Effectiveness of magnetocardiography to identify patients in need of coronary artery revascularization: a cross-sectional study
Introduction
Patients with angina-like symptoms need further examination to determine whether revascularization is necessary. Several approaches have been attempted to predict the necessary of revascularization, such as single-photon emission computed tomography (SPECT), cardiac magnetic resonance imaging (CMRI) and coronary computed tomography angiography (CCTA) and percutaneous coronary angiography (PCA). However, these techniques are difficult to adapt to routine cardiological diagnosis in the general population, which is due to limitations caused by high cost, long examination time, side-effect of iodine contrast agent and radiation hazard. Coronary angiography is an important criterion to determine the need for revascularization. For patients in need of revascularization, undergoing CCTA may delay the treatment and increase the exposure to contrast agents and radiation. PCA is not that suitable for those who don’t need treatment with revascularization, because of the expensiveness and invasiveness. Therefore, a non-invasive, inexpensive, non-radiative diagnostic technique is needed to identify patients who should undergo coronary artery revascularization.
Magnetocardiography (MCG) has been proposed a non-invasive, radiation-free and quick-testing technique to record cardiac magnetic field with high reproducibility (1,2). Magnetic measurements are non-invasive and non-contact, meaning that the magnetic signals detected by MCG are less influenced by body tissues, compared to electric currents detected by electrocardiography (ECG). Therefore, artefacts from unreliable and/or fluctuating electrode-skin contacts, such as in practical ECG, cannot occur (1). Recently, it has been reported that MCG has shown high performance in diagnosing coronary artery disease (CAD) (3-6). Typical parameters useful for CAD diagnosis were current angle, field map angle, pole distance, QTc dispersion and non-dipolar phenomenon in the magnetic field maps (MFMs) (5-7). Although MCG could record spatial and temporal magnetic field signals during repolarization period at the same time, those parameters above couldn’t reflect the spatial and temporal information together. A parameter which contains both spatial and temporal magnetic field information might be more useful in CAD diagnosis.
Myocardial ischemia causes regional ventricular repolarization abnormalities by altering resting membrane potential, reducing membrane excitability, shortening action potential, reducing conduction velocity and prolonging refractory period beyond repolarization in ischemic zone (8-10). Previous studies have shown a parameter of smoothness index derived from the differences of QTc dispersion of spatially nearest neighbors (11). In this study, we introduced a new diagnosis model based on MCG equipment that is able to quantify the uniformity of ventricular repolarization at both spatial and temporal scales, and aimed to evaluate its diagnostic value to identify patients in need of revascularization. We present the following article in accordance with the Strengthening the Reporting of Observational Studies in Epidemiology (STROBE) reporting checklist (available at http://dx.doi.org/10.21037/cdt-20-121).
Methods
Study design and participants
This single center cross-sectional study was approved by hospital ethics committee of the 8th Medical Center of Chinese PLA General Hospital (No. 2016ST008). It was conducted in accordance with the Declaration of Helsinki (amended by the 64th WMA General Assembly, Fortaleza, Brazil, in 2013). Written informed consents were obtained from the patients for publication of this study and any accompanying images. A copy of the written consent is available for review by the Editor-in-Chief of this journal. Between July 27, 2015 and April 10, 2017, hospitalized patients admitted from outpatient department with an indication for coronary angiography due to angina-like symptoms and without a prior history of CAD were recruited consecutively in this study. Angina-like symptoms were defined as crushing, gripping, tight, dull, burning or heavy chest discomfort or pain, which associated with exertion or emotional stress and relieved within about 5 to 20 minutes by rest. Exclusion criteria were ST-segment elevation myocardial infarction (MI) or other cardiac events requiring emergency percutaneous coronary intervention (PCI) treatment, malignant neoplasms, structural heart diseases, valvular heart diseases, cardiomyopathies, malignant arrhythmia (such as atrial fibrillation, atrial flutter and ventricular arrhythmia), and previous revascularization. Because metal materials could affect the results of the MCG examinations, patients who had ever received implantation of metal foreign body, such as pacemaker implantation, bone fracture internal fixation or implantation of metal dentures were also excluded from this study. After enrollment, 12-lead ECGs were performed, and significant ischemia was defined as T-wave inversion, ST elevation or ST depression in adjacent two or more than two leads. Simultaneous recordings of MCG and lead-II of ECG were performed in a standardized schedule before PCA and revascularization. Clinical characteristic data, such as age, gender, history of hypertension, diabetes mellitus and smoking, family history of CAD, full blood count, brain natriuretic peptide, troponin, serum creatinine and uric acid and lipid profiles, were also collected for all participants after enrollment.
MCG recordings scanning procedure
An unshielded 4-channel MCG system (MD-U041001, Shanghai MEDI Instruments Ltd.) was used to perform the MCG recordings (Figure 1). In brief, patient lay in the supine position and the arrayed sensors of superconducting quantum interference device (SQUID) positioned close to, but not in contact with chest wall. Each subject underwent a continuous recording at 9 adjacent positions containing 36 locations (6×6 grid) above the chest for a total recording time of 60 s ×9=540 s. MCG recordings were carried out at rest for 30 s, at a sampling rate of 1,000 Hz. The continuous MCG signals were averaged using 80 to 110 heart beats for each subject. The mean global field power was estimated on the averaged signals across 36 channels. Data between T-wave onset to T-wave end were subsequently obtained from the mean global field according to curves of lead-II of ECG. Finally, magnetic field intensity values from each channel were extracted at every single sampling time point. A typical result of MCG magnetic field map was shown in Figure 2.
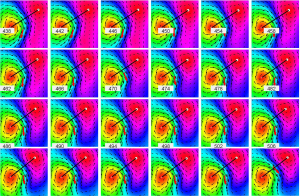
Procedure of PCA and revascularization
A diagnostic coronary angiography was performed for each patient using standard techniques. And multiple projections of coronary arteries were recorded digitally. All angiography was examined by two experienced cardiac intervention doctors. Significant stenosis was defined as at least 70% stenosis in at least one major epicardial coronary artery. The appropriateness of revascularization was determined by two experienced cardiac intervention physicians. The procedures of revascularization followed both the American Heart Association (AHA)’s and Chinese guidelines for PCI. If coronary bypass surgery was necessary, the patient would be recommended to be referred to cardiac surgery ward. Patients were divided into two groups based on whether they needed revascularization.
Calculation of Pearson’s correlation coefficients
According to the locations of detecting channels and inspection sequence, the channels of the MCG were defined as C01 to C36 (Figure S1). For each subject, T waves were paired with each other and expressed as Cmvs. Cn, which meant that there were a total of 36× (36−1) ÷2=630 pairs (Figure S2). The value at every single sampling point was extracted from T wave of each channel in time sequence. After that, bivariate correlation analysis was applied to calculate the Pearson’s correlation coefficient of each pair by IBM SPSS version 20.0. Finally, these coefficients were used as covariates to further establish the binary logistic regression model (Figure 3).
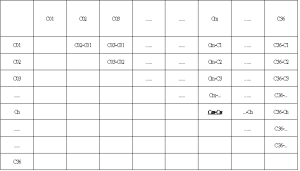
Establishment of binary logistic regression diagnosis model
According to the necessity of revascularization, the subjects were divided into revascularization group and control group. First, Pearson’s correlation coefficients were compared between two groups. If the p values were less than 0.05, the corresponding pairs were chosen and further analyzed by receiver operating characteristic (ROC) curve. If the asymptotic P values were less than 0.05, the corresponding pairs were entered into the binary logistic regression analysis.
Statistical analysis
The sample size was estimated based on test of one ROC curve using a PASS 11 software, at significance level of 5%, power of 90%, area under the ROC curve (AUC)|H0 of 0.7 and AUC|H1 of 0.5. Data analysis were performed using IBM SPSS version 20.0. Data were presented as mean and standard deviation (SD). Independent-samples Student’s t-tests were used to compare Pearson’s correlation coefficients between revascularization group and control group. In the logistic regression model, the classification cut-off was assigned a value of 0.5, maximum iteration was a value of 50, the method of stepwise was set as backward [likelihood ratio (LR)] and the entry and removal probabilities of stepwise were 0.01 and 0.04, respectively. ROC curve analysis was used to determine the diagnostic value of the binary logistic regression model in identifying patients who needed revascularization. Cut-off value was determined by max Youden index. Chi-square tests were used to detect differences in proportions of the two groups. Sensitivity, specificity, positive predictive value (PPV), negative predictive value (NPV) and positive and negative LRs (+/− LR) were calculated. For all analysis, differences with P<0.05 were considered significant.
Results
Participants
Thirteen patients who needed emergency PCI surgery for onset of severe chest pain after admitted in hospital were excluded from this study. Three patients were excluded from this study due to severe mitral stenosis (n=1), moderate aortic valve regurgitation (n=1) and severe tricuspid regurgitation (n=1). Out of the remaining 203 patients enrolled in this study, 85 did not require revascularization (group I) and 118 required (group II). There were significant differences in proportions of gender, family history of CAD and acute myocardial infarction (AMI) between the two group. Thirty-three were diagnosed with AMI with TnI levels higher than 0.5 ng/mL after enrollment, out of which 2 (2.4%) in group I and 31 (26.3%) in group II. Details were listed in Table 1.
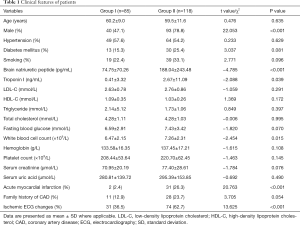
Full table
Pearson’s correlation coefficients
There were 93 pairings of Pearson’s correlation coefficients were significantly different between the two groups, 30 of which were lower in group I, compared to group II. The mean and SD of those correlation coefficients were listed. ROC curve analysis was performed for the 93 pairings, and asymptotic P values were less than 0.05 in 79 pairings (Table S1).

Full table
Binary logistic regression model
Binary logistic regression analysis was done using backward method. Finally, ten pairings were entered into diagnostic regression model as covariates. The summary after logistic regression analysis about the covariates was detailed in Table 2. The fitted model was established:
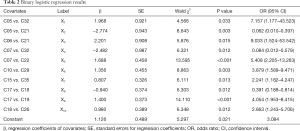
Full table
Z =1/(1+ e−N), N =1.968X1 − 2.774X2 + 2.201X3 − 2.482X4 + 1.688X5 + 1.356X6 + 0.807X7 − 0.940X8 + 1.400X9 + 0.980X10 + 1.126
The ROC curve was drawn (Figure 4) and the AUC was 0.747 (95% CI: 0.680–0.815) with an asymptotic P value less than 0.001. When the cut-off value was set as 0.550, according to the best Youden index of 0.388, 29 were positive in group I, and 86 were positive in group II at MCG. The regression model had an accuracy of 69.9%, a sensitivity of 72.9%, a specificity of 65.9%, a PPV of 74.8% and a NPV of 63.6%, while the positive and negative LRs were 2.138 and 0.441, with corresponding post-test probabilities of 68.1% and 29.1%. 16 were positive in group I, and 74 were positive in group II at ECG. And the accuracy, sensitivity, specificity, PPV and NPV for 12-lead ECG were 67.0%, 62.7%, 63.5%, 70.5% and 55.1%, respectively. However, when those AMI patients were rule out from both groups, the MCG model had an accuracy of 68.2%, a sensitivity of 70.1%, a specificity of 66.3%, a PPV of 68.5% and an NPV of 67.9%. But, the accuracy, sensitivity, specificity, PPV and NPV for 12-lead ECG were 60.0%, 55.2%, 65.1%, 62.3% and 58.1%, respectively (Table 3).
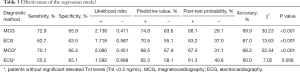
Full table
Discussion
In the present study, bivariate correlation analysis and Pearson’s correlation coefficients were used to quantify the heterogeneities of myocardial repolarization at different sites where the T-wave signals were detected. Out of 630 pairings of T-wave curves, Pearson’s correlation coefficients of 93 were significantly different between the two group. Based on the Pearson’s correlation coefficients, a binary logistic regression model was established, which was efficient to identify the patients who needed revascularization in those with angina -like symptoms.
Myocardial ischaemia causes regional ventricular repolarization abnormalities, which can be identified by S-T segment shift or T wave inversion in ECG. Cohen and coworkers demonstrated the unique potential of MCG for the study of acute myocardial ischaemia was provided in the 1970s using experimental MCG measurements to reveal changes related to ischemic injury currents that were not detected by ECG (12,13). For half a century, MCG has been expected to provide diagnostic information on cardiac activity and has been found to be more accurate for the evaluation of MI and abnormal ventricular repolarization than ECG (4,7,14). Although there is no clear standardization for MCG to diagnose CAD. Several MCG parameters calculated along the ST interval and T-wave were typically abnormal at rest in patients with CAD, and appear to be sensitive diagnostic parameters (3,5,6,11,15-17).
In previous studies, a non-dipolar phenomenon was observed on cardiac MFMs after ST-elevated and non-ST-elevated MI (18,19). This phenomenon has also been found in post-MI patients (20). Lim and his colleagues classified abnormal MFM patterns into 4 different types (compressed, stretched, broken, and rotated pole) and found a significant correlation between the abnormal repolarization patterns at T-peak and myocardial ischaemia (7). In their study, 10 characteristic parameters of MFM patterns were analyzed, including angle and amplitude. MFM patterns strongly associated with the number of abnormal parameters. The more the total number, the worse the MFM patterns. Bang et al. categorized all types of abnormal MFM patterns as a non-dipole pattern and confirmed these two types of MFM patterns in the repolarization phase through analysis of MCG findings from MI patients (5). However, the explanation of this non-dipolar phenomenon is still missing, which makes it difficult for clinicians to understand the results and make further treatment decisions, and might limit the application of MCG.
QT dispersion (QTd) and smoothness index have also proved useful in CAD diagnosis (11). It has been suggested that QTd reflected regional variations in ventricular repolarization and therefore might represent overall variability in repolarization timing (14). Taking the spatial distribution of QT intervals into account was helpful to distinguish CAD patients and non-CAD patients (21). Compared to healthy people, the spatial distribution of QT intervals in CAD patients showed greater dispersion, greater local variability, and a change in overall MCG pattern (22). Thus, examination of the spatial information gave us an insight into the heterogeneity of the repolarization process as registered on the body surface.
In this study, we investigated the heterogeneity of the repolarization process by comparing each two T-waves by bivariate correlation analysis. Of the 630 pairings, only 93 showed significant differences between the two groups. These Pearson’s correlation coefficients, containing both spatial and temporal cardiac magnetic information reflecting the non-uniformity of cardiac repolarization, could quantify the repolarization heterogeneity. The smaller the coefficient, the more heterogeneous the two curves. The results suggested that the uniformity of myocardial repolarization changed in patients who needed revascularization, which might be an explanation of the non-dipolar phenomenon. And these Pearson’s correlation coefficients might be able to identify patients in need of revascularization.
To further investigate the efficacy of these Pearson’s correlation coefficients in distinguishing between patients who needed revascularization or not, a logistic regression model was established. Ten covariates were included into the model. The predictive probability of the formula is easy to calculated and expected to recommend further examinations (e.g., PCA or CCTA). At the cut-off value of 0.55, the sensitivity, specificity, PPV, NPV, and accuracy were 72.9%, 65.9%, 74.8%, 63.6% and 69.9%, and the positive and negative post-test probabilities were 65.9% and 25.7%. According to the regression model, 56 of 85 patients in this study could have been avoided unnecessary invasive angiography. This diagnostic method could facilitate the diagnosis process and avoid unnecessary invasive angiography. As the presence of chest pain with elevated troponin I (TnI) levels is a potent indication of PCA, we evaluated the efficacy of this model to identify patients requiring revascularization in non-AMI patients. The sensitivity of MCG was higher than that of ECG (70.1% vs. 55.2%), whereas the specificity was similar to ECG (66.3% vs. 65.1%). This suggested that this MCG model was also helpful in identifying patients requiring revascularization from chest pain patients without MI.
Study limitation
There are some limitations that should be acknowledged. This study investigated a population with angina-like symptoms and indicated to undergo PCA, but the proportions of severity in coronary lesions and the location of coronary lesions were not considered. Therefore, further studies are needed to evaluate the relationship between the diagnostic model and coronary lesions in a study of large population. The specificity was only 65.9%, which might be due to the number of covariates included into the model was restricted by the number of patients, statistically. Including more covariates might be able to increase the specificity of the regression model in a study of large population.
Despite these limitations, we demonstrated the presence of myocardial repolarization heterogeneity in CAD patients and quantified the heterogeneity via comparing T-waves curves detected by MCG with a bivariate correlation analysis.
Conclusions
This study suggested that patients suffering from angina-like symptoms, with a logistic regression model value over 0.55, should be recommended for PCA.
Acknowledgments
Thanks for the helps from Hao Chen, Pengfei Chen, Chunxia Di, Lejian Lin, Hailong Zhu and Chuang Zhang, who are working in the 8th Medical Center of Chinese PLA General Hospital.
Funding: We gratefully acknowledge the financial supports by the clinical research project (Serial Number: Z161100000516177) supported by Beijing Municipal & Technology Commission.
Footnote
Reporting Checklist: The authors present the study in accordance with the STROBE reporting checklist. Available at http://dx.doi.org/10.21037/cdt-20-121
Data Sharing Statement: Available at http://dx.doi.org/10.21037/cdt-20-121
Peer Review File: Available at http://dx.doi.org/10.21037/cdt-20-121
Conflicts of Interest: All authors have completed the ICMJE uniform disclosure form (available at http://dx.doi.org/10.21037/cdt-20-121). The authors have no conflicts of interest to declare.
Ethical Statement: The authors are accountable for all aspects of the work in ensuring that questions related to the accuracy or integrity of any part of the work are appropriately investigated and resolved. This single center cross-sectional study was approved by hospital ethics committee of the 8th medical center of Chinese PLA general hospital (NO.: 2016ST008). It was conducted in accordance with the Declaration of Helsinki (amended by the 64th WMA General Assembly, Fortaleza, Brazil, in 2013). Written informed consents were obtained from the patients for publication of this study and any accompanying images. A copy of the written consent is available for review by the Editor-in-Chief of this journal.
Open Access Statement: This is an Open Access article distributed in accordance with the Creative Commons Attribution-NonCommercial-NoDerivs 4.0 International License (CC BY-NC-ND 4.0), which permits the non-commercial replication and distribution of the article with the strict proviso that no changes or edits are made and the original work is properly cited (including links to both the formal publication through the relevant DOI and the license). See: https://creativecommons.org/licenses/by-nc-nd/4.0/.
References
- Koch H. Recent advances in magnetocardiography. J Electrocardiol 2004;37 Suppl:117-122. [Crossref] [PubMed]
- Sorbo AR, Lombardi G, La Brocca L, et al. Unshielded magnetocardiography: Repeatability and reproducibility of automatically estimated ventricular repolarization parameters in 204 healthy subjects. Ann Noninvasive Electrocardiol 2018;23:e12526. [Crossref] [PubMed]
- Wu YW, Lee CM, Liu YB, et al. Usefulness of magnetocardiography to detect coronary artery disease and cardiac allograft vasculopathy. Circ J 2013;77:1783-90. [Crossref] [PubMed]
- Li Y, Che Z, Quan W, et al. Diagnostic outcomes of magnetocardiography in patients with coronary artery disease. Int J Clin Exp Med 2015;8:2441-6. [PubMed]
- Bang WD, Kim K, Lee YH, et al. Repolarization Heterogeneity of Magnetocardiography Predicts Long-Term Prognosis in Patients with Acute Myocardial Infarction. Yonsei Med J 2016;57:1339-46. [Crossref] [PubMed]
- Shin ES, Lam YY, Her AY, et al. Incremental diagnostic value of combined quantitative and qualitative parameters of magnetocardiography to detect coronary artery disease. Int J Cardiol 2017;228:948-52. [Crossref] [PubMed]
- Lim HK, Kwon H, Chung N, et al. Usefulness of magnetocardiogram to detect unstable angina pectoris and non-ST elevation myocardial infarction. Am J Cardiol 2009;103:448-54. [Crossref] [PubMed]
- Lazzara R, Scherlag BJ. Electrophysiologic basis for arrhythmias in ischemic heart disease. Am J Cardiol 1984;53:1B-7B. [Crossref] [PubMed]
- Taggart P, Sutton PM, Boyett MR, et al. Human ventricular action potential duration during short and long cycles. Rapid modulation by ischemia. Circulation 1996;94:2526-34. [Crossref] [PubMed]
- Stinstra JG, Shome S, Hopenfeld B, et al. Modelling passive cardiac conductivity during ischaemia. Med Biol Eng Comput 2005;43:776-82. [Crossref] [PubMed]
- Wu YW, Lin LC, Tseng WK, et al. QTc Heterogeneity in Rest Magnetocardiography is Sensitive to Detect Coronary Artery Disease: In Comparison with Stress Myocardial Perfusion Imaging. Acta Cardiol Sin 2014;30:445-54. [PubMed]
- Cohen D, Norman JC, Molokhia F, et al. Magnetocardiography of direct currents: S-T segment and baseline shifts during experimental myocardial infarction. Science 1971;172:1329-33. [Crossref] [PubMed]
- Cohen D, Kaufman LA. Magnetic determination of the relationship between the S-T segment shift and the injury current produced by coronary artery occlusion. Circ Res 1975;36:414-24. [Crossref] [PubMed]
- Higham PD, Furniss SS, Campbell RW. QT dispersion and components of the QT interval in ischaemia and infarction. Br Heart J 1995;73:32-6. [Crossref] [PubMed]
- Park JW, Leithäuser B, Vrsansky M, et al. Dobutamine stress magnetocardiography for the detection of significant coronary artery stenoses - a prospective study in comparison with simultaneous 12-lead electrocardiography. Clin Hemorheol Microcirc 2008;39:21-32. [Crossref] [PubMed]
- Wu Y, Gu J, Chen T, et al. Noninvasive diagnosis of coronary artery disease using two parameters extracted in an extrema circle of magnetocardiogram. Conf Proc IEEE Eng Med Biol Soc 2013;2013:1843-6.
- Park JW, Shin ES, Ann SH, et al. Validation of magnetocardiography versus fractional flow reserve for detection of coronary artery disease. Clin Hemorheol Microcirc 2015;59:267-81. [Crossref] [PubMed]
- Kyoon Lim H, Kim K, Lee YH, et al. Detection of non-ST-elevation myocardial infarction using magnetocardiogram: new information from spatiotemporal electrical activation map. Ann Med 2009;41:533-46. [Crossref] [PubMed]
- Van Leeuwen P, Hailer B, Beck A, et al. Changes in dipolar structure of cardiac magnetic field maps after ST elevation myocardial infarction. Ann Noninvasive Electrocardiol 2011;16:379-87. [Crossref] [PubMed]
- Ikefuji H, Nomura M, Nakaya Y, et al. Visualization of cardiac dipole using a current density map: detection of cardiac current undetectable by electrocardiography using magnetocardiography. J Med Invest 2007;54:116-23. [Crossref] [PubMed]
- Hailer B, Van Leeuwen P, Lange S, et al. Spatial distribution of QT dispersion measured by magnetocardiography under stress in coronary artery disease. J Electrocardiol 1999;32:207-16. [Crossref] [PubMed]
- Van Leeuwen P, Hailer B, Lange S, et al. Spatial distribution of repolarization times in patients with coronary artery disease. Pacing Clin Electrophysiol 2003;26:1706-14. [Crossref] [PubMed]