing from our standard of care. Photon-counting CT scanners are also emerging in this dynamic scenario, adding other potential applications, such as multi-material contrast agent imaging and high-resolution stent and coronary imaging. The goal of this paper is to review the literature about the applications of DECT for coronary imaging. After a brief introduction to the technical aspects, we will present the rationale, scientific background, and current status of knowledge. Last, we will provide some future perspectives with the early development of photon-counting CT.
Technical aspects of DECT
Different techniques have been developed by CT equipment manufacturers for obtaining DECT data. Several publications previously described the principles of image acquisition, reconstruction, and post processing; for example, McCollough et al. (16) provides an excellent introduction to the topic. Table 1 summarizes the main approaches, standard nomenclature (17), techniques, and currently commercially available clinical DECT scanners.
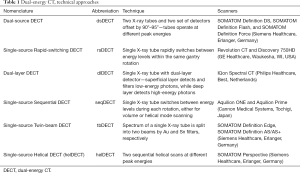
Full table
Material decomposition is the method by which DECT is capable of extracting material density maps from the imaging object. Most commonly, a set of two or three materials with known theoretical attenuation profiles at low and high X-ray energy levels are selected a priori to derive specific material density images, either on the sinogram (e.g., as in rsDECT) or image (e.g., as in dsDECT) space. These density measurements can be displayed as color overlays on top of standard CT, or serve as basis for quantitative imaging on advanced post processing (14,16,18-20). For example, phantom measurements of iodine density obtained in first and second generation dsDECT scanners are found to be accurate against nominal values (21). Other algorithms have also been proposed for decomposition on the image space for three or more materials on DECT, which are based on additional model constraints, such as volume or mass conservation and iterative best model fitting (22,23). Physically, K-edge properties of contrast materials, such as iodine (K-edge energy of 33.2 keV), facilitate their separation from molecules constituted of low-Z-number elements (e.g., water and proteins) (24). Therefore, iodine is a commonly selected material for DECT, and has been applied as surrogate for tissue perfusion given that its distribution matches that of first passage of blood after contrast injection (25-27). While material decomposition allows for iodine-only imaging, it also provides means to generate virtual non-contrast (VNC) images by the same procedure, synthetically subtracting the iodine attenuation component from the CT attenuation number (16). As a result, VNC imaging could obviate the need for pre-contrast acquisition, thus reducing radiation dose. Similarly, specific decomposition of calcium has led to removal of bones and calcified component of atherosclerotic plaques in CT angiography (16).
Another post-processing capability of DECT is generating synthetic images at specific monoenergetic levels, also known as virtual monoenergetic imaging (VMI), from two spectral X-ray projections (28). The main advantage of VMI is eliminating beam-hardening artifacts, which are inherently present in standard spectral X-ray projections. This method is theoretically advantageous when imaging structures near areas with higher X-ray attenuation, as is the case of coronary luminal assessment with stents or highly calcified plaques, and in myocardial perfusion imaging near the diaphragm.
DECT data can also be used in less common ways for coronary imaging. For instance, effective atomic number and electron density imaging (29), as well as the ratio between CT numbers measured on high- and low-energy images (DECT ratio, DER) (16), have also been proposed for tissue characterization, and will be discussed in details ahead. Table 2 summarizes theoretical applications of DECT to coronary imaging, and the following sections will review the current scientific status in each application.
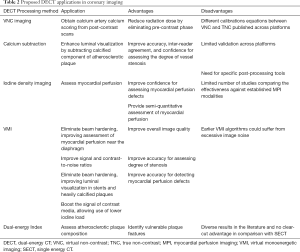
Full table
Coronary artery calcium scoring from post-contrast scans
Clinically, a pre-contrast ECG-gated cardiac CT is usually obtained before a CTCA for calcium scoring purposes. Data from the Prospective Multicenter Imaging Study for Evaluation of Chest Pain (PROMISE) trial revealed that CTCACS obtained prior to CTCA had discriminatory power for identifying patients at risk for major adverse cardiovascular events, which was comparable to that of functional testing (30). In addition, a meta-analysis focused on the prognostic value of a CTCACS of zero, in the setting of acute chest pain without ischemic ECG findings or troponin elevation, revealed a pooled negative likelihood ratio of 0.07 (31). VNC has been proposed as a method to quantify the CTCAS without the necessity of obtaining a pre-contrast phase, which could result in radiation dose savings. In a feasibility study, Yamak et al. (32) investigated if material decomposition of calcium and iodine by DECT could allow for the quantification of coronary artery calcium. Using a phantom with 29 postmortem calcified atherosclerotic plaques for calibration and in vivo data from 6 subjects undergoing both pre- and post-contrast cardiac CT in rsDECT, the authors determined cutoff points of calcium concentration in Calcium (Iodine) images corresponding to the CT-number threshold of 130 HU for plaque calcification, as defined by the Agatston method (33). In vivo measurements of coronary calcium mass obtained from post-contrast rsDECT in this small sample were highly correlated with the Agatston score obtained on true non-contrast (TNC) scans (r=0.98). Schwarz et al. (34) prospectively studied 36 subjects undergoing TNC cardiac CTCACS, followed by dsDECT CTCA. Comparisons were made between VNC images generated from CCTA and TNC. There was high correlation between coronary artery calcium volume between the two methods (r=0.942 –0.948); however, calcium volumes measured on VNC were significantly lower than those measured on TNC (Figure 1). The authors developed and tested the predictive value of VNC-derived calcium volume to predict TNC CTCACS using linear modeling with leave-one-out cross-validation. The intraclass correlation coefficient (ICC) between VNC-predicted and reference CTCACS were 0.696 (95% CI: 0.479–0.832), corresponding to an ICC of 0.909 (95% CI: 0.829–0.952) after conversion to population percentile strata using data from the Multiethnic Study of Atherosclerosis (35). In a later study, Yamada et al. (36) investigated if a VNC algorithm based on three-material decomposition in rsDECT could predict the TNC CTCACS in a cohort of 27 subjects. Although synthetic CTCACS and volumes on VNC were highly correlated with TNC values (respectively, r=0.93 and r=0.94, both P value <0.001), numbers were significantly lower on VNC. Linear models built on the log-transform of the calcium measurements predicted true CTCACS and calcium volumes with respective ICC of 0.93 (95% CI: 0.84–0.97) and 0.92 (95% CI: 0.81–0.97). All subjects with CTCACS >400 and 93% of the subjects with CTCACS >0 and ≤400 were correctly predicted by the model-adjusted VNC method. The authors conclude that a statistically significant decrease in patient dose could be achieved by means of a single rsDECT scan when compared with pre- and post-contrast conventional CT scans (respectively 4.3±0.3 mSv versus 5.4±0.7 mSv, P value <0.0001). Fuchs et al. (37) performed a similar study to Yamada et al. (36) in 52 subjects also using a rsDECT scanner; the main difference between the studies was the use of a “low-radiation-dose” rsDECT CCTA protocol by Fuchs et al., with an estimated effective dose of 1.70±0.53 mSv (versus 4.3±0.3 mSv used in Yamada et al.). Although both groups reached similar general conclusions about VNC-derived CTCACS using similar CT scanners, differences in the calibration equations derived from linear models are noted between studies. Furthermore, in a more recent study including 20 subjects, Nadjiri et al. (38) found that VNC-derived CTCACS values predicted from dlDECT had to be multiplied by a factor of 2.3 to correct for systematic biases between VNC and TNC images. Other feasibility studies also found similar results when comparing CTCACS and aortic valve calcium scoring obtained from VNC and TNC in pre-transcatheter arterial valve replacement CT scans (39) and CTCACS measurements from non-ECG-gated chest DECT (40). Therefore, although many independent feasibility studies have shown the potential value of VNC imaging for substituting TNC imaging for CTCACS purposes, further research including a larger number of subjects is still necessary to determine the specific calibration parameters for correction of systematic biases existing between the two methods.
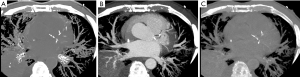
In summary, VNC has the potential to act as a substitute for TNC in calcium scoring; however, further validation is required. The Society of Advanced Body Imaging guidelines acknowledged the promising role of VNC imaging for substituting TNC CTCACS, but was cautionary about its readiness for clinical use, recommending additional multicenter and multivendor clinical trials for determining externally validated conversion algorithms and consistent interpretability of results (41). Although our group is investigating potential applications for coronary artery calcium segmentation using DECT, we do not currently use this technique for routine coronary or aortic valve calcium scoring purposes in clinical practice.
Subtracting calcium
Severely calcified atherosclerotic plaques are a known limitation of CTCA, mainly secondary to the effects of blooming and beam hardening artifact (42). By using material decomposition, DECT is capable of subtracting the calcified component of the plaque, thus improving luminal visualization. De Santis et al. (43) tested the performance of a research software designed to subtract calcifications based on a modified three-material decomposition model (calcium, soft tissue, and iodine) in a retrospective cohort of 29 subjects undergoing CTCA in a third-generation dsDECT. They found that calcium-subtracted images provided better visibility of the coronary lumen, improving inter-reader agreement and diagnostic confidence when compared with standard linear blended non-subtracted image, without compromise of image quality or contrast-to-noise ratio. Similarly, Andreini et al. (44) determined improvements of vessel evaluation and diagnostic performance for detection of ≥50% stenosis in iodine(calcium) images obtained by two-material decomposition in rsDECT. Currently, the use of calcium-subtracted DECT images for coronary assessment is experimental and needs further multi-center and multi-vendor validation. In our experience, calcium subtraction can increase diagnostic confidence for assessing coronary artery stenosis (Figure 2), but clinical translation of the technique still depends on the availability of widely validated diagnostic tools.
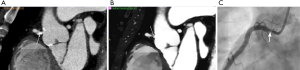
Assessing myocardial perfusion
If on one hand prospective multi-center trials using current CCTA technology confirmed high sensitivity (85–99%) and moderate to high specificity (64–90%) for diagnosing coronary stenosis >50% in subjects with suspected CAD compared to invasive coronary angiography (ICA) (45-47), on the other, recent evidence has suggested that coronary revascularization strategies should rely more on information about myocardial ischemia than degree of coronary stenosis. The Fractional Flow Reserve (FFR) versus Angiography for Multi-vessel Evaluation (FAME) study showed that choosing revascularization for CAD based on FFR as surrogate for myocardial ischemia was not only clinically (48-50), but also economically (51) superior to the therapeutic strategy based on the degree of luminal narrowing. It is known that estimation of luminal narrowing by CTCA and ICA are only weakly correlated with FFR (52).
Computed tomography FFR (CT-FFR) and dynamic myocardial CT perfusion (CTP) are techniques developed in parallel with the aim to improve the specificity of CTCA for determining functional significance (i.e., myocardial ischemia) caused by atherosclerotic plaques. CT-FFR uses computational fluid dynamics simulations based anatomical information directly available from CTCA, which shows good correlation with invasive FFR (10-12). In contrast, CTP protocols assess ischemia by determining dynamic changes in the myocardial distribution during the first pass of iodinated contrast under rest and stress (caused by a vasodilator, such as adenosine or regadenoson) (53). Rather than being competitive methods, there is emerging evidence that CT-FFR and CTP may be complementary to each other (54). Different DECT techniques, such as iodine density imaging and VMI, have been proposed to enhance the performance of myocardial CTP. DECT methods leverage this post-processing techniques for improving sensitivity and specificity for iodine detection, and elimination of beam hardening artifacts, therefore differing from the classic dynamic myocardial CTP approach by using a single post-contrast phase (instead of multiple time-resolved acquisitions).
Ruzsics et al. (55) evaluated the diagnostic performance of rest dsDECT myocardial perfusion imaging to detect ischemia using iodine density (56) as a surrogate for tissue perfusion (Figure 3). In an analysis of 30 subjects undergoing both dsDECT CTCA and single-photon emission tomography (SPECT), the authors found respective per-segment/per-patient sensitivities of 88%/100%, specificity of 89%/67%, and accuracy of 89%/89% for detecting fixed or reversible myocardial perfusion defects on SPECT. However, it is debatable if rest dsDECT myocardial perfusion has any additional diagnostic value to CTCA in subjects with low-to-intermediate risk for obstructive CAD (57), although newer evidence suggest that objective quantitative analysis of rest dsDECT myocardial perfusion maps could provide more accurate results than visual analysis (58). In subjects with acute myocardial infarction, segmental hypoperfusion detected on rest dsDECT were highly correlated with infarcted areas as defined by ICA, troponin I elevation, and ECG changes (59). In our experience, DECT has increased diagnostic confidence for diagnosing chronic and acute ischemia, especially in the inferior and inferolateral left ventricular segments, which are commonly affected by beam-hardening artifacts. We speculate that DECT could have an important role in the detection of ischemia on CCTA obtained at the emergency department for assessment of acute chest pain (Figure 4).
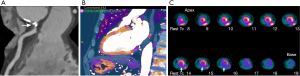
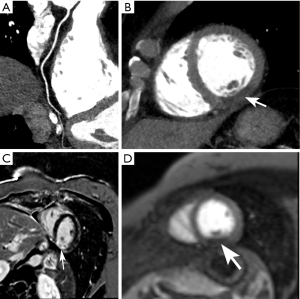
A combination of rest and stress dsDECT perfusion can increase the sensitivity for myocardial ischemia (60). Ko et al. studied 51 subjects with known CAD, undergoing stress dsDECT myocardial perfusion, cardiac magnetic resonance perfusion (CMR-P), and ICA (61). They respectively found sensitivity, specificity, and accuracy of 89%, 78%, and 82% for detecting reversible myocardial ischemia on CMR-P, and 89%, 76%, and 83% for detecting hemodynamically significant CAD on ICA. Kim et al. (62) also compared stress single-phase dsDECT myocardial perfusion against CMR-P in 50 subjects with clinically suspected CAD; sensitivity and specificity of dsDECT myocardial perfusion were 77% (95% CI: 67–87%) and 94% (95% CI: 92–95%), respectively. Multiple other studies have confirmed the incremental diagnostic value of stress dsDECT myocardial CTP, for identification of hemodynamically significant CAD, over CTCA alone (63-67).
The inherent ability of VMI to reduce beam hardening artifacts in the myocardium, especially near the diaphragm, has been investigated in a series of studies determining the potential advantage of using DECT for CTP. Experimental data using phantoms and animal models showed the technical utility of rsDECT with VMI at energy levels ≥70 keV for reducing beam hardening in myocardial perfusion imaging (68,69). VMI imaging has been shown to decrease beam hardening artifacts especially in the basal inferolateral segment (70). Carrascosa et al. (71) examined the diagnostic performance of stress CTP in patients undergoing both rsDECT with VMI and SECT, using SPECT myocardial perfusion as standard of reference. The area under the curve for detecting segmental perfusion defects was 0.90 (95% CI: 0.86–0.94) for VMI versus 0.80 (95% CI: 0.76–0.84) for SECT (P value =0.0004). Noticeably, the performance continued to be superior even when only segments known to be affected by beam-hardening artifacts were included in the analysis. Use of low-energy VMI (40–70 keV) may provide better discrimination of hypoperfusion segments on rest and stress CTP on rsDECT (72) (Figure 5). Last, VMI can also provide ways of minimizing variability in quantitative myocardial perfusion analysis (68).
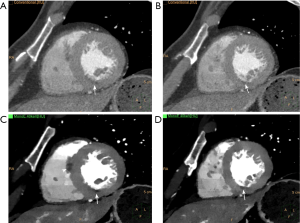
In summary, myocardial perfusion imaging on CCTA may benefit from DECT techniques such as iodine density imaging and VMI, although most of the evidence has originated from relatively small single center studies. The Society of Advanced Body Imaging guidelines support the use of rest DECT myocardial perfusion imaging in patients with high likelihood of myocardial ischemia or infarct (41). Current technique requisites and detailed imaging protocols for performing myocardial perfusion with DECT have been published elsewhere (56,73). Future studies are still needed to determine the comparative effectiveness of stress CTP across multiple centers and platforms versus established and emerging methods for detecting myocardial ischemia, such as SPECT and CT-FFR. For instance, an ongoing study have been designed to compare the diagnostic performance of dlDECT, stress CT perfusion, CT-FFR, and deep learning to increase specificity using anatomical and functional imaging (74).
Improving image quality
Several studies have addressed the advantages of DECT for improving signal-to-noise and contrast-to-noise ratios (SNR and CNR, respectively) in CTCA. For example, in an early ex vivo feasibility study, Boll et al. (75) assessed sequential scanning of coronary artery specimens at 90 and 140 kVp in a 16-slice MDCT. The authors used a weighted logarithmic subtraction between the high and low energy scans (“K-edge” imaging), finding improved CNR between the coronary lumen, the vessel wall, and fibromuscular component of the coronary plaque. In another study, Scheske et al. found that VMI at 60–80 keV could significantly improve SNR and CNR in the myocardium and coronary arteries when compared to SECT (76). In a different sample of 20 subjects undergoing rsDECT CCTA, VMI reconstructions from 65 and 75 keV combined with 40%- to 80%-blending of adaptive iterative reconstruction and filtered back projection improved subjective image quality, CNR and SNR in comparison with conventional polychromatic images, while keeping low radiation dose (1.8±0.7 mSv) (23452997). In another study, Ohta et al. (77) determined that VMI at 70 keV yielded the best CNR between coronary plaque elements and lumen when compared to other VMI levels.
Improvements in image quality may result in better diagnostic performance by providing more precise delineation of atherosclerotic plaque and adjacent arterial lumen. For instance, elimination of blooming and beam hardening artifacts were shown to improve the size estimates of calcified plaques in a phantom study using dlDECT (78) (Figure 6). Alike, Symons et al. (79) conducted a study to determine the effect of various DECT reconstruction methods on image quality for assessing coronary atherosclerotic plaque. The authors prospectively enrolled 51 subjects undergoing CTCA in a single center using a 3rd generation dsDECT scanner, comparing SNR, plaque CNR, and quantitative plaque assessment between conventional linearly-blended reconstructions, conventional VMI, and noise-optimized VMI. Noise-optimized VMI at 40 keV had the highest signal-to-noise ratio, while 40-70 keV noise-optimized VMI had better coronary plaque CNR when compared with conventional linearly-blended images. Another study carried out in a single center using a 3rd generation dsDECT also found improved subjective and objective measurements of image quality in CCTA reconstructed at 40 keV using noise-optimized VMI in comparison with VMI series at 70 keV and conventional linearly blended images (80). However, plaque volume quantified on 40 keV noise-optimized VMI images can be significantly higher than those measured on conventional images, which could have impact on serial comparisons using different techniques (79). In another study, Stehli et al. (81) found that different VMI levels can provide better accuracy for diagnosing stenoses depending on the plaque composition. For example, their results showed that 90 keV may provide the best luminal evaluation for calcified and mixed plaques, while non-calcified plaques could benefit from evaluation at 140 keV (Figure 7).
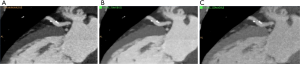
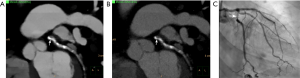
In summary, there is robust evidence showing that VMI can be used to improve image quality, plaque and luminal visualization, potentially resulting in improved diagnostic confidence and inter-reader agreement. As a result, VMI has been routinely incorporated in our clinical practice. Our pipeline consists of routine reconstruction of 40 keV images at the scanner, which are stored in the Picture Archiving and Communication System in addition to conventional (i.e., linearly blended) reconstructions and source spectral base images (dlDECT) or high- and low-kVp images (dsDECT). When necessary, source images can be retrieved for further post-processing on dedicated workstations, generating VMI at different energy levels, VNC, or material density maps.
Assessing stent patency
Generally, assessment for stent restenosis is challenging due to beam hardening artifacts caused by the metallic composition of the struts. As previously discussed, VMI has been successfully used to decrease beam hardening artifacts. However, there is conflicting scientific data on the potential advantages of DECT over SECT for stent imaging (82,83). VMI does not appear to provide significant improvements for stent imaging as compared to SECT; on the contrary, low-energy VMI (i.e., 50 keV) should be actually avoided as luminal diameters may be underestimated by up to 90% (84). At least one phantom study using a 3rd generation dsDECT and noise-optimized VMI showed improved luminal visibility in stents ≤3 mm when compared to standard VMI and SECT, particularly with the use of 130 keV noise-optimized VMI (85) (Figure 8).
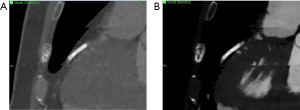
In summary, although cited as appropriate for limited use with stents by the Society of Advanced Body Imaging guidelines (41), VMI does not appear to provide a clear-cut benefit for stent imaging. The use of DECT for improving stent imaging may be considered mostly experimental at this time, and stent-related factors (e.g., diameter, metallic strut composition) may significantly impact the results. Therefore, our group does not routinely use VMI techniques for improving stent imaging. As discussed next, improved spatial resolution obtained with photon-counting CT scanners could be more advantageous for this task.
Reducing iodinated contrast volume
Despite recent debate about the actual role of iodinated contrast agents in contrast-associated kidney injury (86), cautious administration of these agents and supplemental hydration is still recommended for subjects with acute kidney injury or estimated glomerular filtration rate <30 mL/min/1.73 m2 (87). Therefore, in such population, one must limit the iodinated contrast volume to as low as reasonably achievable. VMI at levels near the K-edge energy of iodine (e.g., 40 keV) have been used to boost the signal of contrast agents, allowing for reductions in the iodine load (Figure 9). For example, in a single-center, double-blinded, prospective study, Raju et al. (88) enrolled 53 subjects to SECT CTCA using standard contrast agent dose (80 mL of iodixanol 320 mgI/mL) and 49 subjects to low-iodine dose rsDECT CCTA (35 mL of iodixanol 320 mgI/mL). CNR and adjusted SNR were not different between protocols. Although subjective image quality on the standard contrast agent dose group was better than the low-iodine dose in the opinion of one of the two readers, agreement in diagnostic interpretability were comparable between both groups (96% versus 91%, respectively). In another study, 36 patients were initially scanned using a 50% reduction in the iodine dose using rsDECT, followed by a full iodine dose SECT. Although SNR and CNR were lower on DECT scans, detection of stenosis >50% was not compromised (89). In a head-to-head comparison of rsDECT with half iodine dose and SECT with standard iodine dose sequentially performed in the same subjects, there were no differences in the detection of significant coronary stenosis (89).
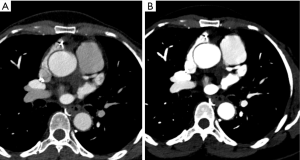
In summary, despite the lack of current recommendations by the Society of Advanced Body Tomography, it is reasonable to propose the use of reduced iodine dose protocols in high-risk subjects (those in acute kidney injury or with estimated glomerular filtration rate <30 mL/min/1.73 m2) undergoing CTCA if a DECT scanner and low-energy VMI are available, until new evidence finally settles the controversy about the existence of any link between the contemporary generation of iodinated contrast agents and kidney injury. In our practice, we have used low-keV VMI as a “rescue” action for improving image quality in studies with limited opacification of the coronary artery lumen.
Assessing coronary plaque composition
Different histopathologic components of coronary plaques have distinct prognostic implications and have been used as targets for invasive and noninvasive imaging (90). There are many features identified on conventional CTCA that have been associated with instability and risk for acute coronary syndrome, such as positive remodeling, low-density core, spotty calcification, and napkin ring sign (6-9,91-95). More recently, studies have also tried to assess if DECT could enhance plaque characterization in comparison to SECT, based on the observation that soft tissue discrimination is improved with the former technique (96).
To determine the potential role of DECT in differentiating coronary atherosclerotic components, Barreto et al. (97) conducted an ex vivo study of seven human coronary arteries obtained from autopsies. A total of 14 atherosclerotic lesions were examined from the specimens using helDECT. Densely calcified atherosclerotic plaques and fibrocalcific plaques, as confirmed by histologic study, had significantly different attenuation values between non-contrast images obtained at 80 and 140 kVp, an observation that was absent in fibrous or lipid-rich plaques. A dual-energy index (DEI) devised to capture differences in attenuation between the two energy levels could discriminate densely calcified plaques from other subtypes, but overlap was noted between fibrocalcific, fibrous, and lipid-rich plaques. In the same study, plaques with a fibrous component (i.e., fibrocalcific and fibrous plaques) showed significant differences from pre- to post-contrast images at both energy levels. The small sample size was a limitation in this preliminary study. Henzler et al. (98) also found that coronary atherosclerotic plaque quantification based on CT attenuation numbers using weighted reconstructions (70% or 140 kV and 30% of 100 kV) was at least comparable to standard SECT CTCA, using histopathology as standard of reference. Instead of looking at the weighted CT numbers, Tanami et al. (2010) (99) studied the CT numbers measured in coronary plaques at four energy levels (i.e., 80 kVp, 100 kVp, 120 kVp, and 140 kVp) using helDECT. In 93 coronary plaques extracted from postmortem specimens of 15 subjects, the authors found that, despite large overlap between CT numbers of lipid-rich and fibrous plaques, the ratio between measurements made on 80 kVp over those made at 140 kVp images were capable of discriminating those two types of plaques with area under the receiver operating curve of 0.952 (standard error: 0.029), which significantly outperformed single measurement obtained on 120 kVp. In a later study, Obaid et al. (100) assessed the discriminatory performance of dsDECT CTCA in identifying different atherosclerotic plaque components as compared with virtual histology extracted from intravascular ultrasound. The analysis of 138 plaque samples in 20 subjects showed that the DEI initially proposed by Barreto et al. could differentiate necrotic core from fibrous and calcific plaque components (P value <0.0001). In an ex vivo validation of the findings in a sample of seven coronary arteries, the DEI showed specificity of 98% (95% CI: 89–100%), similar to that using a cutoff of <37 HU for necrotic core on single-energy CT; however, the sensitivity using DEI tended to be significantly higher (64%, 95% CI: 44–81%) than that using the same threshold on single-energy CT (25%, 95% CI: 11–45%).
Haghighi et al. (101) studied the feasibility of using two physical parameters measured on noncalcified coronary atherosclerotic plaques using dsDECT, namely electron density (ρe) and effective atomic number (Zeff). Their method requires specific scanner calibration using phantoms with known ρe and Zeff, followed by conversion of CT numbers obtained from 100 kVp and 140 kVp images using coefficients of inversion determined by mathematical modeling. Although ρe and Zeff define specific material properties, allowing for potential differentiation between lipids and fibrous tissue in atherosclerotic plaques, its clinical effectiveness for determining plaque composition in vivo remains to be determined. In a subsequent ex vivo study, Mandal et al. (102) established the higher Zeff values in vulnerable plaques as compared to stable plaques. X-ray spectroscopy of plaques suggest that this observation is explained by the ability of a lipid core to bind to high Z elements (calcium, P, Mg), presenting decreased protein content (lower Nitrogen wt%) in comparison with versus thick fibrous plaques (higher Nitrogen wt%).
In summary, coronary plaque characterization with DECT is still an ongoing field of investigation, and further studies determining the comparative effectiveness of newer biomarkers against the classic CTCA features of vulnerability (i.e., positive remodeling, low-density core, spotty calcification, and napkin ring sign) are still necessary before translation into clinical practice. Developments in quantitative imaging and machine learning may be tools that will leverage tissue characterization capabilities of DECT. For example, Yamak et al. (103) showed that an ensemble of supervised machine learning algorithms could be used to differentiate lipid-rich from fibrous plaques in a rsDECT system. In our practice, we do not routinely used DECT data for plaque characterization, relying on classic signs for plaque vulnerability described on SECT.
Future perspectives
Multi-energy CT is at the forefront of medical imaging research, and photon-counting CT scanners hold the promise to take this exciting field to the next level (104). The main difference between photon-counting and energy-integrating scanners (i.e., those currently used in clinical practice) resides at the detector level. All X-ray photons reaching the energy integrating detectors are transduced to light photons at the scintillation layer; subsequently, these photons are integrated by light detectors that convert the information into electric signals, which are proportional to the amount of energy deposited. Differently, photon-counting detectors are usually made from silicon or a combination of cadmium, zinc, and telluride (CZT), materials with properties that allow direct detection of incident X-ray photons, which are in turn converted into electric signals. Not only do these detectors register the number of incident photons, but they can also discriminate their energies into two to eight different bins (104). There are many theoretical benefits for photon counting CT, including decrease in noise levels, which in turn allows for better image quality or decreased radiation doses and elimination of beam hardening artifacts. Thinner detectors also allow for high-resolution images, with preliminary data showing promising results in stent imaging (105). Increased precision in measuring different energy levels leads to decreased noise in material-specific and weighted images (106). Photon counting scanners also facilitate multi-material decomposition (107). In addition, multiple parameters provide more inputs than conventional dual-energy CT, which can be used for more precise modeling of material-specific images, especially when decomposing images for K-edge materials (108), potentially improving the capabilities of multi-energy CT to image different combinations of contrast agents, including nanoparticles agents targeting atherosclerosis (109). Although photon-counting CT holds a great promise for the future, its access is currently limited to a few centers, and many technical challenges still need to be overcome prior to wide implementation (104).
Conclusions
Several applications of DECT have been developed and tested in the last decade for coronary imaging, which has been proposed as a one-stop-shop modality for investigation of CAD. Improvements in image quality are the main benefit gained with DECT scanners, as consistently shown in the literature. Although there are many other potential uses, including coronary plaque characterization, myocardial perfusion imaging, and VNC calcium scoring, future studies are still needed to ensure wide external validation and existence of incremental clinical value when compared to already established diagnostic methods.
Acknowledgments
Funding: None.
Footnote
Provenance and Peer Review: This article was commissioned by the Guest Editor (Luca Saba) for the series “Advanced Imaging in the diagnosis of Cardiovascular Diseases” published in Cardiovascular Diagnosis and Therapy. The article was sent for external peer review organized by the Guest Editor and the editorial office.
Conflicts of Interest: The author has completed the ICMJE uniform disclosure form (available at http://dx.doi.org/10.21037/cdt.2020.04.04). The series “Advanced Imaging in The Diagnosis of Cardiovascular Diseases” was commissioned by the editorial office without any funding or sponsorship. The author has no other conflicts of interest to declare.
Ethical Statement: The author is accountable for all aspects of the work in ensuring that questions related to the accuracy or integrity of any part of the work are appropriately investigated and resolved.
Open Access Statement: This is an Open Access article distributed in accordance with the Creative Commons Attribution-NonCommercial-NoDerivs 4.0 International License (CC BY-NC-ND 4.0), which permits the non-commercial replication and distribution of the article with the strict proviso that no changes or edits are made and the original work is properly cited (including links to both the formal publication through the relevant DOI and the license). See: https://creativecommons.org/licenses/by-nc-nd/4.0/.
References
- Heron M. Deaths: Leading Causes for 2017. National Vital Statistics Reports. Volume 68, number 6. Published on June 24, 2019. Available online: https://www.cdc.gov/nchs/data/nvsr/nvsr68/nvsr68_06-508.pdf
- Benjamin EJ, Muntner P, Alonso A, et al. Heart Disease and Stroke Statistics-2019 Update: A Report From the American Heart Association. Circulation 2019;139:e56-528. [Crossref] [PubMed]
- Grundy SM, Stone NJ. 2018 American Heart Association/American College of Cardiology/Multisociety Guideline on the Management of Blood Cholesterol-Secondary Prevention. JAMA Cardiol 2019;4:589-91. [Crossref] [PubMed]
- National Institute for Health and Care Excellence Chest pain of recent onset: assessment and diagnosis. NICE clinical guideline No 95. London. 2010. Accessed 02/01/2020. Available online: https://www.nice.org.uk/guidance/cg95
- Otsuka K, Fukuda S, Tanaka A, et al. Napkin-ring sign on coronary CT angiography for the prediction of acute coronary syndrome. JACC Cardiovasc Imaging 2013;6:448-57. [Crossref] [PubMed]
- Motoyama S, Kondo T, Sarai M, et al. Multislice computed tomographic characteristics of coronary lesions in acute coronary syndromes. J Am Coll Cardiol 2007;50:319-26. [Crossref] [PubMed]
- Motoyama S, Sarai M, Harigaya H, et al. Computed tomographic angiography characteristics of atherosclerotic plaques subsequently resulting in acute coronary syndrome. J Am Coll Cardiol 2009;54:49-57. [Crossref] [PubMed]
- Motoyama S, Ito H, Sarai M, et al. Plaque Characterization by Coronary Computed Tomography Angiography and the Likelihood of Acute Coronary Events in Mid-Term Follow-Up. J Am Coll Cardiol 2015;66:337-46. [Crossref] [PubMed]
- Puchner SB, Liu T, Mayrhofer T, et al. High-risk plaque detected on coronary CT angiography predicts acute coronary syndromes independent of significant stenosis in acute chest pain: results from the ROMICAT-II trial. J Am Coll Cardiol 2014;64:684-92. [Crossref] [PubMed]
- Koo BK, Erglis A, Doh JH, et al. Diagnosis of ischemia-causing coronary stenoses by noninvasive fractional flow reserve computed from coronary computed tomographic angiograms. Results from the prospective multicenter DISCOVER-FLOW (Diagnosis of Ischemia-Causing Stenoses Obtained Via Noninvasive Fractional Flow Reserve) study. J Am Coll Cardiol 2011;58:1989-97. [Crossref] [PubMed]
- Min JK, Leipsic J, Pencina MJ, et al. Diagnostic accuracy of fractional flow reserve from anatomic CT angiography. JAMA 2012;308:1237-45. [Crossref] [PubMed]
- Nørgaard BL, Leipsic J, Gaur S, et al. Diagnostic performance of noninvasive fractional flow reserve derived from coronary computed tomography angiography in suspected coronary artery disease: the NXT trial (Analysis of Coronary Blood Flow Using CT Angiography: Next Steps). J Am Coll Cardiol 2014;63:1145-55. [Crossref] [PubMed]
- Cademartiri F, Seitun S, Clemente A, et al. Myocardial blood flow quantification for evaluation of coronary artery disease by computed tomography. Cardiovasc Diagn Ther 2017;7:129-50. [Crossref] [PubMed]
- Johnson TR, Krauss B, Sedlmair M, et al. Material differentiation by dual energy CT: initial experience. Eur Radiol 2007;17:1510-7. [Crossref] [PubMed]
- Kalisz K, Halliburton S, Abbara S, et al. Update on Cardiovascular Applications of Multienergy CT. Radiographics 2017;37:1955-74. [Crossref] [PubMed]
- McCollough CH, Leng S, Yu L, et al. Dual- and Multi-Energy CT: Principles, Technical Approaches, and Clinical Applications. Radiology 2015;276:637-53. [Crossref] [PubMed]
- Siegel MJ, Kaza RK, Bolus DN, et al. White Paper of the Society of Computed Body Tomography and Magnetic Resonance on Dual-Energy CT, Part 1: Technology and Terminology. J Comput Assist Tomogr 2016;40:841-5. [Crossref] [PubMed]
- Alvarez RE, Macovski A. Energy-selective reconstructions in X-ray computerized tomography. Phys Med Biol 1976;21:733-44. [Crossref] [PubMed]
- Lehmann LA, Alvarez RE, Macovski A, et al. Generalized image combinations in dual KVP digital radiography. Med Phys 1981;8:659-67. [Crossref] [PubMed]
- Petersilka M, Bruder H, Krauss B, et al. Technical principles of dual source CT. Eur J Radiol 2008;68:362-8. [Crossref] [PubMed]
- Koonce JD, Vliegenthart R, Schoepf UJ, et al. Accuracy of dual-energy computed tomography for the measurement of iodine concentration using cardiac CT protocols: validation in a phantom model. Eur Radiol 2014;24:512-8. [Crossref] [PubMed]
- Liu X, Yu L, Primak AN, et al. Quantitative imaging of element composition and mass fraction using dual-energy CT: three-material decomposition. Med Phys 2009;36:1602-9. [Crossref] [PubMed]
- Mendonca PR, Lamb P, Sahani DV. A Flexible Method for Multi-Material Decomposition of Dual-Energy CT Images. IEEE Trans Med Imaging 2014;33:99-116. [Crossref] [PubMed]
- Riederer SJ, Mistretta CA. Selective iodine imaging using K-edge energies in computerized X-ray tomography. Med Phys 1977;4:474-81. [Crossref] [PubMed]
- Fuld MK, Halaweish AF, Haynes SE, et al. Pulmonary perfused blood volume with dual-energy CT as surrogate for pulmonary perfusion assessed with dynamic multidetector CT. Radiology 2013;267:747-56. [Crossref] [PubMed]
- Vliegenthart R, Pelgrim GJ, Ebersberger U, et al. Dual-energy CT of the heart. AJR Am J Roentgenol 2012;199:S54-63. [Crossref] [PubMed]
- Gordic S, Puippe GD, Krauss B, et al. Correlation between Dual-Energy and Perfusion CT in Patients with Hepatocellular Carcinoma. Radiology 2016;280:78-87. [Crossref] [PubMed]
- Yu L, Leng S, McCollough CH. Dual-energy CT-based monochromatic imaging. AJR Am J Roentgenol 2012;199:S9-S15. [Crossref] [PubMed]
- Landry G, Reniers B, Granton PV, et al. Extracting atomic numbers and electron densities from a dual source dual energy CT scanner: experiments and a simulation model. Radiother Oncol 2011;100:375-9. [Crossref] [PubMed]
- Budoff MJ, Mayrhofer T, Ferencik M, et al. Prognostic Value of Coronary Artery Calcium in the PROMISE Study (Prospective Multicenter Imaging Study for Evaluation of Chest Pain). Circulation 2017;136:1993-2005. [Crossref] [PubMed]
- Chaikriangkrai K, Palamaner Subash Shantha G, Jhun HY, et al. Prognostic Value of Coronary Artery Calcium Score in Acute Chest Pain Patients Without Known Coronary Artery Disease: Systematic Review and Meta-analysis. Ann Emerg Med 2016;68:659-70. [Crossref] [PubMed]
- Yamak D, Pavlicek W, Boltz T, et al. Coronary calcium quantification using contrast-enhanced dual-energy computed tomography scans. J Appl Clin Med Phys 2013;14:4014. [Crossref] [PubMed]
- Agatston AS, Janowitz WR, Hildner FJ, et al. Quantification of coronary artery calcium using ultrafast computed tomography. J Am Coll Cardiol 1990;15:827-32. [Crossref] [PubMed]
- Schwarz F, Nance JW Jr, Ruzsics B, et al. Quantification of coronary artery calcium on the basis of dual-energy coronary CT angiography. Radiology 2012;264:700-7. [Crossref] [PubMed]
- McClelland RL, Chung H, Detrano R, et al. Distribution of coronary artery calcium by race, gender, and age: results from the Multi-Ethnic Study of Atherosclerosis (MESA). Circulation 2006;113:30-7. [Crossref] [PubMed]
- Yamada Y, Jinzaki M, Okamura T, et al. Feasibility of coronary artery calcium scoring on virtual unenhanced images derived from single-source fast kVp-switching dual-energy coronary CT angiography. J Cardiovasc Comput Tomogr 2014;8:391-400. [Crossref] [PubMed]
- Fuchs TA, Stehli J, Dougoud S, et al. Coronary artery calcium quantification from contrast enhanced CT using gemstone spectral imaging and material decomposition. Int J Cardiovasc Imaging 2014;30:1399-405. [Crossref] [PubMed]
- Nadjiri J, Kaissis G, Meurer F, et al. Accuracy of Calcium Scoring calculated from contrast-enhanced Coronary Computed Tomography Angiography using a dual-layer spectral CT: A comparison of Calcium Scoring from real and virtual non-contrast data. PLoS One 2018;13:e0208588. [Crossref] [PubMed]
- Mahoney R, Pavitt CW, Gordon D, et al. Clinical validation of dual-source dual-energy computed tomography (DECT) for coronary and valve imaging in patients undergoing trans-catheter aortic valve implantation (TAVI). Clin Radiol 2014;69:786-94. [Crossref] [PubMed]
- Song I, Yi JG, Park JH, et al. Virtual Non-Contrast CT Using Dual-Energy Spectral CT: Feasibility of Coronary Artery Calcium Scoring. Korean J Radiol 2016;17:321-9. [Crossref] [PubMed]
- De Cecco CN, Schoepf UJ, Steinbach L, et al. White Paper of the Society of Computed Body Tomography and Magnetic Resonance on Dual-Energy CT, Part 3: Vascular, Cardiac, Pulmonary, and Musculoskeletal Applications. J Comput Assist Tomogr 2017;41:1-7. [Crossref] [PubMed]
- Kalisz K, Buethe J, Saboo SS, et al. Artifacts at Cardiac CT: Physics and Solutions. Radiographics 2016;36:2064-83. [Crossref] [PubMed]
- De Santis D, Jin KN, Schoepf UJ, et al. Heavily Calcified Coronary Arteries: Advanced Calcium Subtraction Improves Luminal Visualization and Diagnostic Confidence in Dual-Energy Coronary Computed Tomography Angiography. Invest Radiol 2018;53:103-9. [Crossref] [PubMed]
- Andreini D, Pontone G, Mushtaq S, et al. Diagnostic Accuracy of Rapid Kilovolt Peak-Switching Dual-Energy CT Coronary Angiography in Patients With a High Calcium Score. JACC Cardiovasc Imaging 2015;8:746-8. [Crossref] [PubMed]
- Budoff MJ, Dowe D, Jollis JG, et al. Diagnostic performance of 64-multidetector row coronary computed tomographic angiography for evaluation of coronary artery stenosis in individuals without known coronary artery disease: results from the prospective multicenter ACCURACY (Assessment by Coronary Computed Tomographic Angiography of Individuals Undergoing Invasive Coronary Angiography) trial. J Am Coll Cardiol 2008;52:1724-32. [Crossref] [PubMed]
- Miller JM, Rochitte CE, Dewey M, et al. Diagnostic performance of coronary angiography by 64-row CT. N Engl J Med 2008;359:2324-36. [Crossref] [PubMed]
- Meijboom WB, Meijs MF, Schuijf JD, et al. Diagnostic accuracy of 64-slice computed tomography coronary angiography: a prospective, multicenter, multivendor study. J Am Coll Cardiol 2008;52:2135-44. [Crossref] [PubMed]
- Fearon WF, Tonino PA, De Bruyne B, et al. Rationale and design of the Fractional Flow Reserve versus Angiography for Multivessel Evaluation (FAME) study. Am Heart J 2007;154:632-6. [Crossref] [PubMed]
- Tonino PA, De Bruyne B, Pijls NH, et al. Fractional flow reserve versus angiography for guiding percutaneous coronary intervention. N Engl J Med 2009;360:213-24. [Crossref] [PubMed]
- Pijls NH, Fearon WF, Tonino PA, et al. Fractional flow reserve versus angiography for guiding percutaneous coronary intervention in patients with multivessel coronary artery disease: 2-year follow-up of the FAME (Fractional Flow Reserve Versus Angiography for Multivessel Evaluation) study. J Am Coll Cardiol 2010;56:177-84. [Crossref] [PubMed]
- Fearon WF, Bornschein B, Tonino PA, et al. Economic evaluation of fractional flow reserve-guided percutaneous coronary intervention in patients with multivessel disease. Circulation 2010;122:2545-50. [Crossref] [PubMed]
- Meijboom WB, Van Mieghem CA, van Pelt N, et al. Comprehensive assessment of coronary artery stenoses: computed tomography coronary angiography versus conventional coronary angiography and correlation with fractional flow reserve in patients with stable angina. J Am Coll Cardiol 2008;52:636-43. [Crossref] [PubMed]
- Nieman K, Balla S. Dynamic CT myocardial perfusion imaging. J Cardiovasc Comput Tomogr 2020;14:303-6. [Crossref] [PubMed]
- Pontone G, Baggiano A, Andreini D, et al. Dynamic Stress Computed Tomography Perfusion With a Whole-Heart Coverage Scanner in Addition to Coronary Computed Tomography Angiography and Fractional Flow Reserve Computed Tomography Derived. JACC Cardiovasc Imaging 2019;12:2460-71. [Crossref] [PubMed]
- Ruzsics B, Schwarz F, Schoepf UJ, et al. Comparison of dual-energy computed tomography of the heart with single photon emission computed tomography for assessment of coronary artery stenosis and of the myocardial blood supply. Am J Cardiol 2009;104:318-26. [Crossref] [PubMed]
- Schwarz F, Ruzsics B, Schoepf UJ, et al. Dual-energy CT of the heart--principles and protocols. Eur J Radiol 2008;68:423-33. [Crossref] [PubMed]
- Baumann S, Rutsch M, Becher T, et al. Clinical Impact of Rest Dual-energy Computed Tomography Myocardial Perfusion in Patients with Coronary Artery Disease. In Vivo 2017;31:1153-7. [PubMed]
- Nakahara T, Toyama T, Jinzaki M, et al. Quantitative Analysis of Iodine Image of Dual-energy Computed Tomography at Rest: Comparison With 99mTc-Tetrofosmin Stress-rest Single-photon Emission Computed Tomography Myocardial Perfusion Imaging as the Reference Standard. J Thorac Imaging 2018;33:97-104. [Crossref] [PubMed]
- Han R, Sun K, Lu B, et al. Diagnostic accuracy of coronary CT angiography combined with dual-energy myocardial perfusion imaging for detection of myocardial infarction. Exp Ther Med 2017;14:207-13. [Crossref] [PubMed]
- Meinel FG, De Cecco CN, Schoepf UJ, et al. First-arterial-pass dual-energy CT for assessment of myocardial blood supply: do we need rest, stress, and delayed acquisition? Comparison with SPECT. Radiology 2014;270:708-16. [Crossref] [PubMed]
- Ko SM, Choi JW, Song MG, et al. Myocardial perfusion imaging using adenosine-induced stress dual-energy computed tomography of the heart: comparison with cardiac magnetic resonance imaging and conventional coronary angiography. Eur Radiol 2011;21:26-35. [Crossref] [PubMed]
- Kim SM, Chang SA, Shin W, et al. Dual-energy CT perfusion during pharmacologic stress for the assessment of myocardial perfusion defects using a second-generation dual-source CT: a comparison with cardiac magnetic resonance imaging. J Comput Assist Tomogr 2014;38:44-52. [Crossref] [PubMed]
- Ko SM, Choi JW, Hwang HK, et al. Diagnostic performance of combined noninvasive anatomic and functional assessment with dual-source CT and adenosine-induced stress dual-energy CT for detection of significant coronary stenosis. AJR Am J Roentgenol 2012;198:512-20. [Crossref] [PubMed]
- Ko SM, Park JH, Hwang HK, et al. Direct comparison of stress- and rest-dual-energy computed tomography for detection of myocardial perfusion defect. Int J Cardiovasc Imaging 2014;30 Suppl 1:41-53. [Crossref] [PubMed]
- Ko SM, Song MG, Chee HK, et al. Diagnostic performance of dual-energy CT stress myocardial perfusion imaging: direct comparison with cardiovascular MRI. AJR Am J Roentgenol 2014;203:W605-13. [Crossref] [PubMed]
- Chung HW, Ko SM, Hwang HK, et al. Diagnostic Performance of Coronary CT Angiography, Stress Dual-Energy CT Perfusion, and Stress Perfusion Single-Photon Emission Computed Tomography for Coronary Artery Disease: Comparison with Combined Invasive Coronary Angiography and Stress Perfusion Cardiac MRI. Korean J Radiol 2017;18:476-86. [Crossref] [PubMed]
- Kido T, Watanabe K, Saeki H, et al. Adenosine triphosphate stress dual-source computed tomography to identify myocardial ischemia: comparison with invasive coronary angiography. Springerplus 2014;3:75. [Crossref] [PubMed]
- So A, Lee TY, Imai Y, et al. Quantitative myocardial perfusion imaging using rapid kVp switch dual-energy CT: preliminary experience. J Cardiovasc Comput Tomogr 2011;5:430-42. [Crossref] [PubMed]
- So A, Hsieh J, Narayanan S, et al. Dual-energy CT and its potential use for quantitative myocardial CT perfusion. J Cardiovasc Comput Tomogr 2012;6:308-17. [Crossref] [PubMed]
- Rodriguez-Granillo GA, Carrascosa P, Cipriano S, et al. Beam hardening artifact reduction using dual energy computed tomography: implications for myocardial perfusion studies. Cardiovasc Diagn Ther 2015;5:79-85. [PubMed]
- Carrascosa PM, Cury RC, Deviggiano A, et al. Comparison of myocardial perfusion evaluation with single versus dual-energy CT and effect of beam-hardening artifacts. Acad Radiol 2015;22:591-9. [Crossref] [PubMed]
- Carrascosa P, Deviggiano A, de Zan M, et al. Improved Discrimination of Myocardial Perfusion Defects at Low Energy Levels Using Virtual Monochromatic Imaging. J Comput Assist Tomogr 2017;41:661-7. [Crossref] [PubMed]
- Cannaò PM, Schoepf UJ, Muscogiuri G, et al. Technical prerequisites and imaging protocols for dynamic and dual energy myocardial perfusion imaging. Eur J Radiol 2015;84:2401-10. [Crossref] [PubMed]
- van Hamersvelt RW, Isgum I, de Jong PA, et al. Application of speCtraL computed tomogrAphy to impRove specIficity of cardiac compuTed tomographY (CLARITY study): rationale and design. BMJ Open 2019;9:e025793. [Crossref] [PubMed]
- Boll DT, Hoffmann MH, Huber N, et al. Spectral coronary multidetector computed tomography angiography: dual benefit by facilitating plaque characterization and enhancing lumen depiction. J Comput Assist Tomogr 2006;30:804-11. [Crossref] [PubMed]
- Scheske JA, O'Brien JM, Earls JP, et al. Coronary artery imaging with single-source rapid kilovolt peak-switching dual-energy CT. Radiology 2013;268:702-9. [Crossref] [PubMed]
- Ohta Y, Kitao S, Watanabe T, et al. Evaluation of image quality of coronary artery plaque with rapid kVp-switching dual-energy CT. Clin Imaging 2017;43:42-9. [Crossref] [PubMed]
- Boll DT, Merkle EM, Paulson EK, et al. Calcified vascular plaque specimens: assessment with cardiac dual-energy multidetector CT in anthropomorphically moving heart phantom. Radiology 2008;249:119-26. [Crossref] [PubMed]
- Symons R, Choi Y, Cork TE, et al. Optimized energy of spectral coronary CT angiography for coronary plaque detection and quantification. J Cardiovasc Comput Tomogr 2018;12:108-14. [Crossref] [PubMed]
- Arendt CT, Czwikla R, Lenga L, et al. Improved coronary artery contrast enhancement using noise-optimised virtual monoenergetic imaging from dual-source dual-energy computed tomography. Eur J Radiol 2020;122:108666. [Crossref] [PubMed]
- Stehli J, Clerc OF, Fuchs TA, et al. Impact of monochromatic coronary computed tomography angiography from single-source dual-energy CT on coronary stenosis quantification. J Cardiovasc Comput Tomogr 2016;10:135-40. [Crossref] [PubMed]
- Boll DT, Merkle EM, Paulson EK, et al. Coronary stent patency: dual-energy multidetector CT assessment in a pilot study with anthropomorphic phantom. Radiology 2008;247:687-95. [Crossref] [PubMed]
- Halpern EJ, Halpern DJ, Yanof JH, et al. Is coronary stent assessment improved with spectral analysis of dual energy CT? Acad Radiol 2009;16:1241-50. [Crossref] [PubMed]
- Stehli J, Fuchs TA, Singer A, et al. First experience with single-source, dual-energy CCTA for monochromatic stent imaging. Eur Heart J Cardiovasc Imaging 2015;16:507-12. [Crossref] [PubMed]
- Mangold S, Cannao PM, Schoepf UJ, et al. Impact of an advanced image-based monoenergetic reconstruction algorithm on coronary stent visualization using third generation dual-source dual-energy CT: a phantom study. Eur Radiol 2016;26:1871-8. [Crossref] [PubMed]
- Mehran R, Dangas GD, Weisbord SD. Contrast-Associated Acute Kidney Injury. N Engl J Med 2019;380:2146-55. [Crossref] [PubMed]
- Davenport MS, Perazella MA, Yee J, et al. Use of Intravenous Iodinated Contrast Media in Patients with Kidney Disease: Consensus Statements from the American College of Radiology and the National Kidney Foundation. Radiology. 2020;294:660-8. [Crossref] [PubMed]
- Raju R, Thompson AG, Lee K, et al. Reduced iodine load with CT coronary angiography using dual-energy imaging: a prospective randomized trial compared with standard coronary CT angiography. J Cardiovasc Comput Tomogr 2014;8:282-8. [Crossref] [PubMed]
- Carrascosa P, Leipsic JA, Capunay C, et al. Monochromatic image reconstruction by dual energy imaging allows half iodine load computed tomography coronary angiography. Eur J Radiol 2015;84:1915-20. [Crossref] [PubMed]
- Narula J, Nakano M, Virmani R, et al. Histopathologic characteristics of atherosclerotic coronary disease and implications of the findings for the invasive and noninvasive detection of vulnerable plaques. J Am Coll Cardiol 2013;61:1041-51. [Crossref] [PubMed]
- Hoffmann U, Moselewski F, Nieman K, et al. Noninvasive assessment of plaque morphology and composition in culprit and stable lesions in acute coronary syndrome and stable lesions in stable angina by multidetector computed tomography. J Am Coll Cardiol 2006;47:1655-62. [Crossref] [PubMed]
- Kashiwagi M, Tanaka A, Kitabata H, et al. Feasibility of noninvasive assessment of thin-cap fibroatheroma by multidetector computed tomography. JACC Cardiovasc Imaging 2009;2:1412-9. [Crossref] [PubMed]
- Maurovich-Horvat P, Hoffmann U, Vorpahl M, et al. The napkin-ring sign: CT signature of high-risk coronary plaques? JACC Cardiovasc Imaging 2010;3:440-4. [Crossref] [PubMed]
- Ferencik M, Mayrhofer T, Bittner DO, et al. Use of High-Risk Coronary Atherosclerotic Plaque Detection for Risk Stratification of Patients With Stable Chest Pain: A Secondary Analysis of the PROMISE Randomized Clinical Trial. JAMA Cardiol 2018;3:144-52. [Crossref] [PubMed]
- Williams MC, Moss AJ, Dweck M, et al. Coronary Artery Plaque Characteristics Associated With Adverse Outcomes in the SCOT-HEART Study. J Am Coll Cardiol 2019;73:291-301. [Crossref] [PubMed]
- Zachrisson H, Engstrom E, Engvall J, et al. Soft tissue discrimination ex vivo by dual energy computed tomography. Eur J Radiol 2010;75:e124-8. [Crossref] [PubMed]
- Barreto M, Schoenhagen P, Nair A, et al. Potential of dual-energy computed tomography to characterize atherosclerotic plaque: ex vivo assessment of human coronary arteries in comparison to histology. J Cardiovasc Comput Tomogr 2008;2:234-42. [Crossref] [PubMed]
- Henzler T, Porubsky S, Kayed H, et al. Attenuation-based characterization of coronary atherosclerotic plaque: comparison of dual source and dual energy CT with single-source CT and histopathology. Eur J Radiol 2011;80:54-9. [Crossref] [PubMed]
- Tanami Y, Ikeda E, Jinzaki M, et al. Computed tomographic attenuation value of coronary atherosclerotic plaques with different tube voltage: an ex vivo study. J Comput Assist Tomogr 2010;34:58-63. [Crossref] [PubMed]
- Obaid DR, Calvert PA, Gopalan D, et al. Dual-energy computed tomography imaging to determine atherosclerotic plaque composition: a prospective study with tissue validation. J Cardiovasc Comput Tomogr 2014;8:230-7. [Crossref] [PubMed]
- Haghighi RR, Chatterjee S, Tabin M, et al. DECT evaluation of noncalcified coronary artery plaque. Med Phys 2015;42:5945-54. [Crossref] [PubMed]
- Mandal SR, Bharati A, Haghighi RR, et al. Non-invasive characterization of coronary artery atherosclerotic plaque using dual energy CT: Explanation in ex-vivo samples. Phys Med 2018;45:52-8. [Crossref] [PubMed]
- Yamak D, Panse P, Pavlicek W, et al. Non-calcified coronary atherosclerotic plaque characterization by dual energy computed tomography. IEEE J Biomed Health Inform 2014;18:939-45. [Crossref] [PubMed]
- Willemink MJ, Persson M, Pourmorteza A, et al. Photon-counting CT: Technical Principles and Clinical Prospects. Radiology 2018;289:293-312. [Crossref] [PubMed]
- Bratke G, Hickethier T, Bar-Ness D, et al. Spectral Photon-Counting Computed Tomography for Coronary Stent Imaging: Evaluation of the Potential Clinical Impact for the Delineation of In-Stent Restenosis. Invest Radiol 2020;55:61-7. [Crossref] [PubMed]
- Shikhaliev PM. Computed tomography with energy-resolved detection: a feasibility study. Phys Med Biol 2008;53:1475-95. [Crossref] [PubMed]
- Alessio AM, MacDonald LR. Quantitative material characterization from multi-energy photon counting CT. Med Phys 2013;40:031108. [Crossref] [PubMed]
- Boussel L, Coulon P, Thran A, et al. Photon counting spectral CT component analysis of coronary artery atherosclerotic plaque samples. Br J Radiol 2014;87:20130798. [Crossref] [PubMed]
- Bhavane R, Badea C, Ghaghada KB, et al. Dual-energy computed tomography imaging of atherosclerotic plaques in a mouse model using a liposomal-iodine nanoparticle contrast agent. Circ Cardiovasc Imaging 2013;6:285-94. [Crossref] [PubMed]